Final Exam Notes - Investigating Politics
Document Details
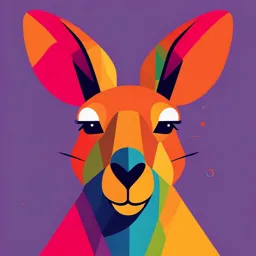
Uploaded by BestKnownFortWorth
University of British Columbia
Tags
Summary
These notes cover concepts, variables, measures, dimensions of a concept and levels of measurement in political science. They are for investigating Politics, an introduction to Scientific Political Analysis.
Full Transcript
lOMoARcPSD|50424300 Final exam notes Investigating Politics: An Introduction To Scientific Political Analysis (The University of British Columbia) Scan to open on Studocu...
lOMoARcPSD|50424300 Final exam notes Investigating Politics: An Introduction To Scientific Political Analysis (The University of British Columbia) Scan to open on Studocu Studocu is not sponsored or endorsed by any college or university Downloaded by Annie Marcovitz ([email protected]) lOMoARcPSD|50424300 Concepts An abstract definition for characteristics of or types of phenomena, groups or individuals Variable A measurable property of a phenomenon, group, person that can potentially take on different values o Derived to capture a concept o Variation across cases or over time Measure A procedure for determining whether or to what degree a concept applies to specific cases based on observation of those cases Dimensions of a concept “Sub-concepts” Intrinsic parts/components of a concept that are independent of one another and are neither causes nor consequences of the concept Four Levels of Measurement Nominal o Categorical in nature o Place cases into discrete groups based on presence/absence of attribute o No category is ranked higher or lower than another o Categories are exhaustive o E.g. religion, partisan affiliation, type of electoral system, occupation Ordinal o Categorical in nature o Categories are ranked, may have a number attached o Cases can be said to have more or less of something o Intervals between categories not meaningful o Relative levels, not absolute level o E.g. university rankings, test score percentiles, ideology, level of education Interval o Numerical in nature o Intervals between values are meaningful and consistent o Difference in values indicates how much more or less of something one case is from another o No meaningful zero point Downloaded by Annie Marcovitz ([email protected]) lOMoARcPSD|50424300 o E.g. years (not years since event), current events quiz score, temperature (Celsius) Ratio o Numerical in nature o Zero is meaningful (indicates absence) o E.g. years since some event, age, counts of events, rates (unemployment, language spoken political party preferences) Independent variable A variable that captures the purported cause of the causal claim (X) Dependent variable A variable that captures the purported outcome of the causal claim (Y) Validity How well a measurement captures a concept When the variable/measure has validity, the variable/measure captures the concept well, while when the variable/measure lacks validity, it either captures too much of the concept or too little of the concept* Measures or variables might lack validity because the measure does not cover enough of the concept, the measure captures things outside the concept or the measure captures different things across units (non-comparability) Reliability How consistently a measurement procedure produces the same result, when repeated for the same case When the variable/measure has reliability, results produced are consistent to the concept(?) every time, and the results would be inconsistent if the variable/measure lacked reliability Measures or variables might lack reliability because there is room for researcher interpretation, procedure for measurement is imprecise or if the measurement is unstable over time Measurement Error Refers to weak validity or weak reliability The two types of measurement error are measurement bias (systematic measurement error) and random measurement error Measurement Bias (Systematic Measurement Error) Error that is produced when our measurement procedure obtains scores that are, on average, too high or too low Downloaded by Annie Marcovitz ([email protected]) lOMoARcPSD|50424300 o Can have upward or downward bias o Even if you repeat the measurement, the value is still too high or too low o Does not refer to political bias o Might apply to all cases or just to certain subgroups This is different from random measurement error because it is related to validity rather than reliability, it is related to another concept, there is an unknown change in results and it is hard to fix, and it is acceptable if it’s uniform across cases Sources of measurement bias include researcher subjectivity/interpretation, gap between concept and measure and obstacle to observation Upward Bias When cases have extraneous features Downward Bias When cases lack extraneous features Random Measurement Error Derives from random features of measurement process or phenomenon o We get a value that is too high or too low by chance o No systematic tilt one way or another This is different from measurement bias because it is related to reliability rather than validity, it is unrelated to other concepts or related to an unrelated concept, it finds no relationship even if there is one (known change in results), it is easier to fix (can cancel out with many trials unlike with measurement bias) and it is acceptable when false negative is better than false positive Sources of random measurement error include imperfect memory, “random” changes in mood/concerns, counting errors and researcher interpretation Sampling A population is the full set of cases we’re interested in learning about A sample is a subset of the population that we observe and measure Inferences are the descriptions that we make about the population based on a sample o We need inferences because it is impossible to survey an entire population How are population, sample and inferences related?** Random sampling is the selection of cases from the population in a way that gives all cases an equal probability of being chosen Random sampling solves the problems of obtaining values not consistent with the true value of the population and also of not having values in a sample be normally distributed** Downloaded by Annie Marcovitz ([email protected]) lOMoARcPSD|50424300 Sampling Error Random sampling error is an error caused by a random process of selecting samples Random sampling error is caused by a random process of selected samples The solution to random sampling error is to sample more cases, as random errors get smaller as N approaches infinity Sampling bias is when the sampling frame or group from which you randomly sample does not equal the population Sampling bias happens when you sample from a group that is different from the group that you care about (not every member of the population will have an equal chance of being in the sample) Measurement Error vs. Sampling Error Measurement error is when you incorrectly describe the world because you incorrectly observed the cases you studied, while sampling error is when you incorrectly describe the world because the cases you study are different from the population you want to learn about Fundamental Problem of Causal Inference The fundamental problem of causal inference refers to how we can never observe a case under the counterfactual condition. Instead, we can only observe a case under one (the factual) condition This makes empirical tests of causality difficult because we don’t know what cases would have looked like if they had the other value of X** Potential outcomes refers to outcomes that cases would take under counterfactual conditions** If two cases are counterfactuals, it implies that the potential outcomes are from the same sample** Internal validity refers to when a study finds that the causal effect of X on Y is not biased (systematically incorrect) External validity is the degree to which the causal relationship we find in a study matches the causal relationship ad the context identified in a causal theory How are internal and external validity related?** Downloaded by Annie Marcovitz ([email protected]) lOMoARcPSD|50424300 i refers to the factual case Y refers to outcome when not exposed to cause i 0 Y refers to outcome when exposed to cause i 1 Y -Y refers to the individual causal effect i 1 i 0 X refers to the value of cause X for case i (1 for cause is present, 0 for cause is i absent) Value of dependent variable when independent variable is 1** (cause is present) Value of dependent variable when independent variable is 0** (cause is absent) The causal effect for each case?** Which of the values on this table are factual? Counterfactual** Comparative Method (Mill’s Method of Difference) Empirical prediction of a causal claim that this method tests: If we observe two cases to be the same in all relevant respects except for value of X, then we should observe that the two cases differ in the value of Y What has to be true for cases we compare in order to find evidence in support of “X causes Y”?** Correlation Empirical prediction of a causal claim that correlation tests: If X→ Y or X causes Y, then X and Y will be correlated Negative correlation is when the correlation is less than 0 and when values of X and Y move in opposite directions Positive correlation is when the correlation is more than 0 and values of X and Y move in the same direction Strong correlation is when values for X and Y cluster strongly along the line Weak correlation is when values for X and Y do not cluster as much along the line Correlation (significance) Downloaded by Annie Marcovitz ([email protected]) lOMoARcPSD|50424300 What is the problem of random association?** Statistical significance is an indication of how likely the correlation we observe could have happened purely by chance A p value is related to statistical significance because it is a numerical measure of statistical significance (lower p value indicates greater statistical significance while higher p value indicates lower statistical significance) Attributes of correlation that increase/decrease statistical significance: strength of correlation** Spurious Correlation Spurious correlation is when two variables are correlated, but the correlation is not the result of a causal relationship between these two variables Why is spurious correlation a kind of bias?** Spurious correlation happens because causes are often “clustered” together A confounding variable is a variable that an additional variable that results in X and Y being correlated What are the different forms spurious correlation can take?** If we know the direction of effects of a confounding variable (W) on X and Y, what is the direction of the bias/spurious relationship?** An intervening variable is a variable through which X causes Y (X→ W → Y) Intervening variables do not produce spurious correlation An antecedent variable is a variable that affects Y only through X (Z→ X→ Y) Antecedent variables can induce spurious correlation when they don’t just affect Y through X Solutions to Spurious Correlation Adjustment extrapolates comparative method to correlation Design-based are solutions that do not identify and measure all confounding variables, and it involves choosing a comparison that eliminates effects of many known and unknown confounding variables Difference between how adjustment and design-based solutions remove spurious correlation induced by confounding variables: design-based solutions do not identify and measure all confounding variables while adjustment-based solutions involve identifying all confounding variables Difference in how conditioning and randomization solve spurious correlation: conditioning holds other variables constant while looking at the relationship between X and Y while randomization** Types of Solutions to Spurious Correlation Uses conditioning or randomization to remove confounding variables/spurious correlation** o Adjustment Types of confounding variables eliminated Downloaded by Annie Marcovitz ([email protected]) lOMoARcPSD|50424300 o Adjustment: Only measured variables o Similar cases: All measured/unmeasured variables that are the same for compared cases o Same case over time: All measured/unmeasured variables that are unchanging for the case o Difference in difference: All measured/unmeasured variables that are unchanging for each case or changing over time and shared by all cases (shared trend) o Natural experiment: All confounding variables What types of confounding variables does it not eliminate? o Adjustment: unmeasured/mismeasured variables o Similar cases: unchanging variables that differ between cases and changing variables that differ between cases o Same case over time: confounding variables that change over time o Difference in difference: confounding variables that change over time but differ across cases o Natural experiment: N/A Assumptions made to conclude X causes Y after solution is applied o Adjustment: condition on all confounding variables and no measurement error on confounding variables o Similar cases: no constant/time-invariant difference between cases that affect X, Y and no changing/time-variant difference between cases that affect X, Y o Same case over time: no confounding variables that change over time for case and no confounding variables that change over time for cases o Difference in difference: no confounding variables that change over time differently across cases and trends are parallel across cases in absence of the cause o Natural experiment: cause is random Tradeoff between internal and external validity o Adjustment: high external validity because it can be done for all relevant cases, but at the cost of internal validity because it requires big assumptions o Similar cases: higher internal validity because it requires fewer assumptions about confounding variables, but at the cost of external validity because very similar cases with different X not possible for all relevant cases o Same case over time: high internal validity because it requires fewer assumptions about confounding variables but at the cost of external validity because result for individual case may not be relevant to all cases o Difference in difference: high internal validity because of even fewer assumptions about confounding variables but lower external validity because cases with similar trends but different “treatments” may be rare Downloaded by Annie Marcovitz ([email protected]) lOMoARcPSD|50424300 o Natural experiment: high internal validity because it requires minimal assumptions about confounding variables with low external validity because cases with randomized cause are unusual/rare Downloaded by Annie Marcovitz ([email protected])