Advanced Statistical Analysis: Event History Analysis 1
Document Details
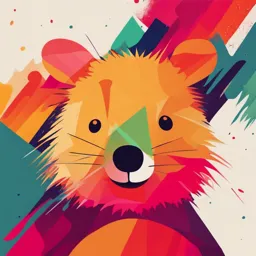
Uploaded by ClearerKoala
University of Groningen
Clara Mulder
Tags
Related
- ASA 6 Answers - Event History Analysis
- Advanced Statistical Analysis: Event History Analysis 1
- Advanced Statistical Analysis: Event History Analysis 2
- Advanced Statistical Analysis: Event History Analysis 3
- Analysis of Padre Faura Witnesses the Execution of Rizal PDF
- Palmer Raids Student Materials PDF
Summary
This document provides an introduction to event history analysis, focusing on definitions, terms, and some practical examples. The document details the theory and methodology behind event history analysis in a concise and accessible manner. This document appears to be a lecture slide presentation from a university course.
Full Transcript
Faculty of Spatial SciencesFaculty of Spatial SciencesFaculty of Spatial SciencesFaculty of Spatial SciencesAdvancedStatisticalAnalysis:EventHistoryAnalysis1ClaraMulder(thankstocolleagues) Facultyof Spatial SciencesFacultyof Spatial SciencesFacultyof Spatial SciencesFacultyof Spatial Sciences | 2Tod...
Faculty of Spatial SciencesFaculty of Spatial SciencesFaculty of Spatial SciencesFaculty of Spatial SciencesAdvancedStatisticalAnalysis:EventHistoryAnalysis1ClaraMulder(thankstocolleagues) Facultyof Spatial SciencesFacultyof Spatial SciencesFacultyof Spatial SciencesFacultyof Spatial Sciences | 2Today:›Eventhistoryanalysis1: •Introduction •Coxregression›Literature: •HandbookChapter9(SurvivalAnalysis) Facultyof Spatial SciencesFacultyof Spatial SciencesFacultyof Spatial SciencesFacultyof Spatial Sciences | 3Someterms›Survivalanalysis(general;alsousedspecificallyfor univariateanalysis!)›Eventhistoryanalysis›Analysisofdurationdata›Hazardanalysis/hazardregression›Intensityregression›Dutch:Gebeurtenissenanalyse›German:Ereignisanalyse Facultyof Spatial SciencesFacultyof Spatial SciencesFacultyof Spatial SciencesFacultyof Spatial Sciences | 4Howdoesthismethodlinktounivariate survivalanalysis/lifetable?›SurvivalanalysisusingKaplanMeier: descriptive methodtolookatthedistributionof timetoevent (for differentgroupsinasample) Facultyof Spatial SciencesFacultyof Spatial SciencesFacultyof Spatial SciencesFacultyof Spatial Sciences | 5Question:Howdoestheageofmarriagedifferbetweenlow,middleandhighly educatedwomeninIndia? SurvivalFunctionAGEMAR 5004003002001000 Cum Survival 1,21,0,8,6,4,20,0-,2 Highesteducational College/universitySecondaryPrimary SurvivalFunctionAGEMAR 5004003002001000 Cum Survival 1,21,0,8,6,4,20,0-,2 Highesteducational College/universitySecondaryPrimary AdvancedStatisticalAnalysisEHA:Thehazardrate&the(Cohort)LifeTableInalifetablethehazardrateisestimatedas(notationofthebook):hazardrate(note:youmayknowr(t)aslx ,n(t)asdx ,h(t)asmx ,withh(t)calculatedovermid-yearpopulation)tr(t)n(t)h(t)S(t)1650090.020.9817491200.040.9418471320.070.88…………………………323930.080.08333610.030.07Event: firstpartnershipSurvival: remainingsingleInterpretation: h(16)=0.02:2%becamepartnered betweenage16and17;h(32)=0.08:ofthosewhowere unparteneredattheir32nd birthday,8% enteredintheirfirstrelationshipbefore33rd birthday. S(32)=.08:8%arestillsingle,1-S(32)= 0.92or92%arepartneredbeforeage33 Facultyof Spatial SciencesFacultyof Spatial SciencesFacultyof Spatial SciencesFacultyof Spatial Sciences | 7Fromdescriptiontoexplanation:›Whatisthe effect ofeducationallevelonthehazard (~probability)ofmarryingatageT?(quantifyingthe difference)› thisisaquestiontobeansweredusingevent historyanalysis Facultyof Spatial SciencesFacultyof Spatial SciencesFacultyof Spatial SciencesFacultyof Spatial Sciences | 8Whenapplicable?›Assessingtheinfluenceofindependentvariables (covariates)on:(a)Durationfromtimezerountiloccurrenceofan event,or(b)Hazard(~probability/rate)ofoccurrenceofanevent atacertaintimepoint,giventhatithasnotoccurred before›Note:(a)and(b)arethesame,onlytheeffectswould beopposite.Effectsareexpressedaseffectson hazard. Facultyof Spatial SciencesFacultyof Spatial SciencesFacultyof Spatial SciencesFacultyof Spatial Sciences | 9Whatisanevent?›Achangeinstatus(e.g.fromalivetodead,renterto homeowner,unmarriedtomarried,marriedto divorced,employedtounemployedortheotherway around): subjectleaves studypopulation= populationatrisk (riskset) uponeventor›Somethingthathappensatacertaintimepoint withoutachangeinstatus(e.g.changeof residence):subject remainsinriskset,newepisode, durationclockbackto0 Facultyof Spatial SciencesFacultyof Spatial SciencesFacultyof Spatial SciencesFacultyof Spatial SciencesWhynot‘ordinary’linearregressionof durationuntilevent? | 10 Facultyof Spatial SciencesFacultyof Spatial SciencesFacultyof Spatial SciencesFacultyof Spatial Sciences | 11Whynot‘ordinary’linearregressionof durationuntilevent?›Cannothandle‘censoreddata’(whatwouldbethe valueofthedependentvariableiftheeventhasnot takenplace?)›Impossibletoincludetime-varyingcovariates(what isthe‘right’valueofanindependentvariableifthe valuechangesbetweentime0andtheevent?) Facultyof Spatial SciencesFacultyof Spatial SciencesFacultyof Spatial SciencesFacultyof Spatial Sciences | 12Eventhistoryanalysis›Thecrucialvariableisthe hazardh(t)›Wefirstintroducetheconcept durationT= timeuntilevent Facultyof Spatial SciencesFacultyof Spatial SciencesFacultyof Spatial SciencesFacultyof Spatial Sciences | 13Whatisahazard?›ahazardislikeaprobability,BUTdefinedoveratiny (infinitelysmall)timeintervalbetweentandt+Δt›remember:aprobabilityisalwaysdefinedwith referencetoacertaintimeperiod:•e.g.inthelifetable:whatistheprobabilityofdying betweenagesxandx+n? Orinagivenyear Facultyof Spatial SciencesFacultyof Spatial SciencesFacultyof Spatial SciencesFacultyof Spatial Sciences | 14Startingfromaprobability:› istheprobabilitythatanevent occursbetweentime t andtime t’ (where t < t’)›Wewanttoknowtheprobabilitythattheeventoccurs between t and t’,giventhatnoeventhasoccurredyet attime t: ›Thatistheconditionalprobability: )'Pr(tTt)(tT )|)'Pr(tTtTt )'Pr(tTt )(tT )|)'Pr(tTtTt Facultyof Spatial SciencesFacultyof Spatial SciencesFacultyof Spatial SciencesFacultyof Spatial Sciences | 15Hazardversus‘ordinary’probability›Probability,e.g.inthelifetable:theprobabilityofdyingbetweenagexandx+1 conditionalonhavingreachedagex›Theprobabilitydependsonthelengthoftime:thelargertheintervalt’-t(=Δt), thehighertheprobability›The hazard is not relatedtoaparticularintervaloftime:›=the propensity or intensity tohavetheeventattimet›Isconditional,or:definedinrelationtothepopulation atrisk, attimet,ofhaving theevent ' ' )|)'Pr( lim)('tt tt tTtTt thtt ' ' )|)'Pr( lim)( ' tt tt tTtTt th tt Facultyof Spatial SciencesFacultyof Spatial SciencesFacultyof Spatial SciencesFacultyof Spatial Sciences | 16Thehazardisthedependentvariableineventhistory analysis›‘Application’ofthehazardleadstoasurvivalfunction:the probabilitythattheeventhasnotoccurredattime t, or equivalentythattheepisode’sdurationisatleast t long (assumingitstartedat0):›Thisis:theprobabilityof(=proportioninthesample/ population) survivinguntiltime t )Pr()(tTtS)Pr()(tTtS Facultyof Spatial SciencesFacultyof Spatial SciencesFacultyof Spatial SciencesFacultyof Spatial Sciences | 17forexample:›thesurvivalfunctioninmigrationistheprobabilitythat nomovehasoccurredattimetsincelastmove,=the probabilitythatthepersonhaslivedinhercurrent placeatleastttimeunits(e.g.years)›theprobabilitythatachildlivesatleasttyears(event =death)›theprobabilitythatnofirstchildhasbeenborn (yet???)atleasttyearsafterpartnershipformation (event=firstchildbirth) Facultyof Spatial SciencesFacultyof Spatial SciencesFacultyof Spatial SciencesFacultyof Spatial Sciences | 18Censoring timea bLeftcensoringRightcensoringObservationwindowSurveyattritionab Facultyof Spatial SciencesFacultyof Spatial SciencesFacultyof Spatial SciencesFacultyof Spatial Sciences | 19Leftcensoring›Problematicinprinciple:durationunknown›Coxregression(thisweek):Noproperwayof handlingleftcensoring›Discrete-timelogit(nextweek):leftcensoringo.k.as longasthereisnoreasontosuspectduration dependenceofeffects(=effectschangethrough time) Facultyof Spatial SciencesFacultyof Spatial SciencesFacultyof Spatial SciencesFacultyof Spatial Sciences | 20Rightcensoring›Noproblemaslongascensoringcanbeassumedto berandom Facultyof Spatial SciencesFacultyof Spatial SciencesFacultyof Spatial SciencesFacultyof Spatial Sciences | 21Comparedescriptivemethods fordurationdata›KaplanMeier(univariatesurvivalanalysis)›Example:jobduration Facultyof Spatial SciencesFacultyof Spatial SciencesFacultyof Spatial SciencesFacultyof Spatial Sciences | 22KaplanMeier(estimatessurvivalfunction):Jobduration bysex Facultyof Spatial SciencesFacultyof Spatial SciencesFacultyof Spatial SciencesFacultyof Spatial Sciences | 23›Graph describes thedifferentsurvivalofmalesand femalesincurrentjob›Butstateddifferently:whatsizeisthe effect ofgender onjobduration–orasitisusuallyexpressed:onthe hazardofendingajob? Facultyof Spatial SciencesFacultyof Spatial SciencesFacultyof Spatial SciencesFacultyof Spatial Sciences | 24Eventhistorymodel›Wearegoingtomodelthehazardrate h(t) ofquitting ajob ›Therate h(t) stateshowlikelyitissomeonequitsthe currentjob(=event)attimet(infinitelysmalltime interval)›Whereasaprobability p(t,t+n) stateshowlikelyitis thatsomeonequitsthecurrentjobbetweentandt+n. Facultyof Spatial SciencesFacultyof Spatial SciencesFacultyof Spatial SciencesFacultyof Spatial Sciences | 25Generalformofevent historymodels:estimator!Meier-Kaplan theisthis modelregressionCox Inthe)()(:yvarsexplanatornohave weif)exp()()( 00ththXthth estimator!Meier -Kaplan theis this model regressionCox Inthe )()( :yvarsexplanator no have weif )exp()()( 00 thth Xthth Facultyof Spatial SciencesFacultyof Spatial SciencesFacultyof Spatial SciencesFacultyof Spatial Sciences | 26TheCoxmodel,introducedby D.R.Coxin1972: )exp()()(0XththBaseline hazard= unspecified, canhaveany formMultipliedby exponentiated valuesofthe explanatory vars)exp()()(0Xthth Facultyof Spatial SciencesFacultyof Spatial SciencesFacultyof Spatial SciencesFacultyof Spatial Sciences | 27Coxmodelisalsocalledthe proportionalhazards model:thehazardisassumed tobea time-constant ratio ofabaselinehazardand Exp(Xβ). Exp(β)isthe multiplicativeeffect ofindependent variable(s) X onthehazard(hazardratio), β = additiveeffect onthelogofthehazard. The plottedhazardsshouldlookliketheyhavethe sameratio throughtime,thelog-hazardsshould looklikethey areparallel whenplottedovertime. Facultyof Spatial SciencesFacultyof Spatial SciencesFacultyof Spatial SciencesFacultyof Spatial Sciences | 28IntroducethevariableX:gender›Males:X=1,Females:X=0›Malehazardfunction:›Femalehazardfunction:›Thehazardratesofmalesandfemalesare assumed tobe (and forcedbythemodel intobeing) proportional bya constantratioexp β exp)()(0ththmales)()(0ththfemalesexp)()(0ththmales )()(0ththfemales AdvancedStatisticalAnalysisTheCoxModel(or:CoxRegression)Thegeneralformofthemodelis: or isthehazardforindividual i attime t isavectorofcovariateswithcoefficients isthe baseline hazardi.e.thehazardwhen=0.Itcanhaveanyform!Note: anindividual’shazarddependsont through Interpretation:Covariates have a multiplicative effect on the hazard. i.e. for each unit increaseinxthehazardismultipliedbyexp(). if=0 if=1 istheratioofthehazardforx=1tox=0alsocalledrelativeriskor hazardratio AdvancedStatisticalAnalysisTheCoxModel(continued)Interpretation:, =0 noeffectofxonthehazardfound.Inourexample,no effectofsexonjobduration., >0 positiveeffectofxonthehazard.Highervaluesofx areassociatedwithshorterdurations , <0 negativeeffectofxonthehazard.Lowervaluesofx areassociatedwithlongerdurations Example:Allelseequal… Thehazardofquittingajobisexp(0.4)=1.49timeshigherforwomenthanfor men Theloghazardofquittingajobis0.4pointshigherforwomenthanformen Women’shazardofquittingajobis49%highercomparedtomen AdvancedStatisticalAnalysisTheCoxModel(continued)Inplotof log-hazard againsttimeweshouldsee parallel linesifproportional hazardassumptionismet(leftside).(whatabout hazard itself?). ProportionalNon-Proportional Facultyof Spatial SciencesFacultyof Spatial SciencesFacultyof Spatial SciencesFacultyof Spatial Sciences | 32Theproportionalityassumptionisrestrictive:›Inmanyapplicationsthehazardratesmaynotbeproportional•E.g.marriage:womenmarryfasterthanmenatyoungages, butmenhaveahighermarriagerateatolderages ›Thereforeyouhavetotestifthisassumptionisvalid›Forexample,useagraphicaltest:plotempiricalsurvivalor hazardfunctionsusingKaplanMeier.Therearealsoformal tests Facultyof Spatial SciencesFacultyof Spatial SciencesFacultyof Spatial SciencesFacultyof Spatial Sciences | 33Whatifthetestfails?›Youmaywanttospecifydifferentbaselinehazardsfor eachcategory: )exp()()(0Xthth SEX Separatebase linehazardrate foreachsexTheexplanatorypart withothervarsmay besimilarorsex-specific)exp()()(0Xthth SEX Facultyof Spatial SciencesFacultyof Spatial SciencesFacultyof Spatial SciencesFacultyof Spatial Sciences | 34Informationindata:›Durationuntileventorcensoring(numberoftime units)›Eventorcensoring?›Alternatively,fordiscrete-timemodels(nextweek!): Whethereventtakesplace(yesorno)intimeunit understudy›Valuesoftheindependentvariables AdvancedStatisticalAnalysisEventHistoryinStata:stsetcommandWe need to tell STATA we want to perform survival analysis. The command is stset.stset:TellsStatathestructureofyoursurvivaldata(soyoudonothavetorepeat);Checksthatthedatastructuremakessense;Allows us to describe complicated rules for when observations are included and excluded(advanced)Generalsyntax: stset durationvariable [if],failure(one_if_failure_var)optionsNote: stsetisusefulincontinuous-timemodels(Coxandparametric). AdvancedStatisticalAnalysisstsetcommand(continued)BeforeAfterDatainEventhistoryformat•Timevariable•Censoringvariable•CovariatesDatainEventhistoryformat•Timevariable•Censoringvariable•Covariatesstsetinformation:•_t0,_t,_d,_st•Structureinformation•DatacheckSTSET AdvancedStatisticalAnalysisstsetcommand(continued)stset createsnewvariables:_t0 and _t: they record the time span in analysis time units(t) for each record. _t0isthestartingand_tistheendingtime; _d:itisequalto1iftheeventoccursand0ifitdoesnot.Ithelpsusidentifying failuresandcensoring;_st: for each observation, the variable = 1 if Stata uses the observation and 0 otherwise.stset hasseveraloptionsdependingonwhichkindofdatawehave.Examples:a.Singleepisode(spell)datab.Multiple episode per person With time constant covariates (e.g. sex)c.Person-yeardatad.Time-varyingcovariates AdvancedStatisticalAnalysisEventhistoryanalysis:PreparationAfterStset:stsum tohaveanideaofyourdatadistributionstdes formoreadvanceddatastructure AdvancedStatisticalAnalysisEventhistoryanalysis:PreparationAfterStset:PlottingthesurvivalcurveusingKaplanMeierPlottingthe(smoothed)hazardstsgraph,cistsgraph,by(sex)stsgraph,hazardby(sex) sex .4121536 .0977979 4.21 0.000 .2204733 .6038338 _t Coef. Std.Err. z P>|z| [95%Conf.Interval] Loglikelihood = -2388.8621 Prob>chi2 = 0.0000 LRchi2(1) = 17.53 Timeatrisk = 38153 No.offailures= 430 No.ofsubjects= 563 Numberofobs = 563 Coxregression--Breslowmethodforties Iteration0: loglikelihood=-2388.8621 Refiningestimates: Iteration2: loglikelihood=-2388.8621 Iteration1: loglikelihood=-2388.8741 Iteration0: loglikelihood=-2397.6278 analysistime_t: jobdur failure_d: event1AdvancedStatisticalAnalysisTheCoxModelinStataExample: CoxregressionmodelofjobdurationFirststepisto stset thedata.stset jobdur,failure(event1)stcox i.sex, nohr=no hazard ratio. Stata shows coefficients, i.e. effectsonlogCategoricalvariable sex .4121536 .0977979 4.21 0.000 .2204733 .6038338 _t Coef. Std.Err. z P>|z| [95%Conf.Interval] Loglikelihood = -2388.8621 Prob>chi2 = 0.0000 LRchi2(1) = 17.53 Timeatrisk = 38153 No.offailures= 430 No.ofsubjects= 563 Numberofobs = 563 Coxregression--Breslowmethodforties Iteration0: loglikelihood=-2388.8621 Refiningestimates: Iteration2: loglikelihood=-2388.8621 Iteration1: loglikelihood=-2388.8741 Iteration0: loglikelihood=-2397.6278 analysistime_t: jobdur failure_d: event1AdvancedStatisticalAnalysisTheCoxModelinStata(continued)Example: CoxregressionmodelofjobdurationFirststepisto stset thedata.stset jobdur,failure(event1)stcox i.sex, nohrNote: If multiple records per individual, add the option vce(cluster id)here. Inthis way standard errors will be robust. sex .4121536 .0977979 4.21 0.000 .2204733 .6038338 _t Coef. Std.Err. z P>|z| [95%Conf.Interval] Loglikelihood = -2388.8621 Prob>chi2 = 0.0000 LRchi2(1) = 17.53 Timeatrisk = 38153 No.offailures= 430 No.ofsubjects= 563 Numberofobs = 563 Coxregression--Breslowmethodforties Iteration0: loglikelihood=-2388.8621 Refiningestimates: Iteration2: loglikelihood=-2388.8621 Iteration1: loglikelihood=-2388.8741 Iteration0: loglikelihood=-2397.6278 analysistime_t: jobdur failure_d: event1AdvancedStatisticalAnalysisTheCoxModelinStata(continued)Example: Coxregressionmodelofjobdurationstset jobdur,failure(event1)stcox i.sex, nohrtest for null hypothesis (H0): If we remove the option nohr,thetestwillbefor AdvancedStatisticalAnalysisTheCoxModelinSTATA:TipIfmorethanoneepisodeperperson(personnumber=id):Useoptionvceorrobustcluster(id)toobtainrobuststandarderrorsstcox i.sex,vce(cluster id)[nohr]orstcox i.sex,robustcluster(id)[nohr] Faculty of Spatial SciencesFaculty of Spatial SciencesFaculty of Spatial SciencesFaculty of Spatial SciencesAdvancedStatisticalAnalysis:EventHistoryAnalysis2ClaraMulder(thankstocolleagues) Facultyof Spatial SciencesFacultyof Spatial SciencesFacultyof Spatial SciencesFacultyof Spatial Sciences | 2Today:›Eventhistoryanalysis2: •Afewwordsonparametricmodels•Thecumulativehazard•Coxregression(continued)›Literature: •HandbookChapter9(SurvivalAnalysis) Facultyof Spatial SciencesFacultyof Spatial SciencesFacultyof Spatial SciencesFacultyof Spatial Sciences | 3Parametriceventhistorymodels(notpracticed):›Specifyafunctionalformthehazardrate h(t)asit evolves/isdistributedthroughtime: mathematical function•e.g.polynomialrates(quadraticfunction)•e.g.Gompertz:hazarddecreasesmonotonically•e.g.Gamma(usedforfertility)•e.g.Weibull(flexible3-parameterfunction)›Estimateparameters ofthisfunction ANDtheeffects oftheindependentvariables Facultyof Spatial SciencesFacultyof Spatial SciencesFacultyof Spatial SciencesFacultyof Spatial Sciences | 4TheCoxmodelissemiparametricbecause:›Thereisnoassumptionabouttheformof h0(t) over time. Itcantakeanyform,thereis no mathematical functiondescribingit›Thisisunlikefullyparametricmodels›There are parametersforthe effects,though(justas infullyparametricmodels) Facultyof Spatial SciencesFacultyof Spatial SciencesFacultyof Spatial SciencesFacultyof Spatial Sciences | 5Relationbetweensurvivalandhazard›Survivalcanonlygodown,hazardmayfluctuate›Thesteeperthesurvivalcurvegoesdown,the [higher?lower?]thehazard›Survivalattime t determinedbythe‘course’ofthe hazard uptotimet,therefore:›No direct relationship…butvia cumulativehazard AdvancedStatisticalAnalysisTheCumulativeHazardfunctionMeasures the total amount of risk (hazard) that has been accumulated uptoacertaintimet.Itisthenumberoftimesthesubject isexpectedtoexperiencetheevent until timeT(e.g. if we are able to resurrect how many time will we die in 10years?)Not a straightforward interpretation. It can be used to compute the survivalfunctionandfortestingmodelperformance.Itis not aprobability asitcanbelargerthanoneH(t)= Facultyof Spatial SciencesFacultyof Spatial SciencesFacultyof Spatial SciencesFacultyof Spatial Sciences | 7Fromcumulativehazardtosurvival:›S(t)=exp(-H(t))SurvivalandCumulativeDistributionFunction:›S(t)=1– F(t), F(t)=1– S(t)›F(t)istheproportionthathasexperiencedtheevent; oftenmoreintuitivethan S(t) Facultyof Spatial SciencesFacultyof Spatial SciencesFacultyof Spatial SciencesFacultyof Spatial Sciences | 8Cumulativedistributionvscumulativehazard›F(t)=1– S(t)canonlygoup,sameforcumulative hazard›Slopeof F(t)dependsonsizeofpopulationatrisk, slopeofcumulativehazarddoesn’t›F(t)canonlybetween0and1,cumulativehazardcan behigherthan1 AdvancedStatisticalAnalysisTheCoxModelinStata(continued)Example: Coxregressionmodelofjobdurationor Plottingthe model=predicted (smoothed)hazardandcumulativehazardstcox i.sexstcurve,hazardat(sex ==0)at(sex ==1) /*proportionalityforced*/stcurve,cumhaz /*thisistheKaplanMeierestimate*/ AdvancedStatisticalAnalysisTheCoxModelinStata(continued)Example: Coxregressionmodelofjobdurationor Plottingthe predicted survivalfunction(KaplanMeier,proportionalityforced)stcox i.sexstcurve,survivalStcurve,survivalat(sex ==0)at(sex ==1) Facultyof Spatial SciencesFacultyof Spatial SciencesFacultyof Spatial SciencesFacultyof Spatial Sciences | 11Questions:›Ifthehazardis constant throughtime:›WhatwilltheCumulativeHazardlooklike?›WhatwilltheSurvivalfunction S(t)looklike?›WhatwilltheCumulativeDistributionfunction F(t) looklike? AdvancedStatisticalAnalysisTheCoxModelinStata(continued)Example: CoxregressionmodelofjobdurationTestingtheproportionalityassumptionLog-logplot:linesshouldnotcrossstcox i.sex stphplot,by(sex) TheCoxModelinStata(continued)Example: CoxregressionmodelofjobdurationTestingtheproportionalityassumptionPlotpredictedagainstobservedsurvival:shouldlookaboutthesame stcox i.sexstcoxkm,by(sex) AdvancedStatisticalAnalysisTheCoxModelinSTATA:TipIfmorethanoneepisodeperperson(personnumber=id):Useoptionvceorrobustcluster(idvar)toobtainrobuststandarderrorsstcox i.sex,vce(cluster id)orstcox i.sex,robustcluster(id)Or,alreadyat stset,usetheoption: id(id) (in the example data for quitting a job this does not work, apparently because some respondentshadtwojobsatthesametime)Minddifferencebetweenrobustandrobustcluster! Facultyof Spatial SciencesFacultyof Spatial SciencesFacultyof Spatial SciencesFacultyof Spatial Sciences | 15 ------------------------------------------------------------------------------ | Robust _t|Coefficient std.err. z P>|z| [95%conf.interval] -------------+---------------------------------------------------------------- sex| women | .4234031 .0990821 4.27 0.000 .2292059 .6176004 ------------------------------------------------------------------------------ | Robust _t|Haz.ratio std.err. z P>|z| [95%conf.interval] -------------+---------------------------------------------------------------- sex| women | 1.52715 .1513132 4.27 0.000 1.257601 1.854473 ------------------------------------------------------------------------------ Whatwouldbecoeff/hazardratioformenifwomenwouldbe reference?Howdoyougetfromcoeffandstderrortoconfidenceinterval?Howdoyougetfromcoefftohazardratio?Andviceversa?Plus:aroughapproximationtogetfrom…andvv Facultyof Spatial SciencesFacultyof Spatial SciencesFacultyof Spatial SciencesFacultyof Spatial Sciences | 16Questions? Faculty of Spatial SciencesFaculty of Spatial SciencesFaculty of Spatial SciencesFaculty of Spatial SciencesAdvancedStatisticalAnalysis:Eventhistoryanalysis3ClaraMulder Faculty of Spatial SciencesFaculty of Spatial SciencesFaculty of Spatial SciencesFaculty of Spatial SciencesThislecture›GeneralfeedbackassignmentCoxRegression›Reminderassignments›Moreabout preparationofanalysis›Analternativemethod:discrete-timeeventhistory analysisusinglogisticregressionofperson-perioddata›Extensions:time-varyingcovariates,non-proportional hazards,multiplerisks›Complications:leftcensoring,non-randomright censoring,periodsofmissinginformation Faculty of Spatial SciencesFaculty of Spatial SciencesFaculty of Spatial SciencesFaculty of Spatial SciencesAssignmentCoxRegression Faculty of Spatial SciencesFaculty of Spatial SciencesFaculty of Spatial SciencesFaculty of Spatial SciencesAssignmentCoxRegression›Alotofeventsbeforeage30(highesthazard)›Hazardscrossatage30•So….Proportional?›Oddfindingsaboveage40•makeslittlesense;bettertotruncate(at40?30?)•Askforconfidenceintervals: stsgraph,hazardby(sex)ci›Respondentsclusteredinhouseholds •So…robustcluster(nohhold) Faculty of Spatial SciencesFaculty of Spatial SciencesFaculty of Spatial SciencesFaculty of Spatial SciencesAssignmentCoxRegression Faculty of Spatial SciencesFaculty of Spatial SciencesFaculty of Spatial SciencesFaculty of Spatial SciencesAssignmentCoxRegression› Predictedcohort survivalusing stcurve: forcedinto proportionality Faculty of Spatial SciencesFaculty of Spatial SciencesFaculty of Spatial SciencesFaculty of Spatial SciencesAssignmentCoxRegression›Interpretationfindingforlevelofeducation •‘Educationstrivedfor’ Faculty of Spatial SciencesFaculty of Spatial SciencesFaculty of Spatial SciencesFaculty of Spatial SciencesReminderassignments›Minimum:4outof5from1st 5weeks,1outof2from last2weeks›Doublecheckwhetheryouattendedthesufficient numberofpracticalsandpassedthesufficient numberofassignments(listwillbeonBrightspacein thefolderAssignment6). ›Ifyouneedtotakearesitassignment:uploaditinthe resitassignmentfolderby29March17:00h.Wewill not graderesitassignmentsuploadedinthewrong folder Faculty of Spatial SciencesFaculty of Spatial SciencesFaculty of Spatial SciencesFaculty of Spatial SciencesPreparation:Defineevent›Sometimesobvious,sometimesnot. E.g.Legaldivorceorseparation?Birthorconception?Allmovesorjustmigration(longdistance)?Moveintohomeownershiporalsobecoming homeownerbytransferofownershipofcurrent home?›Howtochoose? Faculty of Spatial SciencesFaculty of Spatial SciencesFaculty of Spatial SciencesFaculty of Spatial SciencesPreparation:Determine populationatrisk›Thosewhocanexperiencetheevent.Excludethose whocannot›Forchangeinstatus:‘whocannot’includesthose whohavealreadyexperiencedtheevent(example: homeowners,divorced)›Foreventwithoutchangeinstatus:everyone,or somelogicalselection.Do1st,2nd …havemeaning? Faculty of Spatial SciencesFaculty of Spatial SciencesFaculty of Spatial SciencesFaculty of Spatial SciencesPreparation:Determiningtime0(startofclock; riskperiod) ›Fordeath:Birth›Formarriage:Agefromwhichmarriageislegal›Fordivorce:Dateofmarriage(forsomecountries: afterwaitingtime)›Forfertility:Age15?Marriage?Startofpartnership?›Forunemployment:Startofworkhistory?Startof job?›…etcetera Faculty of Spatial SciencesFaculty of Spatial SciencesFaculty of Spatial SciencesFaculty of Spatial SciencesPreparation:Determiningtimeunit ›Years?Months?Days?Hours?Minutes?›Dependsonprocessunderstudy:compareMortalityInfantmortality(in1st year)Neonatalmortality(in1st 28days)DivorceFindingajobfromunemploymentArrivalofnextbus›Dependson… Faculty of Spatial SciencesFaculty of Spatial SciencesFaculty of Spatial SciencesFaculty of Spatial SciencesPreparation:Determiningendofobservation Truncateobservationwhen:›Occurrenceofeventislogicallynolongerpossible›Populationatriskgetstoosmalltogetreliableestimatesofthe hazard›NeventsgetstoosmalltogetreliableestimatesHow?›Coxregression: replace eventindicator =0 if T>Tend (manualcensoring)›recode T (Tend / [highest] =Tend)›Discrete-timelogit: keepif durationvar =<Tend Faculty of Spatial SciencesFaculty of Spatial SciencesFaculty of Spatial SciencesFaculty of Spatial SciencesPreparation:Describinghazard/survival›SurvivalanalysisusingKaplanMeier•Depictingthesurvivalfunction•Depictingthehazardfunction•Calculatingsurvivalstatistics(e.g.mediansurvival time) Faculty of Spatial SciencesFaculty of Spatial SciencesFaculty of Spatial SciencesFaculty of Spatial SciencesFirst-timehome-ownershipbyage:survival functions,Netherlands(Mulder&Wagner1998in UrbanStudies)From:Mulder&Wagner,UrbanStudies1998 Faculty of Spatial SciencesFaculty of Spatial SciencesFaculty of Spatial SciencesFaculty of Spatial SciencesPreparation:Choosingamethod›Possiblyaparametricmethod,ifthehazardhasa clearfunctionalformandotherassumptionsare justified ›Coxregression,ifthehazardsare(mostly) proportional›Discrete-timemodel:e.g.logisticregressionof person-years(orperson-monthsor…) Faculty of Spatial SciencesFaculty of Spatial SciencesFaculty of Spatial SciencesFaculty of Spatial SciencesChoicesinMulder&Wagner:›Event:movingintofirstowner-occupiedhome (includedbuying,inheriting,buyingwithhelp;didnot includebecomingownerwithoutmoving)›Populationatrisk:rentersandthoselivingin parentalhome–neverownedbefore›Time0:age15(couldhavebeen18buttherewere afewaged15-17)›Timeunits:years(couldhavebeenmonthsbut…)›Discrete-timelogisticregression Faculty of Spatial SciencesFaculty of Spatial SciencesFaculty of Spatial SciencesFaculty of Spatial SciencesThediscrete-timelogisticregression(=logit) model›Unitsofanalysis:timeunits(e.g.person-years)›Dependentvariable:Event(1)ornoevent(0)›Timeunitsaftereventoccurrence:notinanalysis(drop ormakesuretouse…if)›Covariates:time-constantortime-varying›Duration:expressedinnumberoftimeunitswithout event›Hazard:assumed(piecewise)constant;exploringform possiblethroughusingdurationvariableandpersonfile! Faculty of Spatial SciencesFaculty of Spatial SciencesFaculty of Spatial SciencesFaculty of Spatial SciencesSatisfactoryapproximationofcontinuoustime?›O.k.if%oftimeunitswithevent(=withvalue1in dependent)<10›Sochooseyourtimeunitsmallenough›Thentheansweris:yes,goahead! Faculty of Spatial SciencesFaculty of Spatial SciencesFaculty of Spatial SciencesFaculty of Spatial SciencesViolationofassumptionofindependenceof observations(isthisamultilevelproblem)?›Standarderrorsdeterminedbycategorywithfewest observationsindependentvariable›Nomatterhowmany0inthedependentvariablewe have,thereisalwaysjustone1(maximum).Andyou havemadesurecategorywithvalue1ismuch smallerthancategorywithvalue0›Sotheansweris:no,goahead(unlessmultiple episodesperperson;thenrobuststandarderrors)! Faculty of Spatial SciencesFaculty of Spatial SciencesFaculty of Spatial SciencesFaculty of Spatial SciencesDatapreparation›Constructionofperson-periodfile,usuallyfrom personfile •Stata: reshapelong Faculty of Spatial SciencesFaculty of Spatial SciencesFaculty of Spatial SciencesFaculty of Spatial SciencesAnalysis›Stata: logit Faculty of Spatial SciencesFaculty of Spatial SciencesFaculty of Spatial SciencesFaculty of Spatial SciencesEventhistoryanalysis:fourextensions›Throughtime, values ofcovariatesmaychangeinthe courseoftheduration(‘Time-varyingcovariates’)›Throughtime, effects ofcovariatesmaychange(non-proportionalhazards)›Multiplespells(>1eventperperson)›Multiplerisks(e.g.unmarriedcohabitationand marriage,ratherthanjustmarriage) Faculty of Spatial SciencesFaculty of Spatial SciencesFaculty of Spatial SciencesFaculty of Spatial SciencesExtension1:Time-varyingcovariates›Solution:Choosemethodthatallowsforthese•Coxmodel:episodesplitting. •Discrete-timelogisticregressionmodel:update valueofcovariateeachtimeunit Faculty of Spatial SciencesFaculty of Spatial SciencesFaculty of Spatial SciencesFaculty of Spatial SciencesExtension2:Non-proportionalhazards (effectschangethroughtime)›Anotherexamplefrom Mulder&Wagner,Urban Studies1998 Faculty of Spatial SciencesFaculty of Spatial SciencesFaculty of Spatial SciencesFaculty of Spatial SciencesHazardoftransitiontohome-ownershipbyage,WestGermany andtheNetherlands(selectedcohortsborn1900-1960)From:Mulder&Wagner,UrbanStudies1998 Faculty of Spatial SciencesFaculty of Spatial SciencesFaculty of Spatial SciencesFaculty of Spatial SciencesWhatistheeffectofdummyvariable‘country’?›Whenassumingproportionality:probablyaroundzero orpositiveiftheNetherlands=1›Clearlywrong Faculty of Spatial SciencesFaculty of Spatial SciencesFaculty of Spatial SciencesFaculty of Spatial SciencesHandlingnon-proportionalhazards›Ifproportionalityassumptionisviolated: ›CoxRegression:Splitepisodes;then: •runmodelseparatelyforyoungerandolderages•ORincludeadummyyoung/oldandaninteractionwith thecovariate›Discrete-timelogisticregression:Includeinteractionof covariatewithdurationvariable:country*ageorcountry* agegroup Faculty of Spatial SciencesFaculty of Spatial SciencesFaculty of Spatial SciencesFaculty of Spatial SciencesExtension3:multiplespells›Re-appearanceinpopulationatrisk›Morethanonespell/eventperperson›Ifthisisnotrare:correctstandarderrorsforclustering ofspellsinpersons(multi-levelforbeginners…)•Statasubcommand: robustcluster (id-var)or vce (cluster id-var) Faculty of Spatial SciencesFaculty of Spatial SciencesFaculty of Spatial SciencesFaculty of Spatial SciencesExtension4:multiplerisks›e.g.fromrenttootherrentortoown›Coxmodel:notpossibleasfarasIknow›Discrete-timemultinomiallogisticregression: dependent0(noevent),1,2,…•Stata: mlogit Faculty of Spatial SciencesFaculty of Spatial SciencesFaculty of Spatial SciencesFaculty of Spatial SciencesComplication1:leftcensoring›Continuous-timemethodsincludingCoxmodel: •Left-censoredcasesuseless Faculty of Spatial SciencesFaculty of Spatial SciencesFaculty of Spatial SciencesFaculty of Spatial SciencesComplication1:leftcensoring›Discrete-timelogisticregression:•Ifnodurationdependence(constanthazard):no problem•Trytofindsubstitutefordurationvariable•Includeadurationvariable,withacategory ‘unknown’?Impute? Faculty of Spatial SciencesFaculty of Spatial SciencesFaculty of Spatial SciencesFaculty of Spatial SciencesComplication2:non-randomrightcensoring Faculty of Spatial SciencesFaculty of Spatial SciencesFaculty of Spatial SciencesFaculty of Spatial SciencesReminder:rightcensoring›Truncationofobservationbecauseof:•Panelloss(paneldata)•Endofobservation(paneldata)•Theinterview(retrospectivedata)•Occurrenceof competing event(e.g.transition rent-own:rentermovesbacktoparents): competingrisks Faculty of Spatial SciencesFaculty of Spatial SciencesFaculty of Spatial SciencesFaculty of Spatial SciencesComplication2:non-randomrightcensoring›e.g.panelattritionbecauseofamovewhilemovingis thedependentvariable›Oftenignoredorjustmentionedasaproblem›Modelpanelattritionasextrarisk? Faculty of Spatial SciencesFaculty of Spatial SciencesFaculty of Spatial SciencesFaculty of Spatial SciencesComplication3:periodsofmissinginformation ›Commonprobleminpaneldata›Similartomissingvaluesincross-sections…but morecomplicated›Nostandardsolution›Copyvaluesfromprevioustimeunit?Impute? Faculty of Spatial SciencesFaculty of Spatial SciencesFaculty of Spatial SciencesFaculty of Spatial SciencesAssignment›Samedataasassignmentlastweek(leavinghome),but transformedintoperson-yearfileandwithextra variables›Reproducelastweek’sresultsusinglogisticregression ofperson-years›[Extra:includetime-varyingcovariatesforwhether respondenthasfinishededucationandhasenteredthe labormarket(=hashadafirstjob)]›Extra:includeinteractionbetweenageandsextoallow fornon-proportionalhazards Faculty of Spatial SciencesFaculty of Spatial SciencesFaculty of Spatial SciencesFaculty of Spatial SciencesAssignment›Note:datainthisformatNOTsuitableforpreparatory survivalanalysis;useperson/episodedata›Part2:Trytoexplainthetransitiontofirst-timehome-ownershipusinginformationavailableinthedata›UseMulder&Wagner1998inUrbanStudiesas inspirationifyouwantto Assignment6EventhistoryanalysisThe aim of this exercise is to find out how to perform a Cox regression model with timeconstantexplanatoryvariableswiththeuseofstatisticalsoftwarepackageSTATA.Createa*.dofilewithSTATAsyntaxtoworktimeefficiently. STATAoutputtablesandfiguresshouldbeneatlypresentedinyourWorddocument.IfyouexperienceanydifficultieswiththeassignmentorStata:1)First,Googleit(oruseStatahelp);2)Then,discussitwithyourfellowstudents;3)Last,askore-mailthe computerlabsupervisors(preferablyduringthecomputerlabsessions).Forallexercises:●Worktogetherinpairsoftwoandindicatebothstudents’namesontheworksheet. ●AlwayspasteyoursyntaxandtheappropriateoutputfromSTATAinyouranswerfile tosupportyouranswers. ●Wheneveranewcommandisused,explainthestructureandcontentofthecode.At alltimes,makesureyourworkistransparentandtraceable!Remembertosaveyourwork.●Upload the answers of your assignment to Brightspace when you are finished. The deadlineisonMonday09:00a.m.Studentnames:StudentIDs: Goodluckwithassignment6!1 ExerciseAUsetheStatasytemfile eventhisfileCox.dta.ThisfilecontainsinformationaboutrespondentsfromtworetrospectivesurveysheldintheNetherlands in the early 1990s: the SSCW-survey (also called Telepanel survey) and theNetherlandsFamilySurvey1993.Inthesesurveysextensiveinformationwasgatheredaboutthe respondents’ life histories. For this assignment we use information about the timing ofleavingtheparentalhomeandasmallnumberofbackgroundvariables.Of course, you are expected to include tables and plots in your report to support yourarguments.Lotsofsuccessandfundoingtheassignment!1.Makeanewvariableindicatingtheageofleavinghomeorcensoring,using informationabouttheyearofbirthandtheyearofleavinghome.Namethis variable“ageleft”.Declarethedatasettobesurvivaldatausingthestset command: thetime-variableis“ageleft” andthefailurevariableis“left”. ExplorefailuredistributionanduseKaplanMeieroftheageofleavinghometo answerthefollowingquestions.Usethecommand“stdescribe”forsummary statistics,andstsgraphforplotsofthesurvivalandsmoothedhazard:a.Whotendstoleavehomeearlier/faster:womenormen?b.Whatisthemedianageofleavinghomeforwomenandmen?c.Aroundwhichageisthehazardrateofleavinghomehighest,forwomenand men?genageleft=yearleft-birthyrlabelvariableageleft"Agewhenleavingparentalhome"stsetageleft,failure(left)sortsexbysex:stdescribe2 failures 1462 .9426177 0 1 1time at risk 35227 22.71244 16 21 73time on gap if gap 0 subjects with gap 0 (final) exit time 22.71244 16 21 73(first) entry time 0 0 0 0no. of records 1551 1 1 1 1no. of subjects 1551 Category total mean min median max per subject analysis time _t: ageleft failure _d: left-> sex = Female failures 1470 .9018405 0 1 1time at risk 40048 24.56933 16 23 67time on gap if gap 0 subjects with gap 0 (final) exit time 24.56933 16 23 67(first) entry time 0 0 0 0no. of records 1630 1 1 1 1no. of subjects 1630 Category total mean min median max per subject analysis time _t: ageleft failure _d: left-> sex = Male stsgraph,by(sex) 3 stsgraph,hazardby(sex) a)Womentendtoleavehomeearlier/faster.4 b)The median age of leaving home is 21 years for women and 23 years for men (seetabledescribingthesurvivaltimedatasetandKaplanMeierestimate).c)Thehazardrateofleavinghomeishighestintheearly/mid-20s(seehazardfunction).2.How about the proportionality assumption: is it justified, or is it violated, andhow? Theproportionalityassumptiondoesnotseemjustified.Beforeage32/33,womenaremorelikelytoleavehome.Afterwards,menaremorelikelytoleave.Aboveage50,hazardsgoup.Thisdoesnotmakemuchsense;itisbettertotruncatetheanalysismuchearlier.3.RunaCoxregressionofleavinghomewithsexastheonlyindependentvariable.What is the estimated difference in the hazard function between women andmen?Considerwhetheritisnecessarytoaccountforclustering.stcoxi.sex,vce(clusternohhold) Female 1.481069 .0487977 11.92 0.000 1.38845 1.579867 sex _t Haz. Ratio Std. Err. z P>|z| [95% Conf. Interval] Robust (Std. Err. adjusted for 1,944 clusters in nohhold)Log pseudolikelihood = -21114.561 Prob > chi2 = 0.0000 Wald chi2(1) = 142.11Time at risk = 75275No. of failures = 2,932No. of subjects = 3,181 Number of obs = 3,181Cox regression -- Breslow method for tiesIteration 0: log pseudolikelihood = -21114.561Refining estimates:Iteration 2: log pseudolikelihood = -21114.561Iteration 1: log pseudolikelihood = -21114.598Iteration 0: log pseudolikelihood = -21169.894 analysis time _t: ageleft failure _d: leftKeeping all the other covariates constant, the hazard of leaving home for women is 48%higherthanformen.Or,inotherwords,thehazardofwomenleavingtheparentalhomeisestimatedtobe1.48timesashighasthehazardofmen.Theestimateisinfluencedmostbythesexdifferenceatyoungerages. 4.Toanalysehowleavingtheparentalhomehaschangedbetweenbirthcohorts:a.Run another Cox regression, but now include birth cohort as an additionalindependentvariablebesidessex.Explainhowleavingtheparentalhomehaschangedbetweenbirthcohorts.b.Plot the predicted cohort survival curves for females using the stcurvecommand. Compare them to the empirical survival curves using sts graph,andinterpretthedifference. 5 stcoxi.sexi.cohno,vce(clusternohhold) nohrbasesurv(surv0) 1965-74 .7517968 .1121559 6.70 0.000 .5319752 .9716183 1955-64 .8262782 .0989664 8.35 0.000 .6323075 1.020249 1945-54 .6847063 .1015396 6.74 0.000 .4856924 .8837202 1935-44 .3625264 .1015541 3.57 0.000 .1634841 .5615688 1925-34 .0913412 .0996504 0.92 0.359 -.1039699 .2866523 cohno Female .422586 .0344428 12.27 0.000 .3550794 .4900926 sex _t Coef. Std. Err. z P>|z| [95% Conf. Interval] Robust (Std. Err. adjusted for 1,944 clusters in nohhold)Log pseudolikelihood = -21006.156 Prob > chi2 = 0.0000 Wald chi2(6) = 338.26Time at risk = 75275No. of failures = 2,932No. of subjects = 3,181 Number of obs = 3,181Cox regression -- Breslow method for tiesIteration 0: log pseudolikelihood = -21006.156Refining estimates:Iteration 3: log pseudolikelihood = -21006.156Iteration 2: log pseudolikelihood = -21006.157Iteration 1: log pseudolikelihood = -21007.298Iteration 0: log pseudolikelihood = -21169.894 analysis time _t: ageleft failure _d: leftThe risk of leaving parental home is greater for the younger birth cohorts. For example,compared to the oldest birth cohort 1900-24 (ref), the birth cohorts 1955-64 and 1965-74significantly increase the chance of leaving house by 0.826 points and 0.752 pointsrespectively. Nowweplotpredictedsurvivalinfemalesofdifferentcohorts.stcurve,survivalat1(cohno=1sex=1)at2(cohno=2sex=1)at3(cohno=3sex=1) at4(cohno=4sex=1)at5(cohno=5sex=1)at6(cohno=6sex=1)Note:stcurveplotsthefunctionatthemeanofunspecifiedcovariates.SoifourCoxmodelhadincludedmorevariablesthanjustcohnoandsex,stcurvewouldhaveplottedthesurvivalfunctionatthemeanvaluesoftheseothervariables.Thisistheadvantageofusingstcurveovermanualcalculationofthesurvivalorhazardfunctions,whenyouhavemorecovariatesthanyouplotseparatelinesfor.6 0.2.4.6.81 Survival203040506070analysistimecohno=1,sex=1cohno=2,sex=1cohno=3,sex=1cohno=4,sex=1cohno=5,sex=1cohno=6,sex=1Coxproportionalhazardsregressionstsgraphifsex==1,by(cohnoThe difference between the two graphs is that the predicted survival is forced intoproportionality.Thisisnotthecasefortheempiricalsurvival.7 5.Thedatasetalsocontainsinformationaboutfinallyachievedlevelofeducation:atime-constantvariablemeasuredatthemomentofinterview.Nowtakealookat the variables ‘year finished education’ and ‘year of interview’. Why is it,strictly speaking, not appropriate to include final level of education in the Coxregression?Education is time-varying and the educational level at the time of leaving home might belowerthantheeducationallevelatthetimeofinterview.Itcanbeassumedthatthelevelofeducation at the time of potentially leaving the parental home is crucial in the decisionwhetherleavingornot.Theageatwhichchildrenleavetheparentalhomeisusuallywheneducationisfinished,butthis is often not the case for university educated persons. Some may leave the parental inorder to enrol in education somewhere else, others stay at home during the(professional/university) education period and only leave home when taking up a job orwhenstartingtolivewithapartner.Alookatthevariable‘yearfinishededucation’showsthat5%oftherespondentswerestillineducationatthetimeoftheinterview.Usingtheinformationon‘finallevelofeducation’mayviolatetheassumptionofcausalityintheanalysis.Thisproblemissometimesalsoreferredtoas“anticipatoryanalysis”.Blossfeld and Rohwer (2002) have devoted chapter 1.2 on “Event History Analysis andCausal Modeling”, where the use time-dependent and time-independent variables ismentioned.6.Include the variable anyway. What do you find? Variable edlong has valuesoutsidetherangeof1-4.Dropthesecases.Howwouldyouinterpretthefindings,givenyouranswertoQuestion5?dropifedlong<=0|edlong>4 stcoxi.sexi.cohnoi.edlong,vce(clusternohhold)Theeffect ofthis variable has to beinterpreted withcare. A possibleinterpretationis thatpeoplewithahigherfinallevelofeducationtendtohaveagreaterriskofleavingparentalhome,perhapsbecausetheywanttoachievethatfinallevel.Itishowevernotpossibletosaythat higher education is the cause of the higher risk of leaving home. Having only time-constant information about the educational level, it is not known which event precedes theother.Inotherwords,wedonotknowwhetherhigheducationprecedesleavingparentalorwhetherleavingparentalhomeprecedeshighereducation.8 higher secu..) .2802169 .0636552 4.40 0.000 .155455 .4049788upper sec/l..) .0649361 .0592547 1.10 0.273 -.0512009 .1810731lower secun..) -.0861302 .0532381 -1.62 0.106 -.1904749 .0182145 edlong 1965-74 .7218987 .1176751 6.13 0.000 .4912598 .9525376 1955-64 .7947564 .1043453 7.62 0.000 .5902435 .9992694 1945-54 .6666931 .1062423 6.28 0.000 .4584621 .8749242 1935-44 .3620078 .1061291 3.41 0.001 .1539985 .5700171 1925-34 .094824 .104068 0.91 0.362 -.1091456 .2987935 cohno Female .4326109 .0351521 12.31 0.000 .3637141 .5015077 sex _t Coef. Std. Err. z P>|z| [95% Conf. Interval] Robust (Std. Err. adjusted for 1,925 clusters in nohhold)Log pseudolikelihood = -20675.408 Prob > chi2 = 0.0000 Wald chi2(9) = 365.83Time at risk = 74101No. of failures = 2,894No. of subjects = 3,139 Number of obs = 3,139Cox regression -- Breslow method for tiesIteration 0: log pseudolikelihood = -20675.408Refining estimates:Iteration 3: log pseudolikelihood = -20675.408Iteration 2: log pseudolikelihood = -20675.408Iteration 1: log pseudolikelihood = -20676.865Iteration 0: log pseudolikelihood = -20857.716 analysis time _t: ageleft failure _d: left9 OPTIONALExerciseB(buthighlyrecommendedtotry!)IntroductionTopic:Jobmobility Research question: How is the likelihood of quitting jobs related to individual and jobcharacteristics?Dataset:tda.dtaThevariablesinthisdatasetare:ididofindividualnojserialnumberofjobtsartstartingtimeofjobtfinendingtimeofjobsex1=men,2=womentidateofinterviewtbdateofbirthTEdateofentryintolabourmarkettmdateofmarriagepresprestigeofjobipresnprestigeofjobi+1eduyearsofformaleducationtfpdurationofjobepisodedescensoring(0=censored,1=notcensored)ReportDownload the Stata data file tda.dta from Nestor to a new working directory and open thedata file. The tda data file will be used to determine the hazard rates of changing jobs(mobility)basedonasetofvariables. 1.Explorethefollowingvariables:tfp,des,sexusingfrequencies,meansetc.Geta feelfortheorganisationandqualityofthedata.Recodethevariablesexinto0= menand1=women.sumtfpdessextab1tfpdessexinspect Note:withtab1youwillgetaone-waytableforeachvariablelisted.Theregularcommandtab(=tabulate)willnotworkformorethantwovariables. sex 600 1.42 .4939703 1 2 des 600 .7633333 .4253906 0 1 tfp 600 67.97 78.36633 2 428 Variable Obs Mean Std.Dev. Min Max 5 4 0.67 3.83 4 9 1.50 3.17 3 7 1.17 1.67 2 3 0.50 0.50 job episode Freq. Percent Cum.duration of (andmore…)10 Total 600 100.00 notcensored 458 76.33 100.00 censored 142 23.67 23.67 censoring Freq. Percent Cum. Total 600 100.00 women 252 42.00 100.00 men 348 58.00 58.00 2 women) Freq. Percent Cum.sex (1 men, recodesex(1=0"men")(2=1"women"),pre(new)Note:thisway,youformanewvariablewiththesamenameastheoldone,butwithprefix “new”.Sonowyouhavetwovariables:sexandnewsex.Insteadofpre(new),youcoulduse theoptiongen(newsex),whichwouldgiveyouthesamething. tabsexnewsex Total 348 252 600 women 0 252 252 men 348 0 348 women) men women Total men, 2 men, 2 women)) sex (1 RECODE of sex (sex (1. tab sex newsexThecrosstableaboveshowsthatthenewlyrecodedvariableiscorrect. 2.Set the database as being survival data, where the time-variable is tfp and thefailure-variableisdes(usecommandstset). stsettfp, failure(des)Note: as default, Stata will assume that des==0 or missing means censored and all othervalues(inourcase,value1)areinterpretedasrepresentingfailure.Thiscanalsobereadinthehelpfileofstset.Wecallitfailurewhentheeventofinterestoccurs.Inourdataset,thisdefaultsettingisthecorrectone,because des==0iscensoredanddes==1isnotcensored. 3.PerformaCoxregressionwiththecovariateSex.Computeboththehazardratioandthecoefficients.Makesuretoclusterthestandarderrorsacrosstheid.Whatis the parameter value of sex? Is it statistically significant? What is theinterpretationofthisparameter? stcoxi.newsex,vce(clusterid)11 women 1.52715 .1513132 4.27 0.000 1.257601 1.854473 newsex _t Haz. Ratio Std. Err. z P>|z| [95% Conf. Interval] Robust (Std. Err. adjusted for 201 clusters in id)Log pseudolikelihood = -2574.7456 Prob > chi2 = 0.0000 Wald chi2(1) = 18.26Time at risk = 40782No. of failures = 458No. of subjects = 600 Number of obs = 600Cox regression -- Breslow method for tiesIteration 0: log pseudolikelihood = -2574.7456Refining estimates:Iteration 2: log pseudolikelihood = -2574.7456Iteration 1: log pseudolikelihood = -2574.763Iteration 0: log pseudolikelihood = -2584.5701 analysis time _t: tfp failure _d: desThehazardratioforsexis1.52715,anditseffectisstatisticallysignificantlydifferentfromzero.Inthiscase,menarethereferencecategory,soitistheratioofthehazardoffemales/hazardofmales.Soifwewouldmultiplythebaselinehazardofmenwith1.52715,wewouldobtain the hazard of women. The model indicates that keeping all the other variablesconstant, women have a 52.72% higher hazard of changing jobs than men. This hazardratio is the exp(beta) and is the multiplicative effect of the independent variable on thehazard. stcoxi.newsex,vce(clusterid)nohrNote:byspecifyingnohr,wetellStatatoestimatecoefficients,nothazardratios. 12 women .4234031 .0990821 4.27 0.000 .2292059 .6176004 newsex _t Coef. Std. Err. z P>|z| [95% Conf. Interval] Robust (Std. Err. adjusted for 201 clusters in id)Log pseudolikelihood = -2574.7456 Prob > chi2 = 0.0000 Wald chi2(1) = 18.26Time at risk = 40782No. of failures = 458No. of subjects = 600 Number of obs = 600Cox regression -- Breslow method for tiesIteration 0: log pseudolikelihood = -2574.7456Refining estimates:Iteration 2: log pseudolikelihood = -2574.7456Iteration 1: log pseudolikelihood = -2574.763Iteration 0: log pseudolikelihood = -2584.5701 analysis time _t: tfp failure _d: desThecoefficientresultisthelog(hazardratio),soln(1.52715),andshowsthattheloghazardofchangingjobis+0.4234pointshigherforwomenthanitisformen. 4.Plotthepredictedsurvivalcurvesformalesandfemalesbya.predictingsurvivalvariablesformalesandfemales,andb.plottingbothpredictionsasalinegraph(command:line).Howdoyouinterpretthetwosurvivalcurvesofmalesandfemales? helplinestcoxi.sex,vce(clusterid)nohrbasesurv(surv0)generatesurv1=surv0^exp((0.4234031))labelvariablesurv0"men"labelvariablesurv1"women"linesurv0surv1_t,c(JJ)sortNote:basesurvcalculatesandsavesthebaselinesurvivorfunction,soatthereference categories,inthiscasemen. Note:_tistheanalysistimewhentherecordends.Option connect(c) incommandlinetells Statatoconnectpoints.Therearedifferentoptions,withJmeaningstairstep:flat,then vertical.OptionsorttellsStatatosortthedatabytheXvariablebeforeconnecting. 13 Thefemalecurveislowerthanthemalecurve:females‘survive’shorterinajob,meaningthey switchfaster between jobs (higher mobility). For instance,after 100 months about 35percentofmalesisestimatedtobestillinthejob,againstonlyabout20percentoffemales(estimatedfromthecurves). 5.Generatethevariable“cohort” from“tb”bycreating -value2(cohort1939-1941)fortb>=468&tb<=504, -value3(cohort1949-1951)fortb>=588&tb<=624, -value1forallothervalues(othercohorts). Furthermore, generate “lfx” as the difference between the starting time of thejobandthedateofentrytothelabormarket. Generate“pnoj” astheserialnumberofjobminus1. Using descriptive statistics, explore these new variable as well as edu and pres.Whichvariablesarecategoricalandwhichvariablesarecontinuous?genlfx =tstart-TEgenpnoj=noj-1recodetb(468/504=2"1939-41")(588/624=3"1949-51")(nonmissing=1"othercohorts"),gen(cohort) sumcohortlfxpnojedupres14 tabulatecohortCohortisacategoricalvariable.Theothervariablesaretreatedascontinuous. 6.Run another Cox regression. Remove the variable “sex” from the list ofcovariates and include the variables “edu, cohort, lfx, pnoj and pres”. Specifywhichvariablesarecategoricalandchooseareferencecategorythatmakesmostsense in terms of ease of interpretation. Estimate the model with coefficients.What is the interpretation of the parameters? Does the log likelihood improvesignificantlybytheinclusionofthevariables?15 pres -.0261678 .0060146 -4.35 0.000 -.0379561 -.0143795 pnoj .0686267 .0389654 1.76 0.078 -.007744 .1449975 lfx -.003989 .0010417 -3.83 0.000 -.0060307 -.0019474 1949-51 .3052514 .122002 2.50 0.012 .0661318 .544371 1939-41 .4113074 .1211748 3.39 0.001 .1738091 .6488057 cohort edu .0668593 .0270978 2.47 0.014 .0137486 .11997 _t Coef. Std. Err. z P>|z| [95% Conf. Interval] Robust (Std. Err. adjusted for 201 clusters in id)Log pseudolikelihood = -2546.7756 Prob > chi2 = 0.0000 Wald chi2(6) = 53.64Time at risk = 40782No. of failures = 458No. of subjects = 600 Number of obs = 600Cox regression -- Breslow method for tiesIteration 0: log pseudolikelihood = -2546.7756Refining estimates:Iteration 4: log pseudolikelihood = -2546.7756Iteration 3: log pseudolikelihood = -2546.7756Iteration 2: log pseudolikelihood = -2546.7823Iteration 1: log pseudolikelihood = -2548.0198Iteration 0: log pseudolikelihood = -2584.5701 analysis time _t: tfp failure _d: des ThereferencecategoryforCOHORTisthefirstone‘othercohorts’aswewanttoestimatetheeffectsspecificallyforthosepeoplebornin1939-41and1949-51.TheWaldchi2valueof53.64ishighlysignificant(p=0.0000)indicatingthattheinclusionofthevariablessignificantlyimprovesthemodel(improvesthelogpseudolikelihood). Note: we nowobtaina Wald chi2 instead of LR.Most important is that you know that thenullhypothesisthatWaldtestsisthatthecoefficientsofinterestaresimultaneouslyequaltozero,soyouinterpretthe“Prob>chi2”thesamewayaswithLR.WerejectthisH0becauseprob=0.0000.a.Oneadditionalschoolyearincreasestheloghazardofleavingby0.066points.thehighereducatedaremoremobile.b.The younger cohorts are more mobile, although this relationship appears to benonlinear as the middle cohort is more mobile than the youngest group. The loghazardis0.41pointshigherforcohort1939-41comparedtoothercohorts,and0.31pointshigherforcohort1949-51comparedtoothercohorts. c.Jobmobilitydecreasesby0.004pointsforeveryyearextraoflaborforceepxerience.d.PNOJisonlysignificantata10%confidencelevel.Wewouldhaveexpectedthatifsomeone tends to move between jobs (evidenced by a high serial number of the16 currentjob)thatwouldhaveapositiveeffectonthatpersonsexitrate.Thepositivecoefficientdoessuggestsuchrelation,althoughweak.e.Peopleinmoreprestigejobsarelessmobile(asexpected).Foreveryunitincreaseinjobprestige,theloghazardofjobmobilitydecreaseswith0.03points.7.Now addthevariableSEXtothemodel.Istheeffectofsexstillimportantandcomparable to the earlier model? Do the other effects change (sign, size andsignificance)asaresultoftheinclusionofsex? women .3698895 .0982921 3.76 0.000 .1772404 .5625385 newsex pres -.0249239 .0056655 -4.40 0.000 -.0360281 -.0138197 pnoj .0903056 .0408095 2.21 0.027 .0103205 .1702907 lfx -.0040866 .0010363 -3.94 0.000 -.0061177 -.0020555 1949-51 .2958657 .1218758 2.43 0.015 .0569934 .5347379 1939-41 .38596 .1128203 3.42 0.001 .1648364 .6070837 cohort edu .0763211 .0251799 3.03 0.002 .0269695 .1256728 _t Coef. Std. Err. z P>|z| [95% Conf. Interval] Robust (Std. Err. adjusted for 201 clusters in id)Log pseudolikelihood = -2539.6788 Prob > chi2 = 0.0000 Wald chi2(7) = 94.07Time at risk = 40782No. of failures = 458No. of subjects = 600 Number of obs = 600Cox regression -- Breslow method for tiesIteration 0: log pseudolikelihood = -2539.6788Refining estimates:Iteration 4: log pseudolikelihood = -2539.6788Iteration 3: log pseudolikelihood = -2539.6788Iteration 2: log pseudolikelihood = -2539.6867Iteration 1: log pseudolikelihood = -2541.043Iteration 0: log pseudolikelihood = -2584.5701 analysis time _t: tfp failure _d: desThecoefficientofsex(0.37)iscomparabletothefittedcoefficientinthefirstmodel(0.42),andstatisticallysignificant.Theconfidenceintervalsofthecoefficientofthepriormodelandinthismodeloverlap,sotheyarenotsignificantlydifferent. Theeffectsoftheothervariablesdonotchangesignandchangejustatinybitinsize.pnojchangesmostinsizeandbecomessignificantatthe0.05level.Apparentlytheeffectofthisvariablewasmaskedsomewhatbynotincludingsex.17 8.Make an LML plot (log-minus-log of survival function, command stphplot)based on each of the categorical variables using the variable of interest as astratumvariableafterexcludingitfromthemodel.Thisensuresyouwillgetanempiricalestimateofthedifferencebetweenthecategoriesratherthanamodeloutcome. Do you have indications for one or more variables if theproportionalityassumptiondoesnothold?stcoxedui.cohortlfxpnojpres,vce(clusterid) stphplot,strata(sex)adjust(cohort)Note:optionadjust adjuststoaveragevaluesofothercovariatesstcoxedu lfxpnojpresi.sex,vce(clusterid) stphplot,strata(cohort)adjust(sex)18 The LML functions for sex are largely parallel, although less so for short durations. TheLMLfunctionsforcohortarealsolargelyparallel,butthislooksbestformiddledurations.My own (Clara Mulder’s) conclusion from these plots would be that the proportionalityassumptionisvalidtoareasonableextentandthemodeloutcomescanbetrusted.19 1 Assignment 7 EventhistoryanalysisII ExerciseA The aim of this workshop is to perform a discrete-time event history analysis using logistic regressionofperson-years. Download the Stata data file eventhisfilepersonperiod.dta from Nestor to a folder of your choice in the usual way. This file is the same as the file you used for the Cox regression of leavingtheparentalhomebutithasbeentransformedintoaperson-yearfile. Rememberyou areonlyallowedtousethedatafile forthisworkshop.Therefore: afteryoufinish,deletethe datafilefromanyfolderorcomputeryouhavesavediton.Ifyouneedtore-runanyanalysis, justdownloaditfromNestoragainandre-runyour dofile. Explorethedatafile(noreportnecessary). 1.Run a regression estimating the odds of leaving the parental home with the independent variables sex and cohort. Decide if you need to correct for any clusteringofobservations. Interprettheresults. Note: The file includes person-years in which the respondent has already left home.Youcannotusetheseintheanalysis (notatriskanymore).Keep onlythose casesinwhichthedependentvariable‘left’hasvalue0or1. dropifleft== -1 dropifleft==2 (or:keepifleft==0|left==1) Wehaveabinaryoutcomevariable(left:0notyet,1left),sowerunabinarylogistic regression.Wecorrectforclusteringofobservations withinthesameindividual. logitlefti.sexi.cohno,vce(cluster pid) Ifwedonotspecifythisotherwise, logitwilluse thefirstcategory ofcategoricalvariables(i.) asbasecategory.Sointhiscase,i.cohnoisthe sameasspecifyingib1.cohort. Thecoefficient(beta)tellsusthatthelog-oddsof leavingtheparental home are0.298points higherforfemalescomparedtomales,holdingallelseconstant.Thismeansthatthe oddsof leavingtheparentalhome are(e 0.298 =) 1.347 times higher (or 34.7% higher)forfemales comparedtomales. Furthermore,thecohort resultsshowasignificantincreaseinthelog-oddsfortheyounger cohorts,whowerebornafter1900-24(referencecategory).Forinstance,thelog-oddsof leavingtheparentalhomeforcohort1955-64are0.776pointshigherthanthelog-oddsfor cohort1900-24.Theonlyexceptioniscohort1925-34,whichisnotsignificantlydifferent fromthereferencegroupofcohort1900-24. 2 2.Aswediscussedinthelecture,itisalwaysagoodideatotruncatetheobservation atatime whentheeventcanlogicallyno longer occur,or when,empirically,the population at risk gets too small (and the hazard starts fluctuating randomly) and/orthehazardgetstoosmall.Determinetheageatwhichyouwanttotruncate theobservationandpreparethedatasothatyourchoiceiseffectuated.Re-runthe analysiswiththenewlyprepareddata.Whatarethedifferencesinoutcomes? tabulateageleft Agein| Lefthome? years|not(yet) event| Total -----------+----------------------+---------- 16| 3,147 34| 3,181 17| 3,043 104| 3,147 18| 2,833 210| 3,043 19| 2,561 265| 2,826 20| 2,250 280| 2,530 21| 1,927 299| 2,226 22| 1,597 314| 1,911 23| 1,264 309| 1,573 24| 979 264| 1,243 25| 740 227| 967 26| 566 166| 732 27| 447 112| 559 28| 375 65| 440 29| 297 72| 369 30| 250 42| 292 31| 214 32| 246 32| 185 27| 212 33| 163 20| 183 _cons -2.78519 .0963071 -28.92 0.000 -2.973948 -2.596431 1965-74 .4765235 .1092618 4.36 0.000 .2623743 .6906727 1955-64 .7762045 .0998988 7.77 0.000 .5804064 .9720026 1945-54 .6490236 .1017396 6.38 0.000 .4496176 .8484295 1935-44 .3854441 .1063662 3.62 0.000 .1769701 .5939181 1925-34 .1819577 .1072481 1.70 0.090 -.0282446 .3921601 cohno Female .298148 .0313746 9.50 0.000 .236655 .3596411 sex left Coef. Std.Err. z P>|z| [95%Conf.Interval] Robust (Std.Err.adjustedfor2,813clustersinpid) Logpseudolikelihood=-9239.9526 PseudoR2 = 0.0107 Prob>chi2 = 0.0000 Waldchi2(6) = 309.97 Logisticregression Numberofobs = 27,560 Iteration4: logpseudolikelihood=-9239.9526 Iteration3: logpseudolikelihood=-9239.9526 Ite