Myoelectric Interfaces and Related Applications PDF
Document Details
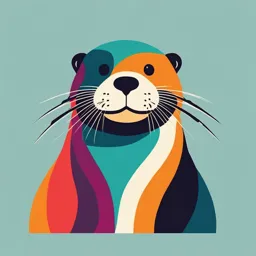
Uploaded by SuaveZirconium
null
Bernabe Rodríguez-Tapia,Israel Soto,Daniela M. Martínez,Norma Candolfi Arballo
Tags
Related
- EMG PDF
- Optimización y clasificación de señales EMG a través de métodos de reconocimiento de patrones (EMG) PDF
- Emergencias en Diabetes Mellitus PDF
- Myoelectric Interfaces and Related Applications: Current State of EMG Signal Processing PDF
- EMG Characterization and Processing in Production Engineering PDF
- Clinical Neurophysiology Intraoperative Monitoring PDF
Summary
This paper provides a systematic review of EMG signal processing techniques for myoelectric interfaces, focusing on their applications in rehabilitation technology, assistive technology and as an input device. The review covers sensor types, channel numbers, and pre-processing techniques.
Full Transcript
Received November 21, 2019, accepted December 19, 2019, date of publication January 3, 2020, date of current version January 15, 2020. Digital Object Identifier 10.1109/ACCESS.2019.2963881 Myoelectric Interfaces and Related Applications: Current State of EMG Signal Processing—A Systematic Review BE...
Received November 21, 2019, accepted December 19, 2019, date of publication January 3, 2020, date of current version January 15, 2020. Digital Object Identifier 10.1109/ACCESS.2019.2963881 Myoelectric Interfaces and Related Applications: Current State of EMG Signal Processing—A Systematic Review BERNABE RODRÍGUEZ-TAPIA 1,2 , ISRAEL SOTO 2, DANIELA M. MARTÍNEZ 1, AND NORMA CANDOLFI ARBALLO 1 1 Facultad de Ciencias de la Ingeniería y Tecnología, Universidad Autónoma de Baja California, Tijuana 21500, Mexico 2 Instituto de Ingeniería y Tecnología, Universidad Autónoma de Ciudad Juárez, Ciudad Juárez 32310, Mexico Corresponding author: Bernabe Rodríguez-Tapia ([email protected]) ABSTRACT The myoelectric interfaces are being used in rehabilitation technology, assistance and as an input device. This review focuses on an insightful analysis of the data acquisition system of EMG signals from these interfaces. According to applications reported in research articles of the last five years, the prop- erties of the sensors, the number of channels, the pre-processing of the EMG signal, as well as the software and hardware used were identified. This analysis was performed for the following applications: monitoring of muscular activation for rehabilitation, muscle activation plans, and identification of possible pathologies, exoskeletons, electric of wheelchairs, prosthetics control, myoelectric bracelets, handwriting recognition and silent speech recognition. The results presented in this review become a guide of recommendations for the myoelectric signal processing according to the application of the interface. The main developments, degrees of research and open challenges are also presented in this direction. INDEX TERMS Emg acquisition system, emg processing, electromyography sensors, myoelectric control, myoelectric signals. I. INTRODUCTION attached to superior limbs, have been used for controlling Electromyography (EMG) is a technique used to measure the devices by means of the electric impulses generated through muscle’s response to electrical stimulus of the nerves. The the muscles. EMG signal acquired from the skin surface around muscle Given all the possibilities for exploiting EMG signals, and joint areas is the summation of the electrical activity of it is critical to ensure that the data collected is reliable and all the muscle-fibred motor unit action potentials (MUAPs) that it is a precise representation of the electrical activ- caused as a result of motion activity. ity of the muscles. Therefore, an important research area, EMG signals have been relevant in several health fields. is the analysis of the specific application design require- The periodical monitoring of EMG signals can be utilized ments of the acquisition system considered for obtaining the to detect diseases like Huntington’s disease, Myopathies, myoelectric signals. Once these requirements are identified, or Muscular dystrophies, and to timely address problems it will be possible to provide widespread recommendations such as heart attacks or stroke occur ,. Furthermore, for the design of efficient and reliable EMG signal acquisition EMG signals could be useful to detect neuromuscular dis- systems. orders that could affect motor units (Mus) and to identify An EMG signal acquisition system consists of four main the origin of such disorders. Recently, in the Human- stages: (i) signal collection (ii) signal amplification, (iii) sig- Computer Interaction (HCI) field, the use of bio signals has nal filtering and (iv) analog-to-digital converting. Each stage opened the way for the development of muscle-computer demands specific requirements according to its operational interfaces. Particularly, EMG signals collected by sensors characteristics. These requirements can be specified in terms of the design parameters necessary for the implementation of The associate editor coordinating the review of this manuscript and EMG signal acquisition systems and will be further discussed approving it for publication was Shaohua Wan. in the rest of this document. This work is licensed under a Creative Commons Attribution 4.0 License. For more information, see http://creativecommons.org/licenses/by/4.0/ 7792 VOLUME 7, 2019 B. Rodríguez-Tapia et al.: Myoelectric Interfaces and Related Applications: Current State of EMG Signal Processing The method for collecting the EMG signal is by using elec- optimal sampling frequency, which makes it another impor- trodes. An electrode is a transductor that represents the level tant design parameter for the ADC stage. of muscle activity by recording the electrical activity in it. Insightful recommendations regarding non-invasive eval- Invasive approaches, also known as needle electromyography uation of muscles were provided by the European project (nEMG), record electrical activity through needle electrodes. SENIAM , published in 2000. Sensor type selection and Whereas, non-invasive approaches, also known as surface sensor placement were remarkable topics addressed in the electromyography (sEMG), record muscle activity from the recommendations. More than a decade later, the considera- skin surface through wet or dry surface electrodes ,. tions draw from SENIAM for sensor placement were updated The suitability of each approach depends on the feasibility. Moreover, key features as signal amplification, filtering of using an invasive or non-invasive approach to collect the and sampling rate were added to the previous recommenda- EMG signal. Although nEMG provides more features of tions. Recently, works have been developed to perform anal- the muscular activity, its main disadvantage emerges from ysis related to filtering, thinking about obtaining minimum the dynamics of muscular activities. Given the inherent inva- sampling frequency parameters to determine more favorable siveness of the approach, it is difficult to repeatedly reposi- conditions for processing time and its implementation in tion the needle electrode if multiple locations of the muscle portable acquisition systems ,. These contributions need to be analyzed. Thus, only a limited number of active have been very valuable for the pre-processing of EMG engine units can be measured. sEMG is preferable for signals, however, none of these previous works study the obtaining information regarding the duration or intensity of relationship among the configuration of the data acquisition superficial muscle activation. The majority of applications system and the specific application of the EMG signal. consider the non-invasive sEMG approach, as it is free of Therefore, this paper focuses on providing an insightful discomfort and observes extremely low risk of infection to analysis of the data acquisition system requirements, from a amputees. measurement and data pre-processing point of view, as related Two critical design parameters for the signal collection to the myoelectric interface for a specific application of the stage are the selection (type) and location (placement) of EMG signal. the electrodes. Given that the signal-to-noise ratio (SNR) depends on the place where the signal is collected, an optimal II. METHODOLOGY selection of the electrode placement is mandatory to achieve A. SEARCH STRATEGY an adequate SNR level –. On the other side, the open- In order to collect significant information regarding the loop gain (output-to-input ratio) as well as the input and parameters of EMG signal acquisition systems, a system- output impedances, are key parameters in the amplification atic search was conducted. The references were indexed stage. by the following keywords: emg acquisition system, emg Given the proximity of many other bio signals in the sur- sensors, electromyography sensors, myoelectric control and roundings of the muscle and power line interference, it is myoelectric signals. The search was conducted in the fol- expected that the collected EMG signals will contain unde- lowing databases: IEEE Xplore R , SCOPUS, Springer and sired features that may obscure important information regard- ScienceDirect search engine to determine the state of the art ing the electrical activity of the muscle. Hence, the optimal of the topic. design of the filtering stage is critical to exclude all the unwanted frequency components. Several variables must be considered in order to efficiently design the filtering stage: the B. REVIEW PROCESS selected muscles, the type of contraction, the configuration Articles found after the previously described search were of the sensor and the source of the specific noise. The evaluated by analyzing the title and the abstract. The fol- characteristics of the amplifiers and filters will determine the lowing criteria was considered for final selection of articles: quality of EMG signal. (i) Articles written in English; (ii) articles published from After the EMG signal is amplified and filtered, it is fed 2014 to 2018; (iii) comprehensive initial search results that into an analogue-to-digital converter (ADC) circuit. For included journal articles only. each specific application, EMG signals must be processed The study was conducted using the systematic review by means of advanced signal processing algorithms, which method proposed by Preferred Reporting Items for Sys- are commonly implemented in computer systems. Therefore, tematic Reviews and Meta-Analysis (PRISMA), as shown the analogue-to-digital conversion stage needs to be carefully in Fig. 1. designed. Three main variables must be considered during the design of the ADC stage: the open-loop gain considered C. INCLUSION CRITERIA during the amplification stage, the maximum output voltage Articles found after the previously described search were at the back-end of the EMG signal acquisition system, and evaluated by analyzing the title and the abstract. The fol- the additive noise. Moreover, in order to reconstruct digitized lowing criteria was considered for final selection of articles: signals with minimal errors, it is necessary to determine the (i) Articles written in English; (ii) articles published from VOLUME 7, 2019 7793 B. Rodríguez-Tapia et al.: Myoelectric Interfaces and Related Applications: Current State of EMG Signal Processing TABLE 1. Parameters considered during the reference scan process. FIGURE 1. Flowchart of searching and screening strategy. 2014 to 2018; (iii) comprehensive initial search results that included journal articles only. III. RESULTS A. STUDY SELECTION The aforementioned applications are classified by From the 12,427 results found in the databases, only 3,886 Hakonen into three categories: (1) Rehabilitative tech- did not duplicate. From these, 3,345 were discarded after nology, that includes activation of exoskeletons and moni- title screening. 541 works were selected to perform abstract toring of muscle activation which is useful for the detection review, resulting in the selection of 243 papers for full-paper and prevention of health problems, as well as for activation review. After the full-paper review process, 30 papers were and strengthening of muscular structures; (2) assistive tech- selected according to the fulfillment of the specifications nology, involving the control of prosthetics and motorized shown in Table 1. It is important to note that although there is wheelchairs by means of EMG signals; and (3) technology a significant amount of publications in the field, only a small as an input device, which includes the use of myoelectric number of publications address the specifications regarding bracelets for the identification of gestures or sign language, the design of EMG signal acquisition systems. myoelectric interfaces for writing interpretation and sketch- All the applications found during the screening process are ing, and myoelectric sensors for silent speech recognition. listed below: After the comprehensive review of the 30 selected papers, Monitoring of muscular activation for rehabilitation, it was found that the requirements of the EMG signal acqui- muscle activation plans, and identification of possible sition system vary from one application to another. In this pathologies , , –. sense, the objective of the present review is to analyze these Support mechanisms based on the estimation of muscu- requirements to provide an adequate property classification lar strength, known as exoskeletons ,. and to define the recommended operational characteristics of Electric control of wheelchairs ,. the EMG signal acquisition systems for each of the previously Prosthetics control –. listed applications. Command control by means of myoelectric bracelets –. B. STAGE ANALYSIS OF THE EMG SIGNAL Handwriting recognition from EMG signals for control- ACQUISITION SYSTEM ling computer peripherals and identification of patholo- EMG systems are characterized for acquiring, analyzing and gies as Parkinson’s disease and dysgraphia –. processing complex bio signals. These bio signals are small Surface EMG-based sketching recognition for the devel- in amplitude but very rich in information, and given that they opment of electronic sketching systems and computer- are controlled by the nervous system, they depend on the aided sketching systems ,. anatomy and physiological properties of the muscles. Silent speech recognition by means of myoelectric sen- The electrical characteristics of EMG signals reported in the sors interface –. reviewed works are listed in Table 2. In the table, it can 7794 VOLUME 7, 2019 B. Rodríguez-Tapia et al.: Myoelectric Interfaces and Related Applications: Current State of EMG Signal Processing TABLE 2. Electrical characteristics of the EMG signal. as well as the software and hardware used, both tables were constructed from the information extracted from the 30 reviewed articles. 1) SENSING STAGE a: PROPERTIES OF THE EMG SENSOR In a myoelectric interface, electrodes are used to detect the biological potential that is generated due to muscle contrac- TABLE 3. Harmful interferers that affect the recording of the EMG signal. tion. EMG signals can be analyzed independently or along with other bio signals that are useful to the better understand- ing of the muscle’s movement. The number of implemented sensors is related to the type of movement that is analyzed, and the amount of information provided by each channel needs to be considered for further processing. Properties such as construction material, configuration and size are variables that need to be considered when designing and implementing the signal acquisition system for each specific application. In this sense, the properties of the sensors considered in the 30 papers selected for this systematic review, were thoroughly be identified that the amplitude in voltage of a muscular analyzed. It is worth mentioning that the selected works cover contraction can vary from 0 to 10mV and the energy is within most of the applications of EMG signals discussed in the the first 500 Hz in the frequency spectrum. previous section. It can be observed in Table 4, that 9 out of On the other hand, Table 3 presents a collection of harm- the 30 research works correspond to applications falling into ful interferers that may affect the performance of the EMG the rehabilitation technology category, 10 out of the 30 works system. Among the main interferences, the following were fall into the assistive technology category, and finally, 11 out identified: moving artifacts affecting low frequencies, trans- of the 30 works belong to the technology as an input device mission line that can affect the frequency of 50 or 6OHz category. depending on the energy sub-ministered of each country, sat- uration of amplifier, physiological interference, specifically, b: SENSING TECHNIQUE (SUPERFICIAL OR the electrocardiogram and noise bio signal. INTRAMUSCULAR) Due to the characteristics described above, EMG signals require specific treatment to take the full advantage of the Bioelectrical activity inside the muscle of a human body is information provided by them. Therefore, the design of the detected by means of EMG electrodes. There are two main EMG signal acquisition system stages must be carried out in types of EMG electrodes: superficial (skin surface electrodes) such a way that this specific treatment is effectively achieved. and intramuscular (needles and thin wires). In general terms, an EMG signal acquisition process requires From 30 works shown in table 4, 28 resorted to the superfi- two main activities: signal sensing and signal pre-processing. cial technique for gathering the EMG data, one work consid- Several authors agree in dividing pre-processing of EMG sig- ered the intramuscular technique (sEMG), and one employed nals into three stages: amplification, filtering, and analog-to- a fusion of both techniques (hybrid approach). Of the works digital conversion , , , ,. Hence, we will that did not report using surface technique, one of the two consider that EMG signal acquisition systems are comprised researches used the intramuscular technique which is focused by four stages: (1) sensing, (2) amplifying, (3) filtering, and on EMG signal classification methods for identifying health (4) analog-to-digital conversion. problems. Signal classification method proposed in As stated before, the main goal of this review is to clas- allows discriminating among normal, myopathy and Amy- sify the application-specific properties of each stage of the otrophic Lateral Sclerosis (ALS) patients. On the other hand, EMG signal acquisition system and to draw recommenda- the work that considered the hybrid approach addresses tions regarding the design requirements for the stages. In this the problem of prosthetics control by means of signal sense, Table 4 abstracts the main properties identified for the classification. sensing stage. The information in Table 4 was completed by identifying in each research article what type of technique c: TYPE OF ANALYZED SIGNAL was used, superficial or intramuscular, if only the EMG sig- As stated before, it is possible that the sensors collect other nal was analyzed or in conjunction with another bio signal, bio signals along with the EMG signal. These bio signals may how many channels were used, and the characteristics of the differ according to the application considered. The analysis sensors used. of the signals used in the studies proposed in each of the While Table 5 summarizes the properties related to the 30 reviewed works, highlights that 21 works consider only amplifying, filtering, and analog-to-digital conversion stages EMG signals, while the remaining 9 publications consider VOLUME 7, 2019 7795 B. Rodríguez-Tapia et al.: Myoelectric Interfaces and Related Applications: Current State of EMG Signal Processing TABLE 4. Properties of the sensors according to the application type (N=30). other signals as: strength, signals acquired by IMU sensors, applications require knowledge regarding additional informa- electrocardiogram (ECG) signals, pressure, acceleration, tion as position, speed, rotation and force to improve the effi- space orientation, electrodermal activity (EDA), adaptive ciency of parameters interpretation. The use of other sensors cruise control (ACC), illuminance (LUX), elbow movement, for monitoring different bio signals has also been reported, photo plethysmography (PPG), bioelectrical impedance anal- although they are not necessarily analyzed all together with ysis (BIA) and position tracking. the EMG signals. In Table 4 it is remarked that the papers that involve the measurement of other bio signals in addition to the EMG d: NUMBER OF EMG CHANNELS signals are, in general, those that study signal classification The number of channels used in an EMG signal acquisition methods for prosthetic control. This is mainly since these system is related to the muscles intended to be analyzed. 7796 VOLUME 7, 2019 B. Rodríguez-Tapia et al.: Myoelectric Interfaces and Related Applications: Current State of EMG Signal Processing TABLE 5. Pre-processing features according to the application (N=30). VOLUME 7, 2019 7797 B. Rodríguez-Tapia et al.: Myoelectric Interfaces and Related Applications: Current State of EMG Signal Processing TABLE 5. (Continued) Pre-processing features according to the application (N=30). hand, periodic expressions as chewing, talking, gargling, and temporary expressions as sadness, surprise, happiness, pout- ing and anger, could be recognized by employing a minimum of three channels. The number of channels documented in applications for the interpretation of command gestures is four ,. These applications are typically used in electrical powered wheelchairs to control functionalities as: mov- ing, stopping, moving forward and reversing. Also, four channels are used in applications for simple hand gesture recognition through myoelectric bracelets , ,. FIGURE 2. Number of channels used for each application. 1 channel , , , ; 3 channels ; 4 channels Exoskeletons , Electric Common uses for these applications are virtual reality gam- powered wheelchair control , , Myoelectric bracelets , ing, computer or mobile phones control, or sign language Handwriting recognition , Silent speech recognition ; interpretation. 6 channels and ; 8 channels Exoskeletons and , Myoelectric bracelets , Handwriting recognition , Works that address applications as writing recognition and and , Prosthetics control , and and 10, 12, 16 y exoskeleton activation have documented the use of, at least, 24 channels , and –. six and eight channels. The number of electrodes used in the prosthesis can vary from 1 to 32 electrodes. The impor- This design parameter will directly affect the amount of infor- tance of this configuration lies in its electro-accuracy control, mation that needs to be processed during the classification production cost, computational load, the type and number of and data interpretation stage of the myoelectric system. tasks to be achieved and degrees of freedom (DoFs). From the analysis shown in the Table 4 is found out that the number of channels is directly related to the specific e: SENSOR FEATURES (CONFIGURATION, CONSTRUCTION application of the EMG signal. Fig. 2 depicts the relation- MATERIAL AND SIZE) ship between the numbers of channels used per application According to the investigation reported in , there are addressed in the thirty reviewed papers. Articles reported three different sensor configurations: monopolar, bipolar and having used between 1, 3, 4, 6, 8, 10, 12, 16 and 24 channels. array/line electrodes. By analyzing the sensor configuration At the bottom of the figure the reference is shown according considered in each of the 30 reviewed works, it was observed to the channel number and its application. that the bipolar configuration is the most used. Only 3 out of According to the information provided by the reviewed the 30 works consider the monopolar configuration, and none papers and Fig. 2, one single channel could be enough for of them consider the array/line sensor configuration. transmitting the muscular activity data necessary for muscu- On the other hand, a desirable feature for data recording lar activation monitoring systems. However, if pattern recog- electrodes is their capability for avoiding overpotentials due nition methods are going to be applied for identifying specific to polarization. A silver chloride (Ag/AgCl) electrode com- limb pathologies, then considering up to ten channels might plies with this feature. In this regard, 13 out of the 27 be necessary. works that consider bipolar sensors, reported that the sen- For silent speech recognition applications, studies report sors were constructed using Ag/AgCl, while the remaining that a considerable amount of words and phrases could 14, do not specify the construction material of the sensors be interpreted by using four channels. On the other employed. 7798 VOLUME 7, 2019 B. Rodríguez-Tapia et al.: Myoelectric Interfaces and Related Applications: Current State of EMG Signal Processing bracelet, which is manufactured by MYO from Thalmic Labs. Thalmic Labs does not provide further information regarding the amplifier stage of the acquisition system of the bracelet. 3) FILTERING STAGE As discussed previously, EMG signals can be affected by other intended or non-intended signals, for example other bio signals (ECG, EEG, etc.) or radiofrequency emissions (cellphones, Wi-Fi, Bluetooth, etc.). Furthermore, tempera- ture fluctuations, compensations in amplification stage, char- acteristics of the sensor, etc., can also affect the waveform FIGURE 3. Percentage of the works per gain type. of the EMG signal. All these undesired signals and random variations can be modelled as noise whose frequency charac- Regarding the size of the sensor, only 10 out of 30 papers teristics may vary depending of the actual noise sources. provided a detailed description of the sensor size and shapes. In this sense, the filtering stage in a signal acquisition system The information is summarized below: is of paramount importance to reduce the detriment of the Rectangular: 41mm x 44mm x 9.4mm, 19.8mm x 35mm, EMG signal caused by noise. 5cm x 3.5cm, 10mm x 20mm x 3mm According to its frequency response, the filters reported in Circular: 8mm, 10mm, 12mm y 5.7cm the reviewed works on Table 5 can be classified into three Needle electrode: 0.07mm categories: (1) Band-pass filters: 2) AMPLIFYING STAGE 500 Hz, 3rd order As mentioned before, EMG signals are very weak. This 5-500 Hz, 2nd order characteristic is exacerbated in some specific muscles. There- 10-200 Hz fore, it is expected that the amplifier gain design must be 10-400 Hz , 3rd order , tailored according to the application in relation to the mus- 10-500 Hz , , cles involved. 14 out of the 30 reviewed works reported the 20-250 Hz 2nd order amplifier gain considered for their systems, the results are 20-450 Hz shown in Fig. 3, considering that 100% corresponds to the 20-500 Hz , 2nd order and 4th order , 14 papers that report the gain type and value used for their 30-300 Hz 4th order systems, it was identified that over the majority of the works, 2-750 Hz 36%, documented earnings between 500 and 1000 over the 13 works do not specify the characteristics of the filter- original signal. ing stage The information provided in Figure 3 can be further (2) Notch filters: 50 Hz and 60 Hz (depending on the described to classify the gain value used per specific appli- frequency of the electrical network in each country). cation: (3) Low-pass filters: Myoelectric bracelet: Gain of 1000. 2 and 10 Hz Prosthetic control: Programmable gain amplifiers , 20 Hz ,. 7 Hz Exoskeleton: Programmable gain from 2000 to 16 Hz 5000. Handwriting: Gain of 1000 , and of 2000. 4) ANALOG-TO-DIGITAL CONVERSION Muscular activation monitoring: Gain of 600 , Applications as pattern recognition or device control through of 1000 , of 4000 , low noise and variable gain EMG signals take place after the EMG signal is converted amplifier. to a digital format. Hence, the sampling frequency and quan- Electric powered wheelchair control: Gain of 455. tization levels of the analog-to-digital converter (ADC) are From the works that do not specify the amplifier gain, important design parameters that must be thoroughly ana- 5 of them are related to prosthetic control applications, 1 to lyzed for each specific application. muscular activation monitoring, 2 to exoskeleton activation, Regarding the sampling frequency, 24 out of the 30 works 3 to writing recognition, 1 to myoelectric bracelet, 1 to from Table 5 presented a detailed report of the sampling electric powered wheelchair control, and 1 to silent speech frequency considered in their applications, summarized as recognition. follows: 21 papers reported sampling frequency values rang- It can be assumed that the lack of information on this matter ing from 1000 to 1500 samples per second; for the remain- is mainly due to the unavailability of the specific parame- ing 3 papers, the sampling frequency ranges from 2000 to ters of design in commercial devices. For example, in 23434 samples per second. The latter are related to appli- the hand control application is based on the myoelectric cations that resort to classification methods for pathology VOLUME 7, 2019 7799 B. Rodríguez-Tapia et al.: Myoelectric Interfaces and Related Applications: Current State of EMG Signal Processing MATLAB (The Mathworks Inc., Natick, EE. UU.) They are mainly used as programming languages. Currently, there are also systems called ‘‘open -source hardware’’, based on platforms like Arduino, Raspberry Pi, Atmel, Pololu, among other whose hardware, software and mechanical design files are available online offering a solu- tion to the high costs and slow pace of innovation of medical devices. FIGURE 4. ADC resolution reported in some EMG signals applications. 8 bits ; 10 bits ; 12 bits Pathology detection muscular activation IV. DISCUSSION , , Exoskeletons , Prosthetic control ; 14 and 16 bits The use of the myoelectric signals has extended in the last Handwriting recognition , Prosthesis control and 24 bits Prosthetic control ,. decades for diverse applications: in its commercial use, it is more remarkable in the medical and rehabilitation area; how- ever, it has gained ground in mechanisms of support to the detection through muscular activation, prosthetic control and upper extremities such as the prosthetics and exoskeletons. writing interpretation. Challenges remain and there are various applications that On the other side, the resolution of the ADC is reported continue to be worked in the laboratory with the challenge in 12 out of the 30 works from Table 5. The classification of of becoming new and ergonomic input peripherals on the the resolution considered in these research works is presented HMI, as are the myoelectric bracelets, the handwriting and in Fig. 4, where it is shown that the ADC can vary between 8, silent speech recognition. In order to add to this branch of 10, 12, 14, 16 and 24 bits, with ADC converters of 12, 14 knowledge, in this review and according to the application, and 16 bits being more used. At the bottom of the figure, the the technical aspects of the main signal acquisition system reference is shown according to the number of bits and their were identified along with important differences in the con- application. figuration of these. The main findings found at each stage are According to the classification provided in Figure 4, it is shown below. possible to infer that applications that require simpler ges- tures interpretation would require lower resolution, as com- A. SENSING STAGE pared to that requiring more complex gestures interpretation, Although there are different techniques to acquire the myo- as in, for example, writing recognition or prosthetic control electric signals, there are enough results in the analyzed with higher degrees of freedom, where higher resolution, works to consider that the superficial type technique (sEMG) ADC is necessary. is the most appropriate when you want to develop more ergonomic devices and equipment for commercial use. The 5) SOFTWARE AND HARDWARE most used sensors on investigations were those of biopo- According to Table 5 it can be seen that there is a diverse tential configuration with silver chloride material (Ag/AgCl) range of commercial systems developed for the monitoring since it has been shown to have the appropriate standards for of EMG signals, these may vary according to the num- the myoelectric interfaces due to its impedance characteris- ber of synchronized channels and the use of these sig- tics. nals. Each of these systems develop the processing and The type of shape and measurements of the sensor have visualization in closed-source hardware/software systems. their variations, however, the most common are the round However, the commercial software of these owners often of 8mm and 10mm diameter. This information is consistent have the opportunity to provide ways to export files for with the recommendations given in the European project use on other platforms such as Matlab, Excel, Labview SENIAM published in 2000. among other. They can be exported by ‘‘Selected signal(s)’’ Regarding the relationship in the number of channels per or ‘‘Raw data set’’. The export of these can be done application, it was identified that according to the number of in different types of files: Bynary, CSV o Matlab files. muscles needed for a more efficient analysis, will be the num- Some suppliers offer the opportunity to access the differ- ber of channels to utilize. This trend, however, in research, ent sensors with some adaptation to be read by Simulink, is to decrease the number of channels. For this, the techniques for example. of electrode location as sensors placement procedures for On the other hand, the works report the development of the sensors sEMG and Crosswalk studies , , are owned systems for research purposes, these systems are usu- important aspects to consider for the reduction or the correct ally just as reliable as the commercial ones and have a degree selection of muscles and channels. of flexibility adequate for analysis and research purposes. According to the analyzed investigations, works with only The general architecture is represented in the type of sen- 3 channels, were able to recognize periodic expressions such sors, the type of amplifiers, the converter card A/D, and the as: chewing, speaking, gargling and transient expressions microcontroller that controls the process. C++, LabVIEW such as: sadness, surprise, happiness, pout and anger. For the (National Instruments Corp., Austin, TX, EE. UU.) and gesture’s recognition defined through myoelectric bracelets, 7800 VOLUME 7, 2019 B. Rodríguez-Tapia et al.: Myoelectric Interfaces and Related Applications: Current State of EMG Signal Processing electric control of wheelchairs and of silent speech recogni- by the artifacts and to preserve the desired signal, this way, tion, 4 channels are commonly used. 8 channels for applica- the majority of the systems utilizes filters between 10 and tions of prosthetics control more than 1 DoF, exoskeletons 500 Hz. However, indicates that utilizing the high-pass and handwriting recognition; 10, 16 and 24 are the most angle frequency of 20 Hz offers the best compromise to opti- utilized channels for complex systems of prosthetics control mize the informational content desired of the SEMG signal, for an important variety of movements. since at low frequencies of the spectrum frequency, noise The design of the surface electrodes can be classified as: sources are involved which overlap with the SEMG signal, 1) Muscle-oriented design, precise location of the muscle for this reason, the determination of filtered characteristics in is necessary when using and adhering pairs of electrodes, this region, has been a focus of attention. 2) Arrangement of the low-density surface electrode (LD), The order of the reported filters can vary from first to it is necessary to have electrodes in certain patterns and fourth order, however, comparisons have been made between distribute them uniformly in the skin forming ring structures the second and fourth order Butterworth high pass, confirm- or belts, what is also known as uniform electrode positioning. ing that a second order can replace the high-pass filter of the The number of channels can vary from 2 to 16, 3) Arrange- fourth order. ment of high density surface electrodes (HD), which collects Although the modern technology is substantially immune the EMG signals from the closely spaced electrodes, allows to some noises, it is not so for the reference noise, such exploiting spatial information through the muscles, therefore, is the case of the noise generated by the power lines. The this strategy can be useful for the study of complex dynamic works reported using the notch filter for frequencies of 50 or tasks in the free space with a greater number of DoFs. How- 60 according to the standards in each country. ever, it remains a challenge to deal with many EMG channels to interact with a practical prosthetic hand. D. ANALOG-TO-DIGITAL CONVERSION The similarities on the works continue per the frequency B. AMPLIFICATION STAGE sampling, most of the scanned works, documented to have From the analyzed documents, it was identified that there is used sampling frequency of 1000 Hz, as stablished by the not much of attention in documenting precise characteristics sampling theory of Nyquist, which must be equal or less than of amplification and filtering. This is more recurrent on the half the speed of sampling of the signal, which it is known as applications with a greater number of channels, as in myo- the greater power (approximately 95%) of the signals, sEMG electric control; however, they documented having worked is explained by harmonics of up to 400 – 500 Hz. However, with programmable amplifiers. This makes sense because some differences were noted: worked with a sampling the efficiency of the classifiers focuses on patter recognition frequency of 23428 Hz, with 2048 Hz and increased and classification algorithms about muscular regions not on up to 10,000 Hz. a single muscle. It is observed that those works that used a greater number The Works that documented the gain value for applications of channels also increased the frequency. Although the use of such as monitoring of muscular activation, the gesture inter- a higher sampling frequency can acquire more myoelectric pretation like electric control of wheelchairs, the handwriting information that can increase the precision of the motion recognition and the silent speech recognition, converge on the classification, this adds more computational complexity and need to amplify the signal with a gain of 500 – 1000 unlike analysis, as well as memory requirements. Some works such work which proposes an amplification of 400. as have shown that it is possible to decrease the sampling Without a doubt, the gain and noise characteristics that frequency up to 500 Hz were you can save approximately these signals have, become complex and although the largest 50% storing memory and reduce 50% data processing time amplitude is desired, you have the risks of saturation in the with a slight accuracy sacrifice (around 2%). amplifier. In , the need for low noise amplifier (LNA) The ADC conversion was achieved with resolutions of 8, and considering the risk of saturation on the amplifiers is 10, 12, 14, 16 and 24 bits. In monitoring applications, a reso- mentioned. The consideration to incorporate a preamplifier lution sufficiency of 8 bits is shown, unlike electric control of is to have a high common mode rejection ratio (CMRR) and wheelchairs and control by means of myoelectric bracelets, high input impedance. which are more efficient to use in a 10-bit resolution. The application that used 12, 14 and 16 bits are those that desire C. FILTERING STAGE to interpret more complex pathologies or gestures such as The signal sEMG is inevitably contaminated, therefore, it is prosthetics control, exoskeletons, handwriting recognition. necessary to consider the different factors to determine the The works that document the use of 24 bits are those that specifications of the filtering which include: the selected interpret complex gestures determined by the movement of muscles, the type of contraction, the configuration of the upper extremities. sensor and the source of the specific noise and adds that Although the ADC conversion varies for each application, it’s important to consider the corner frequency, the roll-off states that a 16 bit converter may be preferable for rate and the circuit topology chosen. The determination of the any acquisition system, since the aggregate resolution may band-pass is important to reduce noise and the contamination eliminate the need for manual gain of each EMG amplifier. VOLUME 7, 2019 7801 B. Rodríguez-Tapia et al.: Myoelectric Interfaces and Related Applications: Current State of EMG Signal Processing E. SOFTWARE AND HARDWARE STAGE The stages of the EMG data acquisition system include According to the results of the documents analyzed, census, amplification, filtering and digital analog conver- 3 types of systems were identified; each of them used sion. When reviewing a variety of papers documented by for specific purposes due to its hardware and software the researchers, it is concluded that the surface technique characteristics. is preferable in its biopotential configuration for the EMG High-end data acquisition platforms. These systems are signal census, the amount of sensors used may vary accord- used for medical and prosthetics applications whose main ing to the application of the interface, for example, inter- characteristics are: off-line processing, they do not focus pretation applications of simple gestures such as motorized on optimization for resource constrained platforms such as wheelchairs control or signal monitoring, use fewer sensors wearables, can obtain high gesture classification accuracy, than the control of prosthetics or the use of exoskeletons; the the used algorithms are not documented and design of real majority of the works documented gains between 500 and time efficient systems is still a challenge. 1000 on the original signal, the considerations to incorpo- Embedded data acquisition platforms: Systems used for rate amplifiers is to have a high CMRR and a high input research purposes, these systems are usually: open source impedance; the most common filtering has to do with the platform, meet real-time requirements, are low power sys- elimination of interference from power lines, the movement tem. For the development of a wearable and low-power sys- of artifacts and other bio signals detected. Butterworth filters tem, targeting high accuracy, the most promising approach of the second or fourth order were the most reported; the most seems to be the synergy between a low power Analog documented sampling frequency was 1000 Hz, as established front-End (AFE) and a microcontroller, merging the sys- by the Nyquist theorem, however, wireless systems usually tem flexibility with a good signal quality and maintaining a use frequencies lower than 250 Hz and higher frequencies good trade-off between power consumption and computing in medical platforms, the resolution of the analog-digital capabilities ,. converter varies according to the application although, 16-bit Low cost wearable device. Used for interactive appli- converters are preferable. cations. Currently, the most interesting solution for wear- According to the results of the documents analyzed, three able EMG gesture recognition is the MYO armband, from types of systems were identified that allow the acquisition of Thalmic Labs. This is a wearable and low-cost device High-end data acquisition platforms, Embedded data acqui- equipped with EMG and inertial sensors. It connects to a sition platforms and Low-cost wearable device, each using PC or tablet via Bluetooth Low Energy (BLE) and allows hardware and software features according to their purpose. both raw data streaming and the use of a proprietary library The results presented in this review can already be recom- for gesture recognition. The signal processing is performed mendation guides for the design of emg signal acquisition and on the host platform and the used algorithms are not doc- processing systems according to their application, however umented. Nevertheless, the device presents low flex- the recommendations are based on the common practices of ibility in terms of possible applications because it lacks the researchers, in order to obtain reliable and optimal crite- embedded computing capabilities and cannot be used as a ria, it is pending to carry out a study with the same approach, stand-alone system. paying attention to the processing times, computation cost and the classification results for those interfaces that involve V. CONCLUSION the interpretation of gestures. Although there are recommendations made by regard- ing the non-invasive evaluation of muscles and those made REFERENCES by –,which make references to key features such Y. Athavale and S. Krishnan, ‘‘Biosignal monitoring using wearables: as amplifier, filter and sampling frequency. The approach Observations and opportunities,’’ Biomed. Signal Process. Control, vol. 38, proposed in this review allowed us to study the relationship pp. 22–33, Sep. 2017, doi: 10.1016/j.bspc.2017.03.011. between the configuration of the data acquisition system and P. Konrad, ‘‘Signal origin and acquisition,’’ in A Practical Introduction to Kinesiological Electromyography. Scottsdale, AZ, USA: Noraxon, 2006, the specific application of the signal. ch. 1, pp. 5–16. The aforementioned applications can be classified into A. Narayan, H. Dasgupta, and H. Pal, ‘‘Instrumentation of a portable EMG three categories: (1) Rehabilitative technology, that includes sensor in a single PCB for human motion pattern detection,’’ in Proc. 2nd Int. Symp. Phys. Technol. Sensors, Dive Deep Into Sensors (ISPTS), 2015, activation of exoskeletons and monitoring of muscle activa- pp. 1–5, doi: 10.1109/ISPTS.2015.7220069. tion, useful for the detection and prevention of health prob- H. P. Da Silva, A. Fred, and R. Martins, ‘‘Biosignals for everyone,’’ lems, as well as for activation and strengthening of muscular IEEE Pervasive Comput., vol. 13, no. 4, pp. 64–71, Oct. 2014, doi: structures; (2) assistive technology, involving the control of 10.1109/mprv.2014.61. G. R. Naik, S. E. Selvan, and H. T. Nguyen, ‘‘Single–channel EMG classifi- prosthetics and motorized wheelchairs by means of EMG cation with ensemble-empirical-mode-decomposition-based ICA for diag- signals; and (3) technology as an input device, which includes nosing neuromuscular disorders,’’ IEEE Trans. Neural Syst. Rehabil. Eng., the use of myoelectric bracelets for the identification of vol. 24, no. 7, pp. 734–743, Jul. 2016, doi: 10.1109/tnsre.2015.2454503. gestures or sign language, myoelectric interfaces for writ- T. S. Saponas, D. S. Tan, D. Morris, and R. Balakrishnan, ‘‘Demonstrating the feasibility of using forearm electromyography for muscle-computer ing interpretation and sketching, and myoelectric sensors for interfaces,’’ in Proc. 26th Annu. CHI Conf. Hum. Factors Comput. Syst. silent speech recognition. (CHI), Florence, Italy, 2008, p. 515, doi: 10.1145/1357054.1357138. 7802 VOLUME 7, 2019 B. Rodríguez-Tapia et al.: Myoelectric Interfaces and Related Applications: Current State of EMG Signal Processing G. R. Naik, K. G. Baker, and H. T. Nguyen, ‘‘Dependence independence J. Rosen, M. Brand, M. Fuchs, and M. Arcan, ‘‘A myosignal-based pow- measure for posterior and anterior EMG sensors used in simple and ered exoskeleton system,’’ IEEE Trans. Syst., Man, Cybern. A, Cybern., complex finger flexion movements: Evaluation using SDICA,’’ IEEE J. vol. 31, no. 3, pp. 210–222, May 2001. Biomed. Health Inform., vol. 19, no. 5, pp. 1689–1696, Sep. 2015, doi: R. Mahendran, ‘‘EMG signal based control of an intelligent wheelchair,’’ 10.1109/jbhi.2014.2340397. in Proc. Int. Conf. Commun. Signal Process., Melmaruvathur, India, M. Ibitoye, E. Estigoni, N. Hamzaid, A. Wahab, and G. Davis, ‘‘The Apr. 2014, pp. 1267–1272. effectiveness of FES–evoked EMG potentials to assess muscle force and A. S. Kundu, O. Mazumder, P. K. Lenka, and S. Bhaumik, ‘‘Omnidirec- fatigue in individuals with spinal cord injury,’’ Sensors, vol. 14, no. 7, tional assistive wheelchair: Design and control with isometric myoelectric pp. 12598–12622, Jul. 2014, doi: 10.3390/s140712598. based intention classification,’’ Procedia Comput. Sci., vol. 105, pp. 68–74, R. Merletti and C. J. De Luca, ‘‘New techniques in surface electromyog- Dec. 2017, doi: 10.1016/j.procs.2017.01.200. raphy,’’ in Computer Aided Electromyography and Expert Systems, vol. 9, J. M. Hahne, S. Dahne, H.-J. Hwang, K.-R. Muller, and L. C. Parra, no. 3. Amsterdam, The Netherlands: Elsevier, 1989, pp. 115–124. ‘‘Concurrent adaptation of human and machine improves simultaneous and R. Chowdhury, M. Reaz, M. Ali, A. Bakar, K. Chellappan, and T. Chang, proportional myoelectric control,’’ IEEE Trans. Neural Syst. Rehabil. Eng., ‘‘Surface electromyography signal processing and classification tech- vol. 23, no. 4, pp. 618–627, Jul. 2015, doi: 10.1109/tnsre.2015.2401134. niques,’’ Sensors, vol. 13, no. 9, pp. 12431–12466, Sep. 2013, doi: S. Muceli, N. Jiang, and D. Farina, ‘‘Extracting signals robust to 10.3390/s130912431. electrode number and shift for online simultaneous and proportional A. J. Young, L. J. Hargrove, and T. A. Kuiken, ‘‘The effects of electrode myoelectric control by factorization algorithms,’’ IEEE Trans. Neu- size and orientation on the sensitivity of myoelectric pattern recognition ral Syst. Rehabil. Eng., vol. 22, no. 3, pp. 623–633, May 2014, doi: systems to electrode shift,’’ IEEE Trans. Biomed. Eng., vol. 58, no. 9, 10.1109/tnsre.2013.2282898. pp. 2537–2544, Sep. 2011, doi: 10.1109/tbme.2011.2159216. K.-H. Park, H.-I. Suk, and S.-W. Lee, ‘‘Position–independent decoding of U. Imtiaz, L. Bartolomeo, Z. Lin, S. Sessa, H. Ishii, K. Saito, M. Zecca, movement intention for proportional myoelectric interfaces,’’ IEEE Trans. and A. Takanishi, ‘‘Design of a wireless miniature low cost EMG sensor Neural Syst. Rehabil. Eng., vol. 24, no. 9, pp. 928–939, Sep. 2016, doi: using gold plated dry electrodes for biomechanics research,’’ in Proc. IEEE 10.1109/tnsre.2015.2481461. Int. Conf. Mechatronics Autom. (ICMA), Aug. 2013, pp. 957–962, doi: E. N. Kamavuako, J. C. Rosenvang, R. Horup, W. Jensen, D. Farina, and 10.1109/icma.2013.6618044. K. B. Englehart, ‘‘Surface versus untargeted intramuscular EMG based E. Vavrinský, M. Daříček, F. Hornek, M. Horniak, and D. Donoval, classification of simultaneous and dynamically changing movements,’’ ‘‘Design of EMG wireless sensor system,’’ in Proc. Int. Conf. Appl. Elec- IEEE Trans. Neural Syst. Rehabil. Eng., vol. 21, no. 6, pp. 992–998, tron., Pilsen, Czech Republic, 2011, pp. 1–4. Nov. 2013, doi: 10.1109/tnsre.2013.2248750. C. Kitchin and L. Counts, ‘‘In-amp basics,’’ in A Designer’s Guide to N. Jarrasse, C. Nicol, A. Touillet, F. Richer, N. Martinet, J. Paysant, and Instrumentation Amplifiers, 3rd ed. Norwood, MA, USA: Analog Devices, J. B. De Graaf, ‘‘Classification of phantom finger, hand, wrist, and elbow 2004, pp. 1–4. voluntary gestures in transhumeral amputees with sEMG,’’ IEEE Trans. Neural Syst. Rehabil. Eng., vol. 25, no. 1, pp. 71–80, Jan. 2017, doi: J. Wang, L. Tang, and J. E Bronlund, ‘‘Surface EMG signal amplification 10.1109/tnsre.2016.2563222. and filtering,’’ Int. J. Comput. Appl., vol. 82, no. 1, pp. 15–22, Nov. 2013, doi: 10.5120/14079-2073. S. Shin, J. H. Lee, and S. H. Son, ‘‘A survey of approaches for recog- nizing hand gestures using EMG signal,’’ in Proc. IEEE 22nd Int. Conf. T. S. Poo and K. Sundaraj, ‘‘Design and development of a low cost EMG Embedded Real-Time Comput. Syst. Appl. (RTCSA), Aug. 2016, p. 263, signal acquisition system using surface EMG electrode,’’ in Proc. IEEE doi: 10.1109/RTCSA.2016.22. Asia–Pacific Conf. Circuits Syst., Dec. 2010, pp. 24–27, doi: 10.1109/apc- R. Meattini, S. Benatti, U. Scarcia, L. Benini, and C. Melchiorri, cas.2010.5774896. ‘‘Experimental evaluation of a sEMG-based human-robot interface for H. J. Hermens, ‘‘Development of recommendations for SEMG sensors human-like grasping tasks,’’ in Proc. IEEE Int. Conf. Robot. Biomimet- and sensor placement procedures,’’ J. Electromyograph. Kinesiol., vol. 10, ics (ROBIO), Dec. 2015, pp. 1030–1035, doi: 10.1109/robio.2015. no. 5, pp. 361–374, Oct. 2000, doi: 10.1016/S1050-6411(00)00027-4. 7418907. M. Hakonen, H. Piitulainen, and A. Visala, ‘‘Current state of digital S. Benatti, F. Casamassima, B. Milosevic, E. Farella, P. Schonle, signal processing in myoelectric interfaces and related applications,’’ S. Fateh, T. Burger, Q. Huang, and L. Benini, ‘‘A versatile embedded Biomed. Signal Process. Control, vol. 18, pp. 334–359, Apr. 2015, doi: platform for EMG acquisition and gesture recognition,’’ IEEE Trans. 10.1016/j.bspc.2015.02.009. Biomed. Circuits Syst., vol. 9, no. 5, pp. 620–630, Oct. 2015, doi: H. Chen, Y. Zhang, Z. Zhang, Y. Fang, H. Liu, and C. Yao, ‘‘Exploring the 10.1109/tbcas.2015.2476555. relation between EMG sampling frequency and hand motion recognition K. Xing, P. Yang, J. Huang, Y. Wang, and Q. Zhu, ‘‘A real- accuracy,’’ in Proc. IEEE Int. Conf. Syst., Man, Cybern. (SMC), Banff, AB, time EMG pattern recognition method for virtual myoelectric hand Canada, Oct. 2017, pp. 1139–1144, doi: 10.1109/smc.2017.8122765. control,’’ Neurocomputing, vol. 136, pp. 345–355, Jul. 2014, doi: A. Phinyomark, R. N. Khushaba, and E. Scheme, ‘‘Feature extraction 10.1016/j.neucom.2013.12.010. and selection for myoelectric control based on wearable EMG sensors,’’ Y. Xu, C. Yang, P. Liang, L. Zhao, and Z. Li, ‘‘Development of a hybrid Sensors, vol. 18, no. 5, p. 1615, May 2018, doi: 10.3390/s18051615. motion capture method using MYO armband with application to teleoper- N. A. I. M. Rosli, M. A. A. Rahman, S. A. Mazlan, and H. Zamzuri, ation,’’ in Proc. IEEE Int. Conf. Mechatronics Autom. (ICMA), Aug. 2016, ‘‘Electrocardiographic (ECG) and Electromyographic (EMG) signals pp. 1179–1184, doi: 10.1109/icma.2016.7558729. fusion for physiological device in rehab application,’’ in Proc. IEEE M. Sathiyanarayanan and S. Rajan, ‘‘MYO armband for physiotherapy Student Conf. Res. Develop. (SCOReD), Dec. 2014, pp. 1–5, doi: healthcare: A case study using gesture recognition application,’’ in Proc. 10.1109/SCORED.2014.7072965. 8th Int. Conf. Commun. Syst. Netw. (COMSNETS), Jan. 2016, pp. 1–6, doi: I. Kim, Y. A. Bhagat, J. Homer, and R. Lobo, ‘‘Multimodal analog 10.1109/comsnets.2016.7439933. front end for wearable bio–sensors,’’ IEEE Sensors J., vol. 16, no. 24, R. Lipovsky and H. A. Ferreira, ‘‘Hand therapist: A rehabilitation pp. 8784–8791, Dec. 2016, doi: 10.1109/jsen.2016.2564942. approach based on wearable technology and video gaming,’’ in Proc. IEEE Y. Li, X. Zhang, Y. Gong, Y. Cheng, X. Gao, and X. Chen, ‘‘Motor 4th Portuguese Meeting Bioeng. (ENBENG), Feb. 2015, pp. 1–2, doi: function evaluation of hemiplegic upper-extremities using data fusion from 10.1109/enbeng.2015.7088817. wearable inertial and surface EMG sensors,’’ Sensors, vol. 17, no. 3, p. 582, M. Linderman, M. A. Lebedev, and J. S. Erlichman, ‘‘Recognition of 2017, doi: 10.3390/s17030582. handwriting from electromyography,’’ PLoS ONE, vol. 4, no. 8, pp. 2–9, M. Balouchestani and S. Krishnan, ‘‘Effective low–power wearable wire- 2009, doi: 10.1371/journal.pone.0006791. less surface EMG sensor design based on analog–compressed sens- C. Carmona-Duarte, R. de Torres-Peralta, M. Diaz, M. A. Ferrer, and ing,’’ Sensors, vol. 14, no. 12, pp. 24305–24328, Dec. 2014, doi: M. Martin-Rincon, ‘‘Myoelectronic signal-based methodology for the 10.3390/s141224305. analysis of handwritten signatures,’’ Hum. Movement Sci., vol. 55, U. J. Naeem, C. Xiong, and A. A. Abdullah, ‘‘EMG-muscle force estima- pp. 18–30, Oct. 2017, doi: 10.1016/j.humov.2017.07.002. tion model based on back-propagation neural network,’’ in Proc. IEEE Int. M. Djioua and R. Plamondon, ‘‘A new methodology to improve myoelec- Conf. Virtual Environ. Hum.-Comput. Interfaces Meas. Syst. (VECIMS), tric signal processing using handwriting,’’ in Proc. Int. Conf. Frontiers Tianjin, China, Jul. 2012. Handwriting Recognit., Jan. 2008, pp. 112–118. VOLUME 7, 2019 7803 B. Rodríguez-Tapia et al.: Myoelectric Interfaces and Related Applications: Current State of EMG Signal Processing G. Huang, D. Zhang, X. Zheng, and X. Zhu, ‘‘An EMG-based hand- S. Pancholi and R. Agarwal, ‘‘Development of low cost EMG data acqui- writing recognition through dynamic time warping,’’ in Proc. Annu. Int. sition system for arm activities recognition,’’ in Proc. Int. Conf. Adv. Conf. IEEE Eng. Med. Biol. Soc., Aug. 2010, pp. 4902–4905, 2010, doi: Comput., Commun. Informat. (ICACCI), Sep. 2016, pp. 2465–2469, doi: 10.1109/IEMBS.2010.5627246. 10.1109/icacci.2016.7732427. S. Tan, Y. An, Y. Wu, and D. Zhang, ‘‘Electromyography based hand- J. G. Abreu, J. M. Teixeira, L. S. Figueiredo, and V. Teichrieb, ‘‘Eval- writing recognition system using LM–BP neural network,’’ in Proc. uating sign language recognition using the MYO armband,’’ in Proc. 9th Int. Conf. Hum. Syst. Interact. (HSI), Jul. 2016, pp. 83–88, doi: 18th Symp. Virtual Augmented Reality (SVR), 2016, pp. 64–70, doi: 10.1109/hsi.2016.7529613. 10.1109/SVR.2016.21. Y. Chen, Z. Yang, H. Gong, and S. Wang, ‘‘Recognition of sketching S. Rawat, S. Vats, and P. Kumar, ‘‘Evaluating and exploring the MYO from surface electromyography,’’ Neural Comput. Appl., vol. 30, no. 9, ARMBAND,’’ in Proc. Int. Conf. Syst. Modeling Adv. Res. Trends pp. 2725–2737, Nov. 2018, doi: 10.1007/s00521-017-2857-3. (SMART), 2016, pp. 115–120, doi: 10.1109/sysmart.2016.7894501. Y. Chen and Z. Yang, ‘‘A novel hybrid model for drawing trace recon- Y. Fang, N. Hettiarachchi, D. Zhou, and H. Liu, ‘‘Multi–modal struction from multichannel surface electromyographic activity,’’ Frontiers sensing techniques for interfacing hand prostheses: A review,’’ Neurosci., vol. 11, pp. 1–17, Feb. 2017, doi: 10.3389/fnins.2017.00061. IEEE Sensors J., vol. 15, no. 11, pp. 6065–6076, Nov. 2015, doi: Y. Cai, Y. Guo, H. Jiang, and M.-C. Huang, ‘‘Machine-learning approaches 10.1109/jsen.2015.2450211. for recognizing muscle activities involved in facial expressions captured J. L. Correa-Figueroa, E. Morales-Sánchez, J. A. Huerta-Ruelas, by multi-channels surface electromyogram,’’ Smart Health, vols. 5–6, J. J. González-Barbosa, and C. R. Cárdenas-Pérez, ‘‘Sistema de adquisi- pp. 15–25, Jan. 2018, doi: 10.1016/j.smhl.2017.11.002. ción de señales SEMG para la detección de fatiga muscular,’’ Revista G. S. Meltzner, J. T. Heaton, Y. Deng, G. De Luca, S. H. Roy, and Mexicana de Ingenieria Biomedica, vol. 37, no. 1, pp. 17–27, 2016, doi: J. C. Kline, ‘‘Silent speech recognition as an alternative communication 10.17488/RMIB.37.1.4. device for persons with laryngectomy,’’ IEEE/ACM Trans. Audio, Speech, L. Mesin, R. Merletti, and A. Rainoldi, ‘‘Surface EMG: The issue of elec- Language Process., vol. 25, no. 12, pp. 2386–2398, Dec. 2017, doi: trode location,’’ J. Electromyograp. Kinesiol., vol. 19, no. 5, pp. 719–726, 10.1109/taslp.2017.2740000. Oct. 2009, doi: 10.1016/j.jelekin.2008.07.006. A. D. C. Chan, K. Englehart, B. Hudgins, and D. F. Lovely, ‘‘MYO-electric P. Artemiadis, ‘‘EMG-based robot control interfaces: Past, present and signals to augment speech recognition,’’ Med. Biol. Eng. Comput., vol. 39, future,’’ Adv. Robot. Autom., vol. 1, no. 2, pp. 10–12, 2012, doi: no. 4, pp. 500–504, Jul. 2001, doi: 10.1007/bf02345373. 10.4172/2168-9695.1000e107. M. B. I. Raez, M. S. Hussain, F. Mohd-Yasin, M. Reaz, M. S. Hussain, J. P. Mogk and P. J. Keir, ‘‘Crosstalk in surface electromyography of the and F. Mohd-Yasin, ‘‘Techniques of EMG signal analysis: Detection, pro- proximal forearm during gripping tasks,’’ J. Electromyograp. Kinesiol., cessing, classification and applications.,’’ Biol. Procedures Online, vol. 8, vol. 13, no. 1, pp. 63–71, Feb. 2003, doi: 10.1016/s1050-6411(02)00071-8. no. 1, pp. 11–35, 2006, doi: 10.1251/bpo115. C. J. De Luca, L. D. Gilmore, M. Kuznetsov, and S. H. Roy, ‘‘Filtering L. Liu, P. Liu, E. A. Clancy, E. Scheme, and Englehart, ‘‘Electromyogram the surface EMG signal: Movement artifact and baseline noise contam- whitening for improved classification accuracy in upper limb prosthe- ination,’’ J. Biomech., vol. 43, no. 8, pp. 1573–1579, May 2010, doi: sis control,’’ IEEE Trans. Neural Syst. Rehabil. Eng., vol. 21, no. 5, 10.1016/j.jbiomech.2010.01.027. pp. 767–774, Sep. 2013, doi: 10.1109/tnsre.2013.2243470. G. Li, Y. Li, L. Yu, and Y. Geng, ‘‘Conditioning and sampling issues T. Supuk, A. Skelin, and M. Cic, ‘‘Design, development and testing of of EMG signals in motion recognition of multifunctional myoelectric a low–cost sEMG system and its use in recording muscle activity in prostheses,’’ Ann. Biomed. Eng., vol. 39, no. 6, pp. 1779–1787, Jun. 2011, human gait,’’ Sensors, vol. 14, no. 5, pp. 8235–8258, May 2014, doi: doi: 10.1007/s10439-011-0265-x. 10.3390/s140508235. E. A. Clancy, E. L. Morin, and R. Merletti, ‘‘Sampling, noise-reduction and H. Fuketa, K. Yoshioka, Y. Shinozuka, and K. Ishida, ‘‘Measure- amplitude estimation issues in surface electromyography,’’ J. Electromyo- ment sheet with 2 V organic transistors for prosthetic hand control,’’ grap. Kinesiol., vol. 12, pp. 1–16, Feb. 2002. IEEE Trans. Biomed. Eng., vol. 8, no. 6, pp. 824–833, 2014, doi: N. Van Helleputte, M. Konijnenburg, J. Pettine, D.-W. Jee, H. Kim, 10.1109/TBCAS.2014.2314135. A. Morgado, R. Van Wegberg, T. Torfs, R. Mohan, A. Breeschoten, N. Prince, S. Nadar, S. Thakare, V. Thale, and J. Desai, ‘‘Design of H. De Groot, C. Van Hoof, and R. F. Yazicioglu, ‘‘A 345 µW multi–sensor front end circuitry for detection of surface EMG using bipolar record- biomedical SoC with bio–impedance, 3-channel ECG, motion artifact ing technique,’’ in Proc. Int. Conf. Control, Instrum., Commun. Comput. reduction, and integrated DSP,’’ IEEE J. Solid-State Circuits, vol. 50, no. 1, Technol. (ICCICCT), Dec. 2016, pp. 594–599, doi: 10.1109/iccicct.2016. pp. 230–244, Jan. 2015, doi: 10.1109/jssc.2014.2359962. 7988019. P. Mccool, G. D. Fraser, A. D. C. Chan, L. Petropoulakis, and J. J. Soraghan, ‘‘Identification of contaminant type in surface electromyo- graphy (EMG) signals,’’ IEEE Trans. Neural Syst. Rehabil. Eng., vol. 22, no. 4, pp. 774–783, Jul. 2014, doi: 10.1109/tnsre.2014.2299573. BERNABE RODRÍGUEZ-TAPIA received the A. Chan and D. MacIsaac, ‘‘CleanEMG: Assessing the quality of EMG B.S. degree in electronics engineering and the signals,’’ in Proc. 34th Conf. Can. Med., Nov. 2011, pp. 17–20. master’s degree in engineering from the Universi- N. Nazmi, M. Abdul Rahman, S.-I. Yamamoto, S. Ahmad, H. Zamzuri, and dad Autónoma de Baja California (UABC). He is S. Mazlan, ‘‘A review of classification techniques of EMG signals during currently pursuing the Ph.D. degree in technology isotonic and isometric contractions,’’ Sensors, vol. 16, no. 8, p. 1304, with the Universidad Autónoma de Ciudad Juarez. Aug. 2016, doi: 10.3390/s16081304. He is also a Professor with the School of Engineer- J. J. A. Mendes, Jr., E. Agostini Jr., S. Okida, and S. L. Stevan, Jr., ‘‘Circuit ing Sciences and Technology, UABC. His research design of amplification and filtration for surface electromyography data interests include control, signal processing, and acquisition,’’ IEEE Latin Amer. Trans., vol. 13, no. 10, pp. 3193–3200, applied artificial intelligence. 2015, doi: 10.1109/TLA.2015.7387221. R. Barioul, S. F. Ghribi, and O. Kanoun, ‘‘A low cost signal acquisition board design for myopathy’s EMG database construction,’’ in Proc. 13th Int. Multi-Conf. Syst., Signals Devices (SSD), Mar. 2016, pp. 274–279, doi: 10.1109/ssd.2016.7473767. ISRAEL SOTO received the M.S. and Ph.D. M. Zahak, ‘‘Signal acquisition using surface EMG and circuit design degrees in electrical engineering from the Insti- considerations for robotic prosthesis,’’ in Computational Intelligence tuto Tecnológico de la Laguna, Torreón, Mexico, in Electromyography Analysis—A Perspective on Current Applications in 2009 and 2014, respectively. He is currently and Future Challenges. London, U.K.: IntechOpen, Oct. 2012, doi: a Research Professor with the Institute of Engi- 10.5772/52556. neering and Technology, Universidad Autónoma K. Kiguchi, M. H. Rahman, M. Sasaki, and K. Teramoto, ‘‘Develop- de Ciudad Juárez. His research interests include ment of a 3DOF mobile exoskeleton robot for human upper-limb motion modeling, design, and control of mechatronics assist,’’ Robot. Auto. Syst., vol. 56, no. 8, pp. 678–691, Aug. 2008, doi: systems. 10.1016/j.robot.2007.11.007. 7804 VOLUME 7, 2019 B. Rodríguez-Tapia et al.: Myoelectric Interfaces and Related Applications: Current State of EMG Signal Processing DANIELA M. MARTÍNEZ received the B.S. NORMA CANDOLFI ARBALLO received the degree in electrical engineering and the Ph.D. B.S. degree in computer engineering and the mas- degree in telecommunications engineering from ter’s degree in engineering from the Universidad the Universidad Autónoma de Baja California Autónoma de Baja California (UABC). She is cur- (UABC), in 2009 and 2014, respectively. She is rently pursuing the Ph.D. degree with the Univer- currently a Professor with the School of Engineer- sity of Guadalajara. She is also a Professor with ing Sciences and Technology, UABC. Her research the School of Engineering Sciences and Technol- interests are within the field of statistical signal ogy, UABC and a Leader of the Academic Corps processing and cognitive radio systems. of Research of Continuous Distance Education. She is also a member of the ICTC Mediated Edu- cation Quality Network, the Education Network Supported in Information, Communication and Collaboration Technologies, and the Mexican Network of Researchers in Emerging Technologies in Education. VOLUME 7, 2019 7805