Econometrics Topic 1 Notes PDF
Document Details
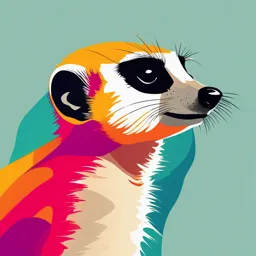
Uploaded by ProminentPortland5860
2024
Judith Y. Guo
Tags
Summary
These are lecture notes for ECON20110/30370, covering econometrics. The notes delve into the mathematical aspects of causality and regression, complementing the lecture slides. The document explores descriptive and causal questions, and highlights the use of regression in both contexts.
Full Transcript
Lecture Notes for ECON20110/30370 Econometrics Judith Y. Guo October 10, 2024 Preface These notes are designed to accomp...
Lecture Notes for ECON20110/30370 Econometrics Judith Y. Guo October 10, 2024 Preface These notes are designed to accompany the corresponding lectures and serve as a deeper dive into the mathematical aspects of the subject matter. They should be viewed as a complement to the lecture slides, not as a replacement. The lecture slides primarily emphasise motivation, intuition, and application, while these notes provide a more careful and technical treatment of the theory. Although the chapter numbering aligns with that of the slides, the arrangement of sections may differ. If you spot any typos or errors, please send me an email. TABLE OF CONTENTS I Causality and Regression 1 1 Descriptive and Causal Questions 2 1.1 Descriptive Questions...................................... 2 1.2 Causal Questions........................................ 3 1.3 Key Takeaway: Correlation vs Causation............................ 7 2 Simple Linear Regression 9 2.1 Introduction........................................... 9 2.2 The Sample Regression Problem and OLS........................... 9 2.3 From the Sample to the Population Regression Problem.................... 12 2.3.1 Solving the Population Regression Problem...................... 13 2.3.2 The Population Regression Model........................... 15 2.4 Linear Regression and the Conditional Expectation...................... 17 2.5 Derivation of the OLS Solutions................................ 19 2.5.1 Some Properties of OLS Solutions........................... 21 2.6 Recap: Sample and Population Regression Problem...................... 22 2.7 Descriptive Interpretation of Regression Coefficients..................... 22 2.8 Comparing Correlation and Slope Coefficient......................... 24 2.9 Causal Model and Linear Regression Model.......................... 24 2.10 Should we assume OR or CMI?................................. 27 Appendices 29 Appendix 2.A Numerical Equivalence of the Causal and Population Regression Models under OR 29 3 Multiple Linear Regressions 31 3.1 The Population Regression Model............................... 31 i Judith Guo ECON20110/30370 3.2 The Sample Regression Model................................. 33 3.3 The FWL Theorem....................................... 34 3.3.1 Introduction...................................... 34 3.3.2 The Need for Orthogonalisation in Multiple Linear Regression............ 35 3.3.3 The Procedure..................................... 35 3.3.4 Do We Always Need to Use Orthogonalisation?................... 38 3.4 Multicollinearity........................................ 39 3.4.1 Perfect Multicollinearity =⇒ Parameters Unidentified................ 39 3.4.2 Imperfect Multicollinearity =⇒ Precision of Estimates Affected........... 40 3.5 Descriptive Interpretation of Regression Coefficients..................... 42 3.5.1 Example: House Pricing................................ 43 3.6 Comparing Correlation and Slope Coefficient......................... 44 3.7 Causal Model and Multiple Linear Regression Model..................... 45 3.8 Proxy (or Control) Variables.................................. 46 3.9 Interpreting Coefficients: Causality and Proxy Variables.................... 48 3.10 Conclusion: Recipes for Regression on Observational Data.................. 49 3.10.1 Using Regression to Estimate Causal Effects..................... 50 3.10.2 Using Regression to Describe Correlations or Make Predictions........... 50 3.10.3 Evaluating Regression Estimates........................... 51 Appendices 52 Appendix 3.A Alternative Representation of the FWL Theorem.................. 52 Appendix 3.B FWL Requires Solving Two FOCs Only...................... 53 Appendix 3.C The Equivalence of the Direct Approach and FWL................. 55 ii Part I Causality and Regression 1 1 Descriptive and Causal Questions In econometrics, we often address two main types of questions: descriptive and causal. When we analyse economic data, we might simply want to describe patterns we observe (descriptive questions), or we might want to dig deeper to understand what is driving those patterns — the underlying cause-and-effect dynamics (causal questions). Both types of questions are crucial for understanding economic relationships, but they serve different purposes and call for different methods. 1.1 Descriptive Questions Descriptive questions focus on identifying statistical patterns or relationships between variables with- out trying to establish a cause-and-effect link. These questions essentially ask, “What is happening?” or “What does the data show?” They provide a snapshot of how variables relate to each other, but do not attempt to explain why those relationships exist. 1. Examples of descriptive questions: Diet and Weight: Do vegetarians, on average, weigh less than people who eat meat? – The goal is not to understand why vegetarians might weigh less or whether being vegetar- ian causes someone to weigh less, but simply to establish whether there is an association based on the data. Smoking and Birth Weight: Are babies born to mothers who smoke generally lighter at birth than those born to non-smoking mothers? – Again, the focus is on identifying a pattern in the data, not explaining the reasons behind it. In other words, we are not trying to determine whether maternal smoking reduces birth- weight. Education and Income: Do people with higher levels of education tend to earn more than those with lower levels of education? 2 Judith Guo ECON20110/30370 – This is about observing a trend, not implying that higher education automatically leads to higher earnings. These types of questions help us describe the world as it is, providing insights that can later inform more detailed, causal investigations. They are particularly useful for summarising trends in large datasets and forming the basis for more complex economic analyses. 2. Why is Regression Analysis Helpful for Descriptive Questions? To answer descriptive questions, we can use comparative analysis. For example, by comparing the average birth weights of babies born to smokers and non-smokers, we can identify any patterns that emerge from the data. A powerful tool for making these comparisons is regression analysis. It summarises how one variable is associated with another, while accounting for many other factors that might also be related (in a statistical sense) to the variables of interest. For instance, when comparing birth weights between babies of smokers and non-smokers, it’s helpful to control for factors such as the mother’s age or socioeconomic status that might also be linked to birth weight. Regression analysis provides a systematic and quantitative way to explore statistical relationships between variables. It goes beyond simple averages by helping us understand the strength and direction of the relationships, and how much variation in one variable is associated with differences in another. For example, regression can tell us not only that babies of smokers tend to weigh less on average, but also by how much, while controlling for some other factors that might also be correlated with birth weight. Additionally, regression (along with hypothesis testing) helps us determine whether the patterns we observe in the data are statistically significant or whether they could simply be due to chance. This makes regression a key tool for reliably summarising patterns and trends, helping to answer descrip- tive questions in a robust manner. 1.2 Causal Questions Causal questions go beyond simply identifying patterns; they aim to understand the cause-and-effect relationship between variables. While descriptive questions answer “What is happening?”, causal questions ask, “Why is this happening?” or “What would happen if...?” In essence, causal questions seek to determine how changes in one variable lead to changes in another. 3 Judith Guo ECON20110/30370 1. Examples of causal questions: Diet and Weight: Would switching to a vegetarian diet lead to weight loss? – Unlike the descriptive question, “Do vegetarians weigh less than meat-eaters?”, which simply observes a pattern, the causal question asks about the effect of making a specific change — switching to a vegetarian diet. The aim is to figure out if changing one’s diet can actively influence weight, assuming all else remains the same. Smoking and Birth Weight: Would a mother quitting smoking result in a heavier newborn? – This causal question investigates if a specific action — quitting smoking — would directly lead to a change in the baby’s birth weight, focusing on isolating the effect of smoking cessation. Education and Income: Would attaining a higher level of education lead to higher earnings? – The descriptive question, “Do people with higher education tend to earn more?”, shows a correlation, but doesn’t reveal whether education is the driving force behind higher earn- ings. The causal question, however, seeks to understand whether increasing education causes higher earnings. The challenge here is to separate the effect of education from other factors, such as innate ability or family background, which could also influence income. In this context, the question focuses on determining whether raising education levels alone would result in higher wages, assuming other factors remain constant. 2. Establishing Causality At the core of causal analysis is the idea that changing one variable, X, will bring about a change in another variable, Y. To establish a causal relationship, two key conditions must be met: (a) Correlation: X must be correlated with Y. A statistical association between the two variables is necessary, but NOT sufficient, for causality. Just because two variables move together doesn’t mean that one causes the other — correlation does not imply causation. For instance, ice cream sales may be correlated with higher crime rates during the summer, but it’s not ice cream consumption causing crime. Instead, both are driven by a third factor — warmer weather. This illustrates why correlation alone cannot confirm a causal link. (b) Temporal Ordering: X must occur before Y. 4 Judith Guo ECON20110/30370 For X to cause Y , the change in X must happen before the change in Y. This may seem straightforward, but it can be tricky to prove in practice. For example, if we observe that people with higher levels of education tend to have higher incomes, we need to be sure that the education came first and that it wasn’t the case that people with higher incomes later invested in more education. Establishing this order of events is crucial in causal analysis. While correlation and temporal ordering are both necessary for establishing causality, they are NOT enough on their own. Even if X and Y are correlated, and X happens before Y , there may still be other variables influencing the relationship. This brings us to the importance of the ceteris paribus condition. 3. The Ceteris Paribus Principle To isolate the causal effect of X on Y , we need to ensure that ALL other factors that could influence Y remain constant. This is known as the ceteris paribus principle, which means ”all else equal” in Latin. The goal is to isolate the effect of X on Y by controlling for all other variables that could affect Y. Only then can we confidently claim that the observed change in Y is due to the change in X and not something else. Although this principle sounds simple, it is difficult to achieve in practice. In the real world, we can rarely hold all other factors constant. For example, if we want to assess whether a mother quitting smoking would lead to a heavier baby at birth, we would also need to account for other factors that could influence birth weight — such as the mother’s diet, access to healthcare, mental health, or alcohol consumption. These factors could change alongside smoking cessation, making it hard to isolate the effect of quitting smoking alone. 4. Answering Causal Questions is Difficult One of the main challenges in economics and the social sciences is that we typically work with observational data rather than experimental data. Ideally, we would rely on experimental data, where variables can be manipulated under con- trolled conditions to directly observe their effects. In an experiment, we can randomly assign subjects to different groups and change one vari- able, X, to see how it affects another variable, Y. This method — where we can directly test “what happens when X changes” — is considered the gold standard for establishing causality 5 Judith Guo ECON20110/30370 because it allows us to control for confounding factors (see below for explanation). However, in economics, running such experiments is often impractical, expensive, or even unethical. For example, it wouldn’t be ethical to randomly assign some pregnant women to smoke and others not to, just to study the effects of smoking on birth weight. – Confounding factors (or confounders) are variables that influence the outcome variable Y and correlate with the explanatory variable X. They make it difficult to determine whether the observed relationship between X and Y is truly causal if not being controlled for. For instance, consider a study on the relationship between coffee consumption (X) and heart disease (Y ). If smokers tend to drink more coffee, and smoking also increases the risk of heart disease, then smoking is a confounding factor. It correlates with coffee consump- tion and affects heart disease risk, making it harder to tell whether coffee itself increases the risk of heart disease, or if the real cause is smoking. Observational data, which is much more common in economics, presents a different challenge. In this case, we observe and record data as it occurs naturally, rather than controlling or altering them as in an experiment. While this type of data can help us identify correlations or patterns to answer descriptive questions, it’s much more difficult to establish causality because we cannot control for all the confounding factors that might influence the relationship between X and Y. For instance, we might observe that people with higher levels of education tend to earn more, but it’s difficult to determine if education directly causes higher earnings. There could be other factors — such as innate ability, motivation, or family background — that correlate with educa- tion and affect income. These unobservable factors, like ‘innate ability’, complicate the analysis because they could be the true drivers of both higher education and higher earnings. Without the ability to manipulate variables and control for these confounders, it becomes chal- lenging to isolate the true causal effect of education on earnings. Researchers must find ways to control for or account for these unobservable factors, which is why answering causal questions with observational data is so complex. 5. The Role of Regression in Causal Analysis Regression techniques are valuable tools in econometrics, particularly when exploring potential causal relationships between variables. However, while regression can reveal associations, it cannot, on its own, establish causality. To draw meaningful conclusions about cause and effect, regression 6 Judith Guo ECON20110/30370 must be combined with careful reasoning and additional causal assumptions. At its core, regression is a statistical method that quantifies the relationship between variables. For example, it can show how changes in X (such as education level) are associated with changes in Y (such as income). However, an observed association doesn’t automatically imply causation. Causal inference — the process of using data to establish causal relationships — requires not only statis- tical techniques but also theoretical reasoning to distinguish between simple correlations and true causal effects. This is where causal assumptions come into play. Key considerations for regression in causal analysis: In some cases, based on the context of the study, we can reasonably assume that the necessary conditions for causality — such as the absence of unobserved/unmeasured confounding factors — are met. For example, if the study controls for the key variables that might influence the outcome, we can be more confident in the results. In other situations, additional studies or experiments may be needed to ensure these assumptions hold. Techniques like randomised controlled trials (where participants are randomly assigned to different groups) or natural experiments (where external factors create conditions similar to an experiment) can help better isolate the effect of one variable on another, strengthening the case for causality. In summary, regression is a powerful tool for identifying associations, but it has limitations. For it to provide meaningful insights into causality, certain assumptions must hold, and researchers must ensure these assumptions are valid in the context of their study. 1.3 Key Takeaway: Correlation vs Causation One key principle to understand is that correlation (or even a detected pattern in regression) does not automatically imply causation. Just because X and Y are correlated doesn’t mean that one causes the other. However, if two variables are causally related, they must be correlated (i.e., statistically related). Consider the classic example of height and basketball. Taller people may tend to play basketball, and there may be a positive correlation between height and playing the sport, but that doesn’t mean playing basketball makes you taller. In reality, both variables are influenced by other factors, such as genetics. While correlation is something we can define and measure mathematically, causation is more complex and goes beyond numbers. Establishing a causal relationship requires a solid theoretical understanding 7 Judith Guo ECON20110/30370 of the variables involved. Before asserting that one variable causes another, we must have a clear, theory- based rationale for doing so. If we suspect a causal relationship between two variables, we need to justify the direction of that relationship before deciding which is X (the cause) and which is Y (the effect). Although correlation alone cannot prove causality, it’s still useful. Causal inference is about determin- ing when a correlation might suggest a causal effect. A better way to express this would be: “Causation cannot be inferred from correlation alone”, or more broadly, “Causation cannot be inferred from statistics alone”. Effective causal analysis requires more than just statistical evidence — it also needs a well-thought- out identification strategy, meaning the assumptions made must be plausible enough to support the idea that the observed statistical relationship is likely causal. So, what’s our goal for this academic year? We’ll be exploring the conditions and assumptions under which correlation might indeed suggest causation. 8 2 Simple Linear Regression 2.1 Introduction Regression models can always be used to conduct descriptive analysis, but when it comes to drawing causal inferences, we need to make some fairly strong assumptions. For instance, we must assume that a causal relationship exists and that we can consistently estimate its effect. Most academic research focuses on using regression for causal inference, which is why econometrics textbooks often concentrate on the challenges of consistently estimating these causal effects. However, outside of academia, particularly with the rise of Big Data, regression is frequently used more for prediction rather than for uncovering causal relationships. In this chapter, we’ll first set aside the idea of causality and start by looking at regression as a tool for prediction. In simple terms, regression helps us explore the association between variables, without making any claims about cause and effect. From a descriptive standpoint, it reveals the conditional distribution of the dependent variable, Y , given the explanatory variables, X. This means that when we interpret the results of a regression model, we focus on the conditional expectation of Y given X. Once we’ve developed a solid understanding of how regression works in this predictive context, we’ll revisit the concept of causality and explore how the two are related. 2.2 The Sample Regression Problem and OLS Suppose we are interested in exploring the relationship between two variables, say Y (for example, wage) and X (years of edu- Y cation). We have collected a sample of data points {(Yi , Xi )}ni=1. A natural starting point is to assume a linear relationship be- tween these variables. This means fitting a straight line, b0 + b1 X, through the scatter of data points. There are many possible lines X 0 with different slopes and intercepts, so the key question is: which Fig. 2.1 Minimise Vertical Distances line best represents the relationship? 9 Judith Guo ECON20110/30370 There are several ways we could approach this problem. We could minimise the vertical distance, horizontal distance, or even the perpendicular distance between the points and the line. The method we focus on is Ordinary Least Squares (OLS), which finds the best-fitting line by minimising the vertical distances from the data points to the line. The OLS method works by solving the following minimisation problem: n min S(b0 , b1 ) := min b0 ,b1 ∑ (Yi − b0 − b1 Xi )2. b0 ,b1 i=1 (2.1) A few important points to note: Minimising the sum of squared errors ∑ni=1 (Yi − b0 − b1 Xi )2 with respect to b0 and b1 is mathemat- ically equivalent to minimising n1 ∑ni=1 (Yi − b0 − b1 Xi )2. The factor 1 n does not affect the location of the minimum, so we omit it for simplicity. The errors (or distances) can be positive or negative. If we simply added these up, positive and negative values could cancel each other out, giving a misleading result. To avoid this, we square the errors, ensuring they all contribute positively. OLS solves the sample regression problem by finding the values of b0 and b1 that minimise the sum of squared prediction errors in the sample. The OLS criterion penalises larger errors more heavily than smaller ones, making the method sensi- tive to outliers (i.e., data points that don’t follow the general pattern of the rest of the data). While OLS is widely used, it’s worth noting that it may not always be the best option. For instance, using the absolute values of errors instead of their squares can sometimes produce a more robust regression line, less sensitive to outliers. However, OLS has some clear advantages: Simplicity: OLS provides a straightforward way to find the best-fitting line, making it easier to use in calculations, both by hand and in software, which is why it’s the default method in many cases. Uniqueness: Under mild conditions, OLS guarantees a unique solution, avoiding ambiguity in the choice of the best-fitting line. To solve for the best-fitting line, we need to minimise the function S(b0 , b1 ). This is done by taking the partial derivatives of S(b0 , b1 ) with respect to (hereafter w.r.t.) b0 and b1 , and setting these derivatives 10 Judith Guo ECON20110/30370 equal to zero. These are known as the first-order conditions (FOCs). The detailed derivation is provided in Section 2.5, but for now, we’ll focus on the solutions, denoted as βb0 and βb1 , which are given by: cov(X,Y ) βb0 = Y − βb1 X c and βb1 =. (2.2) var(X) c βb0 + βb1 X: the sample regression function Y βb1 : slope fitted value of Y → Ybj ubj :residual observed value of Y → Y j (X j ,Y j ): observed data point intercept → βb0 X 0 Xj Fig. 2.2 The Sample Fitted Line The best fitted line that OLS produces is: Yb = βb0 + βb1 X, where Yb : is the predicted/fitted value of Y , e.g. Ybj is the value predicted by the regression line when X = X j. The intercept βb0 represents the predicted value of Y when X is zero. The slope βb1 represents the average rate of change in Y for a one-unit change in X. The sample regression model can be expressed as: Yi = βb0 + βb1 Xi +b u = Ybi + ubi. (2.3) | {z } i =:Ybi where: We obtained the sample regression model by adding a sample prediction error, the residual ub, to the fitted line. 11 Judith Guo ECON20110/30370 Generally, Ybi ̸= Yi — there is always some prediction error. It’s rare for the regression line to fit all the data points perfectly (i.e., it’s nearly impossible for all residuals to be zero). The residuals are the differences between the actual observed values Y and and the fitted values Yb : ubi = Yi − Ybi = Yi − βb0 − βb1 Xi. It’s important to remember that the residuals are not part of the fitted values, and, as we’ll explore in Section 2.5, ubi is orthogonal to Ybi. 2.3 From the Sample to the Population Regression Problem While it’s useful to understand the relationship between variables within a sample, our ultimate goal is often to make inferences about Y the broader population. This raises an important question: What exactly are the OLS estimators, βb0 and βb1 , estimating? Let’s now imagine we have data on X and Y for the entire pop- ulation, denoted as {(Yi , Xi )}∞ i=1. In this case, we are no longer X 0 working with a finite sample but rather an infinite number of data Fig. 2.3 Population Regression Problem points. To find the best-fitting line for this population, we use the same basic logic as we did with the sample. We still focus on minimising squared (prediction) errors. However, instead of using sample moments (which are based on the data we observe), we now frame the problem in terms of population moments. This leads us to the population regression problem: min C(b0 , b1 ) := min E[(Yi − b0 − b1 Xi )2 ]. (2.4) b0 ,b1 b0 ,b1 Remember that expectation is essentially a weighted average, where the weights correspond to proba- bilities. This is the population equivalent of a sample average. [If you need a refresher on this, it may be helpful to revisit your notes from your statistics course.] To keep the notation simple when referring to population moments, we’ll drop the subscript i. For example, instead of writing E(Yi ), we will simply write E(Y ). 12 Judith Guo ECON20110/30370 2.3.1 Solving the Population Regression Problem To solve the population regression problem, we need to minimise the criterion function C(b0 , b1 ) = E[(Y − b0 − b1 X)2 ]. This involves taking the partial derivatives of C(b0 , b1 ) w.r.t. b0 and b1 , which we can do using the chain rule. Since the expectation operator E(·) is linear, we can swap the order of differentiation and taking the expectation. This allows us to differentiate the squared error term (Y − b0 − b1 X)2 first,and then apply the expectation. The chain rule helps us differentiate composite functions. In this case, we are differentiating the squared error term (Y − b0 − b1 X)2 w.r.t. b0 and b1. We first differentiate the outer function (the square) and then multiply by the derivative of the inner function (the linear term A = Y − b0 − b1 X). For example, with A = Y − b0 − b1 X: For b0 : By the chain rule, =A2 z }| { ∂ [(Yi − b0 − b1 Xi )2 ] ∂ A2 ∂ A2 ∂ A = = · ∂ b0 ∂ b0 ∂ A ∂ b0 ∂ A2 Differentiating the outer function gives 2A, i.e., ∂A = 2A, and differentiating the inner function gives ∂A ∂ b0 = −1. So: ∂ [(Yi − b0 − b1 Xi )2 ] = 2A · (−1) = 2 (Y − b0 − b1 X) ·(−1) ∂ b0 | {z } =A ∂A Similarly, for b1 , we get ∂ b1 = −X, so: ∂ [(Yi − b0 − b1 Xi )2 ] ∂ A2 ∂ A2 ∂ A = = · = 2A · (−X) = 2 (Y − b0 − b1 X) ·(−X) ∂ b1 ∂ b1 ∂ A ∂ b1 | {z } =A Rearranging and after reintroducing the expectation operator, the partial derivatives become: ∂C = −2E(Y − b0 − b1 X) = 0 ∂ b0 ∂C = −2E[(Y − b0 − b1 X) · X] = 0 ∂ b1 which by setting them to zero gives the FOCs for minimising the population regression problem. Let (β0 , β1 ) denote the unique solution to the minimisation problem. Substituting this into the FOCs, 13 Judith Guo ECON20110/30370 we have for β0 : 0 = E(Y − β0 − β1 X) =⇒ β0 = E(Y ) − β1 E(X) [recall that the expected value of a constant is the constant, e.g., E(β0 ) = β0 ]; and for β1 : 0 = E[(Y − β0 − β1 X) · X] =(1) E Y − E(Y ) − β1 E(X) −β1 X · X | {z } =β0 n o =E Y − E(Y ) − β1 X − E(X) · X n o = E[ Y − E(Y ) · X] − E β1 X − E(X) · X =(2) E[ Y − E(Y ) · X] − β1 E[ X − E(X) · X], where =(1) follows from plugging in the expression for β0 above, and =(2) comes from the fact that β1 is a constant (as it’s a population parameter) and can be factored out of the expectation operator. Rearranging, we obtain: E[ Y − E(Y ) X] β1 = . E[ X − E(X) X] Now, for the numerator, we can recognise that: cov(X,Y ) = E[ Y − E(Y ) X − E(X) ] = E[ Y − E(Y ) X] − E[ Y − E(Y ) · E(X)] =(3) E[ Y − E(Y ) X] − E(X) · [E Y − E(Y ) ] | {z } =E(Y )−E(Y )=0 = E[ Y − E(Y ) X], where =(3) follows from the fact that E(X) is a constant and can be factored out of the expectation. For the denominator, we can derive a similar result by replacing Y with X: var(X) = cov(X, X) = E[ X − E(X) X]. 14 Judith Guo ECON20110/30370 Thus, we arrive at the final expressions for the population regression coefficients: cov(X,Y ) β0 = E(Y ) − β1 E(X) and β1 =. (2.5) var(X) These are the coefficients obtained from a linear regression of Y on a single variable X in the population. Note that: If Y does not vary at all, or if it does not vary with X, this implies cov(X,Y ) = 0 and consequently β1 = 0. If X does not vary at all, var(X) = 0. In this case, neither β1 nor β0 is identifiable (meaning neither of the two could be uniquely determined), as there exist infinitely many solutions to the minimisation problem. 2.3.2 The Population Regression Model The population regression function: β0 + β1 X ≈ E(Y |X) Y β1 : slope (≈)E[Y j |X j ] u j :the error term Yj (X j ,Y j ): observed data point intercept → β0 X 0 Xj Fig. 2.4 The Population Regression Line In reality, not all observations fall exactly on the regression line. To account for this, we introduce an error term, resulting in the population regression model: Yi = β0 + β1 Xi + ui. (2.6) The error term, ui , represents the difference between the population regression line, β0 + β1 Xi , and the observed value of Y. In other words, ui := Yi − β0 − β1 Xi. By construction, based on the two FOCs, we know: 1. E(u) = 0 — the error has a mean of zero; 15 Judith Guo ECON20110/30370 2. E(uX) = 0 — the error is orthogonal to X. These conditions are jointly referred to as the orthogonality condition (OR), as seen in the lecture slides. The linear regression function/line, β0 + β1 X, provides the best linear predictor of Y on the basis of X. It also serves as the best linear approximation to the conditional expectation of Y given X, i.e., E(Y |X) ≈ β0 + β1 X. The approximation symbol (‘≈’) becomes an equality (‘=’) if the conditional expectation is exactly linear, which happens under the stronger assumption of conditional mean independence (CMI), i.e., E(u|X) = 0. (See the next section for more details on the relationship between the linear regression function and conditional expectation.) Next, let’s compare the expressions for the sample estimators in equation (2.2) with the population parameters in equation (2.5): βb0 = Y − βb1 X vs β0 = E(Y ) − β1 E(X) cov(X,Y c ) cov(X,Y ) βb1 = vs β1 =. var(X) c var(X) From earlier statistics courses, you may recall the law of large numbers (LLN). In simple terms, the LLN states that as the sample size grows larger, the sample averages (like Y and X), get closer to the true population averages E(Y ) and E(X), respectively. This means that, with a sufficiently large sample, the sample estimates of quantities like the mean, covariance, and variance will converge to the true population values. Specifically, for independent and identically distributed (i.i.d.) data, the LLN implies that: 1 n p Y= ∑ Yi → E(Y ) n i=1 1 n p X= ∑ Xi → E(X) n i=1 1 n p cov(X,Y c )= ∑ (Yi −Y )(Xi − X) → cov(X,Y ) = E{[Y − E(Y )][X − E(X)]} n − 1 i=1 1 n p var(X) c = ∑ (Xi − X)2 → var(X) = E{[X − E(X)]2 } n − 1 i=1 16 Judith Guo ECON20110/30370 In other words, as the sample size increases, our sample estimates become more accurate representa- tions of the population parameters. As a result, we conclude that: p p βb0 → β0 and βb1 → β1. Thus, the OLS estimators βb0 and βb1 consistently estimate the corresponding population parameters in the regression model. In simple terms, consistency means that as the sample size grows infinitely large, the estimators will converge to the true population parameters. So, as the sample size increases, the OLS estimates βb0 and βb1 will get closer and closer to the true population values β0 and β1. 2.4 Linear Regression and the Conditional Expectation The regression function, represented by β0 + β1 X, describes the relationship between Y and X by providing the best linear prediction for Y based solely on X. This naturally raises a broader question: Beyond just linear predictions, how does the average value of Y change as X changes? To answer this, we use the conditional expectation of Y given X, defined as: µ(X) := E[Y | X]. Although there is a significant connection between the regression function and the conditional expec- tation, they are not identical. Each solves a different prediction problem: 1. The linear regression function solves the problem of finding the best linear prediction: min E [Y − (b0 + b1 X)]2 (2.7) b0 ,b1 2. The conditional expectation solves a broader problem: it finds the best general prediction among all possible functions of X, including nonlinear ones. This means solving: min E[Y − m(X)]2 (2.8) m(·) where m(X) represents any function of X, whether linear or nonlinear, and the solution is m∗ (X) = E[Y | X] = µ(X), i.e., the best possible function. [Try working through this proof 17 Judith Guo ECON20110/30370 yourself.] When do the regression function and conditional expectation coincide? – They are the same when the conditional expectation is linear. In this case, the linear and general prediction problems have the same solution. The conditional expectation is linear if E(u|X) = 0, which is known as the conditional mean independence (CMI) condition. CMI means that the error term u has an expected value of zero for any value of X. In other words, knowing X gives no additional information about the average value of u. This assump- tion guarantees that the relationship between Y and X is fully captured by a linear function. To understand this, consider: E[Y | X] = E [β0 + β1 X + u | X] by substituting the linear regression model for Y = β0 + β1 X + E[u | X] by linearity of E[· | X] and conditioning. If E[u | X] = 0 (CMI), then the conditional expectation E[Y | X] is linear, i.e., E[Y | X] = β0 + β1 X. Conversely, if E[Y | X] is nonlinear, this nonlinearity must come from E[u | X]. However, we only have E(Xu) = 0 by construction, and CMI is a stronger assumption. – If the conditional expectation is nonlinear, the regression function provides the best linear approximation to E[Y | X]. You can also show that the pair (β0 , β1 ) solves: min E {E[Y | X] − (b0 + b1 X)}2. b0 ,b1 This minimises the distance between the conditional expectation and a best-fitting straight line based on X. [You are asked to show this in Homework 2.] When the two coincide, the conditional expectation and the linear regression function are identical: E[Y | X] = β0 + β1 X. This equality implies: ∂ E[Y | X] β1 = , ∂X which means that β1 represents the rate of change in the conditional mean of Y with respect to X. 18 Judith Guo ECON20110/30370 – In simpler terms, β1 represents the change in the average value of Y associated with a one- unit increase in X. Since we are focusing on the statistical relationship, this is purely a de- scriptive interpretation of the association between X and Y , not a statement about cause and effect. – Even when the linear regression function is not an exact representation of the conditional ex- pectation (i.e., when the conditional expectation is nonlinear), β1 is still often interpreted in a similar way. This is because the linear regression function provides the best linear approxi- mation to the true conditional expectation of Y given X. 2.5 Derivation of the OLS Solutions Recall that the sample regression problem is given by equation (2.1): n min S(b0 , b1 ) := min b0 ,b1 ∑ (Yi − b0 − b1 Xi )2. b0 ,b1 i=1 Our goal is to find the values of b0 and b1 that minimise this sum of squared errors. To do this, we take the partial derivatives of the criterion function S(b0 , b1 ) w.r.t. b0 and b1 , and set them to zero. These are the FOCs: n n ∂ S(b0 , b1 ) = −2 ∑ (Yi − b0 − b1 Xi ) = 0 =⇒ ∑ (Yi − b0 − b1 Xi ) = 0 ∂ b0 i=1 i=1 n n ∂ S(b0 , b1 ) = −2 ∑ (Yi − b0 − b1 Xi )Xi = 0 =⇒ ∑ (Yi − b0 − b1 Xi )Xi = 0. ∂ b1 i=1 i=1 These conditions are uniquely solved by (βb0 , βb1 ), the OLS estimators. Replacing (b0 , b1 ) with (βb0 , βb1 ) in the FOCs gives: n ∑ (Yi − βb0 − βb1 Xi ) = 0 (2.9) i=1 n ∑ (Yi − βb0 − βb1 Xi )Xi = 0. (2.10) i=1 Solving for βb0 : 19 Judith Guo ECON20110/30370 Divide both sides of equation (2.9) by n to obtain: 1 n ∑ (Yi − βb0 − βb1 Xi ) = 0 n i=1 =⇒ βb0 = Y − βb1 X. (2.11) This equation expresses the OLS estimate of the intercept βb0 in terms of the sample means of Y and X, and the slope βb1. Solving for βb1 : Now, substitute equation (2.11) into equation (2.10): n n 0 = ∑ [Yi − (Y − βb1 X) − βb1 Xi ]Xi = ∑ [(Yi −Y ) − βb1 (Xi − X)]Xi. i=1 i=1 Expanding the terms inside the square brackets gives: n n 0 = ∑ (Yi −Y )Xi − βb1 ∑ (Xi − X)Xi. i=1 i=1 Rearranging to isolate βb1 : ∑n (Yi −Y )Xi βb1 = ni=1 ∑i=1 (Xi − X)Xi Now, observe that for the right-hand side (RHS), the following identity holds: =0 z }| { n ∑ni=1 (Yi −Y )(Xi − X) ∑ni=1 (Yi −Y )Xi − X ∑ (Y −Y ) i=1 i ∑ni=1 (Yi −Y )Xi n = n =. ∑i=1 (Xi − X)2 ∑ni=1 (Xi − X)Xi − X ∑ (X − X) i=1 i ∑ni=1 (Xi − X)Xi | {z } =0 Therefore: ∑n (Yi −Y )(Xi − X) βb1 = i=1 n ∑i=1 (Xi − X)2 1 Finally, note that multiplying both the numerator and denominator by n−1 does not change the ratio. Using the definitions of the sample covariance and variance, we obtain: 1 n−1 ∑ni=1 (Yi −Y )(Xi − X) cov(X,Y c ) βb1 = 1 =. (2.12) n−1 ∑ni=1 (Xi − X)2 var(X) c 20 Judith Guo ECON20110/30370 As a result, the OLS estimators are: cov(X,Y ) βb0 = Y − βb1 X c and βb1 =. var(X) c 2.5.1 Some Properties of OLS Solutions The following properties are mechanical, as are derived directly from the FOCs of the OLS problem: 1. The sum and sample average of the OLS residuals are zero: ∑ni=1 ubi = 0 =⇒ ub := 1n ∑ni=1 ubi = 0. This follows from equation (2.9) noting that ubi = Yi − βb0 − βb1 Xi. This property always holds if an intercept is included in the model. Note: For the population error term u in the population regression model, the expected value is zero, E(u) = 0. However, the sample average of u does NOT necessarily equal zero due to sampling variation, meaning u := 1n ∑ni=1 ui ̸= 0. 2. The OLS residuals are orthogonal to the regressors in the sample: ∑ni=1 ubi Xi = 0. This follows from equation (2.10). Because the sample mean of the OLS residuals is zero, this also implies that the sample covariance between the residuals and the regressors is zero: 1 n 1 n 1 n n c u, X) = cov(b ∑ (b ub )(Xi − X) = ui − |{z} ∑ ubi (Xi − X) = [ ∑ ubi Xi −X ∑ ubi ] = 0. n − 1 i=1 n − 1 i=1 n − 1 i=1 i=1 =0 | {z } | {z } =0 =0 3. The OLS residuals are orthogonal to the fitted values in the sample: ∑ni=1 ubiYbi = 0. This can be derived as follows: n n n n ∑ ubiYbi = ∑ ubi (βb0 + βb1 Xi ) = βb0 ∑ ubi +βb1 ∑ ubi Xi = 0. i=1 i=1 i=1 i=1 | {z } | {z } =0 =0 This also shows that the residuals are uncorrelated with the predicted values in the sample, since residuals have mean zero. 21 Judith Guo ECON20110/30370 However, the OLS residuals are correlated with the observed values: n n n n n ∑ ubiYi = ∑ ubi (Ybi + ubi ) = ∑ ubiYbi + ∑ ub2i = ∑ ub2i , i=1 i=1 i=1 i=1 i=1 | {z } =0 where ∑ni=1 ub2i is the sum of squared residuals (RSS). The RSS is generally not zero unless the model provides a perfect fit. 4. The point (X,Y ) is always on the fitted line if an intercept is included. The fitted line is given by Ybi = βb0 + βb1 Xi. Substituting βb0 = Y − βb1 X into this equation gives: Ybi = Y − βb1 X + βb1 Xi = Y + βb1 (Xi − X). When Xi = X, the equation simplifies to Ybi = Y , meaning the fitted line passes through the point (X,Y ). 2.6 Recap: Sample and Population Regression Problem Table 2.1 summarises the relationship between the sample and population regressions. Table 2.1 Sample and Population Regression Sample Population The regression problem min(b0 ,b1 ) ∑ni=1 (Yi − b0 − b1 Xi )2 min(b0 ,b1 ) E[(Y − b0 − b1 X)2 ] The regression model Yi = β0 + β1 Xi + ubi b b Yi = β0 + β1 Xi + ui The regression line βb0 + βb1 X(= 0 + β1 X(≈ ( E[Y |X]) b) Y β ( βb0 = Y − βb1 X β0 = E(Y ) − β1 E(X) Coefficients Sample Estimators b Population Parameters ) cov(X,Y β = var(X) c ) β1 = cov(X,Y ( 1 c ( var(X) ∑ni=1 ubi = 0 E(u) = 0 By construction the residuals: n the error terms: ∑i=1 ubi Xi = 0 E(uX) = 0 Notation With hats No hats p ( βb0 → β0 Relationship if i.i.d. data =⇒ b p β1 → β1 2.7 Descriptive Interpretation of Regression Coefficients Consider the simple linear regression model: Y = β0 + β1 X +u ≈ E[Y |X] + u. | {z } ≈E[Y |X] 22 Judith Guo ECON20110/30370 population regression line Y E[Yi |Xi ] ≈ β0 + β1 Xi △E[Y |X] △Yb sample regression line Ybi = βb0 + βb1 Xi X 0 △x = 1 unit Fig. 2.5 The Simple Linear Regression Model As shown in Figure 2.5, the slope parameter β1 represents the (approximate) change in the condi- tional expectation of Y when X increases by one unit. Formally, we can express this as: ∂ E[Y |X] β1 ≈. ∂X This expression also highlights that a one-unit increase in X is associated with an dentical change in the expected value of Y , regardless of the initial value of X. ∂Y Note that β1 ̸= ∂X , unless OR holds in a causal model,which will be discussed in Section 2.9. The intercept β0 can generally be interpreted as the conditional expectation of Y when X = 0. How- ever, this interpretation only makes sense if X = 0 is a meaningful value. For instance, if X represents height and Y represents wage, it doesn’t make sense to interpret β0 as the expected wage when height is zero. The corresponding sample regression model is: Y = βb0 + βb1 X +b u = Yb + ub. | {z } =Yb The fitted value Yb is the sample counterpart of E[Y |X]. Recall that the expectation is a weighted average of potential outcomes, with the weights determined by the associated probabilities. The fitted value, Yb , which consistently estimates E[Y |X], quantifies the predicted average response of Y when we input a given value of X into the model. The slope estimator, βb1 , which consistently estimates β1 , represents the average change in Y pre- 23 Judith Guo ECON20110/30370 dicted by the model when there is a one unit increase in X. 2.8 Comparing Correlation and Slope Coefficient You may have noticed that the slope coefficient and the correlation coefficient are mathematically related, and their similarity reflects this connection. Recall that the correlation coefficient between X and Y is given by: cov(X,Y ) corr(X,Y ) = p var(X) var(Y ) Meanwhile, the slope in the simple linear regression Yi = β0 + β1 Xi + ui takes the form: cov(X,Y ) β1 =. var(X) Observe that we can rearrange the correlation as: s s cov(X,Y ) var(X) var(X) corr(X,Y ) = = β1. var(X) var(Y ) var(Y ) | {z } =β1 While the sign of the slope (β1 ) will always match the sign of the correlation coefficient, there are two key differences between them: 1. The value of the correlation coefficient reflects the strength of the linear relationship, whereas the slope does not. =⇒ The magnitude of the slope does NOT provide information about the strength of the associ- ation between X and Y. In other words, a steep slope doesn’t necessarily imply a strong relationship. 2. The slope measures the change in the expected value of Y for a one-unit increase in X. Correlation, on the other hand, does not have this specific interpretation. Correlation simply quantifies the linear association between two variables, but it doesn’t provide insight into how much Y changes when X changes. 2.9 Causal Model and Linear Regression Model When discussing the causal relationship between X (the cause) and Y (the outcome), it’s important to recognise that Y is usually influenced by more than just X. Typically, many other factors also affect Y. To 24 Judith Guo ECON20110/30370 make this more manageable (for analysis), we assume that the effects of all causal determinants of Y are additive, meaning they combine in a straightforward way, without interacting with each other. This assumption leads us to a linear causal model, where the combined influence of all other factors is captured by an additive error term. The error term represents everything affecting Y apart from X, and the model is written as: Y = φ0 + φ1 X + ε, where the error term ε captures all factors that influence Y aside from X. It is important to note that causality is a conceptual idea, not something inherently defined by mathe- matics or statistics. As such, it remains abstract and difficult to analyse without a structured mathematical framework. By making certain assumptions, we can express causal relationships in mathematical terms, giving substance to these ideas and transforming them from theoretical notions into something we can anal- yse and estimate. In this case, we have translated the causal relationship between Y and its determinants into a linear model. However, this alone is insufficient, because without additional assumptions and con- ditions, we cannot uniquely determine (or, in econometric terms, identify) the coefficients φ0 and φ1 , nor consistently estimate them. At first glance, this linear causal model appears similar to the population regression model we’ve dis- cussed earlier. However, there is a key difference: in the causal model, the coefficients φ0 and φ1 represent the causal relationship, while in the population regression model, we have β0 and β1 , which describe the best linear prediction. Additionally, the error term ε in the causal model captures all factors influencing Y , whereas in the regression model, the error term u represents the prediction error, i.e., the difference between the observed value of Y and the value predicted by the regression line. Even with data on the entire popu- lation, we cannot directly observe the values of φ0 and φ1 , but we can determine β0 and β1 , which depends on the expectations, covariance and variance of the variables. This raises a fundamental question: Under what conditions can we mathematically link the population regression model to the causal model? In other words, when does a causal model numerically coincide with a population regression model, allowing us to confidently use OLS to estimate the true causal effect? Now, let’s revisit the population regression model: Y = β0 + β1 X + u, 25 Judith Guo ECON20110/30370 where by construction, we know that E(u) = 0 and E(uX) = 0. From this, we can derive: β0 = E(Y ) − β1 E(X) and cov(X,Y ) β1 =. var(X) To uniquely identify φ0 and φ1 in the causal model, one way is to ensure that β0 = φ0 and β1 = φ1. But how can we guarantee these equalities hold? This requires that the error term in the causal model, ε, satisfies the orthogonality condition. Specifically, we need to assume: E(ε) = 0 and E(εX) = 0 in the causal model as well. Under these assumptions, the causal model becomes mathematically equivalent to the population regression model, meaning the β ’s and φ ’s are numerically identical. However, it’s crucial to remember that they remain conceptually distinct objects—the β ’s describe statistical relationships, while the φ ’s reflect causal effects. For a formal proof of this mathematical equivalence, see Appendix 2.A. Note that E(ε) = 0 and E(εX) = 0 together imply cov(X, ε) = 0. This is because: cov(X, ε) = E(εX) − E(ε) ·E(X) = E(εX) = 0. |{z} =0 Thus, the orthogonality condition can also be written as E(ε) = 0 and cov(X, ε) = 0. This formulation is often found in econometrics textbooks, where cov(X, ε) = 0 is sometimes referred to as the exogeneity condition. It is important to recall that the β ’s, the parameters of the population regression model, can always be consistently estimated by OLS. This means that, under the orthogonality condition (OR), the OLS estimators also consistently estimate the φ ’s, which represent the causal effects in a causal model. Therefore, we have: p βb0 = Y − βb1 X → β0 =if OR holds in the causal model φ0 26 Judith Guo ECON20110/30370 and cov(X,Y ) p → β1 =if OR holds in the causal model φ1. c βb1 = var(X) c Another way to understand the importance of OR is through the definition of causal effects. In causal analysis, it is crucial to account for other factors when identifying the causal effect. We need to constantly ask ourselves: Is the observed change in Y due to X alone, or could it be influenced by other determinants captured in the error term ε? The causal effect of X on Y is measured by taking the partial derivative of Y w.r.t. X in the causal model: ∂Y ∂ε = φ1 +. ∂X ∂X When OR holds, we can safely assume the second term on the right-hand side is zero, meaning: ∂Y = φ1. ∂X In other words, under OR, X is uncorrelated with all other factors in the error term, so that a change in X should NOT be associated with a change in ε. This condition allows us to interpret φ1 as the causal effect of X on Y , as we can isolate the effect of X while holding everything else affecting Y constant. It is important to note that the error terms in causal models and descriptive models are conceptually different: In a descriptive model, the error term is defined as the difference between the observed value of Y and the population linear regression function, which satisfies OR by construction. On the other hand, in a causal model, the error term captures all other omitted determinants of Y. These omitted factors may be correlated with the included X-variable, meaning OR must be assumed or verified. 2.10 Should we assume OR or CMI? From a practical standpoint, the choice between the orthogonality condition (OR) and conditional mean independence (CMI) has minimal impact. As shown in the previous section, the OR assumption is sufficient to identify the coefficients (φ0 , φ1 ) in the causal model. Regardless of whether we assume OR or CMI, we use OLS to estimate (φ0 , φ1 ) This raises an important question: Why do most textbooks prefer CMI over OR? While it’s true that 27 Judith Guo ECON20110/30370 cov(X, ε) = 0 (uncorrelation) doesn’t imply E[ε | X] = 0 (CMI), having E[ε | X] ̸= 0 means that the condition cov(X, ε) = 0 holds only if the marginal distribution of X satisfies a specific, somewhat uncommon constraint. What happens when we assume CMI? Using the properties of conditional expectation, we can show that: E[Y | X] = E [φ0 + φ1 X + ε | X] (substituting the causal model for Y ) = φ0 + φ1 E[X | X] + E[ε | X] (applying linearity of E[· | X]) = φ0 + φ1 X (using CMI). (2.13) This shows that φ0 + φ1 X coincides with the conditional expectation of Y given X. Equation (2.13) provides a simpler way to demonstrate that, under CMI, a population linear regression of Y on X will recover (φ0 , φ1 ). Here’s why: – First, since E[Y | X] solves the general prediction problem in equation (2.8), it is the optimal nonlinear predictor of Y based solely on X. – Second, because equation (2.13) shows that this prediction is linear, it is also the best linear predictor for Y. – Therefore, the conditional expectation coincides with the linear regression function, implying that φ0 = β0 and φ1 = β1. In summary, the conditional expectation E[Y | X] depends only on X and is generally nonlinear. However, when E[ε | X] = 0, the conditional expectation becomes linear in X, aligning with the linear regression function and making the causal model numerically equivalent to the population linear regression model. [An interesting observation is that, in this case, we also have E[u | X] = 0. Can you think about why this happens?] 28 Appendix 2.A Numerical Equivalence of the Causal and Population Regression Mod- els under OR Question: Under what assumptions on the causal model does a population regression model of Y on X recover the causal model coefficients, i.e., β0 = φ0 , and β1 = φ1 ? For β0 : β0 = E(Y ) − β1 E(X) =(1) E(φ0 + φ1 X + ε) − β1 E(X) = φ0 + (φ1 − β1 )E(X) + E(ε). where =(1) follows from substituting the causal model for Y. Observe that β0 = φ0 if φ1 = β1 and E(ε) = 0. For β1 : cov(Y, X) cov (φ0 + φ1 X + ε, X) β1 = =(2) var(X) var(X) φ1 var(X) + cov(ε, X) =(3) var(X) cov(ε, X) = φ1 + var(X) where =(2) follows from plugging the causal model into Y , and =(3) comes from: – cov(φ0 , X) = 0 because φ0 is a constant, and the covariance between a constant and any random variable is always zero; – cov(X, X) = var(X). Thus, β1 = φ1 if cov(ε, X) = 0. 29 Judith Guo ECON20110/30370 From the above, if X and the error term ε in the causal model are uncorrelated, we have β1 = φ1. If E(ε) = 0 also holds, this further ensures β0 = φ0. These two conditions jointly imply the orthogonality condition (OR), because if cov(ε, X) = 0 and E(ε) = 0, it follows that E(εX) = 0. Recall that cov(ε, X) = E(εX) − E(ε)E(X). Therefore, when OR holds in the causal model, the parameters of the population regression model mathematically coincide with the causal model coefficients. This highlights the critical role of OR in ensuring that the OLS estimates can be interpreted ‘causally’. 30 3 Multiple Linear Regressions In the previous chapter, we focused on simple linear regressions, where the relationship between a de- pendent variable Y and a single explanatory variable X was explored. While this model provides valuable insights, it is often the case that Y is correlated with several X variables or depends on multiple factors. In this chapter, we generalise the simple linear regression model to the multiple linear regression model, which allows us to examine how Y is related to multiple explanatory variables simultaneously. It is important to emphasise that the population regression model is primarily descriptive: it sum- marises the association between Y and the X variables in the population. This model shows how the aver- age value of Y changes as the X variables vary, but it does not necessarily imply causality. By contrast, a causal model aims to identify the direct effect of changes in the X variables on Y. Establishing causality requires stronger assumptions, such as that all omitted determinants of Y is uncorrelated with the included X variables. Many of the key results from the simple regression case, such as the interpretation of coefficients, the assumptions/conditions underlying the model, and the method of ordinary least squares (OLS), extend natu- rally to this more general setting. We will build on these concepts to explore how multiple regressors affect estimation, interpretation, and inference, while carefully distinguishing between descriptive associations and causal interpretations. 3.1 The Population Regression Model The general form of the population multiple linear regression model is: Y = β0 + β1 X1 + β2 X2 + · · · + βk Xk + u. (3.1) Here: 31 Judith Guo ECON20110/30370 Y is the dependent variable (what we’re trying to explain or predict). X1 , X2 ,... , Xk are the explanatory variables (also known as regressors). β0 is the intercept, and β1 , β2 ,... , βk are the slope coefficients that describe the relationship between each X and Y. The population parameters (β0 , β1 ,... , βk ) are the values that solve the following population regres- sion problem: min C(b0 , b1 ,... , bk ) = min E[(Y − b0 − b1 X1 − b2 X2 − · · · − bk Xk )2 ]. (3.2) b0 ,b1 ,...,bk b0 ,b1 ,...,bk This expression minimises the expected squared difference between the observed value of Y and its predicted value based on a linear function the regressors. u is the error term, representing the difference between the observed value of Y and the value predicted by the population regression function β0 + β1 X1 + β2 X2 + · · · + βk Xk : u = Y − (β0 + β1 X1 + β2 X2 + · · · + βk Xk ). By construction, the error term u must satisfy two key conditions that are similar to those in Sec- tion 2.3: 1. Zero mean: The error term has an expected value of zero, i.e., E(u) = 0. This means that, on average, the model does not systematically underpredict or overpredict Y. 2. Orthogonality: The error term is orthogonal to the regressors, i.e., E(Xl u) = 0 for each l = 1, 2,... , k. These conditions imply that the error term is uncorrelated with the explanatory variables: cov(Xl , u) = 0 ∀l ∈ {1, 2,... , k}. The population regression function, β0 + β1 X1 + β2 X2 + · · · + βk Xk , provides the best linear ap- proximation to the conditional expectation (CE) of Y given the values of X1 , X2 ,... , Xk. This is expressed as: E(Y |X1 , X2 ,... , Xk ) ≈ β0 + β1 X1 + β2 X2 + · · · + βk Xk. 32 Judith Guo ECON20110/30370 The symbol “≈” indicates that this is an approximation, which becomes an exact equality (“=”) if the CE is linear. In that case, the population regression function provides the exact conditional expectation of Y given the values of X1 , X2 ,... , Xk. Note that the CE represents the best guess we can make for Y given the values of X1 , X2 ,... , Xk , while the population regression function is the best linear prediction we can make for Y using these variables. 3.2 The Sample Regression Model In practice, we don’t have access to the entire population, so we use sample data to estimate the population multiple linear regression model. The sample multiple linear regression model (or OLS regression model) is similar to the population model but uses estimates instead of the true population values: Y = βb0 + βb1 X1 + βb2 X2 + · · · + βbk Xk +b u. (3.3) | {z } =:Yb Here: βb0 , βb1 ,... , βbk are our estimated coefficients, and Yb is the fitted value, the predicted value of Y based on the sample regression line. The OLS estimators (βb0 , βb1 ,... , βbk ) are solutions to the sample regression problem (i.e., minimising the sum of squared prediction errors in the sample): n min b0 ,b1 ,...,bk S(b0 , b1 ,... , bk ) := min ∑ (Yi − b0 − b1 X1i − b2 X2i − · · · − bk Xki )2. b0 ,b1 ,...,bk i=1 (3.4) This means the OLS method finds the line that best fits the data by minimising the total prediction error across all observations. The difference between the actual value of Y and the fitted value Yb is called the residual, denoted as ub: ub = Y − Yb = Y − (βb0 + βb1 X1 + βb2 X2 + · · · + βbk Xk ). The residual represents the prediction error, i.e., the part of Y that is not explained by the model. By construction, the residuals have some important properties: 33 Judith Guo ECON20110/30370 1. Zero sample mean: The sum, and the average, of the residuals in the sample is zero, i.e., ∑ni=1 ubi = 0 =⇒ ub = 1n ∑ni=1 ubi = 0. This ensures that, on average, the model fits the data without systematically under- or over-predicting in the sample. 2. Orthogonality to Regressors: The residuals are orthogonal to the regressors in the sample, i.e., ∑ni=1 ubi Xli = 0 for each l ∈ {1, 2,... , k}. The zero sample mean and orthogonality together imply that the sample covariance between the residuals and the regressors is zero: c u, Xl ) = 0 cov(b ∀l ∈ {1, 2,... , k}. 3. Orthogonality to Fitted Values: The residuals are also orthogonal to the fitted values, i.e., ∑ni=1 ubiYbi = 0. This implies that the fitted values and the sample prediction errors are uncorre- c u, Yb ) = 0 lated, cov(b 3.3 The FWL Theorem 3.3.1 Introduction Consider a multiple linear regression model: Y = β0 + β1 X1 + β2 X2 + · · · + βk Xk + u, (3.5) and suppose we are primarily interested in β1 , the coefficient on X1 , but not as much in the other coef- ficients. How can we find the expression for β1 or its OLS estimator, βb1 ? There are several ways to do this. One direct (but somewhat crude) method is to solve the regression problem in (3.2) or (3.4), which involves differentiating the criterion function and solving the k + 1 FOCs for the k + 1 unknowns. While this method works, it has some drawbacks. The computations can be long, the results may be cumbersome, and it’s easy to make mistakes. More importantly, the final outcome may not offer much intuitive insight. Instead, we can use a more elegant approach based on orthogonalisation, known as the Frisch-Waugh- Lovell (FWL) theorem. This method produces the same result as the direct approach but offers several advantages: cleaner notation, simpler calculations, and more intuitive interpretation. There are various ways to apply orthogonalisation, but here we will focus on the most straightforward one. Another method, 34 Judith Guo ECON20110/30370 commonly found in textbooks such as Chapter 3 of Stock and Watson (3rd ed.)1 , involves an additional step but leads to the same outcome. For more details on this alternative, refer to Appendix 3.A. Orthogonalisation is essential in multiple regression models, especially when dealing with correlated regressors. It helps isolate the unique statistical effect of each regressor by extracting the part it orthogonal (uncorrelated) with the others. This allows us to better understand the unique contribution of each X- variable to the dependent variable without the interference of other correlated variables. 3.3.2 The Need for Orthogonalisation in Multiple Linear Regression At its core, regression analysis is about understanding relationships. Suppose you want to know how your test score is related to the number of hours you study. But we also know that your test score could depend on other factors like the number of hours you sleep, the quality of your study materials, or the time of day you study. To account for these other factors, we use multiple linear regression. However, there’s a problem when some of these factors are related to each other. For instance, students who study during the day might also tend to get more sleep. This relationship between predictors makes it hard to isolate the unique effect of each one on your test score. This is where orthogonalisation comes in. It helps ‘disentangle’ these intertwined relationships by ensuring that each predictor captures only its own unique variation, unaffected by the others. In simpler terms, it’s like separating overlapping sections in a Venn diagram so that each predictor stands on its own. This allows us to pinpoint the distinct effect of each variable without interference from the rest. 3.3.3 The Procedure In this subsection, we begin by deriving the OLS estimator for β1 , then extend the approach to the population parameter β1 , and finally generalise the method for any predictor Xl in the model. To derive the OLS estimator for β1 , we can follow these steps: Step 1. Run an OLS regression of X1 on (X2 , X3 ,... , Xk ) to obtain the residuals, X b̃ : 1 X1 = α b0 + α b3 X3 + · · · + α b2 X2 + α bk Xk + X b̃. 1 (3.6) b̃ satisfies the following properties in the sample by construction: The residual X 1 (a) The sum and sample mean are both zero: ∑ni=1 X b̃ = 1 ∑n X b̃ = 0 =⇒ X n i=1 1i = 0. b̃ 1i 1 1 Stock, J.H., and Watson, M.W. (2014). Introduction to econometrics (Updated third edition, Global edition.). Pearson Education Limited. 35 Judith Guo ECON20110/30370 (b) It is orthogonal to the other regressors in the sample: ∑ni=1 X b̃ X = 0 for all l ∈ {2,... , k}. 1i li The key idea here is to break X1 into two parts: b0 + α – One part that depends on the other X-variables: α b3 X3 + · · · + α b2 X2 + α bk Xk. b̃ , which is orthogonal (uncorrelated) to all other X-variables. This – The other part, X 1 residual X b̃ is key to deriving βb , because it captures the unique variation in X. 1 1 1 Why is this step important? We want to isolate the part of X1 that is not explained by the other predictors. By removing the influence of X2 , X3 ,... , Xk from X1 , we get a ‘clean’ version of X1 that is free from any correlation with the other predictors. – Intuition: Imagine X1 is a mixture of multiple flavours. By regressing it on the other variables, we separate out the unique flavour of X1 that isn’t shared with the others. This unique flavour is represented by the residuals, X b̃. 1 Step 2. Run an OLS regression of Y on X b̃ to obtain the OLS estimator for β : 1 1 Y = γb0 + βb1 X b̃ + vb. 1 (3.7) Note that the estimated intercept γb0 and residual vb here are different from the intercept βb0 and residual ub in equation (3.3), where Y is regressed on all X-variables (X1 , X2 ,... , Xk ). To see the difference, plug equation (3.6) into the OLS regression model (3.3) for X1 : Y = βb0 + βb1 X1 + βb2 X2 + · · · + βbk Xk + ub = βb0 + βb1 (α b0 + α b3 X3 + · · · + α b2 X2 + α b̃ ) + βb X + · · · + βb X + ub bk Xk + X 1 2 2 k k = βb0 + βb1 α b̃ + (βb α b +βb X b3 + βb3 )X3 + · · · + (βb1 α b + βb2 )X2 + (βb1 α bk + βbk )Xk + ub | {z }0 1 1 | 1 2 {z } =b γ0 =b v = γb0 + βb1 X b̃ + vb. 1 (3.8) Why is this step important? After isolating the unique variation in X1 (in Step 1), we now want to see how this unique variation relates to Y. This step tells us how much Y changes when this unique part of X1 changes, holding the influence of other predictors constant. 36 Judith Guo ECON20110/30370 – Intuiti