Deep Learning and Artificial Neural Networks for Spacecraft Dynamics, Navigation and Control PDF
Document Details
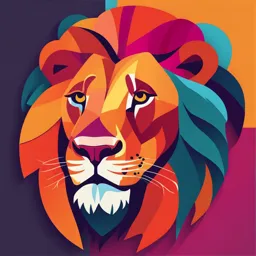
Uploaded by SweepingAqua
Politecnico di Milano
Stefano Silvestrini and Michèle Lavagna
Tags
Summary
This is a review paper on deep learning and artificial neural networks for spacecraft guidance, navigation, and control (GNC). It explores various aspects, including common architectures and training methods, showcasing specific applications, such as system identification and optical navigation. A comparison is provided in quantitative and qualitative metrics. It examines theoretical bases for end-to-end deep learning frameworks within the GNC domain as well as with traditional algorithms.
Full Transcript
drones Review Deep Learning and Artificial Neural Networks for Spacecraft Dynamics, Navigation and Control Stefano Silvestrini * and Michèle Lavagna Department of Aerospace Science and Technologies, Politecnico di Milano, 20156 Mi...
drones Review Deep Learning and Artificial Neural Networks for Spacecraft Dynamics, Navigation and Control Stefano Silvestrini * and Michèle Lavagna Department of Aerospace Science and Technologies, Politecnico di Milano, 20156 Milan, Italy * Correspondence: [email protected] Abstract: The growing interest in Artificial Intelligence is pervading several domains of technology and robotics research. Only recently has the space community started to investigate deep learning methods and artificial neural networks for space systems. This paper aims at introducing the most relevant characteristics of these topics for spacecraft dynamics control, guidance and navigation. The most common artificial neural network architectures and the associated training methods are examined, trying to highlight the advantages and disadvantages of their employment for specific problems. In particular, the applications of artificial neural networks to system identification, control synthesis and optical navigation are reviewed and compared using quantitative and qualitative metrics. This overview presents the end-to-end deep learning frameworks for spacecraft guidance, navigation and control together with the hybrid methods in which the neural techniques are coupled with traditional algorithms to enhance their performance levels. Keywords: ANN; spacecraft; GNC; deep learning; dynamics; autonomous; control; navigation Citation: Silvestrini, S.; Lavagna, M. 1. Introduction Deep Learning and Artificial Neural One of the major breakthrough in the last decade in autonomous systems has been Networks for Spacecraft Dynamics, the development of an older concept named Artificial Intelligence (AI). This term is vast Navigation and Control. Drones 2022, and addresses several fields of research. Moreover, Artificial Intelligence is a broad term 6, 270. https://doi.org/10.3390/ that is often confused with one of its sub-clustering terms. The well-known artificial neural drones6100270 networks (ANNs) are nearly as old as Artificial Intelligence, and they represent a tool, or a Academic Editor: Diego model, rather than a method by which to implement AI in autonomous systems. Nearly all González-Aguilera deep learning algorithms can be described as particular instances of a standard architecture: the idea is to combine a dataset for specification, a cost function, an optimization procedure Received: 31 August 2022 and a model, as reported in. Actually, for guidance, navigation and control, using a Accepted: 19 September 2022 dataset produces poor results due to distribution mismatching. Even for the case where Published: 22 September 2022 training is done in a simulated environment but not during deployment, the need to update Publisher’s Note: MDPI stays neutral the dataset using simulated observations and actions during training is justified by the with regard to jurisdictional claims in mentioned dataset distribution mismatch, as thoroughly presented in. Additionally, published maps and institutional affil- updating the dataset with incremental observations tends to reduce overfitting problems. iations. This survey presents the theoretical basis for the foundational work of [1,3–5]. In this overview, the focus is to catch a glimpse of the current trends in the implementation of AI- based techniques in space applications, in particular for what concerns hybrid applications of artificial neural networks and classical algorithms within the domains of guidance, Copyright: © 2022 by the authors. navigation and control. Even though the survey is restricted to these domains, the topic is Licensee MDPI, Basel, Switzerland. still very broad, and different perspectives can be found in recent surveys [6–12]. Most of the This article is an open access article analyzed surveys focus on a limited application, deeply investigating the technical solutions distributed under the terms and for a particular scenario. Table 1 compares the existing works with this manuscript. conditions of the Creative Commons Attribution (CC BY) license (https:// creativecommons.org/licenses/by/ 4.0/). Drones 2022, 6, 270. https://doi.org/10.3390/drones6100270 https://www.mdpi.com/journal/drones Drones 2022, 6, 270 2 of 39 Table 1. Comparison between recent survey works and this review. Ref. Highlights This Review The survey focuses on machine learning This paper extends the review to techniques in spacecraft control design. navigation and estimation in space. This paper extends the review to online The survey is limited to the relative estimation, AI-aided filtering and navigation task using deep learning machine learning spacecraft control. The survey thoroughly reviews multiple applications of machine learning This paper focuses on GNC applications, techniques, particularly focusing on FDIR. yielding also a mathematical tutorial for Moreover, it reports a review of the most the development of some of the common Edge AI boards applicable to presented applications. space-based systems. This paper entails a significant discussion The survey thoroughly reviews on the hybrid techniques that incorporate end-to-end guidance and control traditional algorithms to applications based on AI. AI-based approaches. The survey thoroughly reviews deep This paper focuses on GNC and learning methods for unmanned estimation for space-based systems. aerial vehicles. This paper extends the discussion to The survey focuses on reinforcement spacecraft navigation and estimation, learning applications for together with tutorial-like analysis of spacecraft control common artificial neural network architectures. The range of applications is from preliminary spacecraft design to mission operations, with an emphasis on guidance and control algorithms coupled with navigation; finally, perturbed dynamics reconstruction and classification of astronomical objects are emerging topics. Due to the very large number of applications, it is the authors’ intent to narrow down the discussion to spacecraft guidance, navigation and control (GNC), and the dynamics reconstruction domain. Nevertheless, besides those falling into the above-mentioned domains, the most promising applications in space of AI-based techniques are mentioned within the discussion of the most common network architectures. The major contributions of this paper are: to introduce the bases of machine learning and deep learning that are rapidly growing within the space community; to present a review of the most common artificial neural network architectures used in the space domain, together with emerging techniques that are still theoretical; to present specific applications extrapolating the underlying cores of the different al- gorithms; in particular, the hybrid applications are highlighted, where novel Artificial Intelligence techniques are coupled with traditional algorithms to solve their shortcomings; to provide a performance comparison of different neural approaches used in guidance, navigation and control applications that exist in the literature. In general, it is hard to attribute quantitative metrics to such evaluations, since the applicative scenarios reported in the literature are different. The paper attempts to condense the information into a more qualitative comparison. The paper is structured as follows: Section 2 presents the foundations of machine learning, deep learning and artificial neural networks, together with a brief theoretical overview of the main training approaches; Section 3 presents an overview of the most used artificial neural networks in the spacecraft dynamics identification, navigation, guidance and control applications. Section 4 reports the applications of several artificial neural networks in the context of spacecraft system identification and guidance, navigation and control systems. Finally, Section 5 draws the conclusions of the paper. Drones 2022, 6, 270 3 of 39 2. Machine Learning and Deep Learning This section provides the theoretical basis of machine learning and deep learning, which are fundamental to understanding the core characteristics of these approaches. The discussion focuses on the domain features that are useful and commonly adopted in specific space-based applications. The research on machine learning (ML) and deep learning (DL) is complex and extremely vast. In order to acquire proper knowledge on the topic, the author suggests referring to. Hereby, only the most relevant concepts are reported in order to contextualize the work developed in the paper. The first important distinction to mark is that between the terms machine learning and deep learning. The highlights of the two approaches are reported in Figure 1. Figure 1. Differences between machine learning and deep learning. Machine Learning learns to map input to output given a certain world representation (features) hand-crafted for each task. Deep learning is a particular kind of machine learning that aims at representing the world as a nested hierarchy of concepts, which are self-detected by the deep learning architecture itself. The paradigm of ML and DL is to develop algorithms that are data-driven. The information to carry out the task is gathered and derived from either structured or un- structured data. In general, one would have a given experience E , which can be easily thought as a set of data D = ( x1 , x2 ,... , xn ). It is possible to divide the algorithms into three different approaches: Supervised learning: Given the known outputs T = (t1 , t2 ,... , tn ), we learn to yield the correct output when new datasets are fed. Unsupervised Learning: The algorithms exploit regularities in the data to generate an alternative representation used for reasoning, predicting or clustering. Reinforcement Learning: Producing actions A = ( a1 , a2 ,... , an ) that affect the envi- ronment and receiving rewards R = (r1 , r2 ,... , rn ). Reinforcement learning is all about learning what to do (i.e., mapping situations to actions) so as to maximize a numerical reward. Even though the boundaries between the approaches are often blurred, the focus of this survey is to discuss algorithms that take advantage and inspiration from supervised and reinforcement learning. For this reason, few additional details are provided for such approaches. Tentative clusters of the different learning approaches and their most used algorithms are reported in Table 2. Drones 2022, 6, 270 4 of 39 Table 2. A comprehensive summary for the different learning approaches. Learning Features Task Algorithms Approach Support Vector Machines, Discriminant Analysis, Nearest Classification Neighbour, Artificial It learns by exploiting Neural Networks Supervised input–output Learning Linear regression, Ensemble methods, data pairs Decision Trees, Support Vector Regression Regression, Artificial Neural Networks K-means, Spectral clustering, It learns to Hierarchical clustering, Gaussian extrapolate patterns Clustering Mixture, Hidden Markov Models, Unsupervised and properties of the Artificial Neural Networks Learning structure of Principal Component Analysis, the dataset Dimensionality Linear Discriminant Analysis, Reduction Artificial Neural Networks It learns the action to Dynamica Programming, undertake based on Model-based Model-given methods, Reinforcement some inputs, in order Model-learned methods Learning to maximize a given reward. Value based methods, Model-free Policy-based methods 2.1. Supervised Learning Supervised learning consists of learning to associate some output with a given input, coherently with the set of examples of inputs ~x and targets ~t. Quite often, the targets ~t are provided by a human supervisor. Nevertheless, supervised learning refers also to approaches in which target states are automatically retrieved by the machine learning model; we use the term this way often throughout this survey. The typical applications of supervised learning are classification and regression. In a few words, classification is the task of assigning a label to a set of input data from among a finite group of labels. The output is a probability distribution of the likelihood of a certain input of belonging to a certain class. On the other hand, regression aims at modeling the relationships between a certain number of features and a continuous target variable. The regression task is largely employed in supervised learning reported in this survey. Supervised learning is applicable to multiple tasks, both offline [13,14] and online [15,16]. 2.2. Unsupervised Learning Unsupervised learning algorithms are fed with a dataset containing many features. The system learns to extrapolate patterns and properties of the structure of this dataset. As reported in , in the context of deep learning, the aim is to learn the underlying probability distribution of dataset, whether explicitly as in density estimation or implicitly for tasks such as synthesis or de-noising. Some other unsupervised learning algorithms perform other tasks, such as clustering, which consists of dividing the dataset into separate sets, i.e., clusters of similar experiences and data. The unsupervised learning approach has not yet seen widespread employment in the spacecraft GNC domain. 2.3. Reinforcement Learning Reinforcement learning is learning what to do, how to map observations to actions, so as to maximize a numerical reward signal. The learner is not told which actions to take, but instead must discover which actions yield the most reward by trying them. Drones 2022, 6, 270 5 of 39 One of the challenges that arises in reinforcement learning, and not in other kinds of learning, is the trade-off between exploration and exploitation. The agent typically needs to explore the environment in order to learn a proper optimal policy, which determines the required action in a given perceived state. At the same time, the agent needs to exploit such information to actually carry out the task. In the space domain, only for online deployed applications, the balance must be shifted towards exploitation, for practical reasons. Another distinction that ought to be made is between model-free and model-based reinforcement learning techniques, as shown in Table 3. Model-based methods rely on planning as their primary component, and model-free methods primarily rely on learning. Although there are remarkable differences between these two kinds of methods, there are also great similarities. We call an environmental model whatever information the agent can use to make predictions on what will be the reaction of the environment to a certain action. The environmental model can be known analytically, partially or completely unknown, i.e., to be learned. The model-based algorithms need a representation of the environment. If the agent requires learning the model completely, the exploration is still very important, especially in the first phases of the training. It is worth mentioning that some algorithms start off by mostly exploring, and adaptively trade off exploitation and exploration during optimization, typically ending with very little exploration. For the reasons above, the model-based approach seems to be beneficial in the context of this survey, as it merges the advantages of analytical base models, learning and planning. It is important to report some of the key concepts of reinforcement learning: Policy: defines the learning agent’s way of behaving at a given time. Mapping from perceived states of the environment to actions to be taken when in those states. Reward: at each time step, the environment sends to the reinforcement learning agent a single number called the reward. Value Function: the total amount of reward an agent can expect to accumulate in the future, starting from that state. Table 3. Differences between model-based and model-free reinforcement learning. In space, a deter- ministic representation of a dynamical model is generally available. Nevertheless, some scenarios are unknown (small bodies) or partially known (perturbations). Model-Free Model-Based Unknown system dynamics Learnt system dynamics The agent is not able to make predictions The agent makes prediction Need for explorations More sample efficient Lower computational cost Higher computational cost The standard reinforcement learning theory states that an agent is capable of obtaining a policy which provides the mapping between a set of states x ∈ X, where X is the set of possible states, for an action a ∈ A, where A is the set of possible actions. The dynamics of the agent are basically represented by a transition probability p( xk+1 | xk , ak ) from one state to another at a given time step. In general, the learned policy can be deterministic π ( xk ) or stochastic π ( ak | xk ), meaning that the control action follows a conditional probability distribution across the states. Every time the agent performs an action, it receives a reward r ( xk , ak ): the ultimate goal of the agent is to maximize the accumulated discounted reward R = ∑iN=k γi−k r ( xi , ai ) from a given time step k to the end of the horizon N, which could be N = ∞ in an infinite horizon. The coefficient γ is the discount rate, which determines how much more current rewards are to be preferred to future rewards. As mentioned, the value function V π is the total amount of reward an agent can expect to accumulate in the future, in a given state. Note that the value function is obviously associated with a policy: V π ( xk ) = E[R| xk , ak = π ( xk )] (1) Drones 2022, 6, 270 6 of 39 In most of the reinforcement learning applications, a very important concept is the action-value function Qπ : Qπ ( xk , ak ) = r ( xk , ak ) + γ ∑ p ( x k +1 | x k , a k ) V π ( x k +1 ) (2) x k +1 The remarkable difference between it and the value function is the fact that the action- value function tells you the expected cumulative reward at a certain state, given a certain action. The optimal policy is the one that maximizes the value function π̃ = argmaxπ V π ( xk ). In general, an important remark is that reinforcement learning was originally developed for discrete Markov decision processes. This limitation, which is not solved for many RL methods, implies the necessity of discretizing the problem into a system with a finite number of actions. This is sometimes hard to grasp in a domain in which the variables are typically continuous in space and time (think about the states or the control action) or often discretized in time for implementation. Thus, the application of reinforcement learning requires smart ways to treat the problem and dedicated recasting of the problem itself. The reinforcement learning problem has been tackled using several approaches, which can be divided into two main categories: the policy-based methods and the value-based methods. The former ones search for the policy that behaves correctly in a specific environment [17–22]; the latter ones try to value the utility of taking a particular action in a specific state of the environment [23,24]. A common categorization adopted in the literature for identifying the different methods is described below: Value-based methods: These methods seek to find optimal value function V and action- value function Q, from which the optimal policy π is directly derived. The value- based methods evaluate states and actions. Value-based methods are, for instance, Q-learning, DQN and SARSA. Policy-based methods: They are methods whose aim is to search for the optimal policy π ∗ directly, which provides a feasible framework for continuous control. The most employed policy-based methods are: advantage actor+critic, cross-entropy methods, deep deterministic policy gradient and proximal policy optimization [17–22]. An additional distinction in reinforcement learning is on-policy and off-policy. On- policy methods attempt to evaluate or improve the policy that is used to make decisions during training, whereas off-policy methods evaluate or improve a policy different from the one used to generate the data, i.e., the experience. A thorough review, beyond the scope of this paper, is necessary to survey the methods and approaches of reinforcement learning and deep reinforcement learning to space. Some very promising examples were developed in [18–20,23,24], and the most active topics in space applications are reviewed in Section 4.3. 2.4. Artificial Neural Networks Artificial neural networks represent nonlinear extensions to the linear machine learn- ing (or deep learning) models presented in Section 2. A thorough description of artificial neural networks is far beyond the scope of this work. Hereby, the set of concepts necessary to understand the work is reported. In particular, the universal approximation theory is described, which forms the foundation for all the algorithms developed in this paper. The most significant categorization of deep neural networks is into feedforward and recurrent networks. Deep feedforward networks, also often called multilayer perceptrons (MLPs), are the most common deep learning models. The feedforward network is designed to approximate a given function f. According to the task to execute, the input is mapped to an output value. For instance, for a classifier, the network N maps an input x to a category y. A feedforward network defines a mapping y = N ( x, w) and learns the values of the parameters w (weights) that result in the best function approximation. These models are called feedforward because information flows from the input layer, through the intermedi- ate ones, up to the output y. Feedback connections are not present in which outputs of the Drones 2022, 6, 270 7 of 39 model are fed back as input to the network itself. When feedforward neural networks are extended to include feedback connections, they are called recurrent neural networks. The essence of deep learning, and machine learning also, is learning world structures from data. All the algorithms falling into the aforementioned categories are data-driven. This means that, despite the possibility of exploiting an analytical representation of the environment, the algorithms need to be fed with structures of data to perform the training. The learning process can be defined as the algorithm by which the free parameters of a neural network are adapted through a process of stimulation by the environment in which it works. The type of learning is the set of instructions for how the parameters are changed, as explained in Section 2. Typically, the following sequence is followed: 1. the environment stimulates the neural network; 2. the neural network makes changes to the free parameters; 3. the neural network responds in a new way according to the new structure. As one might easily expect, there are several learning algorithms that can consequently be split into different types. It is possible to divide the supervised learning philosophy into batch and incremental learning. Batch learning is suitable for the spatial distribution of data in a stationary environment, meaning that there is no significant time correlation of data, and the environment reproduces itself identically in time. Thus, for such applications, it is possible to gather the data into a whole batch that is presented to the learner simulta- neously. Once the training has been successfully completed, the neural networks should be able to capture the underlying statistical behavior of the stationary environment. This kind of statistical memory is used to make predictions exploiting the batch dataset that was presented. This does not mean that batch learning is not capable of transferring knowledge to unseen environments or adapting to real-time applications, as shown in [18,25]. On the other hand, in several applications, the environment is non-stationary, meaning that information signals coming from the environment may vary with time. Batch learning in then inadequate, as there are no means to track and adapt to the varying environmental stimuli. Hence, for on-board learning applications, it is favorable to employ what is called incremental learning (or online or continuous learning) in which the neural network con- stantly adapts its free parameters to the incoming information in a real-time fashion, as proposed in [15,16,26–30]. 2.4.1. Universal Approximation Theorem The universal approximation theorem takes the following classical form. Let ϕ : R → R be a non-constant, bounded and continuous function (called the activation function). Let Im denote the m-dimensional unit hypercube [0, 1]m. The space of real-valued continuous functions on Im is denoted by C ( Im ). Then, given any ε > 0 and any function f ∈ C ( Im ), there exist an integer N, real constants vi , bi ∈ R and real vectors wi ∈ Rm for i = 1,... , N, such that we may define: N F(x) = ∑ vi ϕ wiT x + bi (3) i =1 As an approximate realization of the function f, | F ( x ) − f ( x )| < ε (4) For all x ∈ Im. 2.4.2. Training Algorithms The basis for most of the supervised learning algorithms is represented by back- propagation. In general, finding the weights of an artificial neural network means determin- ing the optimal set of variables that minimizes a given loss function. Given N structured Drones 2022, 6, 270 8 of 39 data, comprising input x and target t, one can define the loss function at the output of neuron j for the pth datum presented: e j ( p) = t j ( p) − y j ( p) (5) where y j ( p) is the output value of the jth output neuron. It is possible to extend this definition to derive a mean indication of the loss function for the complete output layer. We can define a total energy error of the network for the pth presented input–target pair: 1 E= 2 ∑ e2j ( p) (6) j∈C j where Cj is the set of output neurons of the network. As stated, the total energy error of the network represents the loss function to be minimized during training. Indeed, this function is dependent on all the free parameters of the network, synaptic weights and biases. In order to minimize the energy error function, we need to find those weights that vanish the derivative of the function itself and minimize the argument: w,~b) (w, b)T = argmin E (~ (7) Closed-form solutions are practically never available, thus it is common practice to use iterative algorithms that make use of the derivative of the error function to converge to the optimal value. The back-propagation algorithm is basically a smart way to compute those derivatives, which can then be employed using traditional minimization algorithms, such as [31,32]: Batch gradient descent; Stochastic gradient descent; Conjugate gradient; Newton and quasi-Newton methods; Levenberg–Marquardt; Backpropagation through time. A slightly different approach, highly tailored to the specific application, is the training through Lyapunov stability-based methods, which will be discussed for the particular application of dynamics reconstruction. Let us consider a simple method that can be applied specifically to sequential learning, in the most common network architecture, but easily extended to batch learning. With reference to Figure 2, the induced local field of neuron j, which is the input of the activation function φj (·) at neuron j, can be expressed as: v j ( p) = ∑ w ji yi ( p) + bj (8) i ∈Ci where Ci is the set of neurons that share a connection with layer j and b j is the bias term of neuron j. The output of a neuron is the result of the application of the activation function to the local field v j : y j ( p) = φj (v j ( p)) (9) In gradient-based approaches, the correction to the synaptic weights wij is performed according to the direction identified by the partial derivatives (i.e., gradient), which can be calculated according to the chain rule as: ∂E ( p) ∂E ( p) ∂e j ( p) ∂y j ( p) ∂v j ( p) = (10) ∂wij ( p) ∂e j ( p) ∂y j ( p) ∂v j ( p) ∂wij ( p) Drones 2022, 6, 270 9 of 39 Figure 2. Elementary artificial neuron architecture. Hence, the update to the synaptic weights ∆wij is calculated as a gradient descent step in the weight space using the derivative of Equation (10): ∂E ( p) ∆wij = −η (11) ∂wij ( p) where η is the tunable learning-rate parameter. The back-propagation algorithm entails two passages through the network: the forward pass and the backward pass. The former evaluates the output of the network and the function signal of each neuron. The weights are unaltered during the forward pass. The backward pass starts from the output layer by passing the loss function back to the input layer, calculating the local gradient for each neuron. 2.4.3. Incremental Learning Incremental learning stands for the process of updating the weights each time a pair of input–target (( x, t) p ) is presented. The two mentioned passes are executed at each step. This is the mode utilized for an online application where the training process can potentially never stop, as the data keep on being presented to the network. In incremental learning, often referred to online learning, the system is trained continuously as new data instances become available. They could be clustered in mini-batches or come as datum by datum. Online learning systems are tuned to set how fast they should adapt to incoming data: typically, such a parameter is referred to as learning rate. A high learning rate means that the system reacts immediately to new data, by adapting itself quickly. However, a high learning rate means that the system will also tend to forget and replace the old data. On the other hand, a low learning rate makes the system more stiff, meaning that it will learn more slowly. Additionally, the system will be less sensitive to noise present in the new data or to mini-batches containing non-representative data points, such as outliers. In addition, Lyapunov-based methods are very suitable for incremental learning due to their inherent step-wise trajectory evaluation of the stability of the learning rule. Two examples of incremental learning system are shown in Figures 3 and 4. Figure 3. Schematics of online incremental learning for spacecraft guidance. Drones 2022, 6, 270 10 of 39 Figure 4. Example of incremental learning for spacecraft navigation. 2.4.4. Batch Learning Batch learning algorithms execute the weight updates only after all the input–target data are presented to the network [1,33]. One complete presentation of the training dataset is typically called an epoch. Hence, after each epoch it is possible to define an average energy error function, which replaces Equation (6) in the back-propagation algorithm: N 1 Ẽ = 2N ∑ ∑ e2j ( p) (12) p=1 j∈C j The forward and backward passes are performed after each epoch. In batch learning, the system is not capable of learning while running. The training dataset consists of all the available data. This generally takes a lot of time and computational effort, given the typical dataset sizes. For this reason, the batch learning is generally performed on the ground. The system that is trained with batch learning first learns offline and then is deployed and runs without updating itself: it just applies what it has learned. 2.4.5. Overfitting and Online Sampling In machine learning, a very common issue encountered in a wrong training process is overfitting. In general terms, overfitting refers to the behavior of a model to perform well on the training data, without generalizing correctly. Complex models, such as deep neural networks, are capable of extracting underlying patterns in the data, but if the dataset is not chosen coherently, the model will most likely form non-existing patterns, or simply patterns that are not useful for generalization [1,33]. The main causes of overfitting can be: the training dataset is too noisy; the training dataset is too small, which causes sampling noise; the training set includes uninformative features. For instance, in dynamics reconstruction, a high sampling frequency of the state and action is not beneficial for the training. Suppose the first batch of data for learning is very much localized in a given portion of space, say, R9. Several hyper-surfaces approximate the given transition between xk and xk+1. For the sake of explanation, Figure 5 demonstrates the concept in a fictitious 1D model identification. The learning data are enclosed in a restricted region; hence, several curves yield a low loss function in the back-propagation algorithm, but the model is definitely not suitable for generalization. The limitation of the dataset to a bounded and restricted region is not beneficial for identification of dynamics. Drones 2022, 6, 270 11 of 39 Especially in a preliminary learning process, this would drive the neural network to a wrong convergence point. Figure 5. 1D dataset for incremental online learning. 3. Types of Artificial Neural Networks This section provides insights on the most used architecture in the space domain. The reader is suggested to refer to [1,3] for a comprehensive outlook on the core working princi- ples of neural networks. This survey targets the application of artificial neural networks in space systems; thus, only relevant architectures, namely, those actually investigated and implemented in spacecraft GNC systems, are detailed. A summary of the most common neural network architectures is reported in Table 4. Table 4. Most popular activation functions used in MLP. 0 Activation Function Φ Φ Codomain Ref. Hyperbolic Tangent tanh x 1 − tanh x (−1, 1) [27,34] Sigmoid 1/(1 + e− x ) Φ( x )(1 − Φ( x )) (0, 1) [34,35] ReLu max(0, x ) max(0, x ) [0, ∞) [27,36,37] Signum sgn(x) 2δ0 [−1, 1] Heaviside step (sgn( x ) + 1)/2 δ0 [0, 1] Softmax e xi / ∑nj e x j Φi (δij − Φ j ) (0, 1) output 3.1. Feed-Forward Networks Feedforward neural networks (FFNN) are the oldest and most common network architecture, and they form the fundamental basis for most of the deep learning models. The term feedforward refers to the information flow that the network possesses: the network is evaluated starting from ~x to the output ~y. The network generates an acyclic graph. Two important design parameters to take into account when designing a neural network are: Depth: Typical neural networks are actually nested evaluations of different functions, commonly named input, hidden and output layers. In practical applications, low-level features of the dataset are captured by the initial layers up to high-level features learned in the subsequent layer, all the way to the output layer. Width: Each layer is generally a vector valued function. The size of this vector valued function, represented by the number of neurons, is the width of the model or layer. Feedforward networks are a conceptual stepping stone on the path to recurrent networks [1,3,4]. Drones 2022, 6, 270 12 of 39 3.1.1. Multilayer Perceptron The multilayer perceptron is the most used deep model that is developed to build an approximation of a given function f˜ [1,3,4]. The network ~y = N (~x, w ~ ) defines the mapping between input and output and learns the optimal values of the weights w ~ that yield the best function approximation. The elementary unit of the MLP is the neuron. With reference to Figure 2, the induced local field of neuron j, which is the input of the activation function φj (·) at neuron j, can be expressed as: v j ( p) = ∑ w ji yi ( p) + bj (13) i ∈Ci where Ci is the set of neurons that share a connection with layer j; b j is the bias term of neuron j. The output of a neuron is the result of the application of the activation function to the local field v j : y j ( p) = φj (v j ( p)) (14) The activation function (also known as unit function or transfer function) performs a non-linear transformation of the input state. The most common activation functions are reported in Table 5. Among the most commonly used, at least in spacecraft related applications, are the hyperbolic tangent and the ReLu unit. The softmax function is basically an indirect normalization: it maps a n-dimensional vector x into a normalized n-dimensional output vector. Hence, the output vector values represent probabilities for each of the input elements. The softmax function is often used in the final output layer of a network; therefore, it is generally different from the activation functions used in each hidden layer. For the sake of completeness, a perceptron is originally defined as a neuron that has the Heaviside function as the activation function. An example of an MLP is reported in Section 4. The MLP has been successfully applied in classification, regression and function approximations. 3.1.2. Radial-Basis Function Neural Network A radial-basis-function neural network is a single-layer shallow network whose neu- rons are Gaussian functions. This network architecture possesses a quick learning process, which makes it suitable for online dynamics identification and reconstruction. The high- lights of the mathematical expression of the RBFNN are reported here for clarity. For a ~ ∈ Rn , the components of the output vector ~γ ∈ R j of the network are: generic state input δχ m ~ )= γl (δχ ∑ wil Φi (δχ ~ ) (15) i =1 In a compact form, the output of the network can be expressed as: ~ ) = W T Φ(δχ γ(δχ ~ ) (16) where W ~ = [wil ] for i = 1,..., m, l = 1,..., j is the trained weight matrix and ~ Φ(δχ) = [Φ1 (δχ) Φ2 (δχ) · · · Φm (δχ)] T is the vector containing the output of the radial basis functions, evaluated at the current system state. The RBF network learns to designate the input to a center, and the output layer combines the outputs of the radial basis function and weight parameters to perform classification or inference. Radial basis functions are suitable for classification, function approximation and time series prediction problems. Typically, the RBF network has a simpler structure and a much faster training process with respect to MLP, due to the inherent capability of approximating nonlinear functions using shallow architecture. As one could note, the main difference in the RBFNN with respect to the MLP is that the kernel is a nonlinear function of the information flow: in other words, the actual input to the layer is the nonlinear radial function Φ(δχ) evaluated at the input Drones 2022, 6, 270 13 of 39 data δχ, most commonly Gaussian ones. The most used radial-basis functions that can be used and that are found in space applications are [15,29,39]: 2 − (r−c2) Gaussian : Φ(r ) = e 2σ 1 Φ (r ) = ( σ2 + r2 )α Linear : Φ(r ) = r Thin-plate Spline : Φ(r ) = r2 ln(r ) 1 Logistic Function : Φ(r ) = 2 )− θ 1+e ( r/σ where r is the distance from the origin, c is the center of the RBF, σ is a control parameter to tune the smoothness of the basis function and θ is a generic bias. The number of neurons is application-dependent, and it shall be selected by trading off the training time and approximation , especially for incremental learning applications. The same consideration holds for the parameters η = σ1 , which impact the shape of the Gaussian functions. A high value for η sharpens the Gaussian bell-shape, whereas a low value spreads it on the real space. On the one hand, a narrow Gaussian function increases the responsiveness of the RBF network; on the other hand, in the case of limited overlapping of the neuronal functions due to overly narrow Gaussian bells, the output of the network vanishes. Hence, ideally, the parameter η is selected based on the order of magnitude of the exponential argument in the Gaussian function. The output of the neural network hidden layer, namely, the radial functions evaluation, is normalized: ~Φnorm (δχ) = Φ(δχ) (17) ∑im=1 Φi (δχ) The classic RBF network presents an inherent localized characteristic, whereas the normalized RBF network exhibits good generalization properties, which decreases the curse of dimensionality that occurs with classic RBFNN. A schematic of a RBFNN is reported in Figure 6. Figure 6. Architecture of the RBF network. The input, hidden and output layers have J1, J2 and J3 neurons, respectively. Drones 2022, 6, 270 14 of 39 Table 5. Summary of most common ANN architecture used in space dynamics, guidance, navigation and control domain. The training types are supervised (S), unsupervised (U) and reinforcement learning (R). Network Type Architecture Training Algorithm Space Applications Dynamics approximation, MLP S/R Backpropagation value function approximation Backpropagation/ Dynamics approximation, RBFNN S/U/R Lyapunov/K-means regression, time-series clustering prediction Feedforward Dimensionality reduction, state-space modelling, data AE U Backpropagation encoding, anomaly detection Feature detection, image CNN S Backpropagation classification, vision-based navigation Backpropagation Dynamics approximation, LRNN S/R through time time-series prediction Backpropagation Dynamics approximation, NARX S/R through time time-series prediction Combinatorial Backpropagation Recurrent HNN S optimization, system through time identification Backpropagation Time-series prediction, LSTM S/R through time dynamics approximation Time-series prediction, Backpropagation GRU S/R dynamics approximation, through time anomaly detection 3.1.3. Autoencoders The autoencoder is a particular feedforward neural network trained using unsuper- vised learning. The autoencoder learns to reproduce the unit mapping from a certain information input vector ~I ∈ Rn×n to ~I itself. The topological constraint dictates that the number of neurons in the next layer must be lower than the previous one. Such a constraint forces the network to learn a description of the input vector that belongs to the lower-dimensional space of the subsequent layers without losing information. The amount of information lost while encoding a downsizing the input vector is measured by the fitting discrepancy between the input and the reconstructed vector ~I [31,32,40]. The desired lower-dimensional vector concentrating the information contained in the input vector is the layer at which the network starts growing again; see Figure 7. It is important to note that the structure of an autoencoder is exactly the same as the MLP, with the additional constraint of having the same numbers of input and output nodes. The autoencoders are widely used for unsupervised applications: typically, they are used for denoising, dimensionality reduction and data representation learning. 3.1.4. Convolutional Neural Networks Feedforward networks are of extreme importance to machine learning applications in the space domain. A specialized kind of feedforward network, often referred as a stand-alone type, is the convolutional neural network (CNN). Convolutional networks are specifically tailored for image processing; for instance, CNNs are used for object recognition, image segmentation and classification. The main reason why traditional feedforward networks are not suitable for handling images is due to the fact that one Drones 2022, 6, 270 15 of 39 image can be thought of as a large matrix array. The number of weights, or parameters, to efficiently process large two-dimensional images (or three if more image channels are involved) quickly explodes as the image resolution grows. In general, given a network of width W and depth D, the number of parameters nw for a fully connected network is nw ∼ DW 2 + W. For instance, a low resolution image ~I ∈ R32×32 has a width of W 2 , by simply unrolling the image into a 1D array: this means that nw 106. A high resolution image, e.g., ~I ∈ R1024×1024 , quickly reaches nw ∼ 1012. This shortcoming results in complex training procedures, very much subject to overfitting. The convolutional neural network paradigm stands for the idea of reducing the number of parameters starting from the main assumptions: 1. Low-level features are local; 2. Features are translationally invariant; 3. High-level features are composed of low-level features. Such assumptions allow a reduction in the number of parameters while achieving better generalization and improved scalability to large datasets. Indeed, instead of using fully connected layers, a CNN uses local connectivity between neurons; i.e., a neuron is only connected to nearby neurons in the next layer. The basic components of a convolutional neural network are: Convolutional layer: the convolutional layer is core of the CNN architecture. The convolutional layer is built up by neurons which are not connected to every single neuron from the previous layer but only to those falling inside their receptive field. Such architecture allows the network to identify low-level features in the very first hidden layer, whereas high-level features are combined and identified at later stages in the network. A neuron’s weight can be thought of as a small image, called the filter or convolutional kernel, which is the size of the receptive field. The convolutional layer mimics the convolution operation of a convolutional kernel on the input layer to produce an output layer, often called the feature map. Typically, the neurons that belong to a given convolutional layer all share the same convolutional kernel: this is referred to as parameter sharing in the literature. For this reason, the element-wise multiplication of each neuron’s weight by its receptive field is equivalent to a pure convolution in which the kernel slides across the input layer to generate the feature map. In mathematical terms, a convolutional layer, with convolutional kernel W, ~ ~ operating on the previous layer I (being either an intermediate feature map or the input image), performs the following operation: ~) f i,j = (~I ∗ W (18) where f i,j is the (i, j) position of the output feature map. Activation layer: An activation function is utilized as a decision gate that aids the learning process of intricate patterns. The selection of an appropriate activation func- tion can accelerate the learning process. The most common activation functions are the same as those used for the MLP and are presented in Table 4. Pooling layer: The objective of a pooling layer is to sub-sample the input image or the previous layer in order to reduce the computational load, the memory usage and the number of parameters, which prevents overfitting while training [11,33]. The pooling layer works exactly with the same principle of the receptive field. However, a pooling neuron has no weights; hence, it aggregates the inputs by calculating the maximum or the average within the receptive field as output. Fully-connected layer: Similarly to MLP as for traditional CNN architectures, a fully connected layer is often added right before the output layer to further capture non- linear relationships of the input features [11,32]. The same considerations discussed for MLP hold for CNN fully connected layers. An example of a CNN architecture is shown in Figure 8. Drones 2022, 6, 270 16 of 39 Figure 7. Basic autoencoder structure. Figure 8. Example CNN architecture with convolutional, max-pooling and fully connected layers. 3.2. Recurrent Neural Networks Recurrent neural networks comprise all the architectures that present at least one feed- back loop in their layer interactions. A subdivision that is seldom used is between finite and infinite impulse recurrent networks. The former is given by a directed acyclic graph (DAG) that can be unrolled in time and replaced with a feedforward neural network. The latter is a directed cyclic graph (DCG) that cannot be unrolled and replaced similarly. Recurrent neural networks have the capability of handling time-series data efficiently. The connections between neurons form a directed graph, which allows internal state memory. This enables the network to exhibit temporal dynamic behaviors. 3.2.1. Layer-Recurrent Neural Network The core of the layer-recurrent neural network (LRNN) is similar to that of the standard MLP. This means that the same considerations for model depth, width and activation functions hold in the same manner. The only addition is that in the LRNN, there is a feedback loop with a single delay around each layer of the network, except for the last layer. A schematic of the LRNN is sketched in Figure 9. 3.2.2. Nonlinear Autoregressive Exogenous Model The nonlinear autoregressive exogenous model is an extension of the LRNN that uses the feedback coming from the output layer. The LRNN owns dynamics only at the input layer. The nonlinear autoregressive network with exogenous inputs (NARX) is a recurrent dynamic network with feedback connections enclosing several layers of the network. The NARX model is based on the linear ARX model, which is commonly used in time-series modeling. The defining equation for the NARX model is y k = N ( y k −1 , y k −2 ,... , y k − n , u k −1 , u k −2 ,... , u k − n ) (19) Drones 2022, 6, 270 17 of 39 where y is the network output and u is the exogenous input, as shown in Figure 10. Basically, it means that the next value of the dependent output signal y is regressed on previous values of the output signal and previous values of an independent (exogenous) input signal. It is important to remark that, for a one tap-delay NARX, the defining equation takes the form of an autonomous dynamical system. Figure 9. Schematic of a layer-recurrent neural network. The feedback loop is a tap-delayed signal rout. Figure 10. Schematic of a nonlinear autoregressive exogenous model. The feedback loop is a tap- delayed signal rout. 3.2.3. Hopfield Neural Network The formulation of the network was due to Hopfield , but the formulation by Abe is reportedly the most suited for combinatorial optimization problems , which are of great interest in the space domain. For this reason, here the most recent architecture is reported. A schematic of the network architecture is shown in Figure 11. Figure 11. The Hopfield neural network structure. Drones 2022, 6, 270 18 of 39 In synthesis, the dynamics of the i-th out of N neurons is written as: N dpi dt = ∑ wij s j − bi (20) j =1 where pi is the total input of the i-th neuron; wij and bi are parameters corresponding, respec- tively, to the synaptic efficiency associated with the connection from neuron j to neuron i and the bias of the neuron i. The term si is basically the equivalent of the activation function: pi si = ci tanh (21) β where β > 0 is a user-defined coefficient, and ci is the user-defined amplitude of the activation function. The recurrent structure of the network entails the dynamics of the neurons; hence, it would be more correct to refer to p(t) and s(t) as functions of time or any other independent variable. An important property of the network, which will be further discussed in the application for parameter identification, is that the Lyapunov stability theorem can be used to guarantee its stability. Indeed, since a Lyapunov function exists, the only possible long-term behavior of the neurons is to asymptotically approach a point that belongs to the set of fixed points, meaning where ddtV = 0, V being the Lyapunov function of the system, in the form: 1 N N N 1 V=− ∑ ∑ 2 i =1 j =1 wij si s j + ∑ bi si = − ~s T W~s +~s T~b 2 (22) i =1 where the right-hand term is expressed in a compact form, with ~s, the vector of s neuron states, and ~b, the bias vector. A remarkable property of the network is that the trajectories always remain within the hypercube [−ci , ci ] as long as the initial values belong to the hypercube too [44,45]. For implementation purposes, the discrete version of the HNN is employed, as was done in [44,46]. 3.2.4. Long Short-Term Memory The long-short term memory network is a type of recurrent neural network widely used for making predictions based on times series data. LSTM, first proposed by Hochre- iter , is a powerful extension of the standard RNN architecture because it solves the issue of vanishing gradients, which often occur in network training. In general, the repeating module in a standard RNN contains a single layer. This means that if the RNN is unrolled, you can replicate the recurrent architecture by juxtaposing a single layer of nuclei. LSTMs can also be unrolled, but the repeating module owns four interacting layers or gates. The basic LSTM architecture is shown in Figure 12. The core idea is that the cell state lets the information flow: it is modified by the three gates, composed of a sigmoid neural net layer and a point-wise multiplication operation. The sigmoid layer of each gate outputs a value ∈ [0, 1] that defines how much of the core information is let through. The basic components of the LSTM network are summarized here: Cell state (C): The cell state is the core element. It conveys information through different time steps. It is modified by linear interactions with the gates. Forget gate ( f ): The forget gate is used to decide which information to let through. It looks at the input xk and output of the previous step yk−1 and yields a number ∈ [0, 1] for each element of the cell state. In compact form: f = σ (W f · [yk−1 , xk ] + ~b f ] (23) Input gate (i): The input gate is used to decide what piece of information to include in the cell state. The sigmoid layer is used to decide on which value to update, whereas Drones 2022, 6, 270 19 of 39 the tanh describes the entities for modification, namely, the values. It then generates a new estimate for the cell state C̃: i = σ (Wi · [yk−1 , xk ] + ~bi ) (24) C̃k = tanh(Wc · [yk−1 , xk ] + ~bc ) Memory gate: The memory gate multiplies the old cell state with the output of the forget gate and adds it to the output of the input gate. Often, the memory gate is not reported as a stand-alone gate, due to the fact that it represents a modification of the cell state itself, without a proper sigmoid layer: Ck = f Ck−1 + i C̃k (25) Output gate: The output gate is the final step that delivers the actual output of the network yk , a filtered version of the cell state. The layer operations read: o = σ (Wo · [yk−1 , xk ] + ~bo ) (26) yk = o tanh Ck Figure 12. The core components of the LSTM are the cell (C), the input gate (i), the output gate (o) and the forget gate (f ). In contrast to deep feedforward neural networks, having a recurrent architecture, LSTMs contain feedback connections. Moreover, LSTMs are well suited not only for processing single data points, such as input vectors, but efficiently and effectively handle sequences of data. For this reason, LSTMs are particularly useful for analyzing temporal series and recurrent patterns. 3.2.5. Gated Recurrent Unit The gated recurrent unit (GRU) was proposed by Cho to make each recurrent unit adaptively capture dependencies of different time scales. Similarly to the LSTM unit, the GRU has gating units that modulate the flow of information inside the unit, but without having a separate memory cells [48,49]. The basic components of GRU share similarities with LSTM. Traditionally, different names are used to identify the gates: Update gate (u): The update gate defines how much the unit updates its value or content. It is a simple layer that performs: u = σ (Wu · [yk−1 , xk ] + ~bu ) (27) Drones 2022, 6, 270 20 of 39 Reset gate r: The reset gate effectively makes the unit process the input sequence, allowing it to forget the previously computed state: r = σ (Wr · [yk−1 , xk ] + ~br ) (28) The output of the network is calculated through a two-step update, entailing a candi- date update activation ỹk calculated in the activation h layer and the output yk : ỹk = tanh Wh · [yk−1 , xk ] + ~bh (29) y k = (1 − u ) y k −1 + u ỹk A schematic of GRU network is reported in Figure 13. Figure 13. The core components of the GRU are the reset gate (r) and the update gate (u) coupled with the activation output composed of the tanh layer. 3.3. Spiking Neural Networks Spiking neural networks (SNN) are becoming increasingly interesting to the space domain due to their low-power and energy efficiency. Indeed, small satellite missions entail low-computational-power devices and in general lower system power budgets. For this reason, SNNs represent a promising candidate for the implementation of neural- based algorithms used for many machine learning applications: among those, the scene classification task is of primary importance for the space community. SNNs are the third generation of artificial neural networks (ANNs), where each neuron in the network uses discrete spikes to communicate in an event-based manner. SNNs have the potential advantage of achieving better energy efficiency than their ANN counterparts. While generally a loss of accuracy in SNN models is reported, new algorithms and training techniques can help with closing the gap in accuracy performance while keeping the low- energy profile. Spiking neural networks (SNNs) are inspired by information processing in biology. The main difference is that neurons in ANNs are mostly non-linear but continuous function evaluations that operate synchronously. On the other hand, biological neurons employ asynchronous spikes that signal the occurrence of some characteristic events by digital and temporally precise action potentials. In recent years, researchers from the domains of machine learning, computational neuroscience, neuromorphic engineering and embedded systems design have tried to bridge the gap between the big success of DNNs in AI applications and the promise of spiking neural networks (SNNs) [50–52]. The large spike sparsity and simple synaptic operations (SOPs) in the network enable SNNs to outperform ANNs in terms of energy efficiency. Nevertheless, the accuracy performance, Drones 2022, 6, 270 21 of 39 especially in complex classification tasks, is still superior for deep ANNs. In the space domain, the SNNs are at the earliest stage of research: mission designers strive to create algorithms characterized by great computational efficiency and low power applications; hence, the SNNs represent an interesting opportunity that ought to be mentioned in this review, although they are not yet applied to guidance, navigation and control applications. Finally, SNNs on neuromorphic hardware exhibit favorable properties such as low power consumption, fast inference and event-driven information processing. This makes them interesting candidates for the efficient implementation of deep neural networks particularly utilized in image classification. The most peculiar feature of SNNs is that the neurons possess temporal dynamics: typically, an electrical analogy is used to describe their behavior. Each neuron has a voltage potential that builds up depending on the input current that it receives. The input current is generally triggered by the spikes the neuron receives. A schematic of the neuron parameters can be seen in Figures 14 and 15. There are numerous neural architectures that combine these notions into a set of mathematical equations; nevertheless, the two most common alternatives are the integrate-and-fire neuron and the leaky-integrate-and-fire neuron. Figure 14. Architecture of a simple spiking neuron. Spikes are received as inputs, which are then either integrated or summed depending on the neuron model. The output spikes are generated when the internal state of the neuron reaches a given threshold. Figure 15. An illustrative schematic depicting the membrane potential reaching the threshold, which generates output spikes. Drones 2022, 6, 270 22 of 39 3.3.1. Types of Neurons Integrate and fire (IF): The IF neuron model assumes that spike initiation is governed by a voltage threshold. When the synaptic membrane reaches and exceeds a certain threshold, the neuron fires a spike and the membrane is set back to the resting voltage Vrest. In mathematical terms, its simplest form reads: dV (t) C = i (t) (30) dt Leaky integrate and fire (LIF): The LIF neuron is a slightly modified version of the IF neuron model. Indeed, it entails an exponential decrease in membrane potential when not excited. The membrane charges and discharges exponentially in response to injected current. The differential equation governing such behavior can be written as: dV (t) C + λV (t) = i (t) (31) dt where λ is the leak conductance and V is again the membrane potential with respect to the rest value. As mentioned, the list is not extensive, and the reader is suggested to refer to for a comprehensive review of neuron models. 3.3.2. Coding Schemes The transition between dense data and sparse spiking patterns requires a coding mechanism for input coding and output decoding. For what concerns the input coding, the data can be transformed from dense to sparse spikes in different ways, among which the most used are: Rate coding: it converts the input intensity into a firing rate or spike count; Temporal (or latency) coding: it converts the input intensity to a spike time or relative spike time. Similarly, in output decoding, the data can be transformed from sparse spikes to network output (such as classification class) in different ways, among which the most used are: Rate coding: it selects the output neuron with the highest firing rate, or spike count, as the predicted class; Temporal (or latency) coding: it selects the output neuron that fires first, or before a given threshold time, as the predicted class Roughly speaking, the current literature agrees on specific advantages for both the coding techniques. On one hand, the rate coding is more error tolerant given the re- duced sparsity of the neuron activation. Moreover, the accuracy and learning convergence have shown superior results in rate-based applications so far. On the other hand, given the inherent sparsity of the encoding-decoding scheme, latency-based approaches tend to outperform the rate-based architectures in inference, training speed and, above all, power consumption. 4. Applications in Space This section provides an overview of the space domain tasks that are currently being investigated by the research community. The paper highlights the characteristics that are peculiar to GNC algorithms, referencing other domains’ literature when needed. In addition, a short paragraph on the challenges of dataset availability and data validation is presented. Drones 2022, 6, 270 23 of 39 4.1. Identification of Neural Spacecraft Dynamics The capability of using an ANN to approximate the underlying dynamics of a space- craft is used to enhance the on-board model accuracy and flexibility to provide the space- craft with a higher degree of autonomy. There are different approaches in the literature that could be adopted to tackle the system identification and dynamics reconstruction task, as show in Figure 16. In the following section, the three analyzed methods are described; recall the universal approximation theorem reported in Section 2. Dynamics Reconstruction Figure 16. Identification of system dynamics: different approaches to reconstructing system dynami- cal behavior. 4.1.1. Fully Neural Dynamics Learning The dynamical model of a system delivers the derivative of the system state, given the actual system state and external input. Such an input–output structure can be fully approx- imated by an artificial neural network model. The dynamics are entirely encapsulated in the weights and biases of the network N. The neural network is stimulated by the actual state and the external output. In turn, the time derivative of the state, or simply the system state at the next discretization step, is yielded as output, as shown in Figure 17: ~ẋ = N (~x, ~u) → ~xk+1 = Ñ (~xk , ~uk ) (32) System State Prediction Control Input Input Layer ∈ ℝ Hidden Layer ∈ ℝ¹² Hidden Layer ∈ ℝ¹ Output Layer ∈ ℝ Figure 17. Dynamical reconstruction as a neural network model. The method relies on the universal approximation theorem, since it is based on the assumption that there exists an ANN that approximates the dynamical function with a predefined approximation error. The training set is simply composed of input–output pairs, where the input is a stacked vector of system states and control vectors. The result is that the full dynamics is encapsulated into a neural network model that can be used to generate prediction of future states [16,54]. The dynamics reconstruction Drones 2022, 6, 270 24 of 39 based solely on artificial neural networks largely benefits by the employment of recurrent neural networks, rather than simpler feedforward networks. Indeed, literature, although rather poor, employ Recurrent Neural Networks to perform the task [25,27,54]. The re- current architecture owns an inherently more complex structure but maintains a brief evaluation time make it a suitable architecture for on-board applications. As mentioned, Recurrent Networks have the capability of handling time-series data efficiently because the connections between neurons form a directed graph, which allows an internal state mem- ory. This enables the network to exhibit temporal dynamic behaviors. When dealin