Decision Making 4.pdf
Document Details
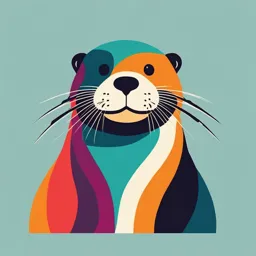
Uploaded by PleasedQuartz
Tags
Full Transcript
Decision-Making Lecture 4 MODERN APPROACHES Acknowledgement of Country Lecture outline Focus questions Lecture sections What are Bayesian models of Bayesian models of decision-making decision-making, and how do they...
Decision-Making Lecture 4 MODERN APPROACHES Acknowledgement of Country Lecture outline Focus questions Lecture sections What are Bayesian models of Bayesian models of decision-making decision-making, and how do they improve on the 'fast-and-frugal' approach? What is machine learning, and what Machine learning and decision-making are the ethical limitations around using it to guide decisions? What is metacognition, and what Metacognition does it tell us about the relationship between confidence and accuracy in decisions? The University of Adelaide Slide 3 Readings for this lecture The University of Adelaide Slide 4 Bayesian models of decision- making Bayes’ rule Bayesian statistics: Mixing evidence with priors to obtain posteriors A cab was involved in a hit-and-run accident at night. Two cab companies, the Green and the Blue, operate in the city. You are given the following data: 85% of the cabs in the city are Green and 15% are Blue. A witness identified the cab as Blue. The court tested the reliability of the witness under the circumstances that existed on the night of the accident and concluded that the witness correctly identified each one of the two colours 80% of the time and failed 20% of the time. What is the probability that the cab involved in the accident was Blue rather than Green? Bayesian statistics: Mixing evidence with priors to obtain posteriors A cab was involved in a hit-and-run accident at night. Two cab companies, the Green and the Blue, operate in the city. You are given the following data: 85% of the cabs in the city are Green and 15% are Blue. A witness identified the cab as Blue. The court tested the reliability of the witness under the circumstances that existed on the night of the accident and concluded that the witness correctly identified each one of the two colours 80% of the time and failed 20% of the time. What is the probability that the cab involved in the accident was Blue rather than Green? Posterior probability that the taxi was Blue: 41% Bayes’ rule: Solving the taxi cab problem Probability that the cab involved in the accident Hypothesis was Blue, given that the ood Prio lih r Evidence witness identified it as Like Blue r Posterio Marginal likelihood What is the probability that the cab involved in the accident was Blue, given that the witness identified it as Blue? Bayes’ rule: Solving the taxi cab problem lihood Prio Like r r Posterio Marginal likelihood = P(taxi is blue) Bayes’ rule: Solving the taxi cab problem lihood Prio Like r r Posterio Marginal likelihood = P(taxi is blue).15 Bayes’ rule: Solving the taxi cab problem lihood Prio Like r r Posterio Marginal likelihood = P(witness says that the taxi is blue | taxi is blue) = P(taxi is blue).15 Bayes’ rule: Solving the taxi cab problem lihood Prio Like r r Posterio Marginal likelihood = P(witness says that the taxi is blue | taxi is blue).80 = P(taxi is blue).15 Bayes’ rule: Solving the taxi cab problem lihood Prio Like r r Posterio Marginal likelihood is the observer’s “hypothesis space” – the set of all hypotheses considered by the observer. Bayes’ rule: Solving the taxi cab problem lihood Prio Like r r Posterio Marginal likelihood is the observer’s “hypothesis space” – the set of all hypotheses considered by the observer. = P(witness says that the taxi is blue | taxi is blue) ⦁ P(taxi is blue) + P(witness says that the taxi is blue | taxi is not blue) ⦁ P(taxi is not blue) Bayes’ rule: Solving the taxi cab problem lihood Prio Like r r Posterio Marginal likelihood is the observer’s “hypothesis space” – the set of all hypotheses considered by the observer..80.15 = P(witness says that the taxi is blue | taxi is blue) ⦁ P(taxi is blue) + P(witness says that the taxi is blue | taxi is not blue) ⦁ P(taxi is not blue).20.85 Bayes’ rule: Solving the taxi cab problem Probability that the cab lihood Prio involved in the accident was Like r Blue, given that the witness identified it as Blue r Posterio Marginal likelihood.80 ⦁.15 (.80 ⦁.15) + (.20 ⦁.85).41 Bayes’ rule: Solving the taxi cab problem Probability that the cab lihood Prio involved in the accident was Like r Blue, given that the witness identified it as Blue r Posterio Marginal likelihood.80 ⦁.15 (.80 ⦁.15) + (.20 ⦁.85) Kahneman and Tversky found.41 that most people’s estimates are higher than this, and concluded that people have a “base rate neglect” bias Bayes’ rule: Solving the taxi cab problem lihood Prio Like r r Posterio Marginal likelihood Scientific common sense lihood Prio Like r r Posterio lihood Prio Like r r Posterio Bayes’ rule: Summary of uses Covered so far Method of deriving rational answers to toy problems; e.g., correct answer to the taxicab problem Bayes’ rule: Summary of uses Covered so far Method of deriving rational answers to toy problems; e.g., correct answer to the taxicab problem Alternative to frequentist statistics for data analysis Bayes’ rule: Summary of uses Covered so far Method of deriving rational answers to toy problems; e.g., correct answer to the taxicab problem Alternative to frequentist statistics for data analysis Method of deriving rational answers to more complex problems Bayes’ rule: Summary of uses Covered so far Method of deriving rational answers to toy problems; e.g., correct answer to the taxicab problem Alternative to frequentist statistics for data analysis Method of deriving rational answers to more complex problems Not covered yet Basis for approaching decision-making (and human perception and cognition more broadly) as a task that involves applying scientific common sense Bayes’ rule: Summary of uses Covered so far Method of deriving rational answers to toy problems; e.g., correct answer to the taxicab problem Alternative to frequentist statistics for data analysis Method of deriving rational answers to more complex Artificial intelligence problems Not covered yet Basis for approaching decision-making (and human perception Bayesian models and cognition more broadly) as a task that involves applying of cognition, scientific common sense including decision-making Both make use of recent Bayes’ rule: Summary of uses innovations in mathematical computing: Markov Chain Monte Covered so far Carlo Graphical models/ Method of deriving rational answers to toy problems; hierarchical Bayesian e.g., correct answer to the taxicab problem models Alternative to frequentist statistics for data analysis Method of deriving rational answers to more complex Artificial intelligence problems Not covered yet Basis for approaching decision-making (and human perception Bayesian models and cognition more broadly) as a task that involves applying of cognition, scientific common sense including decision-making A Bayesian model of decision-making in the German cities problem van Ravenzwaaij et al. (2014) Experiment 2 Graphical model: Search-and-stop model Take-the-best (TTB) and weighted tallying are special cases; that is, cases for which parameter zA takes the value of 1 (TTB) or 0 (weight. tally). Versions of the TTB searching by cue validity only (TTBv) or cue discriminability only (TTBd) were also implemented. Each individual’s search order is determined by a weighting of cue validity v and cue discriminability d for each cue (zW). Participants either terminate their search after encountering the first discriminating cue if zA = 1, or examine all cues if zA = 0. zA cannot take any other values. When the participant stops searching, their response is tij = 1 if the cue points at city aj and their response is tij = 0 if the cue points at city bj. Across all questions in the German cities task, the Bayesian model just shown can act as any one of the following decision-makers: A decision-maker who implements a 1 search-and-stop version of Take-the-Best, ranking cues not only in order of validity but also discriminability. The search-and- stop strategy also permits the consideration of all cues rather than a limited number on some questions. Effectively, on some questions, the search- and-stop strategy permits weighted tallying (a rational strategy from Lecture 3). A decision-maker who follows a 2 version of Take-the-Best in which cue order is determined in the same way as for the search-and- stop version (taking into account both validity and discriminability), but without an option to apply weighted tallying on some questions. A decision-maker who follows the 3 version of Take-the-Best originally proposed by Gigerenzer and colleagues, in which cue search order is determined by cue validity only. A decision-maker who follows a 4 version of Take-the-Best in which cue search order is determined only by cue discriminability. A decision-maker who follows a 5 rational strategy (weighted tallying). Results When comparing the model predictions on each trial to the actual answer given by each participant… Results When comparing the model predictions on each trial to the actual answer given by each participant… Search-and-stop model had the highest level of correspondence: 89.5%. Results When comparing the model predictions on each trial to the actual answer given by each participant… Search-and-stop model had the highest level of correspondence: 89.5%. This finding suggests that strategies adopted in the German cities problem differ across individuals and questions. People apply Take- the-Best, but take more than cue validity into account. In some questions they consider all the available cues. Bayesian models have the flexibility to express strategies that vary across people and questions, and it seems that this flexibility is needed to describe human decision-making, even in toy problems. Bayesian models that do not rely on graphical models Not all Bayesian models of decision-making rely on graphical models. The Bayesian model described in the first reading assumes that people simply apply Bayes’ rule involving distributions when predicting durations and quantities; for example, when asked the following question: Insurance agencies employ actuaries to make predictions about people’s life spans – the age at which they will die – based upon demographic information. If you were assessing an insurance case for an 18-year-old man, what would you predict for his life span? Griffiths & Tenenbaum (2006) Bayesian models that do not rely on graphical models A similar set of assumptions is made by a Bayesian model that seeks to explain why it is rational for people to adjust insufficiently from provided anchors – especially when pepole know little about the subject. Lieder et al. (2018) Lieder et al. (2018) Anchor type Self-generated (56 people) Experimenter-provided (51 people) 4 questions: 4 questions: In what year was George Washington Is the population of Chicago lower or elected President of the United States? higher than 200,000? What is it? ____ In what year did the second European explorer land in the West Indies? Is the height of the tallest redwood tree lower or higher than 65 feet? What is it? What is the freezing point of vodka? ____ What is the boiling point of water on the top of Mount Everest? Is the length of the Mississippi River lower or higher than 2,000 miles? What is it? ____ After all questions were answered, Is the height of Mount Everest lower or participants were asked to indicate whether they knew of a particular relevant value for higher than 45,500 feet? What is it? ____ each question (e.g., for the first question, it was expected that many participants would cite the year of declaration of independence in the US: 1776) Epley & Gilovich, 2005, Study 1 The University of Adelaide Epley & Gilovich, 2005, Study 1 Machine learning Image source Image source Image source Ethical issues “With the dawn of artificial intelligence (AI), a slew of new machine learning tools promise to help protect us—quickly and precisely tracking those who may commit a crime before it happens—through data… The tools themselves, however, present a problem: The data being used to “teach” the software systems is embedded with bias, and only serves to reinforce inequality. Here’s how: Black people are more likely than white people to be reported for a crime—whether the reporter is white or Black. This leads to Black neighborhoods being marked as “high risk” at a disproportionate rate.” Reese, 2022 The University of Adelaide Slide 48 Metacognition Another response to the heuristics and biases perspective Center for the Study of Intelligence (2009) The University of Adelaide Slide 50 Two contributing processes The University of Adelaide Slide 51 A driving force of information processing In research on metacognition, rational cognitive and memory performance is assumed to depend critically on the effectiveness of monitoring and control. As Koriat (2016) summarises: The University of Adelaide Slide 52 Is confidence a valid indicator of accuracy? If two friends give you conflicting advice (e.g., regarding which brand to buy), going with the advice of the more confident friend relies on the assumption that we can meaningfully compare the confidence of two different people. This is the assumption that two friends are ‘well-calibrated’ relative to each other. The University of Adelaide Slide 53 Jin et al., 2022 A caveat: The Dunning-Kruger Effect Score in an exam Dunning, 2011 The University of Adelaide Slide 55 In explaining the effect, Kruger and Dunning argued that the lowest performers in a task suffer a dual burden: not only is their performance poor, but they also have a corresponding metacognitive (monitoring) deficit that impedes their ability to distinguish accurate from inaccurate performance. Unable to discern their own errors, poor performers assume they make fewer errors than, in fact, they do, resulting in overestimation of performance. Recently, McIntosh et al. (2019) demonstrated that the Dunning-Kruger effect is not due to differences in metacognitive ability between high and low performers. Instead: monitoring ability correlates with task performance (providing evidence for metacognition as a driving force of performance), and the Dunning-Kruger effect is due to poorer performers making more errors about which they can been mistaken (in considering them successes) in a task without immediate feedback on errors. The University of Adelaide Slide 57 Summary The confidence- accuracy relationship Monitoring Control Prospect Theory Metacognition (1979) Fast and Heuristics Bayesian models frugal Machine and biases of cognition (1974) heuristics (1994) learning (1991) Bayesian mathematical computing 60 References Bergert, F. B., & Nosofsky, R. M. (2007). A response-time approach to comparing generalized rational and take-the-best models of decision making. Journal of Experimental Psychology. Learning, Memory, and Cognition, 33, 107–129. Dunning, D. (2011). The Dunning–Kruger Effect: On being ignorant of one’s own ignorance. In J. M. Olson & M. P. Zanna (Eds.), Advances in Experimental Social Psychology (Vol. 44, pp. 247–296). Academic Press. Epley, N., & Gilovich, T. (2005). When effortful thinking influences judgmental anchoring: differential effects of forewarning and incentives on self-generated and externally provided anchors. Journal of Behavioral Decision Making, 18(3), 199–212. Koriat, A. (2016). Metacognition: Decision-making processes in self-monitoring and self-regulation. In G. Keren, & G. Wu (Eds.), The Wiley Blackwell handbook of judgment and decision making (Vol 1, pp. 356-379). Wiley–Blackwell. Kruger, J., & Dunning, D. (1999). Unskilled and unaware of it: How difficulties in recognizing one’s own incompetence lead to inflated self-assessments. Journal of Personality and Social Psychology, 77(6), 1121–1134. Lieder, F., Griffiths, T. L., M Huys, Q. J., & Goodman, N. D. (2018). The anchoring bias reflects rational use of cognitive resources. Psychonomic Bulletin & Review, 25(1), 322–349. McIntosh, R. D., Fowler, E. A., Lyu, T., & Della Sala, S. (2019). Wise up: Clarifying the role of metacognition in the Dunning- Kruger effect. Journal of Experimental Psychology. General, 148(11), 1882–1897. van Ravenzwaaij, D., Moore, C. P., Lee, M. D. & Newell B. R. (2014). A hierarchical Bayesian modeling approach to searching and stopping in multi-attribute judgment. Cognitive Science, 38, 1384–1405. The University of Adelaide Slide 61