Customer Lifetime Value (CLV) Lecture 3 PDF
Document Details
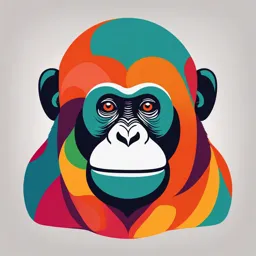
Uploaded by AstonishedCliff
Cavite State University - Imus Campus
Tags
Summary
This lecture details the concept of customer lifetime value (CLV) and explores its key components, including customer acquisition cost, revenue generated, retention rate, and profit margin. It also discusses predictive modeling techniques and their importance in optimizing marketing strategies, increasing retention rates, and personalizing customer interactions.
Full Transcript
RECOGNIZING CUSTOMER LIFETIME VALUE LECTURE 3 CUSTOMER ANALYTICS OFAD 131 Learning Objectives: After studying this chapter, you should be able to: 1. Explain the concept and importance of Customer Lifetime Value (CLV). 2. Identify the components of CLV and how they influen...
RECOGNIZING CUSTOMER LIFETIME VALUE LECTURE 3 CUSTOMER ANALYTICS OFAD 131 Learning Objectives: After studying this chapter, you should be able to: 1. Explain the concept and importance of Customer Lifetime Value (CLV). 2. Identify the components of CLV and how they influence marketing strategies. 3. Learn to calculate and interpret CLV to guide business decisions. 4. Explore best practices for leveraging CLV to drive customer acquisition, retention, and profitability. 5. Develop predictive models to anticipate customer behaviors and maximize CLV through cross-sell and upsell strategies. CUSTOMER ANALYTICS OFAD 131 Customer Lifetime Value (CLV) Customer Lifetime Value (CLV) is a key metric that helps businesses measure the total profit they can expect to earn from a customer over the entirety of their relationship. CUSTOMER ANALYTICS OFAD 131 Key Components of CLV 1. Customer Acquisition Cost (CAC) 2. Margin Generated by the Customer 3. Retention Rate CLV = (Average Purchase Value) × (Purchase Frequency) × (Customer Retention Period) × (Profit Margin) CUSTOMER ANALYTICS OFAD 131 Customer Lifetime Value 1. Average Purchase Value How much the customer spends in one transaction. 2. Purchase Frequency How often the customer buys from your business. 3. Customer Retention Period How long the customer stays with your business. 4. Profit Margin The percentage of revenue that remains after deducting the cost of goods sold. CUSTOMER ANALYTICS OFAD 131 Why is CLV Important? Prioritize high-value customers Optimize customer acquisition efforts Improve retention strategies Enhance customer relationships CUSTOMER ANALYTICS OFAD 131 Challenges in CLV CUSTOMER ANALYTICS OFAD 131 Using CLV to Drive Strategy Target Campaigns Drive Acquisition Retention Strategies In-bound Sales Lead Qualification CUSTOMER ANALYTICS OFAD 131 Best Practices for Maximizing CLV Determine Acquisition Allowance Predict the Best Ways to Win Customers Grow Relationships with Predictive Intelligence Retain Customers for Longer CUSTOMER ANALYTICS OFAD 131 Predictive Modeling in Customer Lifetime Value (CLV) Predictive modeling is a powerful data analysis technique that helps businesses anticipate customer behaviors and make informed decisions about future marketing and sales strategies. It allows companies to forecast the future value of a customer, helping them determine which customers to focus on, how much to spend on acquiring new customers, and how to retain existing ones. CUSTOMER ANALYTICS OFAD 131 Predictive Modeling in Customer Lifetime Value (CLV) Predictive modeling uses historical data, such as past customer interactions, purchase patterns, and engagement history, to build models that predict future outcomes. CUSTOMER ANALYTICS OFAD 131 Importance of Predictive Modeling in CLV Optimize marketing strategies Increase retention rates Personalize customer interactions CUSTOMER ANALYTICS OFAD 131 Steps in Predictive Modeling for CLV 1. Choose Data Sources 2. Prepare the Data 3. Explore the Data 4. Apply Algorithms CUSTOMER ANALYTICS OFAD 131 An algorithm is a set of heuristics and calculations that creates a data mining model from data. That model is then used to predict what a customer is likely to do. o Decision Trees - Predict outcomes by creating a tree-like structure of possible decisions based on customer data. Decision trees can help businesses identify key factors influencing a customer's likelihood to make a purchase. o Clustering - Groups customers with similar behaviors together. This method helps identify customer segments, such as frequent buyers or price-sensitive customers, allowing businesses to target each group with different strategies. o Regression Analysis - Examines the relationship between variables to predict future outcomes. In CLV analysis, regression can help predict how changes in factors like engagement or retention efforts may impact future customer value. CUSTOMER ANALYTICS OFAD 131 Steps in Predictive Modeling for CLV 5. Extract Rules and Patterns 6. Use Predictive Models to Take Action CUSTOMER ANALYTICS OFAD 131 Applications of Predictive Modeling in CLV 1. Cross-Sell and Upsell Opportunities One of the most practical applications of predictive modeling in CLV is identifying opportunities for cross- selling (suggesting complementary products) and upselling (offering premium options). By understanding customer preferences and purchase patterns, businesses can offer products at the right time to increase the overall value of each customer. CUSTOMER ANALYTICS OFAD 131 Applications of Predictive Modeling in CLV 2. Retention and Loyalty Programs 3. Personalizing Customer Journeys CUSTOMER ANALYTICS OFAD 131 Benefits of Predictive Modeling in CLV More accurate forecasting Optimized marketing spend Enhanced customer experience Proactive problem-solving CUSTOMER ANALYTICS OFAD 131 Computing the CLV You own a small coffee shop where a customer spends ₱200 per visit, visits 2 times per week, and stays loyal for 2 years. Your profit margin is 50%. CUSTOMER ANALYTICS OFAD 131 Predictive Modelling A coffee shop wants to predict customer purchases for the next month based on past purchase behavior. The shop has the following data for a sample of customers: Total Visits (Last Average Spend Customer ID Preferred Drink Month) per Visit (₱) 1 10 150 Espresso 2 5 200 Latte 3 8 100 Cappuccino 4 12 250 Americano 5 7 180 Espresso CUSTOMER ANALYTICS OFAD 131 Goal: Predict the total spend for each customer in the next month based on their past purchasing behavior. Assumption: Total Visits and Average Spend per Visit will remain relatively stable over the next month. CUSTOMER ANALYTICS OFAD 131 FINAL WORD "Obstacles don't have to stop you. If you run into a wall, don't turn around and give up. Figure out how to climb it, go through it, or work around it." -Michael Jordan CUSTOMER ANALYTICS OFAD 131