CS826_Transfer Learning.pdf
Document Details
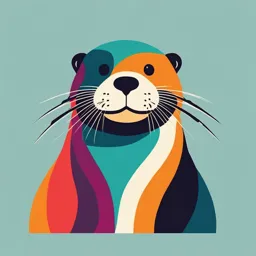
Uploaded by TopQualityBlessing7037
University of Strathclyde
Related
- A Concise History of the World: A New World of Connections (1500-1800)
- Lg 5 International Environmental Laws, Treaties, Protocols, and Conventions
- Ziraat Finans Grubu Uygulamaları
- Psychoanalytic Diagnosis: Understanding Personality Structure
- Dynamics of the Cell Membrane PDF - Istanbul Atlas University
- Health Education Chapter 1 Notes PDF
Full Transcript
CS826 Deep Learning Theory and Practice Transfer Learning Edited by Nur Naim, Oct 2021 Transfer learning Transductive vs Inductive Transfer Learning Pre-training Freezing and Fine-tuning Pre-trained Network Transfer learning approach for Natural Language Processing (NLP) Deep...
CS826 Deep Learning Theory and Practice Transfer Learning Edited by Nur Naim, Oct 2021 Transfer learning Transductive vs Inductive Transfer Learning Pre-training Freezing and Fine-tuning Pre-trained Network Transfer learning approach for Natural Language Processing (NLP) Deep learning methods are data-hungry >50K data items needed for training The distributions of the source and target data must be the same Labeled data in the target domain may be limited This problem is typically addressed with transfer learning training test (labeled) (unlabeled) Classifier 85.5 % New York New York Times Times Ack. From Jing Jiang’s slides training test (labeled) (unlabeled) Classifier 64.1 % Labeled data not available! New York Times Reuters Ack. From Jing Jiang’s slides train test ideal setting NYT NYT 85.5% Classifier New York Times New York Times realistic setting Reuters NYT 64.1 Classifier % Reuters New York Times Ack. From Jing Jiang’s slides Multi-task learning Learn several related tasks at the same time with shared representations Single P(x) but multiple output variables Transfer learning Two stage domain adaptation: select generalizable features from training domains and specific features from test domain 10/49 Inductive Adapt existing supervised training model on new labeled dataset Regression Transductive Adapt existing supervised training model on new unlabeled dataset Classification, Regression Unsupervised Adapt existing unsupervised training model on new unlabeled dataset Clustering, Dimensionality Reduction Transductive transfer No labeled target domain data available Focus of most transfer research in NLP (Natural Language Processing) E.g. Domain adaptation Inductive transfer Labeled target domain data available Goal: improve performance on the target task by training on other task(s) Jointly training on >1 task (multi-task learning) Pre-training (e.g. word embeddings) Labeled Data Model Test set Unlabeled Data Transductive Applications and problems – Labeled examples are scarce but unlabeled data are abundant – Web page classification, review ratings prediction Self-training Give labels to unlabeled data Generative models Unlabeled data help get better estimates of the parameters Transductive SVM Maximize the unlabeled data margin Graph-based algorithms Construct a graph based on labeled and unlabeled data, propagate labels along the paths Distance learning Map the data into a different feature space where they could be better separated Improvement of learning in a new task through the transfer of knowledge from a related task that has already been learned. Weight initialization for CNN Two major strategies ConvNet as fixed feature extractor Fine-tuning the ConvNet Lots of data, time, resources needed to train and tune a neural network from scratch An ImageNet deep neural net can take weeks to train and fine-tune from scratch. Unless you have 256 GPUs, possible to achieve in 1 hour Cheaper, faster way of adapting a neural network by exploiting their generalization properties Learning and Transferring Mid-Level Image Representations using Convolutional Neural Networks [Oquab et al. CVPR 2014] The ImageNet dataset was created by a group of professors and researchers at Princeton, Stanford, and UNC Chapel Hill. ImageNet was originally formed with the goal of populating the WordNet hierarchy with roughly 500-1000 images per concept. Images for each concept were gathered by querying search engines and passing candidate images through a validation step on Amazon Mechanical Turk. The ImageNet Large Scale Visual Recognition Challenge (ILSVRC) is the most commonly used subset of the ImageNet dataset. Within this subset: ~ImageNet contains 1,281,167 training images ~ImageNet contains 50,000 validation images ~ImageNet contains 100,000 test images ~ImageNet contains 1000 object classes New dataset is small and similar to original dataset. train a linear classifier on the CNN codes New dataset is large and similar to the original dataset fine-tune through the full network New dataset is small but very different from the original dataset SVM/softmax classifier from activations somewhere earlier in the network New dataset is large and very different from the original dataset fine-tune through the entire network Overly aggressive fine-tuning causes catastrophic forgetting Too cautious fine-tuning leads to slow convergence and overfitting Proposed approach: gradual unfreezing First unfreeze the last layer and fine-tune the unfrozen layer for one epoch Then unfreeze the next lower frozen layer and fine-tune all unfrozen layers Repeat until we fine-tune all layers until convergence in the last iteration The combination of discriminative fine-tuning, skewed triangular learning rates and gradual unfreezing leads to best performance Pre-Trained Models for Image Classification VGG-16 ResNet50 Inceptionv3 EfficientNet The VGG-16 is one of the most popular pre-trained models for image classification. Introduced in the famous ILSVRC 2014 Conference, it was and remains THE model to beat even today. Developed at the Visual Graphics Group at the University of Oxford, VGG-16 beat the standard of AlexNet and was quickly adopted by researchers and the industry for their image Classification Tasks. A good transfer learning strategy is outlined as following steps: Freezing the lower ConvNet blocks (blue) as fixed feature extractor. Take a ConvNet pretrained on ImageNet, remove the last fully-connected layers, then treat the rest of the ConvNet as a fixed feature extractor for the new dataset. In an VGG16 network, this would compute a 4096-D vector for every image that contains the activations of the hidden layer immediately before the classifier. These features are termed as CNN codes. Training the new fully-connected layers (green, aka. bottleneck layers). Extract the CNN codes for all images, train a linear classifier (e.g. Linear SVM or Softmax classifier) for the new dataset. Fine-tuning the ConvNet. Replace and retrain the classifier on top of the ConvNet on the new dataset, but to also fine-tune the weights of the pre-trained network by continuing the back-propagation to part of the higher layers (yellow+green). Spam filtering Public email collection personal inboxes Intrusion detection Existing types of intrusions unknown types of intrusions Sentiment analysis Expert review articles blog review articles The aim To design learning methods that are aware of the training and test domain difference Transfer learning Adapt the classifiers learnt from the source domain to the new domain https://filebox.ece.vt.edu/~jbhuang/teaching/ece6554/sp17/lectures/Lectu re_04_Supervised_Pretraining.pptx https://ov-research.uwaterloo.ca/MSCI641/Week10_Transfer_learning.pptx https://lisaong.github.io/mldds-courseware/03_TextImage/transfer- learning.slides.html https://towardsdatascience.com/pre-trained-language-models-simplified- b8ec80c62217 https://ruder.io/state-of-transfer-learning-in-nlp/ https://lisaong.github.io/mldds-courseware/03_TextImage/transfer- learning.slides.html Google (image) – VGG16, ImageNet, pre-trained, fine-tuning, transfer learning for NLP.