CS ELEC 3 MODULE.pdf
Document Details
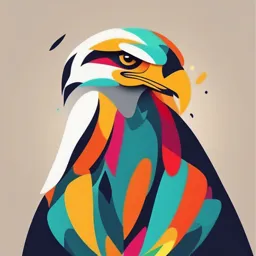
Uploaded by BestHurdyGurdy
Kolehiyo ng Lungsod ng Lipa
2024
Tags
Related
Full Transcript
Reference No: KLL-FO-ACAD-000 | Effectivity Date: August 3, 2020 | Revisions No.: 00 VISION MISSION A center of human de...
Reference No: KLL-FO-ACAD-000 | Effectivity Date: August 3, 2020 | Revisions No.: 00 VISION MISSION A center of human development committed to the pursuit of wisdom, truth, Establish and maintain an academic environment promoting the pursuit of justice, pride, dignity, and local/global competitiveness via a quality but excellence and the total development of its students as human beings, affordable education for all qualified clients. with fear of God and love of country and fellowmen. GOALS Kolehiyo ng Lungsod ng Lipa aims to: 1. foster the spiritual, intellectual, social, moral, and creative life of its client via affordable but quality tertiary education; 2. provide the clients with reach and substantial, relevant, wide range of academic disciplines, expose them to varied curricular and co-curricular experiences which nurture and enhance their personal dedications and commitments to social, moral, cultural, and economic transformations. 3. work with the government and the community and the pursuit of achieving national developmental goals; and 4. develop deserving and qualified clients with different skills of life existence and prepare them for local and global competitiveness Learning Module in CS Elec 3 Intelligent Systems Name: ______________________________________________________ (Last name, First Name MI.) Course, Year & Section: ________________________________________ Address: ____________________________________________________ Marawoy, Lipa City, Batangas 4217 | https://www.facebook.com/KLLOfficial/ MODULE 1 FIRST Semester, AY 2024-2025 I. COURSE CODE/TITLE: CS Elec 3 – Intelligent Systems II. SUBJECT MATTER: TOPICS TIME - FRAME A. Introduction to Artificial Intelligence 1.5 hour 1. History of Artificial Intelligence 1.5 hour Lesson 1. Introduction to Artificial Intelligence III. COURSE OUTCOME A. Define Artificial Intelligence; Give examples of AI Machines and Software B. State the origin of Artificial Intelligences. IV. ENGAGEMENT January 2018, Google CEO Sundar Pichai claimed that artificial intelligence (AI) will be more transformative to humanity than electricity. Artificial Intelligence will save us not destroy us, Google’s CEO, Sundar Pichai, said at Davos. “AI is probably the most important thing humanity has ever worked on. I think of it as something more profound than electricity or fire,” he said. “Any time you work with technology, you need to learn to harness the benefits while minimizing the downsides.” While some thinkers – notably Professor Stephen Hawking – have warned that AI could wipe out mankind, Pichai was optimistic. He said that the technology could eliminate many of the constraints we now face, helping us for example to make “clean, cheap, reliable energy” a reality. Marawoy, Lipa City, Batangas 4217 | https://www.facebook.com/KLLOfficial/ Pichai, who grew up in India, spoke of the transformative power of technology. “Growing up, I didn’t have a phone for a while, I waited five years. We got a telephone, it fundamentally changed our lives... I remember the joys of technology and I think that will be true for AI. It’s important for us to explain that and bring the world along with us.” He conceded that the risks were “important”, and called for international cooperation on the scale of the Paris climate agreement to manage them. “Countries need to demilitarize AI, that’s a common goal countries should work towards,” he said. Google has recently announced it will open AI research centers in China and in France. Marawoy, Lipa City, Batangas 4217 | https://www.facebook.com/KLLOfficial/ LESSON 1 – Introduction to Artificial Intelligence INTRODUCTION What is Intelligence? Intelligence: “The capacity to learn and solve problems.” Artificial Intelligence: Artificial Intelligence (AI) is the simulation of human intelligence by machines. 1) The ability to solve problems. 2) The ability to act rationally. 3) The ability to act like humans. The central principles of Al include: 1) Reasoning, knowledge, planning, learning and communication. 2) Perception and the ability to move and manipulate objects. 3) It is the science and engineering of making intelligent machines, especially intelligent computer programs DEFINITION - Computers with the ability to mimic or duplicate the functions of the human brain. - Artificial Intelligence is the intelligence of machines and the branch of computer science which aims to create it. - "The branch of computer science that is concerned with the automation of intelligent behaviour" (Luger and Stubblefield. 1993). Application of Artificial Intelligence Medicine - A medical clinic can use AI systems to organize bed schedules, make a staff rotation and provide medical information. - AI has also application in fields of cardiology (CRG), neurology (MRI), embryology (sonography), complex operations of internal organs, etc - It also has an application in Image guided surgery and image analysis and enhancement. Marawoy, Lipa City, Batangas 4217 | https://www.facebook.com/KLLOfficial/ Music - Scientists are trying to make the computer emulate the activities of the skillful musician. - Composition, performance, music theory, sound processing are some of the major areas on which research in music and AI are focusing on. Eg : chucks, smart music, etc. Telecommunications - Many telecommunications companies make use of heuristic search in the management of their workforces. - For example BT Group has deployed heuristic search in a scheduling application that provides the work schedules of 20000 engineers. Robotics - A ROBOT is a mechanical or virtual artificial agent, usually an electro mechanical machine that is guided by a computer program or electronic circuitry. Robots can be autonomous or semi-autonomous. A robot may convey a sense of intelligence or thoughts of its own. Gaming - In the earlier days gaming technology was not broadened. - Physicist Willy Higginbotham created the first video game in 1958. - It was called “Tennis For Tow” and was oscilloscope. - But, now AI technology has become vast and standard has also been increased. For Eg : Sudoku, Fear, Fallout, etc Banking - Organize operations, invest in stocks, and manage properties. - In August 2001, robots beat humans in a simulated financial trading competition. - Some other applications include loan investigation, ATM design, safe and fast banking, etc. Some other application: - Credit granting - Information management and retrieval Marawoy, Lipa City, Batangas 4217 | https://www.facebook.com/KLLOfficial/ - AI and expert systems embedded in products - Plant layout - Help desk and assistance - Employee performance evaluation - Shipping - Marketing - Warehouse optimization - In space workstation maintenance - Satellite controls - Network developments - Nuclear management ADAVANTAGES and DISADVANTAGES Marawoy, Lipa City, Batangas 4217 | https://www.facebook.com/KLLOfficial/ Future of Artificial Intelligence Looking at the features and its wide application we may stick to artificial intelligence. Seeing at the development of Al is it that the future world is becoming artificial. Biological intelligence is fixed, because it is an old, mature paradigm but the new paradigm of non- biological computation and intelligence is growing exponentially. The memory capacity of the human brain is probably of the order often thousand million binary digits. But most of this is probably used in remembering visual impressions, and other comparatively wasteful ways. Hence, we can say that as natural intelligence is limited and volatile too world may now depend upon computers for smooth working. Marawoy, Lipa City, Batangas 4217 | https://www.facebook.com/KLLOfficial/ HISTORY OF ARTIFICIAL INTELLIGENCE Artificial Intelligence is not a new word and not a new technology for researchers. This technology is much older than you would imagine. Even there are the myths of Mechanical men in Ancient Greek and Egyptian Myths. Following are some milestones in the history of AI which defines the journey from the AI generation to till date development. Marawoy, Lipa City, Batangas 4217 | https://www.facebook.com/KLLOfficial/ Maturation of Artificial Intelligence (1943-1952) Year 1943: The first work which is now recognized as AI was done by Warren McCulloch and Walter pits in 1943. They proposed a model of artificial neurons. Year 1949: Donald Hebb demonstrated an updating rule for modifying the connection strength between neurons. His rule is now called Hebbian learning. Year 1950: The Alan Turing who was an English mathematician and pioneered Machine learning in 1950. Alan Turing publishes "Computing Machinery and Intelligence" in which he proposed a test. The test can check the machine's ability to exhibit intelligent behavior equivalent to human intelligence, called a Turing test. The birth of Artificial Intelligence (1952-1956) Marawoy, Lipa City, Batangas 4217 | https://www.facebook.com/KLLOfficial/ Year 1955: An Allen Newell and Herbert A. Simon created the "first artificial intelligence program"Which was named as "Logic Theorist". This program had proved 38 of 52 Mathematics theorems, and find new and more elegant proofs for some theorems. Year 1956: The word "Artificial Intelligence" first adopted by American Computer scientist John McCarthy at the Dartmouth Conference. For the first time, AI coined as an academic field. At that time high-level computer languages such as FORTRAN, LISP, or COBOL were invented. And the enthusiasm for AI was very high at that time. The golden years-Early enthusiasm (1956-1974) Year 1966: The researchers emphasized developing algorithms which can solve mathematical problems. Joseph Weizenbaum created the first chatbot in 1966, which was named as ELIZA. Year 1972: The first intelligent humanoid robot was built in Japan which was named as WABOT-1. The first AI winter (1974-1980) The duration between years 1974 to 1980 was the first AI winter duration. AI winter refers to the time period where computer scientist dealt with a severe shortage of funding from government for AI researches. During AI winters, an interest of publicity on artificial intelligence was decreased. A boom of AI (1980-1987) Year 1980: After AI winter duration, AI came back with "Expert System". Expert systems were programmed that emulate the decision-making ability of a human expert. In the Year 1980, the first national conference of the American Association of Artificial Intelligence was held at Stanford University. The second AI winter (1987-1993) The duration between the years 1987 to 1993 was the second AI Winter duration. Again Investors and government stopped in funding for AI research as due to high cost but not efficient result. The expert system such as XCON was very cost effective. The emergence of intelligent agents (1993-2011) Year 1997: In the year 1997, IBM Deep Blue beats world chess champion, Gary Kasparov, and became the first computer to beat a world chess champion. Year 2002: for the first time, AI entered the home in the form of Roomba, a vacuum cleaner. Marawoy, Lipa City, Batangas 4217 | https://www.facebook.com/KLLOfficial/ Year 2006: AI came in the Business world till the year 2006. Companies like Facebook, Twitter, and Netflix also started using AI. Deep learning, big data and artificial general intelligence (2011-present) Year 2011: In the year 2011, IBM's Watson won jeopardy, a quiz show, where it had to solve the complex questions as well as riddles. Watson had proved that it could understand natural language and can solve tricky questions quickly. Year 2012: Google has launched an Android app feature "Google now", which was able to provide information to the user as a prediction. Year 2014: In the year 2014, Chatbot "Eugene Goostman" won a competition in the infamous "Turing test." Year 2018: The "Project Debater" from IBM debated on complex topics with two master debaters and also performed extremely well. Google has demonstrated an AI program "Duplex" which was a virtual assistant and which had taken hairdresser appointment on call, and lady on other side didn't notice that she was talking with the machine. Now AI has developed to a remarkable level. The concept of Deep learning, big data, and data science are now trending like a boom. Nowadays companies like Google, Facebook, IBM, and Amazon are working with AI and creating amazing devices. The future of Artificial Intelligence is inspiring and will come with high intelligence. Marawoy, Lipa City, Batangas 4217 | https://www.facebook.com/KLLOfficial/ ----------------------------------------------------------------------------------------------------------------------------- Name: __________________________________________ Date Accomplished: ____________ Course, Yr. & Sec.: _______________________________ V. ACTIVITY 1 What is Artificial Intelligence? What are the central principles of AI? Is Artificial Intelligence the future? Why? How is Artificial Intelligence helping in our life? VI. OUTPUT (RESULT) Submit your output via email: [email protected] Marawoy, Lipa City, Batangas 4217 | https://www.facebook.com/KLLOfficial/ ----------------------------------------------------------------------------------------------------------------------------- Name: __________________________________________ Date Accomplished: ____________ Course, Yr. & Sec.: _______________________________ VII. EVALUATION After reading the lesson, answer the question “Is Artificial Intelligence more of an advantage, or is it a disadvantage?” at least 10 sentences. (10 pts) AI: A Threat or a Blessing _______________________________________________________________ _______________________________________________________________ _______________________________________________________________ _______________________________________________________________ _______________________________________________________________ _______________________________________________________________ _______________________________________________________________ _______________________________________________________________ _______________________________________________________________ _______________________________________________________________ _______________________________________________________________ _______________________________________________________________ _______________________________________________________________ _______________________________________________________________ ________________________________________________. Rubrics SCORE LEVEL CRITERIA 10 - 9 Excellent Provided in depth answer that clearly met the details of the criteria 8-6 Good Provided good answer that mostly met the details of the criteria but it can be improved further 5-3 Satisfactory Provided limited answer or answer that basically minimally met the details of the criteria 2-1 Needs Improvement Provided unclear answer or answer that poorly met the details of the criteria Marawoy, Lipa City, Batangas 4217 | https://www.facebook.com/KLLOfficial/ MODULE 2 FIRST Semester, AY 2024-2025 I. COURSE CODE/TITLE: CS Elec 3 – Intelligent Systems II. SUBJECT MATTER: TOPICS TIME - FRAME B. Problem Formulation 1. Single State Problem 1 Hour 2. Multiple-State Problem 1 Hour 3. Contingency Problem 1 Hour 4. Exploration Problem 1 Hour Lesson 2. Problem Formulation III. COURSE OUTCOMES A. Differentiate different problem formulations; B. Apply the different problem formulations; C. Familiarize with problem-solving agents and its properties. IV. ENGAGEMENT Magic Squares are square grids with a special arrangement of numbers in them. These numbers are special because every row, column, and diagonal adds up to the same number, which is 15 - the magic number. Could you work this out just by knowing that the square uses the numbers from 1 to 9? MAGIC SQUARE GAME Marawoy, Lipa City, Batangas 4217 | https://www.facebook.com/KLLOfficial/ LESSON 2 : Problem Formulation The reflex agents are known as the simplest agents because they directly map states into actions. Unfortunately, these agents fail to operate in an environment where the mapping is too large to store and learn. Goal-based agent, on the other hand, considers future actions and the desired outcomes. Here, we will discuss one type of goal-based agent known as a problem-solving agent, which uses atomic representation with no internal states visible to the problem-solving algorithms. Problem-solving agent The problem-solving agent performs precisely by defining problems and its several solutions. According to psychology, “a problem-solving refers to a state where we wish to reach to a definite goal from a present state or condition.” According to computer science, a problem-solving is a part of artificial intelligence which encompasses a number of techniques such as algorithms, heuristics to solve a problem. Therefore, a problem-solving agent is a goal-driven agent and focuses on satisfying the goal. Steps performed by Problem-solving agent Goal Formulation: It is the first and simplest step in problem-solving. It organizes the steps/sequence required to formulate one goal out of multiple goals as well as actions to achieve that goal. Goal formulation is based on the current situation and the agent’s performance measure (discussed below). Problem Formulation: It is the most important step of problem-solving which decides what actions should be taken to achieve the formulated goal. There are following five components involved in problem formulation: Initial State: It is the starting state or initial step of the agent towards its goal. Actions: It is the description of the possible actions available to the agent. Transition Model: It describes what each action does. Goal Test: It determines if the given state is a goal state. Path cost: It assigns a numeric cost to each path that follows the goal. The problem-solving agent selects a cost function, which reflects its performance measure. Remember, an optimal solution has the lowest path cost among all the solutions. Note: Initial state, actions, and transition model together define the state-space of the problem implicitly. State-space of a problem is a set of all states which can be reached from the initial state followed by any sequence of actions. The state-space forms a directed map or graph where nodes Marawoy, Lipa City, Batangas 4217 | https://www.facebook.com/KLLOfficial/ are the states, links between the nodes are actions, and the path is a sequence of states connected by the sequence of actions. Search: It identifies all the best possible sequence of actions to reach the goal state from the current state. It takes a problem as an input and returns solution as its output. Solution: It finds the best algorithm out of various algorithms, which may be proven as the best optimal solution. Execution: It executes the best optimal solution from the searching algorithms to reach the goal state from the current state. Goal Formulation Specify the objectives to be achieved goal - a set of desirable world states in which the objectives have been achieved current / initial situation - starting point for the goal formulation actions - cause transitions between world states Problem Formulation - Actions and states to consider states - possible world states accessibility - the agent can determine via its sensors in which state it is consequences of actions - the agent knows the results of its actions levels - problems and actions can be specified at various levels constraints - conditions that influence the problem-solving process performance - measures to be applied costs - utilization of resources PROBLEM TYPES Single-state Problem Complete world state knowledge Complete action knowledge The agent always knows its world state Multiple-state problem Incomplete world state knowledge Marawoy, Lipa City, Batangas 4217 | https://www.facebook.com/KLLOfficial/ Incomplete action knowledge The agent only knows which group of world states it is in Contingency problem It is impossible to define a complete sequence of actions that constitute a solution in advance because information about the intermediary states is unknown. Exploration problem State space and effects of actions unknown. Difficult! SINGLE-STATE Problem Exact prediction is possible. state - is known exactly after any sequence of actions accessibility of the world all essential information can be obtained through sensors consequences of actions are known to the agent goal - for each known initial state, there is a unique goal state that is guaranteed to be reachable via an action sequence simplest case, but severely restricted Marawoy, Lipa City, Batangas 4217 | https://www.facebook.com/KLLOfficial/ MULTI-STATE Problem Semi-exact prediction is possible state is not known exactly, but limited to a set of possible states after each action accessibility of the world not all essential information can be obtained through sensors reasoning can be used to determine the set of possible states consequences of actions are not always or completely known to the agent; actions or the environment might exhibit randomness goal due to ignorance, there may be no fixed action sequence that leads to the goal less restricted, but more complex EXAMPLE: The Vacuum Cleaner World Marawoy, Lipa City, Batangas 4217 | https://www.facebook.com/KLLOfficial/ CONTINGENCY Problem Exact prediction is impossible state unknown in advance, may depend on the outcome of actions and changes in the environment accessibility of the world some essential information may be obtained through sensors only at execution time consequences of action may not be known at planning time Marawoy, Lipa City, Batangas 4217 | https://www.facebook.com/KLLOfficial/ goal instead of single action sequences, there are trees of actions contingency branching point in the tree of actions agent design different from the previous two cases: the agent must act on incomplete plans search and execution phases are interleaved EXAMPLE: Partially observable vacuum world (meaning you don’t know the status of the other square) in which sucking in a clean square may make it dirty. No way to preplan a solution!! A solution starting in a state where you’re in the left room and it’s dirty is: Suck Right if dirty then Suck EXPLORATION Problem Effects of actions are unknown state the set of possible states may be unknown accessibility of the world some essential information may be obtained through sensors only at execution time consequences of actions may not be known at planning time goal can’t be completely formulated in advance because states and consequences may not be known at planning time discovery what states exist experimentation what are the outcomes of actions learning remember and evaluate experiments agent design different from the previous cases: the agent must experiment search requires search in the real world, not in an abstract model realistic problems, very hard Marawoy, Lipa City, Batangas 4217 | https://www.facebook.com/KLLOfficial/ Solving Search Problems Solution A sequence of actions that leads from the initial state to the goal state. o Optimal Solution A solution that has the lowest path cost among all solutions. In a search process, data is often stored in a node, a data structure that contains the following data: A state Marawoy, Lipa City, Batangas 4217 | https://www.facebook.com/KLLOfficial/ Its parent node, through which the current node was generated The action that was applied to the state of the parent to get to the current node The path cost from the initial state to this node Nodes contain information that makes them very useful for the purposes of search algorithms. They contain a state, which can be checked using the goal test to see if it is the final state. If it is, the node’s path cost can be compared to other nodes’ path costs, which allows choosing the optimal solution. Once the node is chosen, by virtue of storing the parent node and the action that led from the parent to the current node, it is possible to trace back every step of the way from the initial state to this node, and this sequence of actions is the solution. However, nodes are simply a data structure — they don’t search, they hold information. To actually search, we use the frontier, the mechanism that “manages” the nodes. The frontier starts by containing an initial state and an empty set of explored items, and then repeats the following actions until a solution is reached: Repeat: 1. If the frontier is empty, o Stop. There is no solution to the problem. 2. Remove a node from the frontier. This is the node that will be considered. 3. If the node contains the goal state, o Return the solution. Stop. Else, * Expand the node (find all the new nodes that could be reached from this node), and add resulting nodes to the frontier. * Add the current node to the explored set. Depth-First Search In the previous description of the frontier, one thing went unmentioned. At stage 1 in the pseudocode above, which node should be removed? This choice has implications on the quality of the solution and how fast it is achieved. There are multiple ways to go about the question of which nodes should be considered first, two of which can be represented by the data structures of stack (in depth-first search) and queue (in breadth-first search; and here is a cute cartoon demonstration of the difference between the two). We start with the depth-first search (DFS) approach. A depth-first search algorithm exhausts each one direction before trying another direction. In these cases, the frontier is managed as a stack data structure. The catchphrase you need to remember Marawoy, Lipa City, Batangas 4217 | https://www.facebook.com/KLLOfficial/ here is “last-in first-out.” After nodes are being added to the frontier, the first node to remove and consider is the last one to be added. This results in a search algorithm that goes as deep as possible in the first direction that gets in its way while leaving all other directions for later. (An example from outside lecture: Take a situation where you are looking for your keys. In a depth- first search approach, if you choose to start with searching in your pants, you’d first go through every single pocket, emptying each pocket and going through the contents carefully. You will stop searching in your pants and start searching elsewhere only once you will have completely exhausted the search in every single pocket of your pants.) Pros: o At best, this algorithm is the fastest. If it “lucks out” and always chooses the right path to the solution (by chance), then depth-first search takes the least possible time to get to a solution. Cons: o It is possible that the found solution is not optimal. o At worst, this algorithm will explore every possible path before finding the solution, thus taking the longest possible time before reaching the solution. Code example: Breadth-First Search The opposite of depth-first search would be breadth-first search (BFS). A breadth-first search algorithm will follow multiple directions at the same time, taking one step in each possible direction before taking the second step in each direction. In this case, the frontier is managed as a queue data structure. The catchphrase you need to remember here is “first-in first- out.” In this case, all the new nodes add up in line, and nodes are being considered based on which one was added first (first come first served!). This results in a search algorithm that takes one step in each possible direction before taking a second step in any one direction. Marawoy, Lipa City, Batangas 4217 | https://www.facebook.com/KLLOfficial/ (An example from outside lecture: suppose you are in a situation where you are looking for your keys. In this case, if you start with your pants, you will look in your right pocket. After this, instead of looking at your left pocket, you will take a look in one drawer. Then on the table. And so on, in every location you can think of. Only after you will have exhausted all the locations will you go back to your pants and search in the next pocket.) Pros: o This algorithm is guaranteed to find the optimal solution. Cons: o This algorithm is almost guaranteed to take longer than the minimal time to run. o At worst, this algorithm takes the longest possible time to run. Greedy Best-First Search Breadth-first and depth-first are both uninformed search algorithms. That is, these algorithms do not utilize any knowledge about the problem that they did not acquire through their own exploration. However, most often is the case that some knowledge about the problem is, in fact, available. For example, when a human maze-solver enters a junction, the human can see which way goes in the general direction of the solution and which way does not. AI can do the same. A type of algorithm that considers additional knowledge to try to improve its performance is called an informed search algorithm. Greedy best-first search expands the node that is the closest to the goal, as determined by a heuristic function h(n). As its name suggests, the function estimates how close to the goal the next node is, but it can be mistaken. The efficiency of the greedy best-first algorithm depends on how good the heuristic function is. For example, in a maze, an algorithm can use a heuristic function that relies on the Manhattan distance between the possible nodes and the end of the maze. The Manhattan distance ignores walls and counts how many steps up, down, or to the sides it would take to get from one location to the goal location. This is an easy estimation that can be derived based on the (x, y) coordinates of the current location and the goal location. Marawoy, Lipa City, Batangas 4217 | https://www.facebook.com/KLLOfficial/ Manhattan Distance However, it is important to emphasize that, as with any heuristic, it can go wrong and lead the algorithm down a slower path than it would have gone otherwise. It is possible that an uninformed search algorithm will provide a better solution faster, but it is less likely to do so than an informed algorithm. A* Search A development of the greedy best-first algorithm, A* search considers not only h(n), the estimated cost from the current location to the goal, but also g(n), the cost that was accrued until the current location. By combining both these values, the algorithm has a more accurate way of determining the cost of the solution and optimizing its choices on the go. The algorithm keeps track of (cost of path until now + estimated cost to the goal), and once it exceeds the estimated cost of some previous option, the algorithm will ditch the current path and go back to the previous option, thus preventing itself from going down a long, inefficient path that h(n) erroneously marked as best. Yet again, since this algorithm, too, relies on a heuristic, it is as good as the heuristic that it employs. It is possible that in some situations it will be less efficient than greedy best-first search or even the uninformed algorithms. For A* search to be optimal, the heuristic function, h(n), should be: Marawoy, Lipa City, Batangas 4217 | https://www.facebook.com/KLLOfficial/ 1. Admissible, or never overestimating the true cost, and 2. Consistent, which means that the estimated path cost to the goal of a new node in addition to the cost of transitioning to it from the previous node is greater or equal to the estimated path cost to the goal of the previous node. To put it in an equation form, h(n) is consistent if for every node n and successor node n’ with step cost c, h(n) ≤ h(n’) + c. Adversarial Search Whereas, previously, we have discussed algorithms that need to find an answer to a question, in adversarial search the algorithm faces an opponent that tries to achieve the opposite goal. Often, AI that uses adversarial search is encountered in games, such as tic tac toe. Minimax A type of algorithm in adversarial search, Minimax represents winning conditions as (-1) for one side and (+1) for the other side. Further actions will be driven by these conditions, with the minimizing side trying to get the lowest score, and the maximizer trying to get the highest score. Representing a Tic-Tac-Toe AI: S₀: Initial state (in our case, an empty 3X3 board) Players(s): a function that, given a state s, returns which player’s turn it is (X or O). Actions(s): a function that, given a state s, return all the legal moves in this state (what spots are free on the board). Result(s, a): a function that, given a state s and action a, returns a new state. This is the board that resulted from performing the action a on state s (making a move in the game). Terminal(s): a function that, given a state s, checks whether this is the last step in the game, i.e. if someone won or there is a tie. Returns True if the game has ended, False otherwise. Utility(s): a function that, given a terminal state s, returns the utility value of the state: -1, 0, or 1. How the algorithm works: Recursively, the algorithm simulates all possible games that can take place beginning at the current state and until a terminal state is reached. Each terminal state is valued as either (-1), 0, or (+1). Marawoy, Lipa City, Batangas 4217 | https://www.facebook.com/KLLOfficial/ Minimax Algorithm in Tic Tac Toe Knowing based on the state whose turn it is, the algorithm can know whether the current player, when playing optimally, will pick the action that leads to a state with a lower or a higher value. This way, alternating between minimizing and maximizing, the algorithm creates values for the state that would result from each possible action. To give a more concrete example, we can imagine that the maximizing player asks at every turn: “if I take this action, a new state will result. If the minimizing player plays optimally, what action can that player take to bring to the lowest value?” However, to answer this question, the maximizing player has to ask: “To know what the minimizing player will do, I need to simulate the same process in the minimizer’s mind: the minimizing player will try to ask: ‘if I take this action, what action can the maximizing player take to bring to the highest value?’” This is a recursive process, and it could be hard to wrap your head around it; looking at the pseudo code below can help. Eventually, through this recursive reasoning process, the maximizing player generates values for each state that could result from all the possible actions at the current state. After having these values, the maximizing player chooses the highest one. Marawoy, Lipa City, Batangas 4217 | https://www.facebook.com/KLLOfficial/ The Maximizer Considers the Possible Values of Future States. To put it in pseudocode, the Minimax algorithm works the following way: Given a state s o The maximizing player picks action a in Actions(s) that produces the highest value of Min- Value(Result(s, a)). o The minimizing player picks action a in Actions(s) that produces the lowest value of Max- Value(Result(s, a)). Function Max-Value(state) o v = -∞ o if Terminal(state): return Utility(state) o for action in Actions(state): v = Max(v, Min-Value(Result(state, action))) return v Marawoy, Lipa City, Batangas 4217 | https://www.facebook.com/KLLOfficial/ Function Min-Value(state): o v=∞ o if Terminal(state): return Utility(state) o for action in Actions(state): v = Min(v, Max-Value(Result(state, action))) return v Alpha-Beta Pruning A way to optimize Minimax, Alpha-Beta Pruning skips some of the recursive computations that are decidedly unfavorable. After establishing the value of one action, if there is initial evidence that the following action can bring the opponent to get to a better score than the already established action, there is no need to further investigate this action because it will decidedly be less favorable than the previously established one. This is most easily shown with an example: a maximizing player knows that, at the next step, the minimizing player will try to achieve the lowest score. Suppose the maximizing player has three possible actions, and the first one is valued at 4. Then the player starts generating the value for the next action. To do this, the player generates the values of the minimizer’s actions if the current player makes this action, knowing that the minimizer will choose the lowest one. However, before finishing the computation for all the possible actions of the minimizer, the player sees that one of the options has a value of three. This means that there is no reason to keep on exploring the other possible actions for the minimizing player. The value of the not-yet-valued action doesn’t matter, be it 10 or (-10). If the value is 10, the minimizer will choose the lowest option, 3, which is already worse than the preestablished 4. If the not-yet-valued action would turn out to be (-10), the minimizer will this option, (-10), which is even more unfavorable to the maximizer. Therefore, computing additional possible actions for the minimizer at this point is irrelevant to the maximizer, because the maximizing player already has an unequivocally better choice whose value is 4. Marawoy, Lipa City, Batangas 4217 | https://www.facebook.com/KLLOfficial/ Depth-Limited Minimax There is a total of 255,168 possible Tic Tac Toe games, and 10²⁹⁰⁰⁰ possible games in Chess. The minimax algorithm, as presented so far, requires generating all hypothetical games from a certain point to the terminal condition. While computing all the Tic-Tac-Toe games doesn’t pose a challenge for a modern computer, doing so with chess is currently impossible. Depth-limited Minimax considers only a pre-defined number of moves before it stops, without ever getting to a terminal state. However, this doesn’t allow for getting a precise value for each action, since the end of the hypothetical games has not been reached. To deal with this problem, Depth-limited Minimax relies on an evaluation function that estimates the expected utility of the game from a given state, or, in other words, assigns values to states. For example, in a chess game, a utility function would take as input a current configuration of the board, try to assess its expected utility (based on what pieces each player has and their locations on the board), and then return a positive or a negative value that represents how favorable the board is for one player versus the other. These values can be used to decide on the right action, and the better the evaluation function, the better the Minimax algorithm that relies on it. Marawoy, Lipa City, Batangas 4217 | https://www.facebook.com/KLLOfficial/ V. ACTIVITY 2 A. Directions: Read the following statements carefully. Choose the letter that best describe the answer and write it on the space provided. 1. They are known as the simplest agents because they directly map states into actions. ______________ a. Goal formulation b. Reflex agents c. Initial state d. Problem Formulation 2. In this type of problem, the state is known exactly after any sequences of action. _____________ a. Single-State Problem b. Multi-State Problem c. Contingency Problem d. Exploration Problem 3. It performs precisely by defining problems and its several solutions. ________ a. Problem Solving Agent b. Reflex Agent c. Goal-based Agent d. Goal Formulation 4. It is the starting state or initial step of the agent towards its goal. _________ a. Goal Test b. Path Cost c. Initial State d. Transition Model 5. It assigns a numeric cost to each path that follows the goal. The problem- solving agent selects a cost function, which reflects its performance measure. ____________ a. Path Cost b. Goal Formulation c. Actions d. Problem-Solving Agent 6. It identifies all the best possible sequence of actions to reach the goal state from the current state. It takes a problem as an input and returns solution as its output. ___________ a. Solution b. Search c. Execution d. Agent 7. It executes the best optimal solution from the searching algorithms to reach the goal state from the current state. _____________ a. Search b. Solution c. Problem Marawoy, Lipa City, Batangas 4217 | https://www.facebook.com/KLLOfficial/ d. Execution 8. It finds the best algorithm out of various algorithms, which may be proven as the best optimal solution. _____________ a. Search b. Solution c. Problem d. Execution 9. It describes what each action does. __________ a. Initial State b. Transition Model c. Actions d. Goal Test 10. It is the most important step of problem-solving which decides what actions should be taken to achieve the formulated goal. ___________ a. Goal Formulation b. Problem Formulation c. Problem Solving Agent d. Goal-Driven Agent B. Directions: Explain briefly the difference between stack (depth-first search) and queue (breadth-first search) using at least 10 sentences. Write your answer on the space provided below. (20 pts.) ______________________________________________________________________ ______________________________________________________________________ ______________________________________________________________________ ______________________________________________________________________ ______________________________________________________________________ ______________________________________________________________________ ______________________________________________________________________ ______________________________________________________________________ ______________________________________________________________________ ______________________________________________________________________ ______________________________________________________________________ ______________________________________________________________________ ______________________________________________________________________ VI. OUTPUT (RESULT) _________. Submit your output via email:[email protected] Marawoy, Lipa City, Batangas 4217 | https://www.facebook.com/KLLOfficial/ ----------------------------------------------------------------------------------------------------------------------------- Name: __________________________________________ Date Accomplished: ____________ Course, Yr. & Sec.: _______________________________ VII. EVALUATION Direction: Explain how the Tic-Tac-Toe AI algorithm works. _________________________________________________________________ _________________________________________________________________ _________________________________________________________________ _________________________________________________________________ _________________________________________________________________ _________________________________________________________________ _________________________________________________________________ _________________________________________________________________ _________________________________________________________________ _________________________________________________________________ _________________________________________________________________ _________________________________________________________________ _________________________________________________________________ _________________________________________________________________ ________________________________________________________________. Rubrics SCORE LEVEL CRITERIA 10 - 9 Excellent Provided in depth answer that clearly met the details of the criteria 8-6 Good Provided good answer that mostly met the details of the criteria but it can be improved further 5-3 Satisfactory Provided limited answer or answer that basically minimally met the details of the criteria 2-1 Needs Improvement Provided unclear answer or answer that poorly met the details of the criteria Marawoy, Lipa City, Batangas 4217 | https://www.facebook.com/KLLOfficial/ MODULE 3 FIRST Semester, AY 2024-2025 I. COURSE CODE/TITLE: CS Elec 3 – Intelligent Systems II. SUBJECT MATTER: TOPICS TIME - FRAME C. Knowledge Representation 1. Knowledge and Representation 0.5 Hour 2. Different Types of Knowledge 0.5 Hour 3. Category of Knowledge 1 Hour 4. Knowledge Typology Map 1 Hour 5. Framework of Knowledge 1 Hour 6. KR Systems requirements 0.5 Hour 7. KR Schemes 0.5 Hour Lesson 3. Knowledge Representation III. COURSE OUTCOMES A. Identify the difference between Knowledge and Representation; B. Differentiate Knowledge Types; C. Explain different categories of Knowledge; D. Understand the KR Typology Map; E. Understand the framework of Knowledge; F. Identify the KR systems requirements; G. Understand KR Schemes. IV. ENGAGEMENT Before going through the lesson, based on your understanding, explain briefly the difference between common sense and knowledge. __________________________________________________________________ __________________________________________________________________ __________________________________________________________________ __________________________________________________________________ __________________________________________________________________ __________________________________________________________________ __________________________________________________________________ __________________________________________________________________ Marawoy, Lipa City, Batangas 4217 | https://www.facebook.com/KLLOfficial/ What is knowledge representation? Humans are best at understanding, reasoning, and interpreting knowledge. Human knows things, which is knowledge and as per their knowledge they perform various actions in the real world. But how machines do all these things comes under knowledge representation and reasoning. Hence we can describe Knowledge representation as following: Knowledge representation and reasoning (KR, KRR) is the part of Artificial intelligence which concerned with AI agents thinking and how thinking contributes to intelligent behavior of agents. It is responsible for representing information about the real world so that a computer can understand and can utilize this knowledge to solve the complex real world problems such as diagnosis a medical condition or communicating with humans in natural language. It is also a way which describes how we can represent knowledge in artificial intelligence. Knowledge representation is not just storing data into some database, but it also enables an intelligent machine to learn from that knowledge and experiences so that it can behave intelligently like a human. What to Represent: Following are the kind of knowledge which needs to be represented in AI systems: Object: All the facts about objects in our world domain. E.g., Guitars contains strings, trumpets are brass instruments. Events: Events are the actions which occur in our world. Performance: It describe behavior which involves knowledge about how to do things. Meta-knowledge: It is knowledge about what we know. Facts: Facts are the truths about the real world and what we represent. Knowledge-Base: The central component of the knowledge-based agents is the knowledge base. It is represented as KB. The Knowledgebase is a group of the Sentences (Here, sentences are used as a technical term and not identical with the English language). Knowledge: Knowledge is awareness or familiarity gained by experiences of facts, data, and situations. Following are the types of knowledge in artificial intelligence: Types of knowledge Following are the various types of knowledge: Marawoy, Lipa City, Batangas 4217 | https://www.facebook.com/KLLOfficial/ 1. Declarative Knowledge: Declarative knowledge is to know about something. It includes concepts, facts, and objects. It is also called descriptive knowledge and expressed in declarative sentences. It is simpler than procedural language. 2. Procedural Knowledge It is also known as imperative knowledge. Procedural knowledge is a type of knowledge which is responsible for knowing how to do something. It can be directly applied to any task. It includes rules, strategies, procedures, agendas, etc. Procedural knowledge depends on the task on which it can be applied. 3. Meta-knowledge: Knowledge about the other types of knowledge is called Meta-knowledge. 4. Heuristic knowledge: Marawoy, Lipa City, Batangas 4217 | https://www.facebook.com/KLLOfficial/ Heuristic knowledge is representing knowledge of some experts in a filed or subject. Heuristic knowledge is rules of thumb based on previous experiences, awareness of approaches, and which are good to work but not guaranteed. 5. Structural knowledge: Structural knowledge is basic knowledge to problem-solving. It describes relationships between various concepts such as kind of, part of, and grouping of something. It describes the relationship that exists between concepts or objects. The relation between knowledge and intelligence: Knowledge of real-worlds plays a vital role in intelligence and same for creating artificial intelligence. Knowledge plays an important role in demonstrating intelligent behavior in AI agents. An agent is only able to accurately act on some input when he has some knowledge or experience about that input. Let's suppose if you met some person who is speaking in a language which you don't know, then how you will able to act on that. The same thing applies to the intelligent behavior of the agents. As we can see in below diagram, there is one decision maker which act by sensing the environment and using knowledge. But if the knowledge part will not present then, it cannot display intelligent behavior. Marawoy, Lipa City, Batangas 4217 | https://www.facebook.com/KLLOfficial/ AI knowledge cycle: An Artificial intelligence system has the following components for displaying intelligent behavior: Perception Learning Knowledge Representation and Reasoning Planning Execution The above diagram shows the interaction of an AI system with the real world and the components involved in showing intelligence. The Perception component retrieves data or information from the environment. WReith the help of this component, you can retrieve data from the environment, find out the source of noises and check if the AI was damaged by anything. Also, it defines how to respond when any sense has been detected. Then, there is the Learning Component that learns from the captured data by the perception component. The goal is to build computers that can be taught instead of programming them. Learning focuses on the process of self-improvement. In order to learn new things, the system requires knowledge acquisition, inference, acquisition of heuristics, faster searches, etc. The main component in the cycle is Knowledge Representation and Reasoning which shows the human-like intelligence in the machines. Knowledge representation is all about understanding intelligence. Instead of trying to understand or build brains from the bottom up, its goal is to understand and build intelligent behavior from the top-down and focus on Marawoy, Lipa City, Batangas 4217 | https://www.facebook.com/KLLOfficial/ what an agent needs to know in order to behave intelligently. Also, it defines how automated reasoning procedures can make this knowledge available as needed. The Planning and Execution components depend on the analysis of knowledge representation and reasoning. Here, planning includes giving an initial state, finding their preconditions and effects, and a sequence of actions to achieve a state in which a particular goal holds. Now once the planning is completed, the final stage is the execution of the entire process. Approaches to knowledge representation: There are mainly four approaches to knowledge representation, which are given below: 1. Simple relational knowledge: It is the simplest way of storing facts which uses the relational method, and each fact about a set of the object is set out systematically in columns. This approach of knowledge representation is famous in database systems where the relationship between different entities is represented. This approach has little opportunity for inference. Example: The following is the simple relational knowledge representation. Player Weight Age Player1 65 23 Player2 58 18 Player3 75 24 2. Inheritable knowledge: In the inheritable knowledge approach, all data must be stored into a hierarchy of classes. All classes should be arranged in a generalized form or a hierarchal manner. In this approach, we apply inheritance property. Elements inherit values from other members of a class. This approach contains inheritable knowledge which shows a relation between instance and class, and it is called instance relation. Every individual frame can represent the collection of attributes and its value. In this approach, objects and values are represented in Boxed nodes. We use Arrows which point from objects to their values. Example: Marawoy, Lipa City, Batangas 4217 | https://www.facebook.com/KLLOfficial/ 3. Inferential knowledge: Inferential knowledge approach represents knowledge in the form of formal logics. This approach can be used to derive more facts. It guaranteed correctness. Example: Let's suppose there are two statements: 1. Marcus is a man 2. All men are mortal Then it can represent as; man(Marcus) ∀x = man (x) ----------> mortal (x)s 4. Procedural knowledge: Procedural knowledge approach uses small programs and codes which describes how to do specific things, and how to proceed. In this approach, one important rule is used which is If-Then rule. In this knowledge, we can use various coding languages such as LISP language and Prolog language. We can easily represent heuristic or domain-specific knowledge using this approach. But it is not necessary that we can represent all cases in this approach. Marawoy, Lipa City, Batangas 4217 | https://www.facebook.com/KLLOfficial/ Categories of Knowledge Knowledge can be categorized into two major types: Tacit knowledge Explicit knowledge Tacit knowledge is the knowledge which exists within a human being. It does correspond to informal or implicit type of knowledge. It is quite difficult to articulate formally and is also difficult to communicate and share. Explicit knowledge is the knowledge which exists outside a human being. It corresponds to formal type of knowledge. It is easier to articulate compared to tacit knowledge and is easier to share, store or even process. Knowledge Typology Map Marawoy, Lipa City, Batangas 4217 | https://www.facebook.com/KLLOfficial/ The Understanding Continuum Requirements for knowledge Representation system: A good knowledge representation system must possess the following properties. 1. Representational Accuracy: KR system should have the ability to represent all kinds of required knowledge. 2. Inferential Adequacy: KR system should have ability to manipulate the representational structures to produce new knowledge corresponding to existing structure. 3. Inferential Efficiency: The ability to direct the inferential knowledge mechanism into the most productive directions by storing appropriate guides. 4. Acquisitional efficiency- The ability to acquire the new knowledge easily using automatic methods. Marawoy, Lipa City, Batangas 4217 | https://www.facebook.com/KLLOfficial/ Knowledge Representation Schemes Introduction to Knowledge Representation Scheme: Knowledge Representation (KR) originated as a sub-field of Artificial Intelligence (AI). In the early days of AI, it was sometimes imagined that to endow a computer with intelligence it would be sufficient to give it a capacity for pure reasoning; it quickly became apparent, however, that the exercise of intelligence inevitably involves interaction with an external world, and such interaction cannot take place without some kind of knowledge of that world. Thus, it became clear that part of the quest for AI must involve the development of methods for endowing computer systems with knowledge. This in turn brought to the fore the question of how such knowledge is to be represented within the computer. This question can be approached in many different ways, but one can broadly distinguish between approaches which seek to discover, and thereby emulate, the forms in which knowledge is represented in the human brain, and those which take their inspiration from the external forms of representation used by humans to encode their knowledge notably language, mathematics and formal logic. The term Knowledge Representation (KR), when used in the AI context, is generally taken to refer to approaches of the latter kind rather than the former, which are regarded as more within the province of Cognitive Science. We thus find that KR is characterised in the literature in terms which emphasise the quest for explicit symbolic representations of knowledge which are suitable for use by computer some views are: Knowledge representation and reasoning is the area of Artificial Intelligence (AI) concerned with how knowledge can be represented symbolically and manipulated in an automated way by reasoning programs. Knowledge representation is the study of how to put knowledge into a form that a computer can reason with… Knowledge representation research studies the problem of finding a language in which to encode that knowledge so that the machine can use it. Knowledge Representation is no longer, however, exclusively the preserve of AI, as witnessed by the following remark by Sowa: Marawoy, Lipa City, Batangas 4217 | https://www.facebook.com/KLLOfficial/ Today, advanced systems everywhere are performing tasks that used to require human intelligence… As a result, the AI design techniques have converged with techniques from other fields, especially database and object-oriented systems. n particular, Knowledge Representation is closely allied with Formal Ontology, which is concerned with the systematic enumeration and classification of the various kinds of entity represented within a given conceptualisation of the world, together with an account of their properties and relationships. Having started life as a discipline within Philosophy, Formal Ontology has become an important strand within information systems research, with particular application to the problems of maintaining coherence and consistency when combining large bodies of knowledge from different sources, as happens ever more frequently with the expansion of the World Wide Web. Metrics of Knowledge Representation Scheme: 1. Ease of Representation: The ease with which a problem can be solved depends upon knowledge representation scheme. For example, imagine we have a 3 x 3 chess board with a knight in each corner and we want to know (Fig. 6.1) how many moves will it take to move knight round the next corner. Looking at diagrammatic representation of Fig. 6.1., the solution is not obvious, but if we label each square and present valid moves as adjacent points on a circle (Fig. 6.2) the solution becomes more obvious; each knight takes two moves to reach its new position so the minimum number of moves is eight. Marawoy, Lipa City, Batangas 4217 | https://www.facebook.com/KLLOfficial/ 2. Granuality of Representation: Granuality of knowledge representation can effect its usefulness, that is, how detailed the knowledge need to be represented. This will depend on the application and the function to which the knowledge will be put. For example, if a knowledge base about family relationships is to be built and we start with ‘cousin’. We may represent the definition of the relation ‘cousin’ as follows: Your cousin is a child of sibling of your parent. When we want to know the gender of the cousin this information may not be enough. For a female cousin your cousin is a daughter of a sibling of your parent and for a male cousin your cousin is a son of sibling of your parent. If we want to know to which side of the parents your cousin belongs we need more information than that our cousin is a child of a sibling of our parents. A full description of all the possible variations is given below: i. Your cousin is a daughter of a sister of your mother, ii. Your cousin is a daughter of a sister of your father, iii. Your cousin is a daughter of a brother of your mother, iv. Your cousin is a daughter of a sister of your father, v. Your cousin is a son of a sister of your mother, vi. You cousin is a son of a sister of your father, vii. You cousin is a son of a brother of your mother, and viii. You cousin is a son of a brother of your father. It concerns the level of detail with which information is recorded. Most obviously, this could be a matter of resolution; e.g., a map which records the presence or absence of a particular species of plant in each 2 km square has a finer granularity than one which is based on 10 km squares. Similarly a road map which only shows the main trunk roads has a coarser granularity than one which shows all the minor roads in addition. And a map of India showing the state boundaries only has a coarser granularity than that of states. This is not just a matter of scale; in general the larger the scale of a map, the finer its granularity is likely to be but this correlation is not exact. An approach to a formal treatment of granularity in Al is proposed by Hobbs (1985). Granularity affects both time and space and the two in combination. Granularity related problems arise in a specific form in cartography in relation to map Marawoy, Lipa City, Batangas 4217 | https://www.facebook.com/KLLOfficial/ generalization, that is, the task to reducing the level of detail in a map while preserving the correct relationship amongst the features which remain. 3. Property Inheritances: World can be represented by facts and facts are related to each other. A fact may be specific instance of another more general fact. “Spotty dog barks” for example is a specific instance of the fact “all dogs bark”. This is a case of property inheritance, in which properties of attributes of the main class are inherited by instance of that class. So we represent the knowledge that “dogs bark” and that “spotty is a dog”, allowing us to deduce by inheritance, the fact that “spotty dog barks”. 4. Expressiveness: An expressive representation scheme will be able to handle different types and levels of granularity of knowledge and knowledge structures and the relationships between them. It will have means of representing specific facts and generic information (say by using variables). Expressiveness also relates to the clarity of the representation scheme. Ideally the scheme should use a notation which is natural and usable both by the knowledge engineer and the domain expert. Schemes which are too complex for a domain expert to understand can result in incorrect knowledge being held, since the expert may not be able to critique knowledge adequately, that is knowledge representation should be characterised by completeness and clarity. 5. Effectiveness: In order to be effective the scheme must provide a means of inferring new knowledge from old. It should also be amenable to computation, allowing proper use of adequate tool support. 6. Explicitness: A good knowledge representation scheme should be able to provide an explanation of its inference and allow justifications of its reasoning. The chain of reasoning should be explicit. 7. Efficiency: The scheme should not only support inference of new knowledge from old but must do so efficiently in order for new knowledge to be used. In addition, scheme should facilitate efficient knowledge gathering and representation. Many highly expressive representations are too inefficient for use in certain classes of problems. Sometimes, expressiveness must be sacrificed to improve efficiency. This must be done without limiting the representation’s ability to capture essential problem-solving knowledge. Optimising the trade-off between efficiency and expressiveness is a major task for designers of intelligent systems. Marawoy, Lipa City, Batangas 4217 | https://www.facebook.com/KLLOfficial/ Categories of Knowledge Representation Schemes: Over the past 40 years, numerous representational scheme have been proposed and implemented, each of them having its own strength and weakness. According to Mylopoulos and Levesque (1984) they have been classified into four categories: 1. Logical Representation Scheme: This class of representation uses expressions in formal logic to represent a knowledge base. Inference rules and proof procedures apply this knowledge to problem solving. First order predicate calculus is the most widely used logical representation scheme, and PROLOG is an ideal programming language for implementing logical representation schemes. 2. Procedural Representation Scheme: Procedural scheme represents knowledge as a set of instructions for solving a problem. In a rule- based system, for example, an if then rule may be interpreted as a procedure for searching a goal in a problem domain: to arrive at the conclusion, solve the premises in order. Production systems are examples of a procedural representation scheme. 3. Network Representation Scheme: Network representation captures knowledge as a graph in which the nodes represent objects or concepts in the problem domain and the arcs represent relations or associations between them. Examples of network representations include semantic network, conceptual dependencies and conceptual graphs. 4. Structured Representation Scheme: Structured representation languages extend networks by allowing each node to be a complex data structure consisting of named slots with attached values. These values may be simple numeric or complex data, such as pointers to other frames, or even procedures. Marawoy, Lipa City, Batangas 4217 | https://www.facebook.com/KLLOfficial/ ----------------------------------------------------------------------------------------------------------------------------- Name: __________________________________________ Date Accomplished: ____________ Course, Yr. & Sec.: _______________________________ V. ACTIVITY 3 A. Identification: Identify the following. Write your answer on the space provided after each number. 1. It is the central component of the knowledge-based agents. It is represented as KB. _________________________ 2. It is the awareness or familiarity gained by experiences of facts, data, and situations. ___________________ 3. It is also known as imperative knowledge. _________________________ 4. It is knowledge about the other types of knowledge. ______________________ 5. It is to know about something. Int includes concepts, facts, and objects. ________________ 6. It is representing the knowledge of some experts in a field or subject. ________________ 7. It retrieves data or information from the environment. ________________________ 8. The component that learns from the captured data by the perception component. ________________________________ 9. It shows the human-like intelligence in the machines. __________________________ 10. These components depend on the analysis of knowledge representation and reasoning. _______________ B. MULTIPLE CHOICE: Choose the best answer. Write the letter of your answer on the space provided after each number. 1. KR system should have the ability to represent all kinds of required knowledge. ______________ a. Inferential Adequacy b. Representational Accuracy c. Acquisitional Efficiency d. Inferential Efficiency 2. The ability to acquire new knowledge easily using automatic methods. ___________ a. Inferential Adequacy b. Representational Accuracy c. Acquisitional Efficiency d. Inferential Efficiency 3. This class of representation uses expressions in formal logic to represent a knowledge base. ___________ a. Network Representation Scheme b. Structured Representation Scheme Marawoy, Lipa City, Batangas 4217 | https://www.facebook.com/KLLOfficial/ c. Logical Representation Scheme d. Procedural Representation Scheme 4. Captures knowledge as a graph in which the nodes represent objects or concepts in the problem domain and the arcs represent relations or associations between them. _______________ a. Network Representation Scheme b. Structured Representation Scheme c. Logical Representation Scheme d. Procedural Representation Scheme 5. It represents knowledge as a set of instructions for solving a problem. ___________ a. Network Representation Scheme b. Structured Representation Scheme c. Logical Representation Scheme d. Procedural Representation Scheme VI. OUTPUT (RESULT) Submit your output via email:[email protected] Marawoy, Lipa City, Batangas 4217 | https://www.facebook.com/KLLOfficial/ ----------------------------------------------------------------------------------------------------------------------------- Name: __________________________________________ Date Accomplished: ____________ Course, Yr. & Sec.: _______________________________ VII. EVALUATION Direction: Give a real-life example and application of Knowledge Representation and Reasoning and explain its function. Real-World Knowledge Representation and Reasoning _______________________________________________________________ _______________________________________________________________ _______________________________________________________________ _______________________________________________________________ _______________________________________________________________ _______________________________________________________________ _______________________________________________________________ _______________________________________________________________ _______________________________________________________________ _______________________________________________________________ _______________________________________________________________ _______________________________________________________________ _______________________________________________________________ _______________________________________________________________ ________________________________________________. Rubrics SCORE LEVEL CRITERIA 10 - 9 Excellent Provided in depth answer that clearly met the details of the criteria 8-6 Good Provided good answer that mostly met the details of the criteria but it can be improved further 5-3 Satisfactory Provided limited answer or answer that basically minimally met the details of the criteria 2-1 Needs Improvement Provided unclear answer or answer that poorly met the details of the criteria Marawoy, Lipa City, Batangas 4217 | https://www.facebook.com/KLLOfficial/ MODULE 4 FIRST Semester, AY 2024-2025 I. COURSE CODE/TITLE: CS Elec 3 – Intelligent Systems II. SUBJECT MATTER: TOPICS TIME - FRAME D. Logic Programming 1. Concept of Logic Programming 2.5 hour 2. Characteristics of Logic Programming 2.5 hour Lesson 4. Logic Programming III. COURSE OUTCOMES A. Understand the concept of logic programming; B. Familiarize with the characteristics of logic programming. IV. ENGAGEMENT Consider the following arguments: Alice likes everyone who likes logic. Alice likes logic. ------------------------------------------------------- Alice likes herself. Question: Is this argument valid? How do you know? ________________________________________________________________________ _____________________________________________________________________________ _____________________________________________________________________________ _____________________________________________________________________________ _____________________________________________________________________________ _____________________________________________________________________________ Marawoy, Lipa City, Batangas 4217 | https://www.facebook.com/KLLOfficial/ LESSON 4 - LOGIC PROGRAMMING Logic Logic is not concerned with what is true. Logic is the study of what follows from what. What conclusions follow from a set of premises. It can be defined as study of principles of correct reasoning. The main thing we study in logic are principles governing the validity of arguments and check that whether certain conclusion follows from some given assumption. The logic process takes in some information called premises and produces some outputs called conclusions. Symbolic Logic Method of representing logical expressions through the use of symbols and variables, rather than in ordinary language. It provides the benefit of removing the ambiguity that is generally seen in ordinary languages like English. It is normally divided into two branches: 1.Propositional Logic 2.Predicate Logic Propositional Logic Simplest form of formal logic. All statements made are called propositions. The FORMAL LOGIC is concerned with syntax of statements and not with their semantics. It deals with manipulation of logical variables which represents proposition. Propositional Logic is concerned with the subset of declarative sentences that can be either true or false. Propositional Logic takes only two values, either TRUE or FALSE. Syntax of Propositional Logic 1.Letters A, B,….Z and these letters with subscripted numerals are well-formed atomic propositions. Marawoy, Lipa City, Batangas 4217 | https://www.facebook.com/KLLOfficial/ 2.If A and B are well-formed propositions so are: Why we use Propositional Logic? Its easier to check formulas. We can exploit the Boolean nature for efficient reasoning. Its easier to incrementally add formulas. It can be extended infinitely to many variables using logical quantifiers. Marawoy, Lipa City, Batangas 4217 | https://www.facebook.com/KLLOfficial/ First-order Predicate Logic First-order predicate calculus (FOPL) was developed by logicians to extend the expressiveness of Propositional Logic. It is generalization of propositional logic that permits reasoning about world entities (objects) as well as classes and subclasses of objects. Prolog is also based on FOPL. Predicate logic uses variables and quantifiers which is not present in propositional logic. Why First-Order Predicate Logic ? Suppose we are having 2 statements , based on which we have to draw a conclusion. Statement 1: All students must take Java. Statement 2: John is a student. According to human inference, John must take Java. but not according to Propositional logic. (Disadvantage) First-order predicate calculus First-order predicate calculus classifies the different parts of such statements as follows: 1.Constants 2.Predicates 3.Functions Marawoy, Lipa City, Batangas 4217 | https://www.facebook.com/KLLOfficial/ 4.Variables 5.Connectives 6.Quantifiers 7.Punctuation Symbols Predicates These are names for functions that are true or false, like Boolean functions in a program. Defined as a relation that binds two atoms together. Example: Amit likes sweets. likes(amit, areoplanes). amit and sweets are atoms; likes is a predicate Predicates can take a number of arguments. In Example , the predicate natural takes one argument. natural(n). Predicates Its possible to have a function as an argument Eg. Ravi’s father is Rani’s father father(father(ravi), rani). Father is a predicate and father(ravi) is a function to indicate Ravi’s father. ▪ Constants These are usually numbers or names. Sometimes they are called atoms, since they cannot be broken down into subparts. Example natural(0). 0 is a constant ▪ Variables Stands for Quantities that are yet Unspecified. Example valuable(X) X is a variable. ▪ Functions Marawoy, Lipa City, Batangas 4217 | https://www.facebook.com/KLLOfficial/ First-order predicate calculus distinguishes between functions and predicates. Predicates - true or false and all other are functions which represent non-Boolean values. Marawoy, Lipa City, Batangas 4217 | https://www.facebook.com/KLLOfficial/ Some points to remember Arguments to predicates and functions can only be terms which is a combination of variables, constants and functions. Terms cannot contain predicates, quantifiers or connectives. Marawoy, Lipa City, Batangas 4217 | https://www.facebook.com/KLLOfficial/ ----------------------------------------------------------------------------------------------------------------------------- Name: __________________________________________ Date Accomplished: ____________ Course, Yr. & Sec.: _______________________________ V. ACTIVITY 4 A. Answer the following questions. Choose the best answer. Write the letter of your answer on the space provided after each number. 1. It is the method of representing logical expressions through the use of symbols and variables, rather than in ordinary language. ___________ a. Logic b. Symbolic Logic c. Propositional Logic d. Predicate Logic 2. It is the simplest form of formal logic. ______________ a. Logic b. Symbolic Logic c. Propositional Logic d. Predicate Logic 3. It is the generalization of propositional logic that permits reasoning about world entities (objects) as well as classes and subclasses of objects. __________ a. First-Order Predicate Logic b. Variables c. Predicates d. Quantifiers 4. These are the names for functions that are true or false, like Boolean functions in a program. ______________________ a. First-Order Predicate Logic b. Variables c. Predicates d. Quantifiers 5. Declares the scope or range of variables in a logical expression. a. First-Order Predicate Logic b. Variables c. Predicates d. Quantifiers Marawoy, Lipa City, Batangas 4217 | https://www.facebook.com/KLLOfficial/ B. Explain briefly. Why do we use Propositional Logic? ________________________________________________________________________ ________________________________________________________________________ ________________________________________________________________________ ________________________________________________________________________ ________________________________________________________________________ ________________________________________________________________________ ________________________________________________________________________ ________________________________________________________________________ ________________________________________________________________________ ________________________________________________________________________ ________________________________________________________________________ ________________________________________________________________________ ________________________________________________________________________ VI. OUTPUT (RESULT) Submit your output via email:[email protected] Marawoy, Lipa City, Batangas 4217 | https://www.facebook.com/KLLOfficial/ ----------------------------------------------------------------------------------------------------------------------------- Name: __________________________________________ Date Accomplished: ____________ Course, Yr. & Sec.: _______________________________ VII. EVALUATION Direction: Create a simple python program that displays the largest of the three input numbers. Rubrics SCORE LEVEL CRITERIA 10 - 9 Excellent Provided in depth answer that clearly met the details of the criteria 8-6 Good Provided good answer that mostly met the details of the criteria but it can be improved further 5-3 Satisfactory Provided limited answer or answer that basically minimally met the details of the criteria 2-1 Needs Improvement Provided unclear answer or answer that poorly met the details of the criteria Marawoy, Lipa City, Batangas 4217 | https://www.facebook.com/KLLOfficial/ References: Artificial Intelligence Intelligent System by Tutorial Points Stuart Russell and Peter Norvig. (2014). Artificial Intelligence: A Modern Approach. Edinburg Gate, Pearson Education Limited Patrick Henry Winston. Artificial Intelligence (3rd Edition). (1992). Addison-Wesley Longman Publishing Co., Inc.75 Arlington Street, Suite 300 Boston, MA United States Nils J Nilsson. Artificial Intelligence: A New Synthesis. (1998) Morgan Kaufmann Publishers Christopher M Bishop. Pattern Recognition and Machine Learning. (2011). Springer Jeff Heaton. Artificial Intelligence for Humans. vol.1 (2013). vol. 2 (2014). Createspace Independent Publishing Platform. vol. 3 (2015) Heaton Research, Inc. Prepared by: GRACE R. FABILA, MMEM Instructor III Marawoy, Lipa City, Batangas 4217 | https://www.facebook.com/KLLOfficial/