CQF_Jan_Maths_Primer_2013_Probability_Blank.pdf
Document Details
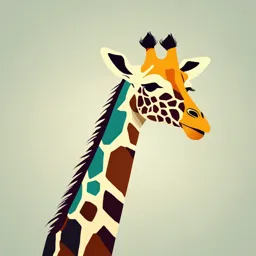
Uploaded by MarvelousOrientalism6818
2011
Tags
Full Transcript
CERTIFICATE IN FINANCE CQF Certificate in Quantitative Finance Subtext t here GLOBAL STAN...
CERTIFICATE IN FINANCE CQF Certificate in Quantitative Finance Subtext t here GLOBAL STANDARD IN FINANCIAL ENGINEERING A4 PowerPoint cover Portrait2.indd 1 21/10/2011 10:53 Certificate in Quantitative Finance Probability and Statistics June 2011 1 1 PROBABILITY 1 Probability 1.1 Preliminaries An experiment is a repeatable process that gives rise to a number of outcomes. An event is a collection (or set) of one or more out- comes. An sample space is the set of all possible outcomes of an experiment, often denoted Ω. Example In an experiment a dice is rolled and the number ap- pearing on top is recorded. Thus Ω = {1, 2, 3, 4, 5, 6} If E1 , E2 , E3 are the events even, odd and prime occur- ring, then E1 ={2, 4, 6} E2 ={1, 3, 5} E3 ={2, 3, 5} 2 1.1 Preliminaries 1 PROBABILITY 1.1.1 Probability Scale Probability of an Event E occurring i.e. P (E) is less than or equal to 1 and greater than or equal to 0. 0 ≤ P (E) ≤ 1 1.1.2 Probability of an Event The probability of an event occurring is defined as: The number of ways the event can occur P (E) = Total number of outcomes Example A fair dice is tossed. The event A is defined as the number obtained is a multiple of 3. Determine P (A) Ω ={1, 2, 3, 4, 5, 6} A ={3, 6} 2 ∴ P (A) = 6 1.1.3 The Complimentary Event E 0 An event E occurs or it does not. If E is the event then E 0 is the complimentary event, i.e. not E where P (E 0 ) = 1 − P (E) 3 1.2 Probability Diagrams 1 PROBABILITY 1.2 Probability Diagrams It is useful to represent problems diagrammatically. Three useful diagrams are: Sample space or two way table Tree diagram Venn diagram Example Two dice are thrown and their numbers added to- gether. What is the probability of achieving a total of 8? 5 P (8) = 36 Example A bag contains 4 red, 5 yellow and 11 blue balls. A ball is pulled out at random, its colour noted and then 4 1.2 Probability Diagrams 1 PROBABILITY replaced. What is the probability of picking a red and a blue ball in any order. P(Red and Blue) or P(Blue and Red) = 4 11 11 4 11 × + × = 20 20 20 20 50 Venn Diagram A Venn diagram is a way of representing data sets or events. Consider two events A and B. A Venn diagram to represent these events could be: A∪B ”A or B” 5 1.2 Probability Diagrams 1 PROBABILITY A∩B ”A and B” Addition Rule: P (A ∪ B) = P (A) + P (B) − P (A ∩ B) or P (A ∩ B) = P (A) + P (B) − P (A ∪ B) Example In a class of 30 students, 7 are in the choir, 5 are in the school band and 2 students are in the choir and the school band. A student is chosen at random from the class. Find: a) The probability the student is not in the band b) The probability the student is not in the choir nor in the band 6 1.2 Probability Diagrams 1 PROBABILITY 5 + 20 P (not in band) = 30 25 5 = = 30 6 20 2 P (not in either) = = 30 3 Example A vet surveys 100 of her clients, she finds that: (i) 25 own dogs (ii) 53 own cats (iii) 40 own tropical fish (iv) 15 own dogs and cats (v) 10 own cats and tropical fish 7 1.2 Probability Diagrams 1 PROBABILITY (vi) 11 own dogs and tropical fish (vii) 7 own dogs, cats and tropical fish If she picks a client at random, Find: a) P(Owns dogs only) b) P(Does not own tropical fish) c) P(Does not own dogs, cats or tropical fish) 6 P (Dogs only) = 100 6 + 8 + 35 + 11 60 P (Does not own tropical fish) = = 100 100 11 P (Does not own dogs, cats or tropical fish) = 100 8 1.3 Conditional Probability 1 PROBABILITY 1.3 Conditional Probability The probability of an event B may be different if you know that a dependent event A has already occurred. Example Consider a school which has 100 students in its sixth form. 50 students study mathematics, 29 study biology and 13 study both subjects. You walk into a biology class and select a student at random. What is the probability that this student also studies mathematics? 13 P (study maths given they study biology) = P (M |B) = 29 In general, we have: 9 1.3 Conditional Probability 1 PROBABILITY P (A ∩ B) P (A|B) = P (B) or, Multiplication Rule: P (A ∩ B) = P (A|B) × P (B) Example You are dealt exactly two playing cards from a well shuffled standard 52 card deck. What is the probability that both your cards are Kings ? Tree Diagram! 4 3 1 P (K ∩ K) = × = =≈ 0.5% 52 51 221 or 3 4 P (K∩K) = P (2nd is King | first is king)×P (first is king) = × 51 52 We know, P (A ∩ B) = P (B ∩ A) 10 1.3 Conditional Probability 1 PROBABILITY so P (A ∩ B) = P (A|B) × P (B) P (B ∩ A) = P (B|A) × P (A) i.e. P (A|B) × P (B) = P (B|A) × P (A) or Bayes’ Theorem: P (A|B) × P (B) P (B|A) = P (A) Example You have 10 coins in a bag. 9 are fair and 1 is double headed. If you pull out a coin from the bag and do not examine it. Find: 1. Probability of getting 5 heads in a row 2. Probability that if you get 5 heads the you picked the double headed coin 11 1.3 Conditional Probability 1 PROBABILITY P (5heads) = P (5heads|N ) × P (N ) + P (5heads|H) × P (H) 1 9 1 = × + 1× 32 10 10 41 = 320 ≈ 13% P (5heads|H) × P (H) P (H|5heads) = P (5heads) 1 1 × 10 = 41 320 320 = 410 ≈ 78% 12 1.4 Mutually exclusive and Independent events 1 PROBABILITY 1.4 Mutually exclusive and Independent events When events can not happen at the same time, i.e. no outcomes in common, they are called mutually exclu- sive. If this is the case, then P (A ∩ B) = 0 and the addition rule becomes P (A ∪ B) = P (A) + P (B) Example Two dice are rolled, event A is ’the sum of the out- comes on both dice is 5’ and event B is ’the outcome on each dice is the same’ When one event has no effect on another event, the two events are said to be independent, i.e. P (A|B) = P (A) and the multiplication rule becomes P (A ∩ B) = P (A) × P (B) 13 1.5 Two famous problems 1 PROBABILITY Example A red dice and a blue dice are rolled, if event A is ’the outcome on the red dice is 3’ and event B ’is the outcome on the blue dice is 3’ then events A and B are said to be independent. 1.5 Two famous problems Birthday Problem - What is the probability that at least 2 people share the same birthday Monty Hall Game Show - Would you swap ? 14 1.6 Random Variables 1 PROBABILITY 1.6 Random Variables 1.6.1 Notation Random Variables X, Y, Z Observed Variables x, y, z 1.6.2 Definition Outcomes of experiments are not always numbers, e.g. two heads appearing; picking an ace from a deck of cards. We need some way of assigning real numbers to each ran- dom event. Random variables assign numbers to events. Thus a random variable (RV) X is a function which maps from the sample space Ω to the number line. Example let X = the number facing up when a fair dice is rolled, or let X represent the outcome of a coin toss, where 1 if heads X= 0 if tails 1.6.3 Types of Random variable 1. Discrete - Countable outcomes, e.g. roll of a dice, rain or no rain 2. Continuous - Infinite number of outcomes, e.g. exact amount of rain in mm 15 1.7 Probability Distributions 1 PROBABILITY 1.7 Probability Distributions Depending on whether you are dealing with a discrete or continuous random variable will determine how you define your probability distribution. 1.7.1 Discrete distributions When dealing with a discrete random variable we de- fine the probability distribution using a probaility mass fucntion or simply a probability function. Example The RV X is defined as’ the sum of scores shown by two fair six sided dice’. Find the probability distribution of X A sample space diagram for the experiment is: The distribution can be tabulated as: x 2 3 4 5 6 7 8 9 10 11 12 1 2 3 4 5 6 5 4 3 2 1 P (X = x) 36 36 36 36 36 36 36 36 36 36 36 16 1.7 Probability Distributions 1 PROBABILITY or can be represented on a graph as 1.7.2 Continuous Distributions As continuous random variables can take any value, i.e an infinite number of values, we must define our probability distribution differently. For a continuous RV the probability of getting a spe- cific value is zero, i.e P (X = x) = 0 and so just as we go from bar charts to histograms when representing discrete and continuous data, we must use a probability density function (PDF) when describing the probability distribution of a continuous RV. 17 1.7 Probability Distributions 1 PROBABILITY Z b P (a < X < b) = f (x)dx a Properties of a PDF: f (x) ≥ 0 since probabilities are always positive R +∞ ∞ f (x)dx = 1 Rb P (a < X < b) = a f (x)dx Example The random variable X has the probability density function: k 1 11) c) P (13 < X < 15) a) X − µ 14 − 12 Z= = = 0.5 σ 4 Therefore we want P (Z ≤ 0.5) = Φ(0.5) = 0.6915 (from tables) b) 42 1.13 Important Distributions 1 PROBABILITY 11 − 12 Z= = −0.25 4 Therefore we want P (Z > −0.25) but this is not in the tables. From symmetry this is the same as P (Z < 0.25) i.e. Φ(0.25) thus P (Z > −0.25) = Φ(0.25) = 0.5987 c) 43 1.13 Important Distributions 1 PROBABILITY 13 − 12 Z1 = = 0.25 4 15 − 12 Z2 = = 0.75 4 Therefore P (0.25 < Z < 0.75) = Φ(0.75) − Φ(0.25) = 0.7734 − 0.5987 = 0.1747 1.13.5 Common regions The percentages of the Normal Distribution lying within the given number of standard deviations either side of the mean are approximately: One Standard Deviation: Two Standard Deviations: 44 1.13 Important Distributions 1 PROBABILITY Three Standard Deviations: 45 1.14 Central Limit Theorem 1 PROBABILITY 1.14 Central Limit Theorem The Central Limit Theorem states: Suppose X1 , X2 ,......, Xn are n independent random variables, each having the same distribution. Then as n increases, the distributions of X1 + X2 +...... + Xn and of X1 + X2 +...... + Xn n come increasingly to resemble normal distributions. Why is this important ? The importance lies in the fact: (i) The common distribution of X is not stated - it can be any distribution (ii) The resemblance to a normal distribution holds for remarkably small n (iii) Total and means are quantities of interest If X is a random variable with mean µ and standard devaition σ fom an unknown distribution, the central limit theorem states that the distribution of the sample means is Normal. But what are it’s mean and variance ? Let us consider the sample mean as another random variable, which we will denote X̄. We know that X1 + X2 +......Xn 1 1 1 X̄ = = X1 + X2 +...... + Xn n n n n 46 1.14 Central Limit Theorem 1 PROBABILITY We want E(X̄) and V ar(X̄) 1 1 1 E(X̄) = E X1 + X2 +...... + Xn n n n 1 1 1 = E(X1 ) + E(X2 ) +...... + E(Xn ) n n n 1 1 1 = µ + µ +...... + µ n n n 1 = n µ n = µ i.e. the expectation of the sample mean is the popu- lation mean ! 1 1 1 V ar(X̄) = V ar X1 + X2 +...... + Xn n n n 1 1 1 = V ar X1 + V ar X2 +...... + V ar Xn n n n 2 2 2 1 1 1 = V ar(X1 ) + V ar(X2 ) +..... + V ar(Xn ) n n n 2 2 2 1 2 1 2 1 = σ + σ +..... + σ2 n n n 2 1 = n σ2 n 2 σ = n Thus CLT tells us that where n is a sufficiently large 47 1.14 Central Limit Theorem 1 PROBABILITY number of samples. σ2 X̄ ∼ N (µ, ) n Standardising, we get the equivalent result that X̄ − µ ∼ N (0, 1) √σ n This analysis could be repeated for the sum Sn = X1 + X2 +....... + Xn and we would find that Sn − nµ √ ∼ N (0, 1) σ n Example Consider a 6 sided fair dice. We know that E(X) = 3.5 and V ar(X) = 3512. Let us now consider an experiment. The experiment consists of rolling the dice n times and calculating the average for the experiment. We will run 500 such exper- iments and record the results in a Histogram. n=1 In each experiment the dice is rolled once only, this experiment is then repeated 500 times. The graph below shows the resulting frequency chart. 48 1.14 Central Limit Theorem 1 PROBABILITY This clearly resembles a uniform distribution (as ex- pected). Let us now increase the number of rolls, but continue to carry out 500 experiments each time and see what happens to the distribution of X̄ n=5 49 1.14 Central Limit Theorem 1 PROBABILITY n=10 n=30 We can see that even for small sample sizes (number of dice rolls), our resulting distribution begins to look more like a Normal distribution. we can also note that as n increases our distribution begins to narrow, i.e. the 2 variance becomes smaller σn , but the mean remains the same µ. 50 2 STATISTICS 2 Statistics 2.1 Sampling So far we have been dealing with populations, however sometimes the population is too large to be able to anal- yse and we need to use a sample in order to estimate the population parameters, i.e. mean and variance. Consider a population of N data points and a sample taken from this population of n data points. We know that the mean and variance of a population are given by: PN i=1 xi population mean, µ= N and PN 2 i=1 (xi − x̄)2 population variance, σ = N 51 2.1 Sampling 2 STATISTICS But how can we use the sample to estimate our pop- ulation parameters? First we define an unbiased estimator. An unbiased estimator is when the expected value of the estimator is exactly equal to the corresponding population parame- ter, i.e. if x̄ is the sample mean then the unbiased estimator is E(x̄) = µ where the sample mean is given by: PN i=1 xi x̄ = n 2 If S is the sample variance, then the unbiased esti- mator is E(S 2 ) = σ 2 where the sample variance is given by: Pn 2 2 i=1 (xi − x̄) S = n−1 2.1.1 Proof From the CLT, we know: E(X̄) = µ and σ2 V ar(X̄) = n Also V ar(X̄) = E(X̄ 2 ) − [E(X̄)]2 52 2.1 Sampling 2 STATISTICS i.e. σ2 = E(X̄ 2 ) − µ2 n or σ2 + µ2 E(X̄ 2 ) = n For a single piece of data n = 1, so E(X̄i2 ) = σ 2 + µ2 Now hX i hX i 2 E (Xi − X̄) = E Xi2 − nX̄ 2 X = E(Xi2 ) − nE(X̄)2 2 σ = nσ 2 + nµ2 − n + µ2 n = nσ + nµ − σ − nµ2 2 2 2 = (n − 1)σ 2 P E (Xi − X̄)2 ∴ σ2 = n−1 53 2.2 Maximum Likelihood Estimation 2 STATISTICS 2.2 Maximum Likelihood Estimation The Maximum Likelihood Estimation (MLE) is a sta- tistical method used for fitting data to a model (Data analysis). We are asking the question: ”Given the set of data, what model parameters is most likely to give this data?” MLE is well defined for the standard distributions, however in complex problems, the MLE may be unsuit- able or even fail to exist. Note:When using the MLE model we must first as- sume a distribution, i.e. a parametric model, after which we can try to determine the model parameters. 2.2.1 Motivating example Consider data from a Binomial distribution with random variable X and parameters n = 10 and p = p0. The parameter p0 is fixed and unknown to us. That is: 10 f (x; p0 ) = P (X = x) = P0x (1 − p0 )10−x x Now suppose we observe some data X = 3. Our goal is to estimate the actual parameter value p0 based on the data. 54 2.2 Maximum Likelihood Estimation 2 STATISTICS Thought Experiments: let us assume p0 = 0.5, so probability of generating the data we saw is f (3; 0.5) = P (X = 3) 10 = (0.5)3 (0.5)7 3 ≈ 0.117 Not very high ! How about p0 = 0.4, again f (3; 0.4) = P (X = 3) 10 = (0.4)3 (0.6)7 3 ≈ 0.215 better...... So in general let p0 = p and we want to maximise f (3; p), i.e. 10 f (3; p) = P (X = 3) = P 3 (1 − p)7 3 Let us define a new function called the likelihood func- tion `(p; 3) such that `(p; 3) = f (3; p). Now we want to maximise this function. Maximising this function is the same as maximising the log of this function (we will explain why we do this 55 2.2 Maximum Likelihood Estimation 2 STATISTICS later!), so let L(p; 3) = log `(p; 3) therefore, 10 L(p; 3) = 3 log p + 7 log(1 − p) + log 3 dL To maximise we need to find dp =0 dL = 0 dp 3 7 − = 0 p 1−p 3(1 − p) − 7p = 0 3 p = 10 3 Thus the value of p0 that maximises L(p; 3) is p = 10. This is called the Maximum Likelihood estimate of p0. 2.2.2 In General If we have n pieces of iid data x1 , x2 , x3 ,....xn with prob- ability density (or mass) function f (x1 , x2 , x3 ,....xn ; θ), where θ are the unknown parameter(s). Then the Max- imum likelihood function is defined as `(θ; x1 , x2 , x3 ,....xn ) = f (x1 , x2 , x3 ,....xn ; θ) and the log-likelihood function can be defined as 56 2.2 Maximum Likelihood Estimation 2 STATISTICS L(θ; x1 , x2 , x3 ,....xn ) = log `(θ; x1 , x2 , x3 ,....xn ) Where the maximum likelihood estimate of the param- eter(s) θ0 can be obtained by maximising L(θ; x1 , x2 , x3 ,....xn ) 2.2.3 Normal Distribution Consider a random variable X such that X ∼ N (µ, σ 2 ). Let x1 , x2 , x3 ,....xn be a random sample of iid observa- tions. To find the maximum likelihood estimators of µ and σ 2 we need to maximise the log-likelihood function. f (x1 , x2 , x3 ,....xn ; µ, σ) = f (x1 ; µ, σ).f (x2 ; µ, σ).......f (xn ; µ, σ) `(µ, σ; x1 , x2 , x3 ,....xn ) = f (x1 ; µ, σ).f (x2 ; µ, σ).......f (xn ; µ, σ) ∴ L(µ, σ; x1 , x2 , x3 ,....xn ) = log `(µ, σ; x1 , x2 , x3 ,....xn ) = log f (x1 ; µ, σ) + log f (x2 ; µ, σ) +..... + log f (xn ; µ n X = logf (xi ; µ, σ) i=1 For the Normal distribution 1 − (x−µ) 2 f (x; µ, σ) = √ e 2σ 2 σ 2π 57 2.2 Maximum Likelihood Estimation 2 STATISTICS so " n # X 1 (x −µ)2 − i2σ2 L(µ, σ; x1 , x2 , x3 ,....xn ) = log √ e i=1 σ 2π n n 1 X = − log(2π) − n log(σ) − 2 (xi − µ)2 2 2σ i=1 To maximise we differentiate partially with respect to µ and σ set the derivatives to zero and solve. If we were to do this, we would get: n 1X µ= xi n i=1 and n 2 1X σ = (xi − µ)2 n i=1 58 2.3 Regression and Correlation 2 STATISTICS 2.3 Regression and Correlation 2.3.1 Linear regression We are often interested in looking at the relationship be- tween two variables (bivariate data). If we can model this relationship then we can use our model to make pre- dictions. A sensible first step would be to plot the data on a scatter diagram, i.e. pairs of values (xi , yi ) Now we can try to fit a straight line through the data. We would like to fit the straight line so as to minimise the sum of the squared distances of the points from the line. The different between the data value and the fitted line is called the residual or error and the technique of often referred to as the method of least squares. 59 2.3 Regression and Correlation 2 STATISTICS If the equation of the line is given by y = bx + a then the error in y, i..e the residual of the ith data point (xi , yi ) would be ri = yi − y = yi − (bxi + a) We want to minimise n=∞ 2 P n=1 ri , i.e. n=∞ X n=∞ X S.R = ri2 = [yi − (bxi + a)]2 n=1 n=1 Pn=∞ We want to find the b and a that minimise n=1 ri2. X yi2 − 2yi (bxi + a) + (bxi + a)2 S.R = X yi2 − 2byi xi − 2ayi + b2 x2i + 2baxi + a2 = or = ny¯2 − 2bnxy¯ − 2anȳ + b2 nx¯2 + 2banx̄ + na2 60 2.3 Regression and Correlation 2 STATISTICS To minimise, we want ∂(S.R) (i) ∂b =0 ∂(S.R) (ii) ∂a =0 (i) ∂(S.R) ¯ + 2bnx¯2 + 2anx̄ = 0 = −2nxy ∂b (ii) ∂(S.R) = −2nȳ + 2bnx̄ + 2an = 0 ∂a These are linear simultaneous equations in b and a and can be solved to get Sxy b= Sxx where (xi )2 X X P Sxx = (xi − x̄)2 = 2 (xi ) − n and P P X X ( xi )( yi ) Sxy = (xi − x̄)(yi − ȳ) = x i yi − n a = ȳ − bx̄ Example x 5 10 15 20 25 30 35 40 y 98 90 81 66 61 47 39 34 X X X X X xi = 180 yi = 516 x2i = 5100 yi2 = 37228 xi yi = 9585 61 2.3 Regression and Correlation 2 STATISTICS 180 × 516 Sxy = 9585 − = −2025 8 1802 Sxx = 5100 − = 1050 8 −2025 ∴b= = −1.929 1050 180 516 x̄ = = 22.5 ȳ = = 64.5 8 8 ∴ a = 64.5 − (−1.929 × 22.5) = 107.9 i.e. y = −1.929x + 107.9 62 2.3 Regression and Correlation 2 STATISTICS 2.3.2 Correlation A measure of how two variables are dependent is their correlation. When viewing scatter graphs we can often determine if their is any correlation by sight, e.g. 63 2.3 Regression and Correlation 2 STATISTICS It is often advantageous to try to quantify the corre- lation between between two variables, this can be done in a number of ways, two such methods are described. 2.3.3 Pearson Product-Moment Corre- lation Coefficient A measure often used within statistics to quantify this is the Pearson product-moment correlation coeffi- cient. This correlation coefficient is a measure of linear dependence between two variables, giving a value be- tween +1 and −1. Sxy PMCC r = p Sxx Syy Example Consider the previous example, i.e. x 5 10 15 20 25 30 35 40 y 98 90 81 66 61 47 39 34 We calculated, 64 2.3 Regression and Correlation 2 STATISTICS Sxy = −2025 and Sxx = 1050 also, (yi )2 X X P 2 Syy = (yi − ȳ) = (yi2 ) − n i.e 5162 Syy = 37228 − = 3946 8 therefore, −2025 r=√ = −0.995 1050 × 3946 This shows a strong negative correlation and if we were to plot this using a scatter diagram, we can see this vi- sually. 2.3.4 Spearman’s Rank Correlation Co- efficient Another method of measuring the relationship between two variables is to use the Spearman’s rank corre- 65 2.3 Regression and Correlation 2 STATISTICS lation coeffieint. Instead of dealing with the values of the variables as in the product moment correlation coefficient, we assign a number (rank) to each variable. We then calculate a correlation coefficient based on the ranks. The calculated value is called the Spearmans Rank Correlation Coefficient, rs , and is an approxima- tion to the PMCC. 6 d2i P rs = 1 − n(n2 − 1) where d is the difference in ranks and n is the number of pairs. Example Consider two judges who score a dancing championship and are tasked with ranking the competitors in order. The following table shows the ranking that the judges gave the competitors. Competitor A B C D E F G H JudgeX 3 1 6 7 5 4 8 2 JudgeY 2 1 5 8 4 3 7 6 calculating d2 , we get dif f erence d 1 0 1 1 1 1 1 4 dif f erence2 d2 1 0 1 1 1 1 1 16 X ∴ d2i = 22 and n = 8 6 × 22 rs = 1 − = 0.738 8(82 − 1) 66 2.4 Time Series 2 STATISTICS i.e. strong positive correlation 2.4 Time Series A time series is a sequence of data points, measured typi- cally at successive times spaced at uniform time intervals. Examples of time series are the daily closing value of the Dow Jones index or the annual flow volume of the Nile River at Aswan. Time series analysis comprises methods for analyzing time series data in order to extract meaningful statistics and other characteristics of the data. Two methods for modeling time series data are (i) Moving average models (MA) and (ii) Autoregressive models. 2.4.1 Moving Average The moving average model is a common approach to modeling univariate data. Moving averages smooth the 67 2.4 Time Series 2 STATISTICS price data to form a trend following indicator. They do not predict price direction, but rather define the current direction with a lag. Moving averages lag because they are based on past prices. Despite this lag, moving averages help smooth price action and filter out the noise. The two most pop- ular types of moving averages are the Simple Moving Average (SMA) and the Exponential Moving Average (EMA). Simple moving average A simple moving average is formed by computing the average over a specific number of periods. Consider a 5-day simple moving average for closing prices of a stock. This is the five day sum of closing prices divided by five. As its name implies, a moving average is an average that moves. Old data is dropped as new data comes available. This causes the average to move along the time scale. Below is an example of a 5-day moving average evolving over three days. The first day of the moving average simply covers the 68 2.4 Time Series 2 STATISTICS last five days. The second day of the moving average drops the first data point (11) and adds the new data point (16). The third day of the moving average contin- ues by dropping the first data point (12) and adding the new data point (17). In the example above, prices grad- ually increase from 11 to 17 over a total of seven days. Notice that the moving average also rises from 13 to 15 over a three day calculation period. Also notice that each moving average value is just below the last price. For example, the moving average for day one equals 13 and the last price is 15. Prices the prior four days were lower and this causes the moving average to lag. Exponential moving average Exponential moving averages reduce the lag by apply- ing more weight to recent prices. The weighting applied to the most recent price depends on the number of pe- riods in the moving average. There are three steps to calculating an exponential moving average. First, calcu- late the simple moving average. An exponential moving average (EMA) has to start somewhere so a simple mov- ing average is used as the previous period’s EMA in the first calculation. Second, calculate the weighting multi- plier. Third, calculate the exponential moving average. The formula below is for a 10-day E. Ei+1 = 2−(n+1) (Pi+1 − Ei ) + Ei 69 2.4 Time Series 2 STATISTICS A 10-period exponential moving average applies an 18.18% weighting to the most recent price. A 10-period EMA can also be called an 18.18% EMA. A 20-period EMA applies a 9.52% weighing to the 2 most recent price 20+1 =.0952. Notice that the weight- ing for the shorter time period is more than the weighting for the longer time period. In fact, the weighting drops by half every time the moving average period doubles. 70 2.4 Time Series 2 STATISTICS 2.4.2 Autoregressive models Autoregressive models are models that describe random processes (denote here as et ) that can be described by a weighted sum of its previous values and a white noise error. An AR(1) process is a first-order one process, meaning that only the immediately previous value has a direct effect on the current value et = ret−1 + ut where r is a constant that has absolute value less than one, and ut is a white noise process drawn from a distri- 71 2.4 Time Series 2 STATISTICS bution with mean zero and finite variance, often a normal distribution. An AR(2) would have the form et = r1 et−1 + r2 et−2 + ut and so on. In theory a process might be represented by an AR(∞). 72