Component Life Time - PDF
Document Details
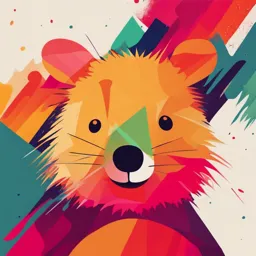
Uploaded by FortunateRisingAction
Edith Cowan University
Dr Ana Vafadar
Tags
Summary
This document is a lecture about Principles of Industrial Maintenance. Topics covered include reliability, failure rates, and component life.
Full Transcript
Course materials provided for personal study only and subject to the disclaimer on the ECU website http://www.ecu.edu.au/supplemental/disclaimer Principles of Industrial Maintenance...
Course materials provided for personal study only and subject to the disclaimer on the ECU website http://www.ecu.edu.au/supplemental/disclaimer Principles of Industrial Maintenance Lecturer: Dr Ana Vafadar [email protected] This lecture Coveragein this lecture will be largely derived from … Stephens, M. P. (2010) Productivity and reliability-based maintenance management. New Jersey: Pearson Education, Inc. (ISBN 9781557535924). Content from other sources will be indicated where applicable. This lecture – the plan Thislecture material is largely based on … Statistical Applications (CHP 2) (Stephens, 2010) 2.1 Reliability 2.2 System Reliability 2.2.1 Series Systems 2.3 Failure Rate 2.3.1 Determining the Failure Rate 2.4 Mean Time Between Failures, Availability, and Mean Downtime 2.4.1 MTBF Defined 2.4.2 Calculating MTBF 2.5 Improving System Reliability 2.5.1 Parallel Systems 2.5.2 Combinations of Series and Parallel Systems 2.6 Equipment Life Cycle Failure Rate 2.6.1 Infant Mortality (in equipment) 2.6.2 Chance Failure 2.6.3 Wear-out Phase 2.7 Exponential Probability Distribution This lecture in a nutshell This lecture will basically … Introduce the concept of unit reliability Identify how reliability changes based on: System configuration: series versus parallel Length of operation Define some key lifetime parameters relevant to maintenance (including) Failure rate Mean Time Between Failure Identify typical trends and stages in the life of a component Identify some basic statistical distributions and highlight which of these is applicable during the useful life time of a product Component Life Time Slide 5 Defining Reliability Component life times are intrinsically linked to the concept of reliability. Lets take a closer look at one definition for reliability Reliability: “Equipment reliability is the probability that equipment, machinery, or systems will perform required functions satisfactorily under specific conditions within a certain period of time. It can also be thought of as the likelihood that problems (quality defects and breakdowns) will not occur over a given period.” (Nakajima, 1989, pg 49) Reliability is therefore intrinsically linked to … The likelihood of remaining functional Stated probabilities which relate to a specific period of time in the product life Nakajima, S. (ed.) (1989). TPM development program: implementing total productive maintenance. Cambridge, USA: Productivity Press, Inc. Principles of Industrial Maintenance Edith Cowan University 2018 Component Life Time Slide 6 Defining Reliability Reliability can be defined as some level of … ‘expectation’ or ‘likelihood/probability’ … that systems (and their components) will be able to deliver their stated functional requirements Reliability can also be thought of as the chance that some process will remain functional … at some instant in time … under specific operating conditions System reliability is therefore affected by individual component life expectancy and maintenance program (and its effectiveness). System reliability will change with the progress of time. Principles of Industrial Maintenance Edith Cowan University 2018 Component Life Time Slide 7 Defining Reliability Nakajima breaks divides the factors affecting reliability into a number of forms (1989, pgs 49-50): Maintenance reliability Poor reliability as a consequence of errors associated with maintenance Design reliability Through inaccurately designed jigs or selecting sub-assemblies (components) with short lifetimes, design reliability is also affected Fabrication reliability Exemplified by poor accuracy in dimensions (size/tolerance), geometry (shape) or assembly Installation reliability This is primarily a consequence of installation errors that lead to poor operational characteristics (e.g., excessive noise or vibration) Operation and manipulation reliability Errors during set-up, adjustment and inconsistent operational conditions contribute here Principles of Industrial Maintenance Edith Cowan University 2018 Component Life Time Slide 8 Component Versus System Reliability Systems are constructed out of multiple smaller components (e.g, machines) Machines (in turn) are made up of smaller sub-components Overall system reliability is affected by … The number of components forming these systems (1, 2, 3, … etc) The reliability associated with each system component (R 1, R2, R3, …etc) Configuration of components Series arrangements (below left) Parallel arrangements (below right) Combined parallel and series R1 R1 R2 R2 Principles of Industrial Maintenance Edith Cowan University 2018 Component Life Time Slide 9 System Reliability Series Configurations R1 Rn Series reliability (RS) is obtained by the product of reliabilities for 100% 90% individual components (it is assumed System Reliability 80% n=1 1part at that the reliability of individual 70% R=98% n=2 60% components is independent of that for 50% n = 10 n = 50 others) 40% 30% n = 100 RS R1 R2 ... Rn 20% n = 200 10% Overall system reliability decreases in 0% 100% 99% 98% 97% 96% 95% 94% 93% this type of configuration compared to Component Reliability the (maximum) reliability possible for any single component When more components are arranged in series, This effect is more pronounced as the even if units each have relatively high reliability (e.g., 97%), overall system reliability decreases drastically number of components increases as the number of units (n) increases. A component failing in a series configuration renders the system Source: Figure 2-2. Stephens, Matthew P. (2004) Productivity and reliability- inoperative (no redundancy) based maintenance management. New Jersey, USA: Pearson Education, Inc. Principles of Industrial Maintenance Edith Cowan University 2018 Component Life Time Slide 10 System Reliability Series Configurations EXAMPLE Adapted from Stephens (2004, pg 27) Given A device requires all four interlocks (switches) to be closed for it to operate. At a specific point in the lifetime of the device, the operational reliability (readiness to work) for each of the four interlocks is given as 0.85, 0.91, 0.90 and 0.96, respectively. Task Establish the overall reliability for this device (considering these four components only). RS R1 R2 ... Rn RS 0.85 0.91 0.90 0.96 RS 0.67 This indicates that device is probably going to be available only 67% of the time (or a failure likelihood of about 33%) Note how parts are treated here as being related in series (even though it is not mentioned in the problem). This is because they all need to be operational for the device to work. Principles of Industrial Maintenance Edith Cowan University 2018 Component Life Time Slide 11 System Reliability Parallel Configurations When system components are related to each other in parallel associations, overall (parallel) system reliability (R P) is calculated as: R1 RP 1 (1 R1 )(1 R2 )...(1 Rn ) R2 Overall system reliability increases (with more components) compared to the (maximum) reliability possible for individual components Note how there is the potential to direct work to alternate pathways (assuming excess capacity exists) The presence of redundancy means that for the overall system to fail completely, all its components must have failed Principles of Industrial Maintenance Edith Cowan University 2018 Component Life Time Slide 12 System Reliability Parallel Configurations EXAMPLE Given Two conveyors located side-by-side are used in materials handling. Each conveyor is (only) used to half its capacity (at any moment). One of the conveyors is older and has a reliability of ~0.83 while the other’s reliability is ~0.92. Task Establish the overall reliability for this materials handling system. RP 1 (1 R1 )(1 R2 )...(1 Rn ) RP 1 (1 0.83)(1 0.92) RP 0.99 This indicates the system is probably going to be available 99% of the time (or a failure likelihood of about 1%) Principles of Industrial Maintenance Edith Cowan University 2018 Component Life Time Slide 13 SystemReliability Combined Parallel and Series Configurations More complicated systems (not simply series or parallel) can be broken down into sub-sets of series and parallel configurations. EXAMPLE Adapted from Stephens (pg 32) Task R=0.90 Establish the overall reliability of R=0.75 the system shown. R=0.80 R=0.90 RP1 1 (1 0.75)(1 0.85) R=0.85 RP1 0.9625 R=0.70 RP 2 1 (1 0.90)(1 0.80)(1 0.70) RP 2 0.994 parallel group1 parallel group2 RS 0.9625 0.994 0.90 RS 0.86 series (group 1 and group 2 combined) Principles of Industrial Maintenance Edith Cowan University 2018 Quiz Slide 14 Please calculate the overall system reliability for the below configuration. R=0.70 R=0.75 R=0.85 R=0.70 R=0.80 Principles of Industrial Maintenance Edith Cowan University 2018 Component Life Time Slide 15 Failure Rates: Parameters A failure rate () is a measure for the lack of reliability … just as a high level of reliability signals a low failure rate R 1 The failure rate itself can be derived from machine history data if the number of failures occurring over a specific time period is known. It can also be determined by life (reliability) testing. A definition of failure rate is: Number of Failures failure hour Length of Operating Hours What parameters can be used to The length of operational time (accumulated hours) for characterise the a given number of failures to occur changes through the time associated with failure? life cycle of the product (as it gets older). This means that reliability also changes with time Principles of Industrial Maintenance Edith Cowan University 2018 Component Life Time Slide 16 FailureRates: Parameters Mean Time Between Failures (MTBF) Expressed in time units, it represents the expected (on average) time period at which equipment will likely fail. If the MTBF is small number it means that the frequency of failures (or the failure rate) is significant. EXAMPLE Adapted from Stephens (2004, pg 29) Given Seven units of the same product are tested over an 80hr period. Four units remain working for 80hrs with the rest failing after 15, 35, and 60hrs. Task Establish the failure rate (), reliability (R) and MTBF for this product in the first 80hrs of operation. Number of Failures 1 Length of Operating Hours R 1 0.993 MTBF λ 3 failure λ 0.007 MTBF 142.85hrs 15 35 60 4 80 hr Principles of Industrial Maintenance Edith Cowan University 2018 Component Life Time Slide 17 Failure Rates: Parameters Mean Time To Failure (MTTF) MTTF is different to MTBF. The term MTTF is applicable to components that once failed, cannot be brought back into an acceptable operational condition (i.e., disposable items). For non-repairable items, it equates to the life expectancy Mean Down Time (MDT) Total time needed to bring failed equipment back into an acceptable operational state The following contribute to the length of MDT: Time needed to issue the (maintenance) work order PLUS Time for arrival of maintenance crew at the failed equipment PLUS Time for completing all maintenance tasks and the checks needed to ensure the equipment has indeed been returned to an operational status Availability Availabili ty MTBF MTBF MDT Proportion of time over which equipment is available for use Principles of Industrial Maintenance Edith Cowan University 2018 Component Life Time Slide 18 Failure Rates: Time Dependence Failure rate largely changes during lifetime (accumulated operational time since initial commissioning) and typically follows a “bath-tub” trend. early chance wear-out failures failures failures Principles of Industrial Maintenance Edith Cowan University 2018 Component Life Time Slide 19 FailureRates: Time Dependence Early Failures 1 Unit failures during this phase are also termed “initial failures” or “early time failures” (Harrington, 1999, pg 82). The term “infant mortality” also applies to this stage. Failures during this phase are largely due to manufacturing and design flaws as well as incorrect installation. Product defects that are not picked up before shipment (to the user) manifest themselves at this stage. Example: In a diesel engine, this phase may be responsible for the first 1,000hrs of usage. Principles of Industrial Maintenance Edith Cowan University 2018 Component Life Time Slide 20 Failure Rates: Time Dependence Chance Failures 2 Also termed “random failures”, this essentially represents the normal operating period (Harrington, 1999, pg 82) or productive phase of the life cycle. Failures occur randomly (in units) but at an almost constant rate. The effectiveness of preventive maintenance is a significant influencing factor on the failure rate during this stage. MTBF data can be calculated here. Principles of Industrial Maintenance Edith Cowan University 2018 Component Life Time Slide 21 FailureRates: Time Dependence Wear-Out Failure 3 Superimposed on causes for chance failures, units now also undergo a physical wear-out that leads to increased failure rates (Harrington, 1999, pg 83). Equipment reaching this stage is quite depreciated and likely to be replaced. Subsequent use for equipment arriving into this (advanced) phase might include retaining these for back-up (redundant) capacity or for providing spare parts. Principles of Industrial Maintenance Edith Cowan University 2018 Component Life Time Slide 22 Failure Rates: Time Dependence Deviations in Failure Rates – the Bath-Tub Curve Failure rate trends for different systems may vary from the ‘bath-tub’ shape as may the trend for individual components that form part of a bigger system. To exemplify, the following might apply to components on a diesel engine. Source: Figure 2-7. Stephens, Matthew P. (2004) Productivity and reliability-based maintenance management. New Jersey: Pearson Education, Inc. Principles of Industrial Maintenance Edith Cowan University 2018 Component Life Time Slide 23 Failure Rates: Time Dependence Mean Time Between Failures (MTBF) Caution ! If the MTBF is calculated in a period where wear-out failures are starting to occur (i.e., the failure rate is not fairly constant but changing), the value of this MTBF will also vary (with time). Calculating the MTBF in this manner can be considered an inaccurate (oversimplification) of use. MTBF Principles of Industrial Maintenance Edith Cowan University 2018 Component Life Time Slide 24 Failure Rates: Time Dependence Mean Time Between Failures (MTBF)... during wear-out ! EXAMPLE Adapted from Harrington (1999, pg 119) Given A total of 10 batteries were applied to generator sets. A survey revealed no batteries failed over the first 24 months. Beyond that period, the following failure data was recorded: Number of Failed Batteries in Month Month of Failure___________ 30 months 1 27 40 months 2 38 50 months 1 47 1 48 2 51 52 months 3 52 Task Show that the MTBF for this batch of 10 batteries varies over the test period. Base your MTBF calculations on the first 50months or 52 months of operation. Principles of Industrial Maintenance Edith Cowan University 2018 Component Life Time Slide 25 FailureRates: Time Dependence Mean Time Between Failures (MTBF)... during wear-out ! … example continued: Total time (5x50) represents the Length of Operating Hours MTBF remaining items which have not yet failed at the Total end of the 50hr period. Number of Failures failures MTBF over 50 months 2 months difference only ! MTBF over 52 months MTBF 27 47 48 2 38 5 50 27 47 48 2 38 2 51 3 52 MTBF 5 10 Number of fails in period. MTBF 89.6months MTBF 45.6months Note: If other MTBF calculations are done for usage over 30months and 40months these will show MTBF equal to 297months and 127.6months, respectively. The above demonstrates how significantly MTBF changes for a batch of similar products as they progress into the wear-out phase. MTBF at such late stages of the life cycle is very unlike the MTBF when failure rates are fairly constant. Caution must be exercised when interpreting MTBF (if the data used to extract it does not pertain to middle portion of the bath-tub curve). Principles of Industrial Maintenance Edith Cowan University 2018 Component Life Time Slide 26 Failure Rates: Time Dependence Deviations in Failure Rates – Overall Trends (Harrington, 1999, pp84-87) Lets look at some variations of failure rate trends over time. Failure rate here is taken as the “.. ratio of failures (in time) to the initial amount of items”. Products with ‘infinite’ lifetimes Products that essentially do not move into a wear-out phase within the useful lifetime of a product like some electronics. Adapted from Fig 4-2: Harrington & Anderson (1999) Harrington, H. J., & Anderson, L. C. (1999). Reliability simplified: going beyond quality to keep customers for life. New-York: The McGraw-Hill Co., Inc. Principles of Industrial Maintenance Edith Cowan University 2018 Component Life Time Slide 27 Failure Rates: Time Dependence Deviations in Failure Rates – Overall Trends (Harrington, 1999, pp84-87) Products with an inherent (designed) wear-out Products such as incandescent light bulbs that attain wear-out after a designated period of operation (e.g., 1,000-1,500 hrs). Recall the battery example and how the MTBF changed drastically within the wear-out period. Adapted from Fig 4-3: Harrington & Anderson (1999) Harrington, H. J., & Anderson, L. C. (1999). Reliability simplified: going beyond quality to keep customers for life. New-York: The McGraw-Hill Co., Inc. Principles of Industrial Maintenance Edith Cowan University 2018 Component Life Time Slide 28 Failure Rates: Time Dependence Deviations in Failure Rates – Overall Trends (Harrington, 1999, pp84-87) Products where are all efforts are done to prevent failure Products requiring an outstanding safety record might be listed in this category. Stringent maintenance and inspection helps achieve this. Redundant systems and back-up capacity exists to prevent any chance overall system failure. No wear-out phase is allowed to creep into the service providing period (e.g., as in aircraft). Failures that occur are due to ‘incidents’ rather than due to ‘normal’ failure rates. Adapted from Fig 4-4: Harrington & Anderson (1999) Harrington, H. J., & Anderson, L. C. (1999). Reliability simplified: going beyond quality to keep customers for life. New-York: The McGraw-Hill Co., Inc. Principles of Industrial Maintenance Edith Cowan University 2018 Quiz Slide 29 Which of the following item(s) is not correct? o For most systems, failure occurs at during the product life. o The effectiveness of preventive maintenance is a significant influencing factor on the failure rate during wear out stage. o The term MTBF is applicable to components that once failed, cannot be operated again. o A failure rate () is a measure for the lack of reliability. oEquipment reliability changes with time. Principles of Industrial Maintenance Edith Cowan University 2018 Component Life Time Slide 30 Product Reliability: Exponential Distribution Many similar products will exhibit a fairly constant failure rate () during the productive phase of their lifetime. The exponential probability distribution has been found to lend itself to reliability analyses during this phase. This statistical approach to analysing data can also be known as: the exponential distribution of reliability the exponential distribution of failures or simply the exponential distribution Principles of Industrial Maintenance Edith Cowan University 2018 Component Life Time Slide 31 Failure Rate Variation with Time Normal lifetime distribution (Cox and Tait, 1998, pp 15-18) late failures Lifetime histogram Failure density function @1400- 1600hrs Fig a Fig b mean 22/417=0.0528 failure 0.0528/25=0.0021 ~ 22 early failures @200- 400hrs Hazard or failure rate Reliability Fig c Fig d Adapted from Fig 2.3: Cox and Tait (1998) Cox, S. & Tait, R. (1998). Safety, reliability and risk management: an integrates approach (2 nd ed). Oxford: Butterworth-Heinemann Principles of Industrial Maintenance Edith Cowan University 2018 Component Life Time Slide 32 Failure Rate Variation with Time Not all items follow exponential distributions (of failure) throughout their lifetime. Lets look at two types of distribution (normal and exponential). Normal lifetime distribution (Cox and Tait, 1998, pp 15-18) Example: To highlight the case of a normal probability (lifetime) distribution take the example of 417 like units of a single product which are tested (e.g., 40W light bulbs). All units are new (unused) at time=0hrs. Testing then commences and the number of failed units in every 25hr slot is counted. Refer to Figures a, b, c and d. Figure a: the height of each grey coloured column refers to the number of failed units in every 25hr slot (a tally of failed units in that time period). This figure can be called a lifetime histogram. The data represented by column heights shows fluctuations (some columns are taller than others). However, the entire trend can be smoothed into a curve. Smoother curves can be obtained by increasing the number of tested units. The shape of this trend line can be called a ‘normal’ curve. It is symmetrical about the peak (max) lifetime of 22failures and is centred at ~1050hrs. Cox, S. & Tait, R. (1998). Safety, reliability and risk management: an integrates approach (2 nd ed). Oxford: Butterworth-Heinemann Principles of Industrial Maintenance Edith Cowan University 2018 Component Life Time Slide 33 FailureRate Variation with Time Normal lifetime distribution (Cox and Tait, 1998, pp 15-18) Figure b: lets consider one way to account for the size of the test set. This will be based on the original or starting number of units (i.e., 417). One way of normalising for (i.e., mathematically excluding the effect of) the size of the test set is to divide the number of failed units (in each time interval, 25hr slot) by the total size of the test set at t=0hrs (417 units). This gives a new trend (shown in red). The shape of this curve (Figure b) does not change from the normal curve (Figure a) but each point that falls on this line now takes on a different value. The values have changed (from those in Figure a) since all the values are being scaled (divided) by a constant (i.e., division by 417). The peak value (at 1050hrs) now becomes 22/417=0.0528 (per 25hr time slot). If we again divide the peak value of (0.0528) by 25 (the length of the time slot) we are able to obtain the number of failures for every hour rather than every 25hr slot. This value is about 0.0021. The corresponding curve (in Figure b) is called the ‘failure density function’ f(t). Note that because we divided by the size of the original test set (417), the curve therefore represents the failure rates … but … relative to the starting number of samples (417 units). Principles of Industrial Maintenance Edith Cowan University 2018 Component Life Time Slide 34 Failure Rate Variation with Time Normal lifetime distribution (Cox and Tait, 1998, pp 15-18) Figure c: lets consider a second way to account for the size of the test set. This will be based on the remaining units (not to the original number). The failure rate calculated in this manner will also have to be valid at any instant in time throughout the operational life. Why do we need to do this? Lets say that after 825hrs (Figure a) the number of failed units (in a single 25hr slot) is 10. We have already said that the (normal distribution) is fairly symmetrical about the mean value (1050hrs in this example). Note that 825hrs is 225hrs from (short of) 1050hrs. Similarly, 1275hrs is 225hrs (longer than) from 1050hrs. The number of failed units at 1275hrs will also probably be 10 units. Lets assume the operational units (those which have not yet failed) at 825hrs is 360units (out of the original 417). This is much larger compared to the number after 1275hrs (assume this to be a total of 55 units at 1275hrs). This means that (at 1275hrs) 10 units failing out of 55 units (for a 25hr slot) gives a higher failure rate compared to (at 825hrs) 10 units failing out of 360 units (for a 25hr slot). Plotting these failure rates (and also divided by 25hrs) results in a curve called the ‘hazard rate’ h(t) or ‘failure rate’. We see that the trend reflects the above description in that failure rates increase with time. (compare the failure rate at 825hrs with another at 1275hrs). Principles of Industrial Maintenance Edith Cowan University 2018 Component Life Time Slide 35 FailureRate Variation with Time Normal lifetime distribution (Cox and Tait, 1998, pp 15-18) Figure d: the failure density function (Figure b) as well as the hazard or failure rate (Figure c) are expressed in terms of the number of items failing per time interval. We can use this data to define a dimensionless expression for Reliability. This will be expressed as a probability with values ranging from a minimum 0.00 (i.e., 0%) to a maximum 1.00 (i.e., 100%). Reliability: a fractional value representing the number of items remaining relative to the total number (at any instant in time) At t=0, reliability is R(0)=1.00 … i.e., all units operational (100%) At t=1050hrs, reliability is R(1050)=0.50 … i.e., half units operational (50%) For a normal distribution (Figure a), the calculated hazard or failure rate (Figure c) increases with time. It is now evident why the normal distribution is not taken as representative of the middle phase of a (typical) bath-tub curve. The failure rate in the central part of the bath-tub curve was not increasing (or decreasing) but fairly constant during that phase. This does not happen here (Figure c). Principles of Industrial Maintenance Edith Cowan University 2018 Component Life Time Slide 36 Failure Rate Variation with Time Exponential lifetime distribution (Cox and Tait, 1998, pp 18-21) Lifetime histogram Failure density function Fig e Fig f early late failures failures Hazard or failure rate Reliability Fig g Fig h Adapted from Fig 2.4: Cox and Tait (1998) Cox, S. & Tait, R. (1998). Safety, reliability and risk management: an integrates approach (2 nd ed). Oxford: Butterworth-Heinemann Principles of Industrial Maintenance Edith Cowan University 2018 Component Life Time Slide 37 Failure Rate Variation with Time Exponential lifetime distribution (Cox and Tait, 1998, pp 18-21) Example: To highlight the case of exponential probability (lifetime) distributions take the example of 903 like units of a single product. All these undergo failure testing. Units are new (unused) at time=0hrs. Testing then commences and the number of failed units in every 20hr slot is counted. All units are found to fail after 1000hrs of testing. Refer to Figures e, f, g and h Figure e: once again, the height of each grey coloured column refers to the number of failed units in every time slot (20hrs in this example). The resulting figure is also called a lifetime histogram. The data in this figure (represented by column heights) shows fluctuations but the entire trends can be smoothed into a curve. Smoother curves can be obtained by increasing the number of tested units. The shape of this trend line can be called an ‘exponential’ curve Cox, S. & Tait, R. (1998). Safety, reliability and risk management: an integrates approach (2 nd ed). Oxford: Butterworth-Heinemann Principles of Industrial Maintenance Edith Cowan University 2018 Component Life Time Slide 38 Failure Rate Variation with Time Exponential lifetime distribution (Cox and Tait, 1998, pp 18-21) Figure f: the failure density function is also found by taking the number of failed units in each test period (20hrs), divided by the time interval (20hrs) and the original size of the test set (903 units). So, if 100 units fail in the first 20 hrs of testing, the failure density function at 20hrs is … f(20hrs)=100/(20*903)=0.0056 failures/hr Figure g: the hazard rate or failure rate takes on a constant value. This means “… the likelihood of each individual sample failing in a particular time interval does not depend on how long the sample has been running” (Cox and Tait, 1998, pg 20). Another way of saying this is h(t)=. The hazard rate is the reciprocal of the mean sample life (1/0.0056=179hrs) The shape of the reliability curve is the same as the failure density function The hazard rate (at operational time=t) is considered the probability of an item failing in the next time interval given that it is functional at the start of that interval. It is a conditional probability (Jardine & Tsang, 2006). Jardine, A. K. S. & Tsang, A. H. C. (2006). Maintenance, replacement, and reliability: theory and applications. Boca Raton (U.S): CRC Press. Principles of Industrial Maintenance Edith Cowan University 2018 Component Life Time Slide 39 ProductReliability: Exponential Distribution A summary of some basic parameters and relationships: 1. Product reliability can be expressed using a decimal fraction R(t), or S(t). This represents the number of surviving items to operational time (t). R(t) e λt R(t) e t MTBF λ failure rate Note that the formula R=1- is used to find the reliability (only) at the beginning of operation, or the first hour of operation where t=1. Beyond this time, reliability deteriorates with time and is then assessed using R=e-t. Principles of Industrial Maintenance Edith Cowan University 2018 Component Life Time Slide 40 Failure Rate Variation with Time For an exponential distribution (Figure e), the calculated hazard or failure rate (Figure g) is constant with time. This provides more clarity on why the exponential distribution is taken as representative of the middle phase of a (typical) bath-tub curve. Reliability “… reliability or survival function of the component at time t … gives the probability that the component survives upto time t with no failures.” (Zio, 2007, pg49) So, we have learned that reliability varies with operational time (Figure h). The same expressions for parallel and series reliability (expressed earlier in slides 9 and 11) can be given in more generalised terms, R(t) Series N N Parallel Rt Ri t Rt 1 1 Ri t i 1 i 1 Zio, E. (2007). An introduction to the basics of reliability and risk analysis. Singapore: World Scientific. Principles of Industrial Maintenance Edith Cowan University 2018 Component Life Time Slide 41 Product Reliability: Exponential Distribution A summary of some basic parameters and relationships: 2. MTBF (in the exponential distribution) will be the point in the lifetime where t=1. If we substitute this into the equation for reliability (R=e-t), we see that the corresponding reliability (at the MTBF) is about 37%. R(t) e λt R(t) e 1 R(t) 0.37 This infers that when the time of operation is equal to the MTBF, the expectation (or likelihood) is that 0.37 (or 37%) of the test units have survived to that point (or that about 63% of them will likely fail). MTBF is an estimate. It gives maintenance planners a ‘number’ to consider when attempting to plan preventive maintenance schedules (Stephens, 2010). If at a specific (desired) level of reliability (for example 70%) and failure rate (for example 0.015), the task is to estimate the new MTBF, this can be achieved by substituting the variables into the equation for reliability as follows: ln 0.70 Refer to additional R(t) e λt 0.70 e 0.015t ln 0.70 0.015t t 23.8hrs notes at the end of this lecture 0.015 Principles of Industrial Maintenance Edith Cowan University 2018 Component Life Time Slide 42 ProductReliability: Exponential Distribution A summary of some basic parameters and relationships: 3. The decimal fraction representing failing items to operational time (t). failures(t) 1 e λt Note how this fraction of failures (at time=t) when added to the fraction of surviving items needs to equal ‘unity’ (1) or 100%. The entire test is obviously broken down into (a fraction of) failing or surviving items. survivors(t) failures(t) 1 Principles of Industrial Maintenance Edith Cowan University 2018 Component Life Time Slide 43 ProductReliability: Exponential Distribution A summary of some basic parameters and relationships: Notes The larger the number of data samples used, the better confidence levels in the predictions (Harrington, 1999, pg 116). The exponential (probability) distribution is part of a broader type of statistical distribution called the Weibull distribution. Unlike the exponential distribution, Weibull distributions can lend themselves to increasing, decreasing or constant failure rates The exponential is only used with fixed failure rates Principles of Industrial Maintenance Edith Cowan University 2018 Component Life Time Slide 44 Product Reliability: Exponential Distribution EXAMPLE Adapted from Harrington (1999, pg 116) Given A set of 400 units (similar products) are found to have a failure rate of =0.0002 failure/hr. Task Predict how many units will fail in 500hrs of operation? R(t) e λt R( 500 ) e(0.0002500) 0.904 The above represents the (decimal) fraction for the predicted number of units to survive after 500 hours. This R(t) is then multiplied by the number of units at the start of this period (400). Surviving Units 0.904 400 362 Failing units (those requiring replacemen t) 400 362 38 Refer to additional notes at the end of this lecture Principles of Industrial Maintenance Edith Cowan University 2018 Component Life Time Slide 45 Product Reliability: Exponential Distribution EXAMPLE Adapted from Harrington (1999, pg 117) Given Field application of 50,000 similar units over a six month period showed that some 156 failed. On average, each failure was estimated to occur after 730hrs of operation. Task Predict the total number of units estimated to fail after 3,000 hours. It is unknown what the exact operational time for each failure was in the population of 50,000 units. This is needed to precisely calculate for the product set. Since the units that failed did so after an estimated 730hrs, the total operational time for 50,000 units will be estimated at 50,000units x 730 hrs. Number of Failures 156 failures 4.27 10 6 Length of Operating Hours 50,000 730 hr Note the exceedingly low failure rate (typical of some electronics). Principles of Industrial Maintenance Edith Cowan University 2018 Component Life Time Slide 46 ProductReliability: Exponential Distribution The next step in the solution is to identify a decimal fraction representing how many units are expected to survive after 3,000hrs at the failure rate λ 4.27 10 6 R(t) e λt (4.27106 failures/hr 3,000hrs) R(3,000) e 0.987 We then need to translate this decimal fraction (0.987) into the actual number of surviving units. This is done as follows: Surviving units (after 3,000 operationa l hours) 0.987 50,000 49,350 The number of failing units can then be predicted as: Failing units (after 3,000 operationa l hours) 50,000 49,350 650 Principles of Industrial Maintenance Edith Cowan University 2018 Component Life Time Slide 47 Thank you Principles of Industrial Maintenance Edith Cowan University 2018