Introduction To Pattern Recognition System PDF
Document Details
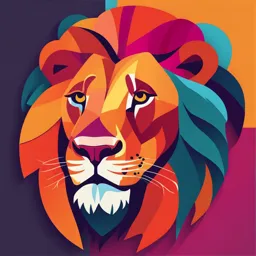
Uploaded by ComfortableUtopia9976
Tags
Summary
This document provides an introduction to pattern recognition systems. It covers the fundamental concepts, history, and various aspects of recognizing patterns in data. The document also discusses the different approaches and techniques involved in pattern recognition.
Full Transcript
Chapter one INTRODUCTION TO PATTERN RECOGNITION SYSTEM 1.1 Overview One of the most important capabilities of mankind is learning by experience, by our endeavors, by our faults. By the time we attain an age of five most of us are able to recognize digits, character...
Chapter one INTRODUCTION TO PATTERN RECOGNITION SYSTEM 1.1 Overview One of the most important capabilities of mankind is learning by experience, by our endeavors, by our faults. By the time we attain an age of five most of us are able to recognize digits, characters; whether it is big or small, uppercase or lowercase, rotated, tilted. We will be able to recognize, even if the character is on a mutilated paper, partially occluded or even on the clustered background. Looking at the history of the human search for knowledge, it is clear that humans are fascinated with recognizing patterns in nature, understand it, and attempt to relate patterns into a set of rules. But the question is how this experience can be used to make machines to learn. The most important challenge is how to generalize these experiences, how do we make decisions and how our experiences can be built into a machine? This has been one of the main fundamental principles behind the development of vast range of theories and concepts that are based on the natural world. Looking at the history, pattern recognition system has come a long way. Earlier it was confined to theoretical research in the field of statistics for deriving various models out of the large amount of data. With the advent in computer technology, number of practical applications is increased in manifold which lead to further theoretical developments. At present, pattern recognition has become integral part of any machine intelligence system that exhibit decision making capabilities. Many different mathematical techniques are used for this purpose. Pattern recognition is concerned with the design and development of systems that recognize patterns in data. The purpose of a pattern recognition program is to analyze a scene in the real world and to arrive at a description of the scene which is useful for the accomplishment of some task. The real world observations are gathered through sensors and pattern recognition system classifies or describes these observations. A feature extraction mechanism computes numeric or symbolic information from these observations. These extracted features are then classified or described using a classifier. The process used for pattern recognition consists of many procedures that ensure efficient description of the patterns. 1.2 Pattern Recognition Pattern recognition can be defined as the categorization of input data into identifiable classes via the extraction of significant features or attributes of the data from a background of irrelevant detail. Duda and Hart defined it as a field concerned with machine recognition of meaningful regularities in noisy or complex environments. A simpler definition is search for structure in data. According to Jain et al. pattern recognition is a general term to describe a wide range of problems like recognition, description, classification, and grouping of patterns. Pattern recognition is about guessing or predicting the unknown nature of an observation, a discrete quantity such as black or white, one or zero, sick or healthy, real or fake. Watanabe defined a pattern as “opposite of a chaos; it is an entity, vaguely defined, that could be given a name.” For example, a pattern could be a fingerprint image, a handwritten word, a human face, or a speech signal. The pattern recognition problems are important in a variety of engineering and scientific disciplines such as biology, psychology, medicine, marketing, artificial intelligence, computer vision and remote sensing. The field of pattern recognition is concerned mainly with the description and analysis of measurements taken from physical or mental processes. It consists of acquiring raw data and taking actions based on the “class” of the patterns recognized in the data. Earlier it was studied as a specialized subject due to higher cost of the hardware for acquiring the data and to compute the answers. The fast developments in computer technology and resources enhanced possible various practical applications of pattern recognition, which in turn contributed to the demands for further theoretical developments. The design of a pattern recognition system essentially involves the following three aspects: data representation, Classification and finally, Prototyping. The problem domain dictates the choice of sensors, pre-processing techniques, representation scheme, and decision making model Representation - It describes the patterns to be recognized; Classification - It recognizes the “category” to which the patterns provided belong to; Prototyping - It is the mechanism used for developing the prototypes or models. Prototypes are used for representing the different classes to be recognized. A general pattern recognition system is shown in the Figure 1. In the first step data is acquired and preprocessed, this step is followed by feature extraction, feature reduction and grouping of features, and finally the features are classified. In the classification step, the trained classifier assigns the input pattern to one of the pattern classes based on the measured features. The training set used during construction of the classifier is different from the test set which is used for evaluation. This ensures different performance environment. Figure 1 1.3 Pattern Recognition approaches Patterns generated from the raw data depend on the nature of the data. Patterns may be generated based on the statistical feature of the data. In some situations, underlying structure of the data decides the type of the pattern generated. In some other instances, neither of the two situation exits. In such scenarios a system is developed and trained for desired responses. Thus, for a given problem one or more of these different approaches may be used to obtain the solution. Hence, to obtain the desired attributes for a pattern recognition system, there are many different mathematical techniques. The four best-known approaches for the pattern recognition are: 1. Template matching 2. Statistical classification 3. Syntactic matching 4. Neural networks In template matching, the prototype of the pattern to be recognized is compared against the pattern to be recognized. In the statistical approach, the patterns are described as random variables, from which class densities can be inferred. Classification is done based on the statistical modeling of data. In the syntactic approach, a pattern is seen as being composed of simple sub-patterns which are themselves built from yet simpler sub-patterns, the simplest being the primitives. Inter relationships between these primitive patterns are used to represent a more complex pattern. The neural network approach to pattern recognition is strongly related to the statistical methods, since they can be regarded as parametric models with their own learning scheme. The models proposed need not be independent and sometimes the same pattern recognition method exists with different interpretations. A hybrid system may be built involving multiple models. 1.3.1 Template matching One of the simplest and earliest approaches to pattern recognition is based on template matching as shown in Fig. 1. Matching is carried out to determine the similarity between two entities such as points, curves, or shapes of the same type. In template matching, a template or a prototype of the pattern to be recognized is available. The pattern to be recognized is matched against the stored template while taking into account all allowable operations such as translation, rotation and scale changes. Match observed object to stored images - “A” is recognized by matching it to stored photograph like image of previously seen “A”. Problems – Too many templates needed One template for “A” is probably not enough Need a template for “A” with a specific size, orientation, color, etc. Can this problem be solved with pre-processors? – Ignores intuition that objects are composed of smaller parts 1.3.2 Statistical Pattern Recognition The statistical pattern recognition approach assumes statistical basis for classification of data. It generates random parameters that represent the properties of the pattern to be recognized. The main goal of statistical pattern classification is to find to which category or class a given sample belongs. Statistical methodologies such as statistical hypothesis testing, correlation and Bayes classification are used for implementing this method. The effectiveness of the representation is determined by how well pattern from different classes are well separated. To measure the nearness of the given sample with one of the classes, statistical pattern recognition uses probability of error. Bayesian classifier is a natural choice in applying statistical methods to pattern recognition. However, its implementation is often difficult due to the complexity of the problems and especially when the dimensionality of the system is high. One can also consider simpler solution such as a parametric classifier based on assumed mathematical forms such as linear, quadratic or piecewise. Initially a parametric form of the decision boundary is specified; then the best decision boundary of the specified form is found based on the classification of training samples. Another important issue concerned with statistical pattern recognition is the estimation of the values of the parameters since they are not given in practice. In these systems it is always important to understand how the number of samples affects the classifier design and performance. 1.3.3 Syntactic Pattern Recognition In many situations there exist interrelationship or interconnection between the features associated with a pattern. In such circumstances it is appropriate to assume a hierarchical relationship where a pattern is viewed as being consist of simple sub patterns which are themselves built with yet another sub pattern. This is the basis of Syntactic pattern recognition. In this method symbolic data structures such as arrays, strings, trees, or graphs are used for pattern representation. These data structures define the relations between fundamental pattern components and allow the representation of hierarchical models. Thus complex patterns can be represented from simpler ones. The recognition of an unknown pattern is accomplished by comparing its symbolic representation with a number of predefined objects. This comparison helps to compute the similarity measurement between the unknown input and with known patterns. 1.3.4 Neural Network Neural computing is based on the way by which biological neural system store and manipulates information. It can be viewed as parallel computing environment consisting of interconnection of large number of simple processors. Neural networks have been successfully applied in many tasks of pattern recognition and machine learning systems. The structure of neural system is drawn from analogies with biological neural systems. 1.4 Feature Extraction and Reduction Feature selection is the process of choosing input to the pattern recognition system. Many methods can be used to extract the features. The feature selected is such that it is relevant to the task at hand. These features can be obtained from the mathematical tools or by applying feature extraction algorithm or operator to the input data. The level at which these features are extracted determines the amount of necessary preprocessing and may influence the amount of error introduced into the feature extracted. Features many be represented as continuous, discrete, or discrete binary variables. During the features extraction phase of the recognition process objects are measured. A measurement is the value of some quantifiable property of an object. A feature is a function of one or more measurements, computed so that it quantifies some significant characteristic of the object. This process produces a set of features that, taken together, forms the feature vector. A number of transformations can be used to generate features. The basic idea is to transform a given set of measurements to a new set of features. Transformation of features can lead to a strong reduction of information as compared with the original input data. In most of the situations relatively small number of features is sufficient for correct recognition. Obviously feature reduction is a sensitive procedure since if the reduction is done incorrectly the whole recognition system may fail or may not produce the expected results. Examples of such transformations are the Fourier transform, Empirical mode decomposition, and the transform. Feature generation via linear transformation techniques is just one of the many possibilities. Feature extraction also depends on application in hand and may use different techniques such as moment-based features, chain codes, and parametric models to obtain required features. 1.5 Cluster Analysis The main objective in clustering techniques is to partition a given data set into homogeneous clusters. The term homogeneous is used in the sense that all points in the same group are similar to each other and are not similar to points in other groups. The similarity of these points is defined according to some established criteria. While the use of clustering in pattern recognition and image processing is relatively recent, cluster analysis is not a new field. It has been used in other disciplines, such as biology, psychology, geology and information retrieval. The majority of the clustering algorithms find clusters of a particular shape. Most of the real problems involve clustering in higher dimension. And the difficulties with the natural interpretation of data embedded in a high dimensional space are evident. Clustering method is a very active field in pattern recognition and data mining. Thus a large amount of clustering algorithms continues to appear in the literature. Most of these algorithms are based on proximity measures. Even though, there are a class of algorithm based on different combinations of a proximity measure and a clustering scheme. Clustering is a major tool used in a number of applications, which can be basically used in four different ways namely data reduction, hypothesis generation, hypothesis testing and prediction based on group. 1.6 Classifiers Design Classifiers are designed to perform the classification stage of the pattern recognition system. A Classifier partitions the feature space into different regions. The border of each decision region is a decision boundary. The determination of region to which the feature vector belongs to is a challenging task. There are many approaches for the design of the classifier in a pattern recognition system and they can be grouped in three classes: classifiers based on Bayes decision theory, linear and nonlinear classifiers. The first approach builds upon probabilistic arguments stemming from the statistical nature of the generated features. This is due to the statistical variation of the patterns as well as to possible noise obtained in the signal acquisition phase. The objective of this type of design is to classify an unknown pattern in the most probable class as deduced from the estimated probability density functions. Even though linear classifiers are more restricted in their use, the major advantage is their simplicity and computational demand in solving problems which do not require more sophisticated nonlinear model. Examples of linear classifiers are the perceptron algorithm and least squares methods. For problems that are not linearly separable and for which the design of a linear classifier, even in an optimal way, does not lead to satisfactory performance, the use of nonlinear classifier are mandatory. Table 2 Examples of different pattern recognition applications.