Chapter 26 - Uncertainty - In-Depth PDF
Document Details
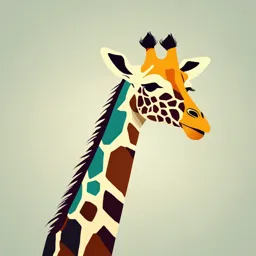
Uploaded by UnbiasedBinary8950
Tags
Related
Summary
This document is a chapter from a management accounting textbook, discussing the management accountant's role in decision-making under uncertainty and methods of communicating uncertainty. It details probability distributions, payoff tables, and graphs as tools to analyze uncertainty.
Full Transcript
# Chapter 26: Uncertainty - In-Depth ## Chapter 26: Uncertainty - In-Depth This chapter discusses the management accountant's role in decision-making under uncertainty, the importance of information quality, and methods of communicating uncertainty. ### Lessons * Uncertainty in Management Accounti...
# Chapter 26: Uncertainty - In-Depth ## Chapter 26: Uncertainty - In-Depth This chapter discusses the management accountant's role in decision-making under uncertainty, the importance of information quality, and methods of communicating uncertainty. ### Lessons * Uncertainty in Management Accounting * Uncertainty - In-Depth - Summary Problem ## Uncertainty - In-Depth ### Probability distribution | Possible outcomes | Probability | | - | - | | XX | XX% | | XX | XX% | | XX | XX% | | XX | XX% | | XX | XX% | | XX | XX% | ### Payoff table | | Possible event 1 | Possible event 2 | | ------------- | ------------- | ------------- | | Possible action 1 | $XX | $XX | | Possible action 2 | $XX | XX | ## Lesson 1: Uncertainty in Management Accounting ### Technical competencies: * Evaluates management information requirements * Documents and assesses business processes, systems and data requirements and recommends improvements to meet information needs ### Learning outcomes: * Explain the communication of uncertainty using methods such as probability distributions, payoff tables, and graphs. * Describe the value of information in decision-making. * Discuss the need for professional skepticism when using big data in decision-making. ## 26.1 Communicating Uncertainty The Uncertainty - Overview chapter introduced the concepts of uncertainty and risk in decision-making, as well as using professional skepticism over data and the dimensions of information quality. This chapter focuses on ways to communicate uncertainty within an organization. Management accountants have a variety of tools at their disposal that can be used to communicate uncertainty to management and other parties. This section discusses three such tools: probability distributions, payoff tables, and graphs. ### 26.1.1 Probability Distributions for Event Uncertainty Decision makers assess event uncertainty, which is uncertainty regarding whether a future event will occur or at what value, using probability distributions. #### 26.1.1.1 Discrete Probability Distributions In a discrete probability distribution, each of a finite number of events is assigned a probability of occurring. In business settings, discrete distributions often appear as in the form below. A marketing manager describes their beliefs about the outcome of a marketing program as follows: Sales (in units) | Probability --- | --- 100,000 | 10% 120,000 | 15% 140,000 | 40% 160,000 | 20% 180,000 | 15% #### 26.1.1.2 Expected Value Calculation Expected value is the sum of each outcome weighted where there is event uncertainty. It builds on the discrete probability distribution by arriving at a single expected value to assist with planning. To compute the expected value of sales in the above distribution, each sales amount (outcome) is multiplied by its probability, and all of the weights are summed. The following table shows the expected value of sales units: Sales (in units) | Probability | Weighted value --- | --- | --- 100,000 | 10% | 10,000 120,000 | 15% | 18,000 140,000 | 40% | 56,000 160,000 | 20% | 32,000 180,000 | 15% | 27,000 Expected value | | 143,000 The expected value is 143,000 units, but it does not mean that the organization will sell exactly 143,000 units. Instead, the expected value represents an amount that is most likely given the probability of each level of sales. When considering all the various outcomes in the table above, and the chances of each level of sales occurring, sales are expected to be about 143,000 units. When compared to the most likely outcome (140,000 in sales, at 40% probability), this number appears reasonable. ## 26.1a Let's Look at an Example Fir Co. produces and sells an electric snowblower. Customers regard Fir's products as superior because of the organization’s outstanding reputation for product quality and performance. For this reason, Fir is a price setter. A single customer is asking for bids to supply 50,000 snowblower units each year for 10 years. The computed total price per unit is subject to uncertainty. Based on the underlying cost data, the Fir cost analyst believes the total product cost per unit is now estimated to be between $370 and $400, with all values in this range equally likely. Fir charges a 20% markup on all snowblowers it sells. The bid is to be expressed as a price per unit. Based on an analysis of past bidding situations, the marketing manager believes any bid equal to or more than $480 will lose with certainty and any bid less than or equal to $380 will win with certainty. The probability of a bid between $380 and $480 being accepted is P= (480-bid amount)/100. For example, a bid of $400 would have an 80% probability of being accepted. The probability distribution can be presented as follows: Price | Probability --- | --- $380 | 90% 390 | 80% 400 | 70% 410 | 60% 420 | 50% 430 | 40% 440 | 30% 450 | 20% 460 | 10% 470 | - 480 | - Using the expected value criterion developed previously, the expected value of any bid can be written as being equal to the probability the bid will be accepted, multiplied by the profit of the difference between the bid amount and the expected cost per unit, and the total number of units in the order. The total costs were expected to be between $370 and $400 with a probability of 50%/50% for each, so the expected cost per unit will be $385 [(400+370) / 2], and the expected value of any bid (b) will be: *Expected Value of Profit = [($480-b)/100] x (b$385) × (50,000 products per year x 10 years)* Use trial and error or Excel Solver (linear programming, covered in the In-Depth Quantitative Modelling - Linear Programming and Regression Analysis chapter), the bid amount that maximizes expected profit is $432.50 and the expected profit associated with this bid is $11,281,250 over the 10 years. | | A | B | | ------------- | ------------- | ------------- | | 1 | | | 2 | | | 3 | Bid price | $432.50 | | 4 | | | 5 | Objective to maximize the profit | $11,281,250 | | 6 | | | 7 | Maximum bid price | $480 | | 8 | Minimum bid price | $380 | However, the expected profit margin range for this bid is a high of 16.89% [($432.50-$370)/$370] to a low of 8.13% [($432.50-$400)/400], and the expected value is 12.34% [($432.50-$385)/385]. Therefore, with this expected-profit-maximizing bid, Fir has no chance of achieving its target markup of 20% on costs. If Fir focuses on long term price setting instead of one-time profit maximization, it would likely bid higher. To achieve its target markup, the organization would have to bid at least $444 ($370 x 1.20) and no more than $480. Fir does not expect that a bid of $480 would be accepted, so Fir should bid between $444 and $479. Although this bid will have a lower chance of being accepted, Fir would like to cover its costs, and a bid of at least $444 will achieve the 20% target profit margin. If the bid is not accepted, Fir can focus on selling snowblowers to other customers, as it is a price setter. The role of accurate cost information is critical in this exercise, since it provides the basis for the best bid. ## 26.1b Let's Look at an Example Gallant Co. manufactures a unique thermostat that yields dramatic cost savings through effective climate control of large buildings. The efficiency of the thermostat depends on the quality of a specialized thermocouple. These thermocouples are purchased from an external supplier for $15 each. For the past few years, an average of 10% of the thermocouples purchased from the supplier have not met Gallant’s quality requirements. The number of unusable thermocouples has ranged from 5% to 25% of the total number purchased and has resulted in failures in meeting production schedules. In addition, Gallant has incurred additional costs to replace the defective units because the rejection rate of the units is within the range agreed on in the contract. Gallant is considering a proposal from its engineering department to manufacture the thermocouples internally. Gallant has the facilities and equipment to produce the components. The engineering department has designed a manufacturing system that will produce the thermocouples with a defect rate of 4% of the number of units produced. The following schedule presents the engineer's estimates of the probabilities that different levels of variable manufacturing cost per thermocouple will be incurred under this system. Additional annual fixed costs incurred by Gallant, if it manufactures the thermocouple, will amount to $32,500. Estimated variable manufacturing cost per thermocouple unit | Probability of occurrence --- | --- $10 | 10% $12 | 30% $14 | 40% $16 | 20% Gallant will need 18,000 thermocouples to meet its annual demand requirements. Expected variable cost to make: *($10 x 0.10) + (12 × 0.30) + (14 × 0.40) + (16 x 0.20) = $13.40 per unit* *Cost to buy [18,000/(100%-10% defect rate) x $15] = $300,000* *Cost to make:* *Variable costs (18,000/ (100%-4% defect rate) x $13.40] = $251,250* *Additional fixed costs = $32,500* *Total Cost to make = $283,750* *Estimated annual savings if thermocouples are made = $16,250* The organization will save approximately $16,250 a year based on the estimates and probabilities of costs if it makes the thermocouples rather than buying them, based on the current price and cost information. However, if variable costs actually end up being higher than expected, for example, at $16 per unit, it will cost $32,500 per year more to make the thermocouples. If variable costs end up being lower, at $14 each (most probable outcome), the cost savings is lower, at $5,000. | | Variable Costs of $16 | Variable Costs of $14 | | ------------- | ------------- | ------------- | | Cost to buy | $300,000 | $300,000 | | Cost to make | (300,000) | (262,500) | | Variable Costs | (32,500) | (32,500) | | Additional fixed costs | | Total | $(32,500) | $5,000 | For each 1% change in defect rate, costs go up about $2,000. The indifference point between the make or buy decision would be the $14.27 of variable costs, as calculated below: *18,000/96% X + $32,500 = $300,000* *18,750X = $300,000-$32,500* *X = $267,500/18,750* *X = $14.27* As there are a number of variables and unknown future conditions, there is no best decision. Instead, the management accountant prepares an estimate, using professional skepticism to determine if the amounts are reasonable and considering how sensitivity may change the estimate ## 26.1.2 Payoff Tables Decision makers use payoff tables to set the actions they may take against the events they cannot control to estimate the expected payoff of the alternative actions. Consider the following setting: Golden Beer Co. is planning an advertising promotion for its major product. The sales and marketing team believes the success of the promotion will depend on the actions of its major competitors, which are difficult to predict with any certainty. The team has developed the following payoff table and has decided on two possible strategies: a low-level promotion or a high-level promotion. These are the actions in this decision problem. Since promotions are planned in secret and only announced at the last minute, competitors cannot react. What determines outcomes is whether competitors are planning their own promotions. The outcomes noted below are the incremental cash flows from each action-event pair: | | Competitors have no promotions planned | Competitors have promotions planned | | ------------- | ------------- | ------------- | | Low-level promotion | $1,000,000 | $200,000 | | High-level promotion | $3,000,000 | ($2,000,000) | Based on its knowledge of competitors’ current focuses, the team believes that the probability they are planning promotions is 40%, and therefore the probability they have no promotions planned is 60% (1-40%). This information can be used to compute the expected value of Golden Beer’s two possible actions: | | Competitors have no promotions planned p = 60% | Competitors have promotions planned p = 40% | | ------------- | ------------- | ------------- | | Low-level promotion | $1,000,000 | $200,000 | | High-level promotion | $3,000,000 | ($2,000,000) | *Expected value of low-level promotion = (probability × outcome) + (probability × outcome)* *=(60% × 1,000,000) + (40% × 200,000) = $680,000* *Expected value of high-level promotion = (probability × outcome) + (probability × outcome)* *=(60% × 3,000,000) + (40% × -2,000,000) = $1,000,000* Based on this information, if Golden Beer’s objective is to maximize expected value, it would choose the high-level promotion as the expected value is higher by $320,000. ## 26.1c Let’s Look at an Example Reyes Entertainment Group (REG) is planning a holiday-themed outdoor light show for the month of December. When the weather is clear, REG can expect revenues of $20,000 and costs of $12,000 per day. However, if the weather is poor, revenues decrease to $2,000 and costs remain at $12,000. Therefore, if REG expects poor weather, the evening’s light show is cancelled. When this happens, customer payments are refunded but costs of $4,000 are still incurred. Based on past weather records, REG expects that there will be poor weather one-third of the days in the month. The management accountant has been asked to determine the expected profit whether REG runs the light show or cancels it, using a payoff table. Recall that the payoff table for the light show is as follows: | | Revenues | Costs | Profit | | ------------- | ------------- | ------------- | ------------- | | REG runs light show - Clear weather | $20,000 | $12,000 | $8,000 | | REG runs light show - Rainy weather | $2,000 | $12,000 | ($10,000) | | REG cancels light show | - | $4,000 | ($4,000) | Assuming REG runs the light show, the expected profit can be calculated as: *Expected profit if REG runs the light show = (probability × outcome) + (probability × outcome)* *=(33.33% × -$10,000) + (66.67% × $8,000) = -$4,000* Expected profit if REG cancels the light show is ($4,000). Therefore, unless the organization is sure there will be rain for the evening, it will be more profitable to run the light show if the weather forecast is uncertain. ## 26.1.3 Graphs Graphs provide a visual representation to management or other users of the possible range of outcomes, often by showing an error bar with a range of possible values. ## 26.1d Let’s Look at an Example To continue the REG example from above, the management accountant has projected the high and low daily revenue the days the organization runs the light show as follows: | | Low | High | | ------------- | ------------- | ------------- | | Day 1 | 8,000 | 12,000 | | Day 2 | 9,500 | 13,500 | | Day 3 | 12,000 | 16,000 | | Day 4 | 13,000 | 17,000 | | Day 5 | 13,500 | 17,500 | | Day 6 | 14,000 | 18,000 | | Day 7 | 14,500 | 18,500 | | Day 8 | 15,000 | 19,000 | | Day 9 | 15,500 | 19,500 | | Day 10 | 16,000 | 20,000 | | Day 11 | 17,000 | 21,000 | | Day 12 | 17,500 | 21,500 | | Day 13 | 18,000 | 22,000 | | Day 14 | 20,000 | 24,000 | | Day 15 | 21,000 | 25,000 | | Day 16 | 21,000 | 25,000 | | Day 17 | 21,000 | 25,000 | | Day 18 | 21,000 | 25,000 | | Day 19 | 21,000 | 25,000 | | Day 20 | 17,000 | 21,000 | | Day 21 | 14,000 | 18,000 | The daily revenue can be graphed to show management the expected higher level and lower level of revenue each day the light show runs. To create a graph, copy the data into Excel. Select the high and low revenue data and insert a line chart, as follows: Alternatively, the range in total revenue expected based on the high and low daily revenue can also be calculated and graphed (as shown below) to show the range of total revenue expected over the month of December for the light show, based on the expected number of clear days. This allows further forecasting of cash flows and expected profit during the month. ## 26.2 Value of Information. Today, an abundance of data is available. The key is figuring out what data can be turned into useful information and what data cannot. As discussed in the Management Accounting Today chapter, “big data” describes the increasing availability of data from both internal and external sources. Before the cloud and big data, this was a very important consideration because most information was generated internally, which meant the organization had to gather, store, and process this data. Given the costs of both processing power and storage capacity just 15 to 20 years ago compared with today, making a mistake could be very costly in terms of wasted resources with no tangible benefits. As such, organizations gathered data and turned it into useful information only for financial reporting and cost management. Because this information was used by investors and various levels of government, the focus was on accuracy. Timeliness was not as important, because the reports were submitted several months after the reporting period, so getting it right was more important than getting it fast. As computing power and data storage costs drop, more data can be collected, stored, and turned into information. Organizations now have access to an abundance of data about costs and production, which can be used to streamline these processes to drive down costs. This is referred to as the aggregation of data. It means the data has been compiled in such a way as to provide useful information. The quicker a company can access this information, the faster it can be acted on, giving the company a competitive advantage over its competitors. As information becomes timelier and more accurate, users of an organization’s information, such as lenders and suppliers, are more demanding with their information needs. ## 26.2a Let’s Look at an Example Belding Manufacturing Co. (Belding) produces three sizes of stand-up fans. The cost accountant has been asked by management if there is data that can be used to determine if changes in the materials used and manufacturing process affect the cost and sales of each product. Belding has the following data available since its inception (10 years ago): * manufacturing cost by product by month, extracted from the inventory management system * details of changes to the materials and manufacturing process, detailed in a monthly memo * sales revenue by product, extracted from the accounting information system The manufacturing costs and sales data are both structured data and may be usable in spreadsheet format with very little data cleansing required. The data regarding details of changes to manufacturing is found within monthly memos and is unstructured, as it has no underlying data model. The cost and sales data will therefore be easier for the cost accountant to use, while the manufacturing changes data will need to be read. With this data, the cost accountant could create a graphical representation of the costs and sales per product over time. The details of changes to the manufacturing process could also be incorporated, such as through showing when key changes occurred. For example, if Belding changed the materials composition in a product in Year 3, this could be added to the graph with a line or arrow showing when this occurred. If new packaging equipment was purchased in Year 5, this could also be shown on the graph. The resulting graph could provide management of Belding with information that shows how changes in the materials and manufacturing process have affected costs and sales since the inception of the organization. This could be used to make future decisions about materials changes, because management will be able to see whether past materials changes have led to changes in costs and sales revenue. ## 26.2.1 Big Data and Uncertainty Big data can help organizations take a more proactive approach to external competitive forces. For example, banks have been using business analytics for years to market different products to individual clients based on income, credit rating, where they live, trends in past credit card purchases, and general economic conditions in Canada. The difference today is the type of information they can get from the data they collect. As more and more data from a variety of different sources becomes available, it would seem logical to expect that concerns with uncertainty would diminish. After all, using more observations from a data set reduces the risk of prediction error. And this is the case when the proper expertise is used to effectively use big data as a competitive resource. However, it is important to understand some of the challenges in assessing and making sense of the volumes of data available in a big data environment. This can be summarized this using a “four V” approach: volume, velocity, veracity, and variety. | | | | ------------- | ------------- | | Volume | Volume refers to the vast amount of data generated daily, including accounting transactions, emails, texts, pictures, videos, and social media posts. As IT advances and costs get driven down, it is possible to capture ever-increasing volumes of data. | | Velocity | Velocity means getting data as quickly as possible or converting that data into usable information as quickly as possible. | | Veracity | Veracity looks at the trustworthiness or messiness of the data. The quality and accuracy of information can be an issue with social media posts, tweets, and the unlimited sources of information available on the internet. | | Variety | Variety means obtaining data from many sources, including text, audio, pictures, social media, and websites, and financial sources like financial statements, invoices, and accounting records. | Veracity, or trustworthiness, is a key concern in the use of big data to reduce uncertainty. While big data provides massive amounts of data, it does not guarantee the accuracy or usefulness of the data, thus increasing uncertain outcomes. Consider the Golden Beer situation from the previous section. If Golden Beer is based in Regina and wants to market to the rest of the province, it could use data from local liquor stores to determine the type of beer normally consumed in the province. Now, consider increasing the variety by sourcing additional data that could help Golden Beer expand its sales. Examples could include Facebook posts relating to beverages consumed, tweets relating to favourite types of beer, commodity costs of beer ingredients from NASDAQ, health reports relating to alcohol consumption, and advertising campaigns of other brewing companies, including graphic representations of beer consumption, that have effectively drawn in sales. While these additional resources can provide insights into how to market Golden Beer, the introduction of each new dimension brings in more uncertainty. Examples of this include a Facebook conversation between a friend in Regina and one in Vietnam discussing beer consumed in Vietnam, numerous health reports reporting both good and bad effects of beer consumption, or a specialty craft beer’s marketing approach that equates it to a fine wine. This becomes more complex when these various data sources overlap, such as a Facebook post that relates to health risks of beer in another country. For these reasons, accountants must use professional skepticism when using big data in decision-making. Rules similar to audit and assurance, such as a questioning mind and alertness to contrived data association and irrelevant data sources, are just a few of the steps that can be taken. Due to the ever-increasing complexities of big data, accountants must develop communication skills to work with business analysts and data scientists to reduce uncertainty when using big data to make better strategic decisions. ## Lesson 2: Uncertainty - In-Depth - Summary Problem ### Technical competencies: * Evaluates management information requirements * Documents and assesses business processes, systems, and data requirements, and recommends improvements to meet information needs ### Learning outcome: * Determine the expected value of an outcome using probability forms and payoff tables ## Summary Problem Wayne has been employed by a local library for many years and has decided to leave his job and open a bookstore. He has done some research and determined that there is a 60% chance that the local book market will sell 300,000 books per year and a 40% chance the local market will sell 225,000 books per year. Wayne will invest $50,000 of his savings to open the store, and he is currently trying to identify the best location for his investment. Wayne has identified two possible locations for his store: Jacksonville Square, which has annual rent of $18,000, and Peace Village, which has annual rent of $8,000. Jacksonville would allow Wayne to capture 25% of the total market, and Peace would allow him to capture 20%. The predicted values are based on a selling price of $10 per book. Variable costs are $6 per book. Fixed costs in addition to rent are $70,000 per year at Jacksonville and $25,000 per year at Peace. ### Required Determine the expected value at each location to assist Wayne in deciding where he should open his shop. ### Solution The first step is to create the payoff table for Wayne. The formula to determine the potential profit range is (market size x market share %) × (selling price - variable cost) - fixed costs. | | Market size = 300,000 60% | Market size = 225,000 40% | | ------------- | ------------- | ------------- | | Jacksonville Square | $212,000 | $137,000 | | Peace Village | $207,000 | $147,000 | (300,000 × 25%) × ($10-$6) - ($18,000+$70,000) (225,000 x 25%) × ($10-$6) - ($18,000+ $70,000) (300,000 × 20%) × ($10-$6) - ($8,000 $25,000) (225,000 × 20%) × ($10 $6) - ($8,000 $25,000) The next step is to factor the probability of the market size of each location. *Expected value of Jacksonville Square = ($212,000 × 60%) + ($137,000 × 40%) = $182,000.* *Expected value of Peace Village = ($207,000 × 60%) + ($147,000 × 40%) = $183,000.* Therefore, based on the quantitative information, Wayne should choose to open in Peace Village. However, as the figures are only $1,000 apart, qualitative information such as the ability to find staff, neighbourhood demographics/trends, proximity of competitors, and ease of parking, should be used to support the final decision.