Chapter 1 - Artificial Intelligence PDF
Document Details
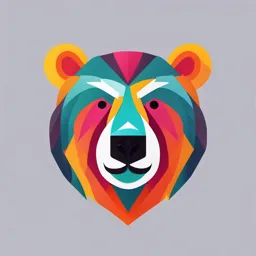
Uploaded by CleanlyConnemara7096
Tags
Summary
This document provides an introduction to artificial intelligence (AI) and its various aspects. It details the objectives, including defining AI, deep learning, and machine learning; explaining its societal importance; and discussing how AI works. The chapter also covers examples, applications, advantages, disadvantages, and the history of AI.
Full Transcript
CHAPTER 1 - ARTIFICIAL INTELLIGENCE Introduction Most people are not very familiar with the concept of artificial intelligence (AI). Yet, despite its widespread lack of familiarity, AI is a technology transforming every walk of life. It is a wide-ranging tool that enables people to rethink...
CHAPTER 1 - ARTIFICIAL INTELLIGENCE Introduction Most people are not very familiar with the concept of artificial intelligence (AI). Yet, despite its widespread lack of familiarity, AI is a technology transforming every walk of life. It is a wide-ranging tool that enables people to rethink how we integrate information, analyze data, and use the resulting insights to improve decision-making. Objectives: At the end of the chapter, the students should be able to: 1) Define artificial intelligence, deep learning and machine learning; 2) Explain the importance of artificial intelligence in society and our life; 3) Discuss how does AI works; 4) Enumerate the examples, applications, advantages and disadvantages of AI; 5) Differentiate the cognitive skills, types, categories and big ideas of AI; and 6) Identify the history of artificial intelligence. Lesson Proper Lesson 1 - Artificial Intelligence What is Artificial Intelligence? The simulation of human intelligence processes by machines, especially computer systems. The ability of a digital computer or computer-controlled robot to perform tasks commonly associated with intelligent beings. Makes it possible for machines to learn from experience, adjust to new inputs and perform human-like tasks. Frequently applied to the project of developing systems endowed with the intellectual processes characteristic of humans, such as the ability to reason, discover meaning, generalize, or learn from experience. Artificial intelligence leverages computers and machines to mimic the problem- solving and decision-making capabilities of the human mind. A branch of computer science dedicated to creating intelligent machines that work and react like humans. Specific applications of AI include 1) expert systems 2) natural language processing 3) speech recognition 4) machine vision Most AI examples that you hear about today – from chess-playing computers to self-driving cars – rely heavily on deep learning and natural language processing. Using these technologies, computers can be trained to accomplish specific tasks by processing large amounts of data and recognizing patterns in the data. From SIRI to self-driving cars, artificial intelligence (AI) is progressing rapidly. While science fiction often portrays AI as robots with human-like characteristics, AI can encompass anything from Google’s search algorithms to IBM’s Watson to autonomous weapons. Artificial intelligence today is properly known as narrow AI (or weak AI), in that it is designed to perform a narrow task (e.g. only facial recognition or only internet searches or only driving a car). However, the long-term goal of many researchers is to create general AI (AGI or strong AI). While narrow AI may outperform humans at whatever its specific task is, like playing chess or solving equations, AGI would outperform humans at nearly every cognitive task. How does AI work? In general, AI systems work by ingesting large amounts of labeled training data, analyzing the data for correlations and patterns, and using these patterns to make predictions about future states. In this way, a chatbot that is fed examples of text chats can learn to produce lifelike exchanges with people, or an image recognition tool can learn to identify and describe objects in images by reviewing millions of examples. AI programming focuses on three cognitive skills: learning, reasoning and self- correction. 1) Learning processes. This aspect of AI programming focuses on acquiring data and creating rules for how to turn the data into actionable information. The rules, which are called algorithms, provide computing devices with step-by-step instructions for how to complete a specific task. 2) Reasoning processes. This aspect of AI programming focuses on choosing the right algorithm to reach a desired outcome. 3) Self-correction processes. This aspect of AI programming is designed to continually fine-tune algorithms and ensure they provide the most accurate results possible. Why is Artificial Intelligence important? AI is important because it can give enterprises insights into their operations that they may not have been aware of previously and because, in some cases, AI can perform tasks better than humans. Particularly when it comes to repetitive, detail- oriented tasks like analyzing large numbers of legal documents to ensure relevant fields are filled in properly, AI tools often complete jobs quickly and with relatively few errors. This has helped fuel an explosion in efficiency and opened the door to entirely new business opportunities for some larger enterprises. Prior to the current wave of AI, it would have been hard to imagine using computer software to connect riders to taxis, but today Uber has become one of the largest companies in the world by doing just that. It utilizes sophisticated machine learning algorithms to predict when people are likely to need rides in certain areas, which helps proactively get drivers on the road before they're needed. As another example, Google has become one of the largest players for a range of online services by using machine learning to understand how people use their services and then improving them. In 2017, the company's CEO, Sundar Pichai, pronounced that Google would operate as an "AI first" company. Today's largest and most successful enterprises have used AI to improve their operations and gain advantage on their competitors. Here are another reasons why is artificial intelligence important: AI automates repetitive learning and discovery through data. Instead of automating manual tasks, AI performs frequent, high-volume, computerized tasks. And it does so reliably and without fatigue. Of course, humans are still essential to set up the system and ask the right questions. AI adds intelligence to existing products. Many products you already use will be improved with AI capabilities, much like Siri was added as a feature to a new generation of Apple products. Automation, conversational platforms, bots and smart machines can be combined with large amounts of data to improve many technologies. Upgrades at home and in the workplace, range from security intelligence and smart cams to investment analysis. AI adapts through progressive learning algorithms to let the data do the programming. AI finds structure and regularities in data so that algorithms can acquire skills. Just as an algorithm can teach itself to play chess, it can teach itself what product to recommend next online. And the models adapt when given new data. AI analyzes more and deeper data using neural networks that have many hidden layers. Building a fraud detection system with five hidden layers used to be impossible. All that has changed with incredible computer power and big data. You need lots of data to train deep learning models because they learn directly from the data. AI achieves incredible accuracy through deep neural networks. For example, your interactions with Alexa and Google are all based on deep learning. And these products keep getting more accurate the more you use them. In the medical field, AI techniques from deep learning and object recognition can now be used to pinpoint cancer on medical images with improved accuracy. AI gets the most out of data. When algorithms are self-learning, the data itself is an asset. The answers are in the data. You just have to apply AI to find them. Since the role of the data is now more important than ever, it can create a competitive advantage. If you have the best data in a competitive industry, even if everyone is applying similar techniques, the best data will win. What are the advantages and disadvantages of Artificial Intelligence? Artificial neural networks and deep learning artificial intelligence technologies are quickly evolving, primarily because AI processes large amounts of data much faster and makes predictions more accurately than humanly possible. While the huge volume of data being created on a daily basis would bury a human researcher, AI applications that use machine learning can take that data and quickly turn it into actionable information. As of this writing, the primary disadvantage of using AI is that it is expensive to process the large amounts of data that AI programming requires. Advantages Good at detail-oriented jobs; Reduced time for data-heavy tasks; Delivers consistent results; and AI-powered virtual agents are always available. Disadvantages Expensive; Requires deep technical expertise; Limited supply of qualified workers to build AI tools; Only knows what it's been shown; and Lack of ability to generalize from one task to another. What are examples of AI technology and how is it used today? AI is incorporated into a variety of different types of technology. Here are six examples: Automation When paired with AI technologies, automation tools can expand the volume and types of tasks performed. An example is robotic process automation (RPA), a type of software that automates repetitive, rules-based data processing tasks traditionally done by humans. When combined with machine learning and emerging AI tools, RPA can automate bigger portions of enterprise jobs, enabling RPA's tactical bots to pass along intelligence from AI and respond to process changes. Machine learning This is the science of getting a computer to act without programming. Deep learning is a subset of machine learning that, in very simple terms, can be thought of as the automation of predictive analytics. There are three types of machine learning algorithms: 1) Supervised learning. Data sets are labeled so that patterns can be detected and used to label new data sets. 2) Unsupervised learning. Data sets aren't labeled and are sorted according to similarities or differences. 3) Reinforcement learning. Data sets aren't labeled but, after performing an action or several actions, the AI system is given feedback. Machine vision This technology gives a machine the ability to see. Machine vision captures and analyzes visual information using a camera, analog-to-digital conversion and digital signal processing. It is often compared to human eyesight, but machine vision isn't bound by biology and can be programmed to see through walls, for example. It is used in a range of applications from signature identification to medical image analysis. Computer vision, which is focused on machine-based image processing, is often conflated with machine vision. Natural language processing (NLP) This is the processing of human language by a computer program. One of the older and best-known examples of NLP is spam detection, which looks at the subject line and text of an email and decides if it's junk. Current approaches to NLP are based on machine learning. NLP tasks include text translation, sentiment analysis and speech recognition. Robotics This field of engineering focuses on the design and manufacturing of robots. Robots are often used to perform tasks that are difficult for humans to perform or perform consistently. For example, robots are used in assembly lines for car production or by NASA to move large objects in space. Researchers are also using machine learning to build robots that can interact in social settings. Self-driving cars Autonomous vehicles use a combination of computer vision, image recognition and deep learning to build automated skill at piloting a vehicle while staying in a given lane and avoiding unexpected obstructions, such as pedestrians. Lesson 2 – Applications of Artificial Intelligence (AI) Artificial intelligence has made its way into a wide variety of markets. Here are nine examples. AI in healthcare The biggest bets are on improving patient outcomes and reducing costs. Companies are applying machine learning to make better and faster diagnoses than humans. One of the best-known healthcare technologies is IBM Watson. It understands natural language and can respond to questions asked of it. The system mines patient data and other available data sources to form a hypothesis, which it then presents with a confidence scoring schema. Other AI applications include using online virtual health assistants and chatbots to help patients and healthcare customers find medical information, schedule appointments, understand the billing process and complete other administrative processes. An array of AI technologies is also being used to predict, fight and understand pandemics such as COVID-19. AI in business Machine learning algorithms are being integrated into analytics and customer relationship management (CRM) platforms to uncover information on how to better serve customers. Chatbots have been incorporated into websites to provide immediate service to customers. Automation of job positions has also become a talking point among academics and IT analysts. AI in education AI can automate grading, giving educators more time. It can assess students and adapt to their needs, helping them work at their own pace. AI tutors can provide additional support to students, ensuring they stay on track. And it could change where and how students learn, perhaps even replacing some teachers. AI in finance AI in personal finance applications, such as Intuit Mint or TurboTax, is disrupting financial institutions. Applications such as these collect personal data and provide financial advice. Other programs, such as IBM Watson, have been applied to the process of buying a home. Today, artificial intelligence software performs much of the trading on Wall Street. AI in law The discovery process -- sifting through documents -- in law is often overwhelming for humans. Using AI to help automate the legal industry's labor-intensive processes is saving time and improving client service. Law firms are using machine learning to describe data and predict outcomes, computer vision to classify and extract information from documents and natural language processing to interpret requests for information. AI in manufacturing Manufacturing has been at the forefront of incorporating robots into the workflow. For example, the industrial robots that were at one time programmed to perform single tasks and separated from human workers, increasingly function as cobots: Smaller, multitasking robots that collaborate with humans and take on responsibility for more parts of the job in warehouses, factory floors and other workspaces. AI in banking Banks are successfully employing chatbots to make their customers aware of services and offerings and to handle transactions that don't require human intervention. AI virtual assistants are being used to improve and cut the costs of compliance with banking regulations. Banking organizations are also using AI to improve their decision-making for loans, and to set credit limits and identify investment opportunities. AI in transportation In addition to AI's fundamental role in operating autonomous vehicles, AI technologies are used in transportation to manage traffic, predict flight delays, and make ocean shipping safer and more efficient. Security. AI and machine learning are at the top of the buzzword list security vendors use today to differentiate their offerings. Those terms also represent truly viable technologies. Organizations use machine learning in security information and event management (SIEM) software and related areas to detect anomalies and identify suspicious activities that indicate threats. By analyzing data and using logic to identify similarities to known malicious code, AI can provide alerts to new and emerging attacks much sooner than human employees and previous technology iterations. The maturing technology is playing a big role in helping organizations fight off cyber attacks. There are numerous, real-world applications of AI systems today. Below are some of the most common examples: Speech recognition It is also known as automatic speech recognition (ASR), computer speech recognition, or speech-to-text, and it is a capability which uses natural language processing (NLP) to process human speech into a written format. Many mobile devices incorporate speech recognition into their systems to conduct voice search—e.g. Siri—or provide more accessibility around texting. Customer service Online virtual agents are replacing human agents along the customer journey. They answer frequently asked questions (FAQs) around topics, like shipping, or provide personalized advice, cross-selling products or suggesting sizes for users, changing the way we think about customer engagement across websites and social media platforms. Examples include messaging bots on e-commerce sites with virtual agents, messaging apps, such as Slack and Facebook Messenger, and tasks usually done by virtual assistants and voice assistants. Computer vision: This AI technology enables computers and systems to derive meaningful information from digital images, videos and other visual inputs, and based on those inputs, it can take action. This ability to provide recommendations distinguishes it from image recognition tasks. Powered by convolutional neural networks, computer vision has applications within photo tagging in social media, radiology imaging in healthcare, and self-driving cars within the automotive industry. Recommendation engines: Using past consumption behavior data, AI algorithms can help to discover data trends that can be used to develop more effective cross-selling strategies. This is used to make relevant add-on recommendations to customers during the checkout process for online retailers. Automated stock trading: Designed to optimize stock portfolios, AI-driven high- frequency trading platforms make thousands or even millions of trades per day without human intervention. Lesson 3 - Types of Artificial Intelligence Arend Hintze, an assistant professor of integrative biology and computer science and engineering at Michigan State University, explained in a 2016 article “Understanding the Four Types of Artificial Intelligence (govtech.com)” that AI can be categorized into four types, beginning with the task-specific intelligent systems in wide use today and progressing to sentient systems, which do not yet exist. The types are as follows: 1) Reactive Machines This kind of AI are purely reactive and do not hold the ability to form memories or use past experiences to make decisions. These machines are designed to do specific jobs. These AI systems have no memory and are task specific. An example is Deep Blue, the IBM chess program that beat Garry Kasparov in the 1990s. Deep Blue can identify pieces on the chessboard and make predictions, but because it has no memory, it cannot use past experiences to inform future ones. 2) Limited Memory This kind of AI uses past experience and the present data to make a decision. Self- driving cars are a kind of limited memory AI. These AI systems have memory, so they can use past experiences to inform future decisions. Some of the decision-making functions in self-driving cars are designed this way. Limited memory artificial intelligence has the ability to store previous data and predictions when gathering information and weighing potential decisions — essentially looking into the past for clues on what may come next. Limited memory artificial intelligence is more complex and presents greater possibilities than reactive machines. Limited memory AI is created when a team continuously trains a model in how to analyze and utilize new data or an AI environment is built so models can be automatically trained and renewed. When utilizing limited memory AI in machine learning, six steps must be followed: Training data must be created, the machine learning model must be created, the model must be able to make predictions, the model must be able to receive human or environmental feedback, that feedback must be stored as data, and these these steps must be reiterated as a cycle. There are three major machine learning models that utilize limited memory artificial intelligence: Reinforcement learning, which learns to make better predictions through repeated trial-and-error. Long Short Term Memory (LSTM), which utilizes past data to help predict the next item in a sequence. LTSMs view more recent information as most important when making predictions and discounts data from further in the past, though still utilizing it to form conclusions Evolutionary Generative Adversarial Networks (E-GAN), which evolves over time, growing to explore slightly modified paths based off of previous experiences with every new decision. This model is constantly in pursuit of a better path and utilizes simulations and statistics, or chance, to predict outcomes throughout its evolutionary mutation cycle. 3) Theory of Mind These AI machines can socialize and understand human emotions. Machines with such abilities are yet to build or develop. Theory of mind is a psychology term. When applied to AI, it means that the system would have the social intelligence to understand emotions. This type of AI will be able to infer human intentions and predict behavior, a necessary skill for AI systems to become integral members of human teams. 4) Self Awareness This is the future of AI. These machines will be super intelligent, sentient and conscious. In this category, AI systems have a sense of self, which gives them consciousness. Machines with self-awareness understand their own current state. This type of AI does not yet exist. Lesson 4 - Categories of Artificial Intelligence Artificial intelligence generally falls under two broad categories: Narrow AI - Sometimes referred to as "Weak AI," this kind of artificial intelligence operates within a limited context and is a simulation of human intelligence. - Narrow AI is often focused on performing a single task extremely well and while these machines may seem intelligent, they are operating under far more constraints and limitations than even the most basic human intelligence. - An AI system that is designed and trained to complete a specific task. Industrial robots and virtual personal assistants, such as Apple's Siri, use weak AI. - Weak AI—also called Narrow AI or Artificial Narrow Intelligence (ANI)—is AI trained and focused to perform specific tasks. Weak AI drives most of the AI that surrounds us today. ‘Narrow’ might be a more accurate descriptor for this type of AI as it is anything but weak; it enables some very robust applications, such as Apple's Siri, Amazon's Alexa, IBM Watson, and autonomous vehicles. Artificial General Intelligence (AGI) - AGI, sometimes referred to as "Strong AI," is the kind of artificial intelligence we see in the movies, like the robots from Westworld or Data from Star Trek: The Next Generation. - AGI is a machine with general intelligence and, much like a human being, it can apply that intelligence to solve any problem. - It describes programming that can replicate the cognitive abilities of the human brain. When presented with an unfamiliar task, a strong AI system can use fuzzy logic to apply knowledge from one domain to another and find a solution autonomously. In theory, a strong AI program should be able to pass both a Turing Test and the Chinese room test. - Strong AI is made up of Artificial General Intelligence (AGI) and Artificial Super Intelligence (ASI). o Artificial general intelligence (AGI), or general AI, is a theoretical form of AI where a machine would have an intelligence equaled to humans; it would have a self-aware consciousness that has the ability to solve problems, learn, and plan for the future. o Artificial Super Intelligence (ASI)—also known as superintelligence— would surpass the intelligence and ability of the human brain. While strong AI is still entirely theoretical with no practical examples in use today, that doesn't mean AI researchers aren't also exploring its development. In the meantime, the best examples of ASI might be from science fiction, such as HAL, the superhuman, rogue computer assistant in 2001: A Space Odyssey. Narrow Artificial Intelligence Narrow AI is all around us and is easily the most successful realization of artificial intelligence to date. With its focus on performing specific tasks, Narrow AI has experienced numerous breakthroughs in the last decade that have had "significant societal benefits and have contributed to the economic vitality of the nation," according to "Preparing for the Future of Artificial Intelligence," a 2016 report released by the Obama Administration. A few examples of Narrow AI include: Google search Image recognition software Siri, Alexa and other personal assistants Self-driving cars IBM's Watson Machine Learning & Deep Learning Much of Narrow AI is powered by breakthroughs in machine learning and deep learning. Understanding the difference between artificial intelligence, machine learning and deep learning can be confusing. Venture capitalist Frank Chen provides a good overview of how to distinguish between them, noting: "Artificial intelligence is a set of algorithms and intelligence to try to mimic human intelligence. Machine learning is one of them, and deep learning is one of those machine learning techniques." Simply put, machine learning feeds a computer data and uses statistical techniques to help it "learn" how to get progressively better at a task, without having been specifically programmed for that task, eliminating the need for millions of lines of written code. Machine learning consists of both supervised learning (using labeled data sets) and unsupervised learning (using unlabeled data sets). Deep learning is a type of machine learning that runs inputs through a biologically- inspired neural network architecture. The neural networks contain a number of hidden layers through which the data is processed, allowing the machine to go "deep" in its learning, making connections and weighting input for the best results. Artificial General Intelligence The creation of a machine with human-level intelligence that can be applied to any task is the Holy Grail for many AI researchers, but the quest for AGI has been fraught with difficulty. The search for a "universal algorithm for learning and acting in any environment," (Russel and Norvig 27) isn't new, but time hasn't eased the difficulty of essentially creating a machine with a full set of cognitive abilities. AGI has long been the muse of dystopian science fiction, in which super-intelligent robots overrun humanity, but experts agree it's not something we need to worry about anytime soon. Deep learning vs. machine learning Since deep learning and machine learning tend to be used interchangeably, it’s worth noting the nuances between the two. As mentioned above, both deep learning and machine learning are sub-fields of artificial intelligence, and deep learning is actually a sub-field of machine learning. Deep learning is actually comprised of neural networks. “Deep” in deep learning refers to a neural network comprised of more than three layers—which would be inclusive of the inputs and the output—can be considered a deep learning algorithm. This is generally represented using the following diagram: The way in which deep learning and machine learning differ is in how each algorithm learns. Deep learning automates much of the feature extraction piece of the process, eliminating some of the manual human intervention required and enabling the use of larger data sets. You can think of deep learning as "scalable machine learning" as Lex Fridman noted in same MIT lecture from above. Classical, or "non-deep", machine learning is more dependent on human intervention to learn. Human experts determine the hierarchy of features to understand the differences between data inputs, usually requiring more structured data to learn. "Deep" machine learning can leverage labeled datasets, also known as supervised learning, to inform its algorithm, but it doesn’t necessarily require a labeled dataset. It can ingest unstructured data in its raw form (e.g. text, images), and it can automatically determine the hierarchy of features which distinguish different categories of data from one another. Unlike machine learning, it doesn't require human intervention to process data, allowing us to scale machine learning in more interesting ways. Lesson 5 – History of Artificial Intelligence: Key dates and names The idea of 'a machine that thinks' dates back to ancient Greece. But since the advent of electronic computing (and relative to some of the topics discussed in this article) important events and milestones in the evolution of artificial intelligence include the following: 1950: Alan Turing publishes Computing Machinery and Intelligence. In the paper, Turing—famous for breaking the Nazi's ENIGMA code during WWII—proposes to answer the question 'can machines think?' and introduces the Turing Test to determine if a computer can demonstrate the same intelligence (or the results of the same intelligence) as a human. The value of the Turing test has been debated ever since. 1956: John McCarthy coins the term 'artificial intelligence' at the first-ever AI conference at Dartmouth College. (McCarthy would go on to invent the Lisp language.) Later that year, Allen Newell, J.C. Shaw, and Herbert Simon create the Logic Theorist, the first-ever running AI software program. 1967: Frank Rosenblatt builds the Mark 1 Perceptron, the first computer based on a neural network that 'learned' though trial and error. Just a year later, Marvin Minsky and Seymour Papert publish a book titled Perceptrons, which becomes both the landmark work on neural networks and, at least for a while, an argument against future neural network research projects. 1980s: Neural networks which use a backpropagation algorithm to train itself become widely used in AI applications. 1997: IBM's Deep Blue beats then world chess champion Garry Kasparov, in a chess match (and rematch). 2011: IBM Watson beats champions Ken Jennings and Brad Rutter at Jeopardy! 2015: Baidu's Minwa supercomputer uses a special kind of deep neural network called a convolutional neural network to identify and categorize images with a higher rate of accuracy than the average human. 2016: DeepMind's AlphaGo program, powered by a deep neural network, beats Lee Sodol, the world champion Go player, in a five-game match. The victory is significant given the huge number of possible moves as the game progresses (over 14.5 trillion after just four moves!). Later, Google purchased DeepMind for a reported USD 400 million. A Brief History of Artificial Intelligence Intelligent robots and artificial beings first appeared in the ancient Greek myths of Antiquity. Aristotle's development of syllogism and its use of deductive reasoning was a key moment in mankind's quest to understand its own intelligence. While the roots are long and deep, the history of artificial intelligence as we think of it today spans less than a century. The following is a quick look at some of the most important events in AI. 1940s (1943) Warren McCullough and Walter Pitts publish "A Logical Calculus of Ideas Immanent in Nervous Activity." The paper proposed the first mathematical model for building a neural network. (1949) In his book The Organization of Behavior: A Neuropsychological Theory, Donald Hebb proposes the theory that neural pathways are created from experiences and that connections between neurons become stronger the more frequently they're used. Hebbian learning continues to be an important model in AI. 1950s (1950) Alan Turing publishes "Computing Machinery and Intelligence, proposing what is now known as the Turing Test, a method for determining if a machine is intelligent. (1950) Harvard undergraduates Marvin Minsky and Dean Edmonds build SNARC, the first neural network computer. (1950) Claude Shannon publishes the paper "Programming a Computer for Playing Chess." (1950) Isaac Asimov publishes the "Three Laws of Robotics." (1952) Arthur Samuel develops a self-learning program to play checkers. (1954) The Georgetown-IBM machine translation experiment automatically translates 60 carefully selected Russian sentences into English. (1956) The phrase artificial intelligence is coined at the "Dartmouth Summer Research Project on Artificial Intelligence." Led by John McCarthy, the conference, which defined the scope and goals of AI, is widely considered to be the birth of artificial intelligence as we know it today. (1956) Allen Newell and Herbert Simon demonstrate Logic Theorist (LT), the first reasoning program. (1958) John McCarthy develops the AI programming language Lisp and publishes the paper "Programs with Common Sense." The paper proposed the hypothetical Advice Taker, a complete AI system with the ability to learn from experience as effectively as humans do. (1959) Allen Newell, Herbert Simon and J.C. Shaw develop the General Problem Solver (GPS), a program designed to imitate human problem-solving. (1959) Herbert Gelernter develops the Geometry Theorem Prover program. (1959) Arthur Samuel coins the term machine learning while at IBM. (1959) John McCarthy and Marvin Minsky founded the MIT Artificial Intelligence Project. 1960s (1963) John McCarthy starts the AI Lab at Stanford. (1966) The Automatic Language Processing Advisory Committee (ALPAC) report by the U.S. government details the lack of progress in machine translations research, a major Cold War initiative with the promise of automatic and instantaneous translation of Russian. The ALPAC report leads to the cancellation of all government-funded MT projects. (1969) The first successful expert systems are developed in DENDRAL, a XX program, and MYCIN, designed to diagnose blood infections, are created at Stanford. 1970s (1972) The logic programming language PROLOG is created. (1973) The "Lighthill Report," detailing the disappointments in AI research, is released by the British government and leads to severe cuts in funding for artificial intelligence projects. (1974-1980) Frustration with the progress of AI development leads to major DARPA cutbacks in academic grants. Combined with the earlier ALPAC report and the previous year's "Lighthill Report," artificial intelligence funding dries up and research stalls. This period is known as the "First AI Winter." 1980s (1980) Digital Equipment Corporations develops R1 (also known as XCON), the first successful commercial expert system. Designed to configure orders for new computer systems, R1 kicks off an investment boom in expert systems that will last for much of the decade, effectively ending the first "AI Winter." (1982) Japan's Ministry of International Trade and Industry launches the ambitious Fifth Generation Computer Systems project. The goal of FGCS is to develop supercomputer-like performance and a platform for AI development. (1983) In response to Japan's FGCS, the U.S. government launches the Strategic Computing Initiative to provide DARPA funded research in advanced computing and artificial intelligence. (1985) Companies are spending more than a billion dollars a year on expert systems and an entire industry known as the Lisp machine market springs up to support them. Companies like Symbolics and Lisp Machines Inc. build specialized computers to run on the AI programming language Lisp. (1987-1993) As computing technology improved, cheaper alternatives emerged and the Lisp machine market collapsed in 1987, ushering in the "Second AI Winter." During this period, expert systems proved too expensive to maintain and update, eventually falling out of favor. 1990s (1991) U.S. forces deploy DART, an automated logistics planning and scheduling tool, during the Gulf War. (1992) Japan terminates the FGCS project in 1992, citing failure in meeting the ambitious goals outlined a decade earlier. (1993) DARPA ends the Strategic Computing Initiative in 1993 after spending nearly $1 billion and falling far short of expectations. (1997) IBM's Deep Blue beats world chess champion Gary Kasparov 2000s (2005) STANLEY, a self-driving car, wins the DARPA Grand Challenge. (2005) The U.S. military begins investing in autonomous robots like Boston Dynamics' "Big Dog" and iRobot's "PackBot." (2008) Google makes breakthroughs in speech recognition and introduces the feature in its iPhone app. 2010-2014 (2011) IBM's Watson trounces the competition on Jeopardy!. (2011) Apple releases Siri, an AI-powered virtual assistant through its iOS operating system. (2012) Andrew Ng, founder of the Google Brain Deep Learning project, feeds a neural network using deep learning algorithms 10 million YouTube videos as a training set. The neural network learned to recognize a cat without being told what a cat is, ushering in the breakthrough era for neural networks and deep learning funding. (2014) Google makes the first self-driving car to pass a state driving test. (2014) Amazon's Alexa, a virtual home is released 2015-2021 (2016) Google DeepMind's AlphaGo defeats world champion Go player Lee Sedol. The complexity of the ancient Chinese game was seen as a major hurdle to clear in AI. (2016) The first "robot citizen", a humanoid robot named Sophia, is created by Hanson Robotics and is capable of facial recognition, verbal communication and facial expression. (2018) Google releases natural language processing engine BERT, reducing barriers in translation and understanding by machine learning applications. (2018) Waymo launches its Waymo One service, allowing users throughout the Phoenix metropolitan area to request a pick-up from one of the company's self-driving vehicles. (2020) Baidu releases its LinearFold AI algorithm to scientific and medical teams working to develop a vaccine during the early stages of the SARS-CoV-2 pandemic. The algorithm is able to predict the RNA sequence of the virus in just 27 seconds, 120 times faster than other methods. Lesson 6 - Five Big Ideas of AI Five Big Ideas in AI.pdf Prepared by: Engr. MARY CRIS YAMUYAM-BARION Subject Instructor