Panel Data - Introductory Econometrics for Finance (PDF)
Document Details
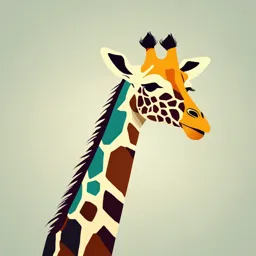
Uploaded by ImpeccableTroll
null
2013
Chris Brooks
Tags
Summary
This document covers panel data, including its properties, advantages, and various models used for analysis. It explains how panel data differs from time series and cross-sectional data. The document also discusses methods such as seemingly unrelated regression (SUR) and fixed/random effects estimators. Key econometric concepts are highlighted, relevant for finance contexts.
Full Transcript
Chapter 11 Panel Data ‘Introductory Econometrics for Finance’ © Chris Brooks 2013 1 The Nature of Panel Data Panel data, also known as longitudinal data, have both time series and cross- sectional dimensions....
Chapter 11 Panel Data ‘Introductory Econometrics for Finance’ © Chris Brooks 2013 1 The Nature of Panel Data Panel data, also known as longitudinal data, have both time series and cross- sectional dimensions. They arise when we measure the same collection of people or objects over a period of time. Econometrically, the setup is yit = + xit + uit where yit is the dependent variable, is the intercept term, is a k 1 vector of parameters to be estimated on the explanatory variables, xit; t = 1, …, T; i = 1, …, N. The simplest way to deal with this data would be to estimate a single, pooled regression on all the observations together. But pooling the data assumes that there is no heterogeneity – i.e. the same relationship holds for all the data. ‘Introductory Econometrics for Finance’ © Chris Brooks 2013 2 The Advantages of using Panel Data There are a number of advantages from using a full panel technique when a panel of data is available. We can address a broader range of issues and tackle more complex problems with panel data than would be possible with pure time series or pure cross- sectional data alone. It is often of interest to examine how variables, or the relationships between them, change dynamically (over time). By structuring the model in an appropriate way, we can remove the impact of certain forms of omitted variables bias in regression results. ‘Introductory Econometrics for Finance’ © Chris Brooks 2013 3 Seemingly Unrelated Regression (SUR) One approach to making more full use of the structure of the data would be to use the SUR framework initially proposed by Zellner (1962). This has been used widely in finance where the requirement is to model several closely related variables over time. A SUR is so-called because the dependent variables may seem unrelated across the equations at first sight, but a more careful consideration would allow us to conclude that they are in fact related after all. Under the SUR approach, one would allow for the contemporaneous relationships between the error terms in the equations by using a generalised least squares (GLS) technique. The idea behind SUR is essentially to transform the model so that the error terms become uncorrelated. If the correlations between the error terms in the individual equations had been zero in the first place, then SUR on the system of equations would have been equivalent to running separate OLS regressions on each equation. ‘Introductory Econometrics for Finance’ © Chris Brooks 2013 4 Fixed and Random Effects Panel Estimators The applicability of the SUR technique is limited because it can only be employed when the number of time series observations per cross-sectional unit is at least as large as the total number of such units, N. A second problem with SUR is that the number of parameters to be estimated in total is very large, and the variance-covariance matrix of the errors also has to be estimated. For these reasons, the more flexible full panel data approach is much more commonly used. There are two main classes of panel techniques: the fixed effects estimator and the random effects estimator. ‘Introductory Econometrics for Finance’ © Chris Brooks 2013 5 Fixed Effects Models The fixed effects model for some variable yit may be written yit = + xit + i + vit We can think of i as encapsulating all of the variables that affect yit cross- sectionally but do not vary over time – for example, the sector that a firm operates in, a person's gender, or the country where a bank has its headquarters, etc. Thus we would capture the heterogeneity that is encapsulated in i by a method that allows for different intercepts for each cross sectional unit. This model could be estimated using dummy variables, which would be termed the least squares dummy variable (LSDV) approach. ‘Introductory Econometrics for Finance’ © Chris Brooks 2013 6 Fixed Effects Models (Cont’d) The LSDV model may be written yit = + xit + 1 D1i + 2 D 2i + 3 D3i + + N DN i + vit where D1i is a dummy variable that takes the value 1 for all observations on the first entity (e.g., the first firm) in the sample and zero otherwise, D2i is a dummy variable that takes the value 1 for all observations on the second entity (e.g., the second firm) and zero otherwise, and so on. The LSDV can be seen as just a standard regression model and therefore it can be estimated using OLS. Now the model given by the equation above has N+k parameters to estimate. In order to avoid the necessity to estimate so many dummy variable parameters, a transformation, known as the within transformation, is used to simplify matters. ‘Introductory Econometrics for Finance’ © Chris Brooks 2013 7 The Within Transformation The within transformation involves subtracting the time-mean of each entity away from the values of the variable. So define yi = Tt=1 yit as the time-mean of the observations for cross-sectional unit i, and similarly calculate the means of all of the explanatory variables. Then we can subtract the time-means from each variable to obtain a regression containing demeaned variables only. Note that such a regression does not require an intercept term since now the dependent variable will have zero mean by construction. The model containing the demeaned variables is yit − yi = ( xit − xi ) + uit − ui We could write this as yit = xit + uit where the double dots above the variables denote the demeaned values. This model can be estimated using OLS, but we need to make a degrees of freedom correction. ‘Introductory Econometrics for Finance’ © Chris Brooks 2013 8 The Between Estimator An alternative to this demeaning would be to simply run a cross-sectional regression on the time-averaged values of the variables, which is known as the between estimator. An advantage of running the regression on average values (the between estimator) over running it on the demeaned values (the within estimator) is that the process of averaging is likely to reduce the effect of measurement error in the variables on the estimation process. A further possibility is that instead, the first difference operator could be applied so that the model becomes one for explaining the change in yit rather than its level. When differences are taken, any variables that do not change over time will again cancel out. Differencing and the within transformation will produce identical estimates in situations where there are only two time periods. ‘Introductory Econometrics for Finance’ © Chris Brooks 2013 9 Time Fixed Effects Models It is also possible to have a time-fixed effects model rather than an entity- fixed effects model. We would use such a model where we think that the average value of yit changes over time but not cross-sectionally. Hence with time-fixed effects, the intercepts would be allowed to vary over time but would be assumed to be the same across entities at each given point in time. We could write a time-fixed effects model as yit = + xit + t + vit where t is a time-varying intercept that captures all of the variables that affect y and that vary over time but are constant cross-sectionally. An example would be where the regulatory environment or tax rate changes part-way through a sample period. In such circumstances, this change of environment may well influence y, but in the same way for all firms. ‘Introductory Econometrics for Finance’ © Chris Brooks 2013 10 Time Fixed Effects Models (Cont’d) Time-variation in the intercept terms can be allowed for in exactly the same way as with entity fixed effects. That is, a least squares dummy variable model could be estimated yit = xit + 1D1t + 2 D 2t +... + T DTt + vit where D1t, for example, denotes a dummy variable that takes the value 1 for the first time period and zero elsewhere, and so on. The only difference is that now, the dummy variables capture time variation rather than cross-sectional variation. Similarly, in order to avoid estimating a model containing all T dummies, a within transformation can be conducted to subtract away the cross-sectional averages from each observation Finally, it is possible to allow for both entity fixed effects and time fixed effects within the same model. Such a model would be termed a two-way error component model, and the LSDV equivalent model would contain both cross-sectional and time dummies ‘Introductory Econometrics for Finance’ © Chris Brooks 2013 11 Investigating Banking Competition with a Fixed Effects Model The UK banking sector is relatively concentrated and apparently extremely profitable. It has been argued that competitive forces are not sufficiently strong and that there are barriers to entry into the market. A study by Matthews, Murinde and Zhao (2007) investigates competitive conditions in UK banking between 1980 and 2004 using the Panzar-Rosse approach. The model posits that if the market is contestable, entry to and exit from the market will be easy (even if the concentration of market share among firms is high), so that prices will be set equal to marginal costs. The technique used to examine this conjecture is to derive testable restrictions upon the firm's reduced form revenue equation. ‘Introductory Econometrics for Finance’ © Chris Brooks 2013 12 Methodology The empirical investigation consists of deriving an index (the Panzar-Rosse H-statistic) of the sum of the elasticities of revenues to factor costs (input prices). If this lies between 0 and 1, we have monopolistic competition or a partially contestable equilibrium, whereas H < 0 would imply a monopoly and H = 1 would imply perfect competition or perfect contestability. The key point is that if the market is characterised by perfect competition, an increase in input prices will not affect the output of firms, while it will under monopolistic competition. The model Matthews et al. investigate is given by ln REVit = 0 + 1 ln PLit + 2 ln PKit + 3 ln PFit + 1 ln RISKASSit + 2 ln ASSETit + 3 ln BRit + 1GROWTH t + i + vit where REVit is the ratio of bank revenue to total assets for firm i at time t, PL is personnel expenses to employees (the unit price of labour); PK is the ratio of capital assets to fixed assets (the unit price of capital); and PF is the ratio of annual interest expenses to total loanable funds (the unit price of funds). ‘Introductory Econometrics for Finance’ © Chris Brooks 2013 13 Methodology (Cont’d) The model also includes several variables that capture time-varying bank- specific effects on revenues and costs, and these are: RISKASS, the ratio of provisions to total assets; ASSET is bank size, as measured by total assets; BR is the ratio of the bank's number of branches to the total number of branches for all banks. Finally, GROWTHt is the rate of growth of GDP, which obviously varies over time but is constant across banks at a given point in time; i is a bank- specific fixed effects and vit is an idiosyncratic disturbance term. The contestability parameter, H is given as 1 + 2 + 3 Unfortunately, the Panzar-Rosse approach is only valid when applied to a banking market in long-run equilibrium. Hence the authors also conduct a test for this, which centres on the regression ln ROAit = 0 '+1 ' ln PLit + 2 ' ln PKit + 3 ' ln PFit + 1 ' ln RISKASSit + 2 ' ln ASSETit + 3 ' ln BRit + 1 ' GROWTH t + i + wit ‘Introductory Econometrics for Finance’ © Chris Brooks 2013 14 Methodology (Cont’d) The explanatory variables for the equilibrium test regression are identical to those of the contestability regression but the dependent variable is now the log of the return on assets (lnROA). Equilibrium is argued to exist in the market if 1' + 2' + 3' Matthews et al. employ a fixed effects panel data model which allows for differing intercepts across the banks, but assumes that these effects are fixed over time. The fixed effects approach is a sensible one given the data analysed here since there is an unusually large number of years (25) compared with the number of banks (12), resulting in a total of 219 bank-years (observations). The data employed in the study are obtained from banks' annual reports and the Annual Abstract of Banking Statistics from the British Bankers Association. The analysis is conducted for the whole sample period, 1980- 2004, and for two sub-samples, 1980-1991 and 1992-2004. ‘Introductory Econometrics for Finance’ © Chris Brooks 2013 15 Results from Test of Banking Market Equilibrium by Matthews et al. Source: Matthews, Murinde and Zhao (2007) ‘Introductory Econometrics for Finance’ © Chris Brooks 2013 16 Analysis of Equilibrium Test Results The null hypothesis that the bank fixed effects are jointly zero (H0: i = 0) is rejected at the 1% significance level for the full sample and for the second sub-sample but not at all for the first sub-sample. Overall, however, this indicates the usefulness of the fixed effects panel model that allows for bank heterogeneity. The main focus of interest in the table on the previous slide is the equilibrium test, and this shows slight evidence of disequilibrium (E is significantly different from zero at the 10% level) for the whole sample, but not for either of the individual sub-samples. Thus the conclusion is that the market appears to be sufficiently in a state of equilibrium that it is valid to continue to investigate the extent of competition using the Panzar-Rosse methodology. The results of this are presented on the following slide. ‘Introductory Econometrics for Finance’ © Chris Brooks 2013 17 Results from Test of Banking Market Competition by Matthews et al. Source: Matthews, Murinde and Zhao (2007) ‘Introductory Econometrics for Finance’ © Chris Brooks 2013 18 Analysis of Competition Test Results The value of the contestability parameter, H, which is the sum of the input elasticities, falls in value from 0.78 in the first sub-sample to 0.46 in the second, suggesting that the degree of competition in UK retail banking weakened over the period. However, the results in the two rows above that show that the null hypotheses that H = 0 and H = 1 can both be rejected at the 1% significance level for both sub-samples, showing that the market is best characterised by monopolistic competition. As for the equilibrium regressions, the null hypothesis that the fixed effects dummies (i) are jointly zero is strongly rejected, vindicating the use of the fixed effects panel approach and suggesting that the base levels of the dependent variables differ. Finally, the additional bank control variables all appear to have intuitively appealing signs. For example, the risk assets variable has a positive sign, so that higher risks lead to higher revenue per unit of total assets; the asset variable has a negative sign, and is statistically significant at the 5% level or below in all three periods, suggesting that smaller banks are more profitable. ‘Introductory Econometrics for Finance’ © Chris Brooks 2013 19 The Random Effects Model An alternative to the fixed effects model described above is the random effects model, which is sometimes also known as the error components model. As with fixed effects, the random effects approach proposes different intercept terms for each entity and again these intercepts are constant over time, with the relationships between the explanatory and explained variables assumed to be the same both cross-sectionally and temporally. However, the difference is that under the random effects model, the intercepts for each cross-sectional unit are assumed to arise from a common intercept (which is the same for all cross-sectional units and over time), plus a random variable i that varies cross-sectionally but is constant over time. i measures the random deviation of each entity’s intercept term from the “global” intercept term . We can write the random effects panel model as yit = + xit + it , it = i + vit ‘Introductory Econometrics for Finance’ © Chris Brooks 2013 20 How the Random Effects Model Works Unlike the fixed effects model, there are no dummy variables to capture the heterogeneity (variation) in the cross-sectional dimension. Instead, this occurs via the i terms. Note that this framework requires the assumptions that the new cross- sectional error term, i, has zero mean, is independent of the individual observation error term vit, has constant variance, and is independent of the explanatory variables. The parameters ( and the vector) are estimated consistently but inefficiently by OLS, and the conventional formulae would have to be modified as a result of the cross-correlations between error terms for a given cross-sectional unit at different points in time. Instead, a generalised least squares (GLS) procedure is usually used. The transformation involved in this GLS procedure is to subtract a weighted mean of the yit over time (i.e. part of the mean rather than the whole mean, as was the case for fixed effects estimation). ‘Introductory Econometrics for Finance’ © Chris Brooks 2013 21 Quasi-Demeaning the Data Define the ‘quasi-demeaned’ data as yit* = yit − yi and similarly for xit, will be a function of the variance of the observation error term, v2, and of the variance of the entity-specific error term, 2: v =1− T 2 + v2 This transformation will be precisely that required to ensure that there are no cross-correlations in the error terms, but fortunately it should automatically be implemented by standard software packages. Just as for the fixed effects model, with random effects, it is also conceptually no more difficult to allow for time variation than it is to allow for cross-sectional variation. In the case of time-variation, a time period-specific error term is included and again, a two-way model could be envisaged to allow the intercepts to vary both cross-sectionally and over time. ‘Introductory Econometrics for Finance’ © Chris Brooks 2013 22 Fixed or Random Effects? It is often said that the random effects model is more appropriate when the entities in the sample can be thought of as having been randomly selected from the population, but a fixed effect model is more plausible when the entities in the sample effectively constitute the entire population. More technically, the transformation involved in the GLS procedure under the random effects approach will not remove the explanatory variables that do not vary over time, and hence their impact can be enumerated. Also, since there are fewer parameters to be estimated with the random effects model (no dummy variables or within transform to perform), and therefore degrees of freedom are saved, the random effects model should produce more efficient estimation than the fixed effects approach. However, the random effects approach has a major drawback which arises from the fact that it is valid only when the composite error term it is uncorrelated with all of the explanatory variables. ‘Introductory Econometrics for Finance’ © Chris Brooks 2013 23 Fixed or Random Effects? (Cont’d) This assumption is more stringent than the corresponding one in the fixed effects case, because with random effects we thus require both i and vit to be independent of all of the xit. This can also be viewed as a consideration of whether any unobserved omitted variables (that were allowed for by having different intercepts for each entity) are uncorrelated with the included explanatory variables. If they are uncorrelated, a random effects approach can be used; otherwise the fixed effects model is preferable. A test for whether this assumption is valid for the random effects estimator is based on a slightly more complex version of the Hausman test. If the assumption does not hold, the parameter estimates will be biased and inconsistent. To see how this arises, suppose that we have only one explanatory variable, x2it that varies positively with yit, and also with the error term, it. The estimator will ascribe all of any increase in y to x when in reality some of it arises from the error term, resulting in biased coefficients. ‘Introductory Econometrics for Finance’ © Chris Brooks 2013 24 Credit Stability of Banks in Central and Eastern Europe: A Random Effects Analysis Foreign participants in the banking sector may improve competition and efficiency to the benefit of the economy that they enter. They may have a stabilising effect on credit provision since they will probably be better diversified than domestic banks and will therefore be more able to continue to lend when the host economy is performing poorly. But on the other hand, it is also argued that foreign banks may alter the credit supply to suit their own aims rather than that of the host economy. They may act more pro-cyclically than local banks, since they have alternative markets to withdraw their credit supply to when host market activity falls. Moreover, worsening conditions in the home country may force the repatriation of funds to support a weakened parent bank. ‘Introductory Econometrics for Finance’ © Chris Brooks 2013 25 The Data There may also be differences in policies for credit provision dependent upon the nature of the formation of the subsidiary abroad – i.e. whether the subsidiary's existence results from a take-over of a domestic bank or from the formation of an entirely new startup operation (a ‘greenfield investment’). A study by de Haas and van Lelyveld (2006) employs a panel regression using a sample of around 250 banks from ten Central and East European countries. They examine whether domestic and foreign banks react differently to changes in home or host economic activity and banking crises. The data cover the period 1993-2000 and are obtained from BankScope. ‘Introductory Econometrics for Finance’ © Chris Brooks 2013 26 The Model The core model is a random effects panel regression of the form: grit = + 1Takeoverit + 2Greenfieldi + 3Crisisit + 4 Macroit + 5Contrit + ( i + it ) where the dependent variable, grit, is the percentage growth in the credit of bank i in year t; Takeover is a dummy variable taking the value one for foreign banks resulting from a takeover and zero otherwise; Greenfield is a dummy taking the value one if bank is the result of a foreign firm making a new banking investment rather than taking over an existing one; crisis is a dummy variable taking the value one if the host country for bank i was subject to a banking disaster in year t. Macro is a vector of variables capturing the macroeconomic conditions in the home country (the lending rate and the change in GDP for the home and host countries, the host country inflation rate, and the differences in the home and host country GDP growth rates and the differences in the home and host country lending rates). ‘Introductory Econometrics for Finance’ © Chris Brooks 2013 27 The Model (Cont’d) Contr is a vector of bank-specific control variables that may affect the dependent variable irrespective of whether it is a foreign or domestic bank. These are: weakness parent bank, defined as loan loss provisions made by the parent bank; solvency is the ratio of equity to total assets; liquidity is the ratio of liquid assets / total assets; size is the ratio of total bank assets to total banking assets in the given country; profitability is return on assets and efficiency is net interest margin. and the 's are parameters (or vectors of parameters in the cases of 4 and 5), i is the unobserved random effect that varies across banks but not over time, and it is an idiosyncratic error term. ‘Introductory Econometrics for Finance’ © Chris Brooks 2013 28 Estimation Options de Haas and van Lelyveld discuss the various techniques that could be employed to estimate such a model. OLS is considered to be inappropriate since it does not allow for differences in average credit market growth rates at the bank level. A model allowing for entity-specific effects (i.e. a fixed effects model that effectively allowed for a different intercept for each bank) is ruled out on the grounds that there are many more banks than time periods and thus too many parameters would be required to be estimated. They also argue that these bank-specific effects are not of interest to the problem at hand, which leads them to select the random effects panel model. This essentially allows for a different error structure for each bank. A Hausman test is conducted, and shows that the random effects model is valid since the bank-specific effects i are found “in most cases not to be significantly correlated with the explanatory variables.” ‘Introductory Econometrics for Finance’ © Chris Brooks 2013 29 Results Source: de Haas and van Lelyveld (2006) ‘Introductory Econometrics for Finance’ © Chris Brooks 2008 30 Analysis of Results The main result is that during times of banking disasters, domestic banks significantly reduce their credit growth rates (i.e. the parameter estimate on the crisis variable is negative for domestic banks), while the parameter is close to zero and not significant for foreign banks. There is a significant negative relationship between home country GDP growth, but a positive relationship with host country GDP growth and credit change in the host country. This indicates that, as the authors expected, when foreign banks have fewer viable lending opportunities in their own countries and hence a lower opportunity cost for the loanable funds, they may switch their resources to the host country. Lending rates, both at home and in the host country, have little impact on credit market share growth. ‘Introductory Econometrics for Finance’ © Chris Brooks 2013 31 Analysis of Results (Cont’d) Interestingly, the greenfield and takeover variables are not statistically significant (although the parameters are quite large in absolute value), indicating that the method of investment of a foreign bank in the host country is unimportant in determining its credit growth rate or that the importance of the method of investment varies widely across the sample leading to large standard errors. A weaker parent bank (with higher loss provisions) leads to a statistically significant contraction of credit in the host country as a result of the reduction in the supply of available funds. Overall, both home-related (‘push’) and host-related (‘pull’) factors are found to be important in explaining foreign bank credit growth. ‘Introductory Econometrics for Finance’ © Chris Brooks 2013 32 Panel Unit Root and Cointegration Tests - Background Dickey-Fuller and Phillips-Perron unit root tests have low power, especially for modest sample sizes It is believed that more powerful versions of the tests can be employed when time-series and cross-sectional information is combined – as a result of the increase in sample size We could increase the number of observations by increasing the sample period, but this data may not be available, or may have structural breaks A valid application of the test statistics is much more complex for panels than single series Two important issues to consider: – The design and interpretation of the null and alternative hypotheses needs careful thought – There may be a problem of cross-sectional dependence in the errors across the unit root testing regressions Early studies that assumed cross-sectional independence are sometimes known as ‘first generation’ panel unit root tests ‘Introductory Econometrics for Finance’ © Chris Brooks 2013 33 The MADF Test We could run separate regressions over time for each series but to use Zellner’s seemingly unrelated regression (SUR) approach, which we might term the multivariate ADF (MADF) test This method can only be employed if T >> N, and Taylor and Sarno (1998) provide an early application to tests for purchasing power parity However, that technique is now rarely used, researchers preferring instead to make use of the full panel structure A key consideration is the dimensions of the panel – is the situation that T is large or that N is large or both? If T is large and N small, the MADF approach can be used But as Breitung and Pesaran (2008) note, in such a situation one may question whether it is worthwhile to adopt a panel approach at all, since for sufficiently large T, separate ADF tests ought to be reliable enough to render the panel approach hardly worth the additional complexity. ‘Introductory Econometrics for Finance’ © Chris Brooks 2013 34 The LLC Test Levin, Lin and Chu (2002) – LLC – develop a test based on the equation: The model is very general since it allows for both entity-specific and time- specific effects through αi and θt respectively as well as separate deterministic trends in each series through δit, and the lag structure to mop up autocorrelation in Δy Of course, as for the Dickey-Fuller tests, any or all of these deterministic terms can be omitted from the regression The null hypothesis is H0: ρi ≡ ρ = 0 ∀ i and the alternative is H1: ρ < 0 ∀ i. So the autoregressive dynamics are the same for all series under the alternative. ‘Introductory Econometrics for Finance’ © Chris Brooks 2013 35 The LLC Test and Nuisance Parameters One of the reasons that unit root testing is more complex in the panel framework in practice is due to the plethora of ‘nuisance parameters’ in the equation which are necessary to allow for the fixed effects (i.e. αi, θt, δit) These nuisance parameters will affect the asymptotic distribution of the test statistics and hence LLC propose that two auxiliary regressions are run to remove their impacts The resulting test statistic is asymptotically distributed as a standard normal variate (as both T and N tend to infinity) Breitung (2000) develops a modified version of the LLC test which does not include the deterministic terms and which standardises the residuals from the auxiliary regression in a more sophisticated fashion. ‘Introductory Econometrics for Finance’ © Chris Brooks 2013 36 The LLC Test – How to Interpret the Results Under the LLC and Breitung approaches, only evidence against the non- stationary null in one series is required before the joint null will be rejected Breitung and Pesaran (2008) suggest that the appropriate conclusion when the null is rejected is that ‘a significant proportion of the cross-sectional units are stationary’ Especially in the context of large N, this might not be very helpful since no information is provided on how many of the N series are stationary Often, the homogeneity assumption is not economically meaningful either, since there is no theory suggesting that all of the series have the same autoregressive dynamics and thus the same value of ρ ‘Introductory Econometrics for Finance’ © Chris Brooks 2013 37 Panel Unit Root Tests with Heterogeneous Processes This difficulty led Im, Pesaran and Shin (2003) – IPS – to propose an alternative approach where the null and alternative hypotheses are now H0: ρi = 0 ∀ i and H1: ρi < 0, i = 1, 2,... , N1; ρi = 0, i = N1 + 1, N1 + 2,... , N So the null hypothesis still specifies all series in the panel as nonstationary, but under the alternative, a proportion of the series (N1/N) are stationary, and the remaining proportion ((N − N1)/N) are nonstationary No restriction where all of the ρ are identical is imposed The statistic in this case is constructed by conducting separate unit root tests for each series in the panel, calculating the ADF t-statistic for each one in the standard fashion, and then taking their cross-sectional average This average is then transformed into a standard normal variate under the null hypothesis of a unit root in all the series While IPS’s heterogeneous panel unit root tests are superior to the homogeneous case when N is modest relative to T, they may not be sufficiently powerful when N is large and T is small, in which case the LLC approach may be preferable. ‘Introductory Econometrics for Finance’ © Chris Brooks 2013 38 The Maddala and Wu (1999) and Choi (2001) Tests Maddala and Wu (1999) and Choi (2001) developed a slight variant on the IPS approach based on an idea dating back to Fisher (1932) Unit root tests are conducted separately on each series in the panel and the p-values associated with the test statistics are then combined If we call these p-values pvi, i = 1, 2,... ,N, under the null hypothesis of a unit root in each series, pvi will be distributed uniformly over the [0,1] interval and hence the following will hold for given N as T → ∞ Note that the cross-sectional independence assumption is crucial The p-values for use in the test must be obtained from Monte Carlo simulations. ‘Introductory Econometrics for Finance’ © Chris Brooks 2013 39 Allowing for Cross-Sectional Heterogeneity The assumption of cross-sectional independence of the error terms in the panel regression is highly unrealistic For example, in the context of testing for whether purchasing power parity holds, there are likely to be important unspecified factors that affect all exchange rates or groups of exchange rates in the sample, and will result in correlated residuals. O’Connell (1998) demonstrates the considerable size distortions that can arise when such cross-sectional dependencies are present We can adjust the critical values employed but the power of the tests will fall such that in extreme cases the benefit of using a panel structure could disappear completely O’Connell proposes a feasible GLS estimator for ρ where an assumed form for the correlations between the disturbances is employed ‘Introductory Econometrics for Finance’ © Chris Brooks 2013 40 Allowing for Cross-Sectional Heterogeneity 2 To overcome the limitation that the correlation matrix must be specified (and this may be troublesome because it is not clear what form it should take), Bai and Ng (2004) propose to separate the data into a common factor component that is highly correlated across the series and a specific part that is idiosyncratic A further approach is to proceed with OLS but to employ modified standard errors – so-called ‘panel corrected standard errors’ (PCSEs) – see, for example Breitung and Das (2005) Overall, however, it is clear that satisfactorily dealing with cross-sectional dependence makes an already complex issue considerable harder still In the presence of such dependencies, the test statistics are affected in a non-trivial way by the nuisance parameters. ‘Introductory Econometrics for Finance’ © Chris Brooks 2013 41 Panel Cointegration Tests Testing for cointegration in panels is complex since one must consider the possibility of cointegration across groups of variables (what we might term ‘cross-sectional cointegration’) as well as within the groups Most of the work so far has relied upon a generalisation of the single equation methods of the Engle-Granger type following the pioneering work by Pedroni (1999, 2004) For a set of M variables yit and xm,i,t that are individually integrated of order one and thought to be cointegrated, the model is The residuals from this regression are then subjected to separate Dickey- Fuller or augmented Dickey-Fuller type regressions for each group The null hypothesis is that the residuals from all of the test regressions are unit root processes and therefore that there is no cointegration. ‘Introductory Econometrics for Finance’ © Chris Brooks 2013 42 The Pedroni Approach to Panel Cointegration Pedroni proposes two possible alternative hypotheses: – All of the autoregressive dynamics are the same stationary process – The dynamics from each test equation follow a different stationary process In the first case no heterogeneity is permitted, while in the second it is – analogous to the difference between LLC and IPS as described above Pedroni then constructs a raft of different test statistics These standardised test statistics are asymptotically standard normally distributed It is also possible to use a generalisation of the Johansen technique We could employ the Johansen approach on each group of series separately, collect the p-values for the trace test and then take −2 times the sum of their logs following Maddala and Wu (1999) above A full systems approach based on a ‘global VAR’ is possible but with considerable additional complexity – see Breitung and Pesaran (2008). ‘Introductory Econometrics for Finance’ © Chris Brooks 2013 43 Panel Unit Root Example: The Link between Financial Development and GDP Growth To what extent are economic growth and the sophistication of the country’s financial markets linked? Excessive government regulations may impede the development of the financial markets and consequently economic growth will be slower On the other hand, if economic agents are able to borrow at reasonable rates of interest or raise funding easily on the capital markets, this can increase the viability of real investment opportunities Given that long time-series are typically unavailable developing economies, traditional unit root and cointegration tests that examine the link between these two variables suffer from low power This provides a strong motivation for the use of panel techniques as in the study by Chrisopoulos and Tsionas (2004) ‘Introductory Econometrics for Finance’ © Chris Brooks 2013 44 Panel Unit Root Example: The Link between Financial Development and GDP Growth 2 Defining real output for country i as yit, financial ‘depth’ as F, the proportion of total output that is investment as S, and the rate of inflation as , the core model is Financial depth, F, is proxied by the ratio of total bank liabilities to GDP Data are from the IMF’s International Financial Statistics for ten countries (Colombia, Paraguay, Peru, Mexico, Ecuador, Honduras, Kenya, Thailand, the Dominican Republic and Jamaica) over the period 1970-2000 The panel unit root tests of Im, Pesaran and Shin, and the Maddala-Wu chi- squared test are employed separately for each variable, but using a panel comprising all ten countries The number of lags of Δyit is determined using AIC The null hypothesis in all cases is that the process is a unit root. ‘Introductory Econometrics for Finance’ © Chris Brooks 2013 45 Panel Unit Root Example: Results The results, are much stronger than for the single series unit root tests and show conclusively that all four series are non-stationary in levels but stationary in differences: ‘Introductory Econometrics for Finance’ © Chris Brooks 2013 46 Panel Cointegration Test: Example The LLC approach is used along with the Harris-Tzavalis technique, which is broadly the same as LLC but has slightly different correction factors in the limiting distribution These techniques are based on a unit root test on the residuals from the potentially cointegrating regression Christopoulos and Tsionis investigate the use of panel cointegration tests with fixed effects, and with both fixed effects and a deterministic trend in the test regressions These are applied to the regressions both with y, and separately F, as the dependent variables The results quite strongly demonstrate that when the dependent variable is output, the LLC approach rejects the null hypothesis of a unit root in the potentially cointegrating regression residuals when fixed effects only are included in the test regression, but not when a trend is also included. ‘Introductory Econometrics for Finance’ © Chris Brooks 2013 47 Panel Cointegration Test: Findings In the context of the Harris-Tzavalis variant of the residuals-based test, for both the fixed effects and the fixed effects + trend regressions, the null is rejected When financial depth is instead used as the dependent variable, none of these tests reject the null hypothesis Thus, the weight of evidence from the residuals-based tests is that cointegration exists when output is the dependent variable, but it does not when financial depth is In the final row of the table, a systems approach to testing for cointegration based on the sum of the logs of the p-values from the Johansen test shows that the null hypothesis of no cointegrating vectors is rejected The conclusion is that one cointegrating relationship exists between the four variables across the panel. ‘Introductory Econometrics for Finance’ © Chris Brooks 2013 48 Panel Cointegration Test: Table of Results ‘Introductory Econometrics for Finance’ © Chris Brooks 2013 49