Biostatistics PDF
Document Details
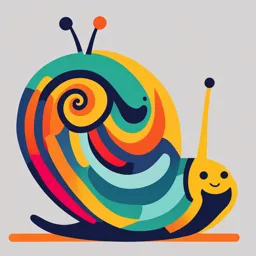
Uploaded by FerventWalrus
Tags
Summary
This document provides a detailed explanation of several biostatistical concepts, including calculations of NNH, NNT, OR, and HR. It also covers various types of medical studies and related tests, such as case-control studies, cohort studies, etc.
Full Transcript
14 I BIOS TATI STI CS NUMBER NEEDED TO HARM NNH is the numbe1· of patients who need to be treated for a certain period of time in order for one patient to experience harm. NNT and NNH are calculated with the same formula (see the NNT formula on the previous page}. There are two differences: • 1....
14 I BIOS TATI STI CS NUMBER NEEDED TO HARM NNH is the numbe1· of patients who need to be treated for a certain period of time in order for one patient to experience harm. NNT and NNH are calculated with the same formula (see the NNT formula on the previous page}. There are two differences: • 1. NNT is rounded !:!P, and NNH is rounded down (see Study Tip Gal). 2. The absolute value of the ARR is used with NNH, as shown in the following example. NNH Calculation A study evaluated the efficacy of clopidogrel versus placebo, both given in addition to aspirin, in reducing the risk of cardiovascular death, MI and stroke. The study reported a 3.9% risk of major bleeding in the treatment group and a 2.8% risk of major bleeding in the control group. Normal rounding rules do not apply: For NNT: O Anything greater than a whole number, round up to the next whole number. This avoids overstating the potential benefit of an intervention. O Example: NNT of 52.1 to 53 n- For NNH: O Anything greater than a whole number, round down to the nearest whole number. This avoids understating the potential harm of an intervention. O Example: NNH of 41.9 round down to 41 ARR== 2.8%- 3.9% = -1.1%; the absolute value is the difference between the two groups. There is a 1.1% higher risk of major bleeding in the treatment group. 90.9, rounded down to 90* NNH O.Dll ' Numbers greater than a whole number are rounded do wn NNH Interpretation One additional case of major bleeding is expected to occur for every 90 patients taking clopidogrel instead of placebo. ODDS RATIO AND HAZARD RATIO ODDS RATIO Odds are the probability that an event will occur versus the probability that it will not occur. Case-control studies, described in the Types of Medical Studies section, are not suitable for relative risk calculations. In case-control studies, the odds ratio is used to estimate the risk of unfavorable events associated with a treatment or intervention. Case-control studies enroll patients who have a clinical outcome or disease that has already occurred (e.g., lung cancer}. The patient medical charts are reviewed retrospectively (in the past) to search for possible exposures (e.g., smoking) that increased the risk of the clinical outcome or disease. In this case, the odds ratio (OR) is used to calculate the odds ofan outcome occurring with an exposure, compared to the odds of the outcome occurring without t h e exposure. ORs are used most commonly with case-control studies but can be used in cohort and cross-sectional studies. OR Formula EXPOSURE/TREATMENT Present Absent OUTCOME PRESENT OUTCOME ABSENT A B t A = # that have the outcome, with exposure B = # without the outcome, with exposure C = # that have the outcome, without exposure D = # without the outcome, without exposure 218 C T D ---, ~ I RxPREP 2022 COURSE BOOK I RxPREP ©2021, ©2022 OR Calculation A case-control study was conducted to assess the risk of falls with fracture (outcome) associated with serotonergic antidepressant (AD) use (exposure) among a cohort of Chinese females 65 years old. Cases were matched with 33,000 controls (1:4, by age, sex and cohort entry date). FALLS W/ FRACTURE (CASES) EXPOSURE/ TREATMENT Serotonergic AD-YES 18,270 ------ 73,517,430 3,259 59,541,930 X 59,541,930 14,730 3,259 14,730 73,517,430 OR= Serotonergic AD-NO X BC = 18,270 -----,- 4,991 4,991 AD FALLS W/O FRACTURE (CONTROLS) = 1.23 Conclusion: serotonergic ADs are associated with a 23% increased risk of falls with fracture (see OR and HR Interpretation below). HAZARD RATIO In a survival analysis (e.g. analysis of death or disease progression), instead of using "risk," a hazard rate is used. A hazard rate is the rate at which an unfavorable event occurs within a short period of time. Similar to RR, the hazard ratio (HR) is the ratio between the hazard rate in the treatment group and the hazard rate in the control group. HR Formula l Hazard rate in the treat~ HR _:_ t g~ Hazard rate in the control group -- - - -1 -- HR Calculation A placebo-controlled study was performed to evaluate whether niacin, when added to intensive statin therapy, reduces cardiovascular risk in patients with established cardiovascular disease. The primary endpoint was the first event of the composite endpoint (death from coronary heart disease, nonfatal myocardial infarction, ischemic stroke, hospitalization for an acute coronary syndrome or coronary or cerebral revascularization). A total of 3,414 patients were enrolled and followed for three years. Calculate the hazard ratio. Primary endpoint Niacin Hazard Rat~ NIACIN PLACEBO N = 1,718 N = 1,696 282 274 1~18 282 J Control Hazard Rate 274 = 0.16 0.16 HA = - - - = l 0.16 = 0.16 1~96 x 100 : 100% Answer can be expressed as a decimal or a percentage; the exam question will specify with instructions Conclusion: there is no benefit to cardiovascular risk when adding niacin to intensive statin therapy (see OR and HR Interpretation below). OR AND HR INTERPRETATION OR and HR are interpreted in a similar way to RR: OR or HR= 1: the event rate is the same in the treatment and control arms. There is no advantage to the treatment. OR or HR> 1: the event rate in the treatment group is higher than the event rate in the control group; for example, a HR of 2 for an outcome of death indicates that there are twice as many deaths in the treatment group. OR or HR< 1: the event rate in the treatment group is lower than the event rate in the control group; for example, a HR of 0.5 for an outcome of death indicates that there are half as many deaths in the treatment group. 219 14 I BIOSTATISTIC S PRIMARY AND COMPOSITE ENDPOINTS The primary endpoint is the main (primary) result that is measured to see if the treatment had a significant benefit. In the metoprolol trial, the primary endpoint was HF progression. Primary Endpoints (distinct and separate) Composite Endpoint (combined into one) A composite endpoint combines multiple individual endpoints into one measurement. This is attractive to researchers, as combining several endpoints increases the likelihood of reaching a statistically significant benefit with a smaller, less costly trial. Death from cardiovascular causes Death from cardiovascular causes or and Nonfatal stroke Nonfatal stroke or and Nonfatal Ml Nonfatal Ml When a composite endpoint is used, each individual endpoint gets counted toward the same (composite) outcome. COMPOSITE ENDPOINTS: CAUTION All endpoints in a composite must be similar in magnitude and have similar, meaningful importance to the patient. For example, the composite endpoint of blood pressure reduction should not be included with heart attack and stroke reduction. The FDA requires each individual endpoint to be measured and reported when a composite endpoint is used. When assessing a composite measurement, it is important to use the composite endpoint value, rather than adding together the values for the individual endpoints. The value of the sum of the individual endpoints may not equal the value of the composite endpoint, since a patient can have more than one non-fatal endpoint during a trial. TYPES OF STATISTICAL TESTS The next step following data collection (and calculation of risks, RR, ARR, HR, etc.) is to analyze the data to check if differences between the treatment and control groups are statistically significant or if there is an association or relationship in the data. Selecting the correct test to analyze the data depends on the type of data and the outcomes measured. CONTINUOUS DATA With continuous data, the type of test used to determine statistical significance depends on the distribution of data (discussed previously). If it is normally distributed, parametric methods are appropriate. If the data is not normally distributed, nonparametric methods are appropriate. T-Tests This is a parametric method used when the endpoint has continuous data and the data is normally distributed. When data from a single sample group is compared with known data from the general population, a one-sample t-test is performed. If a single sample group is used for a pre-/post-measurement (i.e., the patient serves as their own control), a paired t-test is appropriate. A student t-test is used when the study has two independent samples: the treatment and the control groups. For example, a study comparing the reduction in hemoglobin AlC values between metformin and placebo would use an independent or unpaired student t-test. Analysis of Variance Analysis of variance (ANOVA), or the F-test, is used to test for statistical significance when using continuous data with 3 or more samples, or groups. DISCRETE (CATEGORICAL) DATA Chi-Square Test For nominal 01· 01•dinal data, a chi-square test is used to determine statistical significance between treatment groups. For example, if a study assesses the difference in mortality (nominal data) between two groups, or pain scores based on a pain scale (ordinal data), a chi-square test could be used. 220 RxPREP 2022 COURSE BOOK I RxPREP ©2021, ©2022 SELECTING A TEST TO ANALYZE THE DATA Numerical/Continuous Data Discrete/Categorical Data PARAMETRIC TESTS (Data has a normal distribution) NON-PARAMETRIC TESTS (Data does not have a normal distribution) One group One-sample t-test One group Sign test One group Chi-square test Dependent/paired t-test (if one group has before and after measures) Wilcoxon Signed-Rank test (if one group has before and after measures) Wilcoxon Signed-Rank test (if one group has before and after measures) Two groups (e.g. treatment and control groups) Two groups (e.g. treatment and control groups} Independent/unpaired student t-test Mann-Whitney {Wilcoxon Rank-Sum) test Two groups (e.g. treatment and control groups) Chi-square test or Fisher's exact test Mann-Whitney {Wilcoxon Rank-Sum) test (may be preferred for ordinal data) Three or more groups ANOVA (or F-test) Three or more groups Kruskal-Wallis test Three or more groups Kruskal-Wallis test EXAMPLES OF TEST TYPE SELECTION Example 1 A study is performed to assess the safety and efficacy of ketamine-dexmedetomidine (KD) versus ketamine-propofol (KP) for sedation in patients after coronary artery bypass graft surgery. ENDPOINT (MEAN VALUES) Fentanyl dose, mcg Weaning/extubation time, min KP KO 41.94 ± 20.43 152.8 ± 51.2 374.05 ± 20.25 445.23 ± 21.7 Measurements of dose and time are both continuous data. The trial has two independent samples, or groups (KD and KP). An appropriate test is an independent/ unpaired student t-test. If the trial included a third group, ANOVA would be used. Example2 An emergency medical team wants to see if there is a statistically significant difference in death due to multiple drug overdose (OD) with at least one opioid taken, versus no opioid taken. Which test can determine a statistically significant difference in death? ENDPOINT Death (n, %) YES OPIOID NO OPIOID N =250 N = 150 52 (20.8%) T The variable (dead or alive) is nominal. The chi-square test is used to test for significance when there are two groups. 35 (23.3%) CORRELATION AND REGRESSION CORRELATION Correlation is a statistical technique that is used to determine if one variable (such as number of days hospitalized) changes, or is related to, another variable (such as incidence of hospital-acquired infection). When the independent variable (number of hospital days) causes the dependent variable (infections) to increase, the direction of the correlation is positive (increases to the right). When the independent variable causes the dependent variable to decrease, the direction of the correlation is negative (decreases to the right). Different types of data require different tests for correlation. Spearman's rank-order correlation, referred to as Rho, is used to test correlation with ordinal, ranked data. The primary test used for continuous data is the Pearson's correlation coefficient, denoted as r, which is a calculated score that indicates the strength and direction of the relationship between two variables. The values range from -1 to +1, and are described in the figure on the next page. It is not possible to conclude from a correlation analysis that the change in a variable causes the change in another variable. A correlation, whether positive or negative, does not prove a causal t·elationship. 221 14 I BIOSTATISTICS TESTING FOR CORRELATION WITH THE PEARSON CORRELATION COEFFICIENT y • ••• No Correlation The correlation coefficient measures the STRENGTH & DIRECTION of a linear relationship between two variables. shown here as X and Y, on a SCATTERPLOT • r= 0 stronger weak -1 y -0.5 stronger weak Negative correlation Positive correlation t y A perfect downhill (negative) I ' ""' :aeoo•'> 1 0.5 0 t A perfect uphill (positive) I-?~ X 161 ©RxPreµ REGRESSION Regression is used to describe the relationship between a dependent variable and one or more independent (or explanatory) variables, or how much the value of the dependent variable changes when the independent variables changes. Regression is common in observational studies where researchers need to assess multiple independent va1·iables or need to control for many confounding factors. There are three typical types of regressions: 1) linear, for continuous data, 2) logistic, for categorical data, and 3) Cox regression, for categorical data in a survival analysis. SENSITIVITY AND SPECIFICITY Lab and diagnostic tests are used to screen for and diagnose medical conditions. Interpreting sensitivity and specificity correctly is required to answer these two questions concerning the validity of lab or diagnostic test results: • If the result is positive, what is the likelihood of having the disease? • If the result is negative, what is the likelihood of not having the disease? SENSITIVITY, THE TRUE POSITIVE Sensitivity describes how effectively a test identifies patients with the condition. The higher the sensitivity, the better; a test with 100% sensitivity will be positive in all patients with the condition. Sensitivity is calculated from the number of patients who test positive, out of those who actually have the condition (sensitivity is the percentage of "true-positive" results). SPECIFICITY, THE TRUE NEGATIVE Specificity describes how effectively a test identifies patients without the condition. The higher the specificity the better; a test with 100% specificity will be negative in all patients without the condition. Specificity is calculated from the number who test negative, out of those who actually do not have the condition (specificity is the percentage of "true-negative" results). Sensitivity and Specificity Formula TEST RESULT HAVE CONDITION NO CONDITION Positive A B Negative C D Total A+C A = # that have the condition, with a positive test result B = # without the condition, with a positive test result C = # that have the condition, with a negative test result D = # without the condition, with a negative test result 222 B+D A rI Sensitivity A+C I - ---- 100 , D ~ pec: city X B+D J 100 I I ---' RxPREP 2022 COURSE BOOK I RxPREP ©2021, ©2022 Sensitivity and Specificity Calculation and Interpretation The tables below show how sensitivity and specificity is calculated for two lab tests used in the diagnosis of rheumatoid arthritis (RA), cyclic citrulline peptide (CCP) and rheumatoid factor (RF). Based on study data, CCP has a sensitivity of 98% and a specificity of 98% for RA, while RF has a sensitivity of 28% and a specificity of 87%. Using the RF lab test as an example, a sensitivity of 28% means that only 28% of patients with the condition will have a positive RF result; the test is negative in 72% of patients with the disease (and the diagnosis can be missed). A specificity of 87% means that the test is negative in 87% of patients without the disease; but 13% of patients without the disease can test positive (potentially causing an incorrect diagnosis). CCP RESULTS Positive HAVE CONDITION A= 147 Negative C=3 Total A+ C = 150 Sensitivity Specificity NO CONDITION -- RF RESULTS B=9 Positive HAVE CONDITION NO CONDITION A= 21 B = 26 ·r D =441 Negative C = 54 D = 174 B + D = 450 Total A+ C = 75 B+D=200 Sensitivity 21/75 X 100 = 28% 441/450 X 100 = 98% Sped fi city .! 147/ 150 X 100 = 98% 174/200 X 100 = 87% Sensitivity and Specificity Application If an elderly female patient with swollen finger joints is referred to a rheumatologist and lab tests reveal a positive CCP and a positive RF, the positive CCP indicates a very strong likelihood that the patient has RA because it has high sensitivity and specificity (98%). If the RF is positive and the CCP is negative, the rheumatologist would consider the possibility of other autoimmune/inflammatory conditions that could be contributing to swollen joints because of the low sensitivity of RF (28%). INTENTION-TO-TREAT AND PER PROTOCOL ANALYSIS Data from clinical trials can be analyzed in two different ways; intention-to-treat or per protocol. Intention-to-treat analysis includes data for all patients originally allocated to each treatment group (active and control) even if the patient did not complete the trial according to the study protocol (e.g., due to non-compliance, protocol violations or study withdrawal). This method provides a conservative (real-world) estimate of the treatment effect. A per protocol analysis is conducted for the subset of the trial population who completed the study according to the protocol (or at least without any major protocol violations). This method can provide an optimistic estimate of treatment effect since it is limited to the subset of patients who were adherent to the protocol. NONINFERIORITY AND EQUIVALENCE TRIAL DESIGNS The standard design of most trials is to establish that a treatment is superior to another treatment; the researcher wishes to show that the new drug is better than the old drug or a placebo. Perhaps a new treatment is developed that is less expensive or less toxic than the standard of care. Researchers would hope to demonstrate that the new drug is roughly equivalent, or at least not inferior, to the standard of care. Two types of trials are used for this purpose: equivalence and non-inferiority trials. Equivalence trials attempt to demonstrate that the new treatment has roughly the same effect as the old (or reference) treatment. These trials test for effect in two directions, for higher or lower effectiveness, which is called a two-way margin. Non-inferio1•ity trials attempt to demonstrate that the new treatment is no worse than the current standard based on the predefined non-inferiority (delta) margin. The delta margin is the minimal difference in effect between the two groups that is considered clinically acceptable based on previous research. 223 14 I BIOSTATISTICS FOREST PLOTS AND CONFIDENCE INTERVALS Forest plots are graphs that have a "forest" of lines. Forest plots can be used for a single study in which individual endpoints are pooled (gathered together) into a composite endpoint (see figure labeled Pogue, et al. below). More commonly, forest plots are used when the results from multiple studies are pooled into a single study, such as with a meta-analysis (see figure labeled Miller, et al. below). Forest plots provide Cis for difference data or ratio data. Interpreting forest plots correctly can help identify whether a statistically significant benefit has been reached. When interpreting statistical significance using a forest plot: The boxes show the effect estimate. In a meta-analysis, the size of the box correlates with the size of the effect from the single study shown. Diamonds (at the bottom of the forest plot) represent pooled results from multiple studies. The horizontal lines through the boxes illustrate the length of the confidence interval for that particular endpoint (in a single study) or for the particular study (in a meta-analysis). The longer the line, the wider the interval, and the less reliable the study results. The width of the diamond in a meta-analysis serves the same purpose. The vertical solid llne is the line of no effect; a significant benefit has been reached when data falls to the left of the line; data to the right of the line indicates significant harm. The vertical line is set at zero for difference data and at one for ratio data. COMPARING DIFFERENCE DATA The study shown to the right (a meta-analysis by Miller, et al.) uses a forest plot to test for significance with difference data. Recall for difference data, a result is not statistically significant if the confidence interval crosses zero, so the vertical line (line of no difference) is set at zero. Examples (for high-dosage vitamin E): Sludy, YIIH (Reference) All-Cause Mortallly Risk Difference (95% Cl) Low-dosase vitamin E MIN,VIT.AOX, 1999 (35) -a-l Llnxlan A, 1993{36) - ·' SU. VI MAX, 2004 (37) ATBC, 1994 (38, 39) Llnxlan B, 1993 (40) Llnqu, 2001 (41) OISSI, 1999(42) PPP,2001 (43) Totiil(lowd~e) 3rd study (PPS): shows a statistically significant benefit; the data point, plus the entire confidence interval, is to the left of the vertical line and does not cross zero. Hlgh-do588e vitamin E HOPE, 2000 (44) AREDS, 2001 (45) PPS, 19941 (46) VECAT, 200'! (-47) CHAOS, 1996 (8, 9} REACT, 2002 MB) MRC/BHF HPS, 2002 (49) 5th study (CHAOS): the result is not statistically significant; the confidence interval crosses zero. SPACE, 2000 {50) WAVE, 2002 (51) - AOCS, 1997 (51) DAJATO,. ·IJU t5!U 9th study (WAVE): shows a statistically significant harmful outcome; the data point, plus the entire confidence interval, is all to the right of the vertical line and does not cross zero. 1tiU.1tot £1)1 tu1llet Vitamin E Bem1flcJal Vllamln E Hannful Miller, et al. Ann Intern Med. 2005 Jan 4;142/1):37-46. COMPARING RATIO DATA The study shown to the right (by Pogue, et al.) uses a forest plot to test for significance of a composite endpoint reported as ratio data, in this case hazard ratio. Recall for ratio data, the result is not sta.tistically significant if the confidence interval crosses one, so the vertical line (line of no difference) is set at one. Examples: Primary composite endpoint: a statistically significant benefit was shown with treatment; the CI (0.7- 0.99) does not cross one (and the horizontal line representing the CI does not touch or cross the vertical line at one). CV death: shows no statistically significant benefit (or harm); the CI (0.92-1.83) crosses one (and the horizontal line representing the CI crosses the vertical line at one). 224 Treatment Benefit Treatment Harm HR (95% Cl) Primary Composite: - - - CV Death/Ml/CA - • -- 0.84 (0,70-0.99) CV Death 1-30 (0.92-1-83) 0.70 (0.57-0.86) Ml --- (0.60-2.06) Cardiac Arrest 0 .5 1.0 1.5 2.0 2.5 HR Pogue, et al. PLoS One 2012; 7/4): e34785. Rx PREP 2 022 COURS E BOOK TYPES OF MEDICAL STUDIES I Rx PREP © 2021 , ©2022 Most Reliable Evidence-based medicine (EBM), which is largely guideline and protocol-driven, is the foundation of practice and most patient care recommendations are based on valid study data. The type of study that a researcher chooses is a major factor in determining the quality of the study data and the clinical value or impact. The pyramid figure to the right depicts the reliability of each of the major study types. Cohort Studies CoStl•Controllo<IStud~ Common types of studies include: Case-control studies: retrospective comparisons of cases (patients with a disease) and controls (patients without a disease). Cohort studies: retrospective or prospective comparisons of patients with an exposure to those without an exposure. Randomized controlled trials: prospective comparison of patients who were randomly assigned to groups. OtM Sf!ries and Ca.ut RGporU E,q,ert Opinion least Reliable Each type of study has benefits and limitations. The table below describes the various study types and provides an example of each study. Meta-analyses: analyzes the results of multiple studies. STUDVTYPE, BENEFITS AND LIMITATIONS STUDY TYPE EXAMPLE CASE-CONTROL STUDY Predictors of surgical site Infection after open lower extremity bypass (LES) revascularlzatlon. Compares patients with a disease (cases) to those without the disease (controls). The outcome of the cases and controls is already known, but the researcher looks back in time (retrospectively) to see if a relationship exists between the disease (outcome) and various risk factors. Benefits Methods Data was pulled from 35 hospitals for all patients who had LEB during a 3-year period. Cases of surgical site infection (551) were identified and compared to those who did not develop an 551 (controls). An odds ratio (OR) was calculated for various risk factors that might increase 551 risk. Data is easy to get from medical records. Good for looking at outcomes when the intervention is unethical (e.g., exposing patients to a pesticide to test an association with cancer; cases that occurred are used instead). Less expensive than a RCT. Renal failure OR: 4.35 (95% Cl 3.45-5.47; p < 0.001). I Hypertension OR: 4.29 (95% Cl 2.74-6.72; p < 0 .001). BMI 2: 25 kg/m 2 OR: 1.78 (95% Cl 1.23-2.57; p = 0.002). Conclusion Limitations Cause and effect cannot reliably be determined (associations may be proven to be non-existent). COHORT STUDY Statistical Data ™ Compares outcomes of a of patients exposed and not exposed to a treatment; the researcher follows both groups prospectively (in the future) or retrospectively (less common) to see if they develop the outcome. Renal failure, hypertension and BMI 2: 25 kg/m 2 were all associated with an increased risk of 551. Statin use and cosnitive function In adults with type 1 diabetes. Methods Patients with type 1 diabetes who were taking statins (exposed) were compared to those not taking statins (not exposed) and followed for 7-12 years to see if statin use was associated with cognitive impairment (outcome). Benefits Good for looking at outcomes when the intervention would be unethical. Statistical Data 5tatin use and odds of cognitive impairment OR: 4.84 (95% Cl 1.63-14.44; p=0.005). Limitations More time-consuming and expensive than a retrospective study. Can be influenced by confounders, which are other factors that affect the outcome (e.g., smoking, lipid levels), Conclusion In type 1 diabetes, patients taking statins were more likely to develop cognitive impairment compared to those who did not take statins. 225 14 I BIOSTAT ISTI CS PHARMACOECONOMICS BACKGROUND Healthcare costs in the United States rank among the highest of all industrialized countries. In 2017, total healthcare expenditures reached $3.5 trillion, which translates to an average of $10,739 per person, or about 17.9% of the national gross domestic product. The increasing costs have highlighted the need to understand how limited resources can be used most effectively and efficiently in the care of individual patients and society as a whole. It is necessary to scientifically evaluate the value (i.e., costs vs. outcomes) of interventions such as medical procedures or drugs. DEFINITIONS Pharmacoeconomics is a collection of descriptive and analytic techniques for evaluating pharmaceutical interventions (e.g., drugs, devices, procedures) in the healthcare system. Pharmacoeconomic research identifies, measures and compares the costs (direct, indirect an.d in.tangible) and the consequences (clinical, economic and humanistic) of pharmaceutical products and services. Various research methods can be used to determine the impact of the pharmaceutical product or service. These methods include cost-effectiveness analysis, cost-minimization analysis, cost-utility analysis and cost-benefit analysis. The term "pharmacoeconomics" is sometimes referred to as "outcomes research," but they are not the same thing. Pharmacoeconomic methods are specific to assessing the costs and consequences of pharmaceutical products and services. Outcomes research represents a broader research discipline that attempts to identify, measure and evaluate the end result of healthcare services. Healthcare providers, payers and other decision makers use these methods to evaluate and compare the total costs and consequences of pharmaceutical products and services. The results of pharmacoeconomic analyses can vary significantly based on the point of view of the analyst; the study perspective is critical for interpretation. What may be viewed as good value for society or for the patient may not be deemed as such from an institutional or provider perspective (e.g., the costs of lost productivity due to illness are critically important to a patient or employer, but perhaps less so to a health plan) . Pharmacoeconomic analyses provide useful supplemental evidence to traditional efficacy and safety endpoints. They help translate important clinical benefits into economic and patient-centered terms, and can assist providers and payers in determining where, or if, a drug fits into the treatment paradigm for a specific condition. Pharmacoeconomic studies serve to guide optimal healthcare resource allocation in a standardized and evidence-based manner. The ECHO model (Economic, Clinical and Humanisti Outcome ) provides a broad evaluative framework to assess the outcomes associated with diseases and treatments. ~conomic outcomes: include direct, indirect and intangible costs of the drug compared to a medical intervention. Clinical outcomes: include medical events that occur as a result of the treatment or intervention. !:!umanistic Qutcomes: include consequences of the disease or treatment as reported by the patient or caregiver (e.g., patient satisfaction, quality oflife). MEDICAL COST CATEGORIES: DIRECT, INDIRECT AND INTANGIBLE DIRECT I MEDICAL I Drug preparation & administration, including home infusion supplies I I Outpatient direct costs: office & clinic visits 228 INTANGIBLE Lost work time Pain, suffering, anxiety, I j "oN-MEDtCAL I Travel and lodging costs for patients & caregivers traveling to the hospital or clinic (e.g., bus fare, gas, hotel) I Inpatient direct costs: hospital bed, administration, medical staff, surgeries, procedures, labs INDIRECT Household costs such as childcare or eldercare (e.g., when a caregiver is hospitalized or receiving physical therapy) Home health aides, to help with dressing/ bathing & other activities of daily life (ADLs) fatigue Low work productivity D orbidity: costs from having the disea se, nd related disabilit~ Mortality (death) (If: (9RxPrep RxPREP 2022 COURSE BOOK I RxPREP ©202 1, © 2022 AVERAGE AND INCREMENTAL COST-EFFECTIVENESS RATIOS The results of a pharmacoeconomic analysis are commonly expressed in terms of a cost ratio, representing the costs incurred to achieve a particular outcome [e.g., cost per case cured, cost per treatment success, cost per quality-adjusted life year (QALY) gained]. Two fundamental cost ratios are commonly used to communicate results of a pharmacoeconomic analysis. Average Cost-Effectiveness Ratios Average cost-effectiveness ratios reflect the cost per outcome of one treatment independent of other treatment alternatives. For example, if a treatment costs $50 to generate successful outcomes in two patients, the average cost-effectiveness ratio is $25/treatment success ($50/2 successfully treated patients). Incremental Cost-Effectiveness Ratios Incremental cost-effectiveness ratios represent the change in costs and outcomes when two treatment alternatives are compared. An incremental cost-effectiveness ratio is calculated when evaluating costs and outcomes between competing alternatives, and represents the additional costs required to produce an additional unit of effect. It is calculated as shown to the right, where C is for costs and E is for effects. Incremental Cost Ratio • 7 {C 2 -C 1) - - - -- -- {E 2 - E1) I _j Example: if spending $200 on Drug A results in 5 treatment successes while spending $300 on Drug B results in 7 treatment successes, what is the incremental cost ratio? ($300 - $200) $100 (7 - 5) 2 Incremental Cost Ratio " $50 Conclusion: Drug B costs $50 more relative to Drug A for each additional treatment success. PHARMACOECONOMIC METHODOLOGIES Cost-Minimization Analysis Cost-minimization analysis (CMA) is used when two or more interventions have demonstrated equivalence in outcomes, and the costs of each intervention are being compared. CMA measures and compares the input costs of treatment alternatives that have equivalent outcomes. This determination of equivalence is a key consideration in adopting this methodology. Ideally, evidence exists to support the clinical equivalence of the alternatives. In some instances, assumptions are made in the absence of relevant evidence. For example, two ACE inhibitors, captopril and lisinopril, are considered therapeutically equivalent in the literature, but the acquisition cost (the price paid for the drug) and administrative costs may be different (captopril is administered TID and lisinopril is administered once daily). A CMA looks at "minimizing costs" when multiple drugs have equal efficacy and tolerability. Another example of CMA is looking at the same drug regimen given in two different settings (e.g., hospital versus home health care). CMA is considered the easiest analysis to perform, but use of this method is limited given its ability to compare only alternatives with demonstrated equivalent outcomes. Cost-Benefit Analysis Cost-benefit analysis (CBA) is a systematic process for calculating and comparing benefits and costs of an intervention in terms of monetary units (dollars). CBA consists of identifying all the benefits from an intervention and converting them into dollars in the year that they will occur. The costs associated with the intervention are identified, allocated to the year when they occur, and then discounted back to their present day value. Given that all other factors remain constant, the program with the largest present day value of benefits minus costs is the best economic value. In CBA, it can be difficult to assign a dollar amount to a benefit (e.g., measuring the benefit of patient quality of life, which is difficult to quantify, and assigning a dollar value to it). One advantage to using CBA is the ability to determine if the benefits of the intervention exceed the costs of implementation. CBA can also be used to compare multiple programs for similar or unrelated outcomes, as long as the outcome measures can be converted to dollars. 229 14 I BIOSTATISTICS Cost-Effectiveness Analysis Cost-effectiveness analysis (CT.A) is used to compare the clinical effects of two or more interventions to the respective costs. The resources associated with the intervention are usually measured in dollars, and clinical outcomes are usually measured in natural health units (e.g., LDL values in mg/dL, % clinical cures, length of stay) . The main advantage of this method is that the outcomes are easier to quantify when compared to other analyses, and clinicians are familiar with these types of outcomes since they are similar to outcomes seen in clinical trials and practice. CEA is the most common pharmacoeconomic methodology seen in biomedical literature. A disadvantage of CEA is the inability to directly compare diffel!ent types of outcomes. For example, one cannot compare the cost-effectiveness of implementing a diabetes program with implementing an asthma program where the outcome units are different (e.g., blood glucose values versus asthma exacerbations). It is also difficult to combine two or more outcomes into one value of measurement (e.g., comparing one chemotherapeutic agent that prolongs survival, but has significant side effects, to another chemotherapeutic agent that has less effect on prolonging survival but fewer side effects). Cost-Utility Analysis Cost-utility analysi~ (CUA) is a specialized form of CEA that includes a quality-of-life component of morbidity assessments, using common health indices such as quality-adjusted life years (QALYs) and disability-adjusted life years (DALYs). CEA can measure the quantity of life (years gained) but not the "quality" or "utility" of those years. In CUA, the intervention outcome is measured in terms of QALYs gained. QALY takes into account both the quality (morbidity) and the quantity (mortality) of life gained. CUA measures outcomes based on years of life that are adjusted by utility weights, which range from 1 for "perfect health" to O for "dead." These weights can take into account patient and society preferences for specific health states. There is no consensus on the measurement, since both patient and society preferences can vary based on culture. An advantage of CUA is that different types of outcomes, and diseases with multiple outcomes of interest, can be compared (unlike CEA which can only compare one common unit). Four Basic Pharmacoeconomic Methodologies METHODOLOGY COST MEASUREMENT UNIT OUTCOME UNIT Cost-minimization analysis Dollars Demonstrated or assumed to be equivalent in comparative groups Cost-benefit analysis 1 Dollars .I Dollars __ Cost-effectiveness analysis Dollars Natural units (e.g., life-years gained, mmHg blood pressure, % at treatment goal) Cost-utility analysis Dollars Quality-adjusted-life yea r (QALY) or other utilities HEALTH-RELATED QUALITY OF LIFE Health-related quality of life (HRQOL) refers to the effects of a disease and its treatment on an individual's function and well-being, as perceived by that individual. It is commonly included under a broad umbrella of assessments known as patientreported outcomes (PROs) . HRQOL is comprised of several important domains, including physical and mental functioning, role functioning, vitality, social functioning and general health perceptions. HRQOL assessments can provide important patient-centered information related to the effects of a disease or treatment on patient functioning and well-being. These assessments are typically developed as either general (or generic) health status instruments that can be used across a number of disease areas (e.g., the SF-36 Health Survey can be used for asthma, diabetes and other conditions) or disease-specific measures applicable to a limited disease population (e.g., the Asthma Quality of Life Questionnaire). Prior to their use in practice, it is critical that the reliability and validity of HRQOL assessments in specific patient populations has been documented. 230