Big Data and Business Intelligence PDF
Document Details
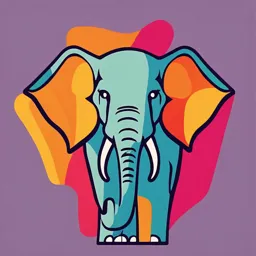
Uploaded by AwestruckBaltimore8841
University of Pretoria
Tags
Related
- Data Analytics In Accounting PDF
- GFQR 1026 Lecture 3: Big Data Analytics in Business (1) PDF
- Business Intelligence PDF
- Technologies Business Intelligence - Big Data Analytics Master 2_S3 - PDF
- Lecture 2: Business Motivations and Drivers for Big Data Adoption PDF
- Customer Relationship Management and Big Data Enabled PDF
Summary
This document provides an overview of big data concepts, including volume, velocity, variety, veracity, and value. It also examines examples of big data scenarios, highlighting the importance of data analytics, and the use of big data by the finance function. The document discusses how to improve business strategy, public health, and resource management.
Full Transcript
Big Business and Data Intelligence Open Rubric Learning outcomes Discuss what the term “big data” refers to and evaluate whether data in a scenario meets the definition thereof (measured against the criteria of volume, velocity, variety, veracity and value). Discu...
Big Business and Data Intelligence Open Rubric Learning outcomes Discuss what the term “big data” refers to and evaluate whether data in a scenario meets the definition thereof (measured against the criteria of volume, velocity, variety, veracity and value). Discuss how big data can be used to create value for an entity. Briefly discuss the implications of PoPIA on the use of data. Describe the ways in which data is used by the finance function. Identify the ways in which the finance function uses data Explain the competencies that finance professionals need. Apply the data analytics mindset: The professional habit of critically thinking through the planning, analysis, and interpretation of data analysis results before making and communicating a professional choice or decision. Criteria for Big Data Big Data refers to large and complex datasets that traditional data processing tools cannot handle efficiently. The "5 V's" of big data — Volume, Velocity, Variety, Veracity, and Value — help to understand the core criteria that define big data and the challenges it presents. Volume: The amount of data. Velocity: The speed at which data is generated and processed. Variety: The different types of data. Veracity: The accuracy and reliability of the data. Value: The usefulness and insights derived from the data. Volume: Volume refers to the large amount of data generated daily. high-volume data sources include social media activity, cell phone metadata, and financial transaction records from banking institutions. For instance, the South African Reserve Bank monitors millions of transactions daily to assess economic health and detect potential fraud, all of which generate massive amounts of data. Velocity: Velocity represents the speed at which new data is created, collected, and processed. In South Africa, high-velocity data is prominent in areas like financial services, where companies track real-time data on stock prices and currency fluctuations on the Johannesburg Stock Exchange (JSE). Mobile money transactions (like those conducted through fintech platforms) also generate data at high speeds, which must be processed instantly to ensure smooth operations. Variety: Variety highlights the diverse formats and types of data, from structured to unstructured. This includes structured banking records, semi-structured data from e-commerce platforms, and unstructured data from social media (e.g., user reviews, tweets, and Facebook posts). Additionally, public health data — such as patient records or geolocation data used to track disease outbreaks — comes in various forms, complicating integration and analysis. Veracity: Veracity refers to data accuracy, reliability, and trustworthiness. Ensuring data veracity is critical in sectors like healthcare and finance. For instance, the South African National Health Laboratory Service collects extensive data on public health. Ensuring this data is accurate and free from error is essential for effective disease management and resource allocation. Similarly, financial institutions must validate customer information and transaction data to maintain data integrity and prevent fraud. Value: Value represents the actionable insights derived from analysing big data. Data-driven insights are invaluable for improving business strategy, public health, and resource management. For example, analysing energy usage data helps Eskom, the national electricity provider, understand consumption patterns and make better decisions about load-shedding. Similarly, insights from retail data can inform strategies to address consumer preferences and improve customer satisfaction. Example Scenario A retail company collects data from customer transactions, social media interactions, and website clicks. Data Characteristics: Volume: Millions of transactions and interactions. Velocity: Real-time processing of transactions and social media updates. Variety: Structured transaction records, unstructured social media comments, and semi- structured website logs. Veracity: High, assuming data is collected accurately and verified. Value: Insights into customer behaviour, sales trends, and marketing effectiveness. Evaluating the Scenario 1. Volume: The company’s data meets the volume criterion with millions of records. 2. Velocity: The data meets the velocity criterion as it is processed in real-time. 3. Variety: The data meets the variety criterion with multiple types of data. 4. Veracity: Assuming data quality checks are in place, the scenario meets the veracity criterion. 5. Value: The data provides actionable insights that can enhance business decisions, fulfilling the value criterion. LO1 Conclusion Big Data encompasses datasets characterized by volume, velocity, variety, veracity, and value. The provided retail scenario effectively meets all these criteria. Understanding and leveraging these characteristics can help organizations derive meaningful insights and drive strategic decisions. How big data can be used to create value for an entity The potential for data to create value for an entity is substantial, particularly when it is effectively analysed and utilized. Big data, characterized by its volume, velocity, and variety, can drive innovation and competitive advantage across various sectors. While the potential for value creation through big data is significant, many organizations struggle to harness this potential due to a lack of understanding or inadequate tools. This gap highlights the need for ongoing education and investment in data capabilities. Enhanced Decision-Making Data-Driven Insights: Big data provides a wealth of information that can be analysed to make informed decisions. For instance, businesses can use historical sales data and customer behaviour analytics to forecast trends and make strategic decisions. Example: Retail Industry: Retailers use big data to analyse purchasing patterns and customer preferences, enabling them to optimize inventory, set pricing strategies, and personalize marketing efforts. Framework for Value Creation Dynamic Capabilities: Organizations must develop dynamic capabilities to explore and exploit big data resources effectively. Integrated Processes: A structured approach to data management, including acquisition, storage, and analysis, is essential for maximizing business value. Real-World Applications: Diverse Use Cases: Industries such as healthcare, finance, and smart cities have successfully implemented big data solutions, demonstrating tangible benefits Emerging Technologies: Innovations like IoT and machine learning are enhancing the ability to extract value from big data. Improved Customer Experience Personalization: By analysing customer data, businesses can tailor their products and services to meet individual needs. This leads to a more personalized experience, improving customer satisfaction and loyalty. Example: E-Commerce Platforms: Online retailers like Amazon use big data to recommend products based on past purchases, browsing history, and user reviews. Operational Efficiency Process Optimization: Big data can streamline operations by identifying inefficiencies and areas for improvement. This can lead to cost savings and more efficient processes. Example: Manufacturing: Predictive maintenance powered by big data analytics can anticipate equipment failures before they occur, reducing downtime and maintenance costs. Innovative Products and Services Market and Competitive Analysis Product Development: Analysing big data can uncover gaps in the market and inspire the development of new products and services that meet emerging needs. Example: Tech Companies: Companies like Google and Apple use big data to develop new features and technologies based on user behaviour and feedback. Market and Competitive Analysis Strategic Positioning: Big data allows entities to analyse market trends, competitor strategies, and customer sentiment to position themselves strategically in the market. Example: Financial Services: Banks and financial institutions use big data to analyse market trends and assess risks, leading to better investment decisions and competitive advantage. Risk Management and Enhanced Marketing Strategies Predictive Analytics: By analysing historical data and identifying patterns, entities can better manage risks and develop strategies to mitigate potential threats. Example: Insurance: Insurance companies use big data to assess risk profiles, personalize premiums, and prevent fraudulent claims. Enhanced Marketing Strategies Targeted Campaigns: Big data enables precise targeting of marketing campaigns by analysing consumer behaviour, preferences, and demographics. Example: Social Media Advertising: Platforms like Facebook and Instagram use big data to deliver targeted ads based on users’ interests, activities, and social interactions. Better Resource Allocation and Fraud Detection and Prevention Better resource allocation Efficiency Improvements: Big data helps organizations allocate resources more effectively by analysing usage patterns and optimizing resource distribution. Example: Energy Sector: Utilities use big data to monitor energy consumption patterns and optimize the distribution of energy across grids. Fraud detection and prevention Anomaly Detection: Big data analytics can identify unusual patterns or anomalies that may indicate fraudulent activity, allowing entities to take preventative measures. Example: Retail and Finance: Credit card companies use big data to detect fraudulent transactions in real-time by analysing transaction patterns and behaviours. Health and Safety Improvements Predictive Health: In the healthcare sector, big data can be used to predict and prevent health issues by analysing patient data, medical histories, and trends. Example: Public Health: Governments and organizations use big data to track and manage disease outbreaks, improve emergency response strategies, and implement public health policies. Big data creates value by providing actionable insights that drive decision- making, enhance customer experiences, improve operational efficiency, and foster innovation. By leveraging these insights, entities can gain a competitive edge, optimize their processes, and better serve their stakeholders. The implications of PoPIA on the use of data. The Protection of Personal Information Act (PoPIA) is South Africa's data protection legislation aimed at safeguarding personal information processed by public and private entities. Its implications on the use of data are significant and multifaceted Data Privacy and Consent Implication: Consent Requirement: Organizations must obtain explicit consent from individuals before collecting, processing, or storing their personal information. This ensures that individuals are aware of and agree to how their data will be used. Impact: Organizations must implement robust mechanisms for obtaining and managing consent, and individuals have greater control over their personal information. Data Minimization and Purpose Limitation Implication: Purpose Specification: Data must only be collected for specific, legitimate purposes and should not be retained longer than necessary for these purposes. Impact: Organizations need to clearly define and communicate the purpose of data collection and ensure they do not hold onto personal data unnecessarily, reducing the risk of data breaches and misuse. Data Security and Protection Implication: Security Measures: Entities are required to implement appropriate security measures to protect personal information from unauthorized access, loss, or damage. Impact: Organizations must invest in strong data security practices, including encryption, access controls, and regular security audits, to safeguard personal information. Data Subject Rights Implication: Access and Correction: Individuals have the right to access their personal information and request corrections or deletions if it is inaccurate or outdated. Impact: Organizations must establish processes for individuals to exercise these rights, including handling requests for access and correction efficiently and transparently. Cross-Border Data Transfers Implication: Restrictions and Safeguards: Transferring personal information outside South Africa requires ensuring that the recipient country provides similar data protection standards or that appropriate safeguards are in place. Impact: Organizations must carefully manage cross-border data transfers, possibly implementing additional contractual or legal safeguards to ensure compliance with PoPIA. Accountability and Compliance Implication: Compliance Obligations: Organizations must demonstrate compliance with PoPIA by maintaining records of data processing activities and appointing Information Officers responsible for data protection. Impact: Regular audits, compliance checks, and staff training on data protection practices become essential for organizations to avoid penalties and maintain adherence to PoPIA. Enforcement and Penalties Implication: Regulatory Oversight: PoPIA provides for regulatory oversight by the Information Regulator, which can impose penalties and take enforcement actions against non-compliant entities. Impact: Organizations must stay vigilant about compliance to avoid potential fines and legal repercussions, making data protection a critical aspect of their operations. Data in the Finance Function What is the Finance Function? Manages financial resources of an organization Involves budgeting, forecasting, financial reporting, and investment management Relies heavily on data to make informed decisions General Uses of Data in Finance Informed Decision-Making: Data provides insights that guide strategic decisions and operational adjustments. Trend Analysis: Identifies patterns and trends in financial performance and market conditions. Performance Monitoring: Tracks key metrics to assess organizational health and efficiency. Compliance and Reporting: Ensures adherence to regulations and accurate reporting of financial status. Data in the Finance Function Financial Planning and Analysis Budgeting: Data helps in creating detailed budgets by analysing past expenditures and revenues. Forecasting: Uses historical data to predict future financial performance and trends. Variance Analysis: Compares actual performance against budgeted figures to identify discrepancies. Scenario Planning: Simulates different financial scenarios to prepare for potential future conditions. Data in Financial Reporting Income Statements: Summarizes revenues, expenses, and profits using accurate data. Balance Sheets: Provides a snapshot of assets, liabilities, and equity based on detailed data. Cash Flow Statements: Tracks cash inflows and outflows to understand liquidity and operational efficiency. Regulatory Compliance: Ensures financial reports meet regulatory requirements and standards. Investment Decisions Risk Assessment: Analyses data to evaluate the risk associated with different investment options. Return Analysis: Uses historical data to estimate potential returns on investments. Valuation: Assesses the value of potential investments through data-driven models. Portfolio Management: Analyses data to balance and optimize investment portfolios for risk and return. Performance Measurement Key Performance Indicators (KPIs): Uses data to measure and evaluate key metrics like profitability, liquidity, and efficiency. Benchmarking: Compares financial metrics against industry standards or competitors. Trend Analysis: Examines data over time to identify patterns and trends. Dashboard Reporting: Utilizes data visualization tools to present performance metrics clearly. Risk Management Credit Risk Analysis: Evaluates the risk of default by analysing credit data. Market Risk Analysis: Uses data to assess the impact of market changes on financial performance. Operational Risk Analysis: Identifies and mitigates risks related to business operations using data. Stress Testing: Simulates extreme conditions to assess the impact on financial stability. Budgetary Control Monitoring Expenses: Tracks actual expenses against the budget to control overspending. Adjustments: Makes data-driven adjustments to budgets based on performance and changing conditions. Reporting: Provides regular reports to management to ensure financial targets are met. Expense Tracking: Monitors actual expenses against budgeted amounts to prevent overspending. Variance Reporting: Analyses discrepancies between budgeted and actual figures to make necessary adjustments. Reforecasting: Updates budgets based on performance data and changing conditions. Cost Management: Uses data to identify cost-saving opportunities and manage expenditures effectively. Decision Support Systems Data Integration: Combines data from various sources to support decision- making. Analytical Tools: Utilizes software tools for data analysis, modelling, and visualization. Scenario Analysis: Evaluates different scenarios using data to predict outcomes and make informed choices. Competencies Required to Use Data Effectively Analytical Skills: Ability to interpret complex data and draw meaningful conclusions. Technical Proficiency: Familiarity with data analysis tools and software (e.g., Excel, financial modelling tools). Attention to Detail: Ensures accuracy and reliability of financial data and reports. Communication Skills: Ability to present data-driven insights clearly to stakeholders. Business Acumen: Understanding of financial principles and their application in decision-making. Data Integrity: Ensures data accuracy and consistency to support reliable analysis and reporting. Problem-Solving Skills: Ability to identify issues and develop data-driven solutions. LO4/5 Conclusion Data is crucial for effective financial management. It supports planning, decision-making, and performance evaluation. Understanding data usage helps in making informed financial decisions and managing resources efficiently. Competencies in Data Strategy and Planning Strategic Thinking: Ability to align data initiatives with organizational goals and objectives. Data Governance: Knowledge of data policies, standards, and practices to ensure data quality and compliance. Data Architecture Design: Skills in designing data frameworks that support efficient data flow and accessibility. Budgeting for Data Initiatives: Competence in estimating and allocating resources for data projects. Change Management: Ability to manage and adapt to changes in data strategy and technology. Competencies in Data Engineering, Extraction, and Mining Data Extraction Techniques: Proficiency in extracting data from various sources, including databases, APIs, and spreadsheets. ETL Processes: Skills in Extracting, Transforming, and Loading (ETL) data for analysis. Database Management: Knowledge of database systems (e.g., SQL, NoSQL) and data warehousing solutions. Data Cleaning and Preprocessing: Ability to clean and preprocess data to ensure accuracy and reliability. Programming Skills: Familiarity with programming languages used in data engineering (e.g., Python, R). Competencies in Data Modelling, Manipulation, and Analysis Data Modelling: Skills in creating data models (e.g., relational, dimensional) to represent data relationships and structures. Statistical Analysis: Ability to apply statistical techniques to interpret data and identify trends. Data Manipulation: Proficiency in manipulating and transforming data to extract useful information. Predictive Analytics: Skills in using historical data to make forecasts and predictions. Data Visualization: Ability to create clear and insightful visual representations of data (e.g., charts, graphs). Competencies in Data and Insight Communication Data Interpretation: Ability to translate complex data findings into understandable insights. Report Writing: Skills in drafting comprehensive and clear financial reports and summaries. Presentation Skills: Proficiency in presenting data and insights effectively to stakeholders using tools like PowerPoint or Tableau. Storytelling with Data: Ability to craft a narrative around data to make it compelling and relevant. Stakeholder Engagement: Skills in tailoring communication to different audiences, including executives, managers, and technical teams. Competencies in Data and Insight Communication Data Interpretation: Ability to translate complex data findings into understandable insights. Report Writing: Skills in drafting comprehensive and clear financial reports and summaries. Presentation Skills: Proficiency in presenting data and insights effectively to stakeholders using tools like PowerPoint or Tableau. Storytelling with Data: Ability to craft a narrative around data to make it compelling and relevant. Stakeholder Engagement: Skills in tailoring communication to different audiences, including executives, managers, and technical teams. Conclusion Mastery in data strategy, engineering, analysis, and communication is essential for finance professionals. Each competency plays a crucial role in leveraging data to drive strategic decisions and organizational value. Developing these skills enhances the ability to manage data effectively and communicate insights clearly. Developing a Data Analytics Mindset in Finance Introduction to Data Analytics Definition: Data analytics involves examining datasets to draw conclusions about the information they contain. Importance in Finance: Helps in making informed decisions, forecasting trends, and improving financial strategies. The Data Analytics Mindset Objective: To systematically approach data with a critical and analytical mindset. Key Components: Planning, Analysis, Interpretation Planning: Setting Up for Success Define the Objective: What question are you trying to answer, or problem are you trying to solve? Example: “What is the impact of interest rate changes on stock prices?” Gather Relevant Data: Identify sources of data that are credible and relevant. Example: Financial statements, market reports, historical data. Choose the Right Tools: Software and methodologies for data collection and analysis. Example: Excel, Python, R, SQL. Analysis: Examining the Data Data Cleaning: Ensuring data is accurate, complete, and formatted correctly. Example: Removing duplicates, handling missing values. Descriptive Statistics: Summarize the basic features of the data. Example: Mean, median, standard deviation. Exploratory Data Analysis (EDA): Visualizing and exploring data to find patterns or anomalies. Example: Histograms, scatter plots, correlation matrices. Interpretation: Drawing Meaningful Conclusions Identify Trends and Patterns: Look for significant trends or patterns in the data. Example: Rising sales trends, seasonal fluctuations. Validate Results: Ensure findings are consistent with expectations and validate with other data if possible. Example: Cross-check with industry benchmarks. Consider Implications: Understand the impact of your findings on decision-making. Example: How does the trend impact future investment decisions? Making and Communicating Decisions Formulate Recommendations: Based on the analysis, develop actionable recommendations. Example: “Based on the analysis, it’s advisable to invest in XYZ stock.” Communicate Clearly: Present findings and recommendations in a clear and concise manner. Example: Use visuals like charts and graphs for clarity. Support with Evidence: Back up recommendations with data and analysis. Example: Use supporting data to justify your investment strategy. Case Study: Practical Example Scenario: Analyzing the impact of a new financial policy on company performance. Planning: Define objectives, gather data on company performance pre- and post-policy. Analysis: Compare financial metrics before and after policy implementation. Interpretation: Assess whether the policy had a positive, negative, or neutral effect. Best Practices for Data Analytics in Finance Continuous Learning: Stay updated with new tools and techniques. Attention to Detail: Ensure accuracy in data handling and analysis. Ethical Considerations: Handle data responsibly and respect privacy.