Bias, CI & Statistical Tests 2024 NOTES PDF
Document Details
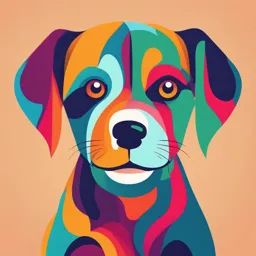
Uploaded by FruitfulIntegral
Wayne State University
2024
Laura Benjamins, Jason Booza
Tags
Summary
This document provides notes on bias, confidence intervals, and statistical tests in evidence-based medicine (EBM). The objectives include understanding types of bias and their effects, reviewing statistical tests, and examining statistical vs. clinical significance. This is a study guide, not a past paper.
Full Transcript
EBM Bias, CI & Statistical Tests 2024 Laura Benjamins, MD, MPH Jason Booza, PhD Objectives: Understand different types of biases and how they affect clinical research Discuss measures of Central Tendency and Standard Deviation...
EBM Bias, CI & Statistical Tests 2024 Laura Benjamins, MD, MPH Jason Booza, PhD Objectives: Understand different types of biases and how they affect clinical research Discuss measures of Central Tendency and Standard Deviation Explain: Confidence Intervals, P-Values, Power, Type I & II Errors Review common statistical tests Review statistical vs. clinical significance Why Understanding Bias is Important in EBM Improves the quality of research By understanding and minimizing bias, researchers can design studies that more accurately reflect the true effects of interventions. Enhances patient care Clinical decisions based on biased evidence may lead to inappropriate treatments or missed opportunities for effective care. Recognizing bias allows clinicians to critically evaluate the strength of evidence and make better-informed decisions for their patients. Facilitates informed interpretation of results Different types of bias, such as selection bias, reporting bias, or publication bias, can influence the outcomes of studies. Understanding these biases helps clinicians interpret the results with caution and consider how these factors might impact the findings. Promotes transparency and accountability Acknowledging bias in research encourages transparency in reporting and strengthens the integrity of the medical literature. It also allows for more critical appraisal by clinicians, researchers, and policymakers. BIAS May be introduced during the study design, data collection, data analysis and publication. Definitions Lack of internal validity or incorrect assessment of the association between an exposure and an effect” – Delgado-Rodriguez and Llorca, 2004 “Deviation of results or inferences from the truth, or processes leading to such deviation” – Grimes and Schulz, 2002 Many types of bias can be introduced at various stages of research design and implementation. For a list of different biases and their definitions check out: https://catalogofbias.org/biases/admission-rate-bias/ EBM Bias, CI & Statistical Tests 2024 Laura Benjamins, MD, MPH Jason Booza, PhD Bias - Recruitment & Follow-up Selection Bias * How a study population is selected or treated (e.g. non-random allocation); study population does not reflect a representative sample of the target population. A sampling bias; external validity is questionable. Measures of association (e.g. RR, OR) can be distorted. Ex: Certain risk factors may be overrepresented in hospitalized patients (Berkson or Admission Bias). Randomization and allocation concealment can reduce selection bias. If losses to follow-up or withdrawals are uneven in the exposure and outcome categories, internal validity is affected; also called Attrition Bias. Performing the Study Recall Bias * Study group participants systematically differ in the way data on exposure or outcome are recalled. Especially problematic in case-control studies (especially if outcome was a long time from the exposure). Individuals who have a disease or adverse health outcome may “remember” possible “causes”. Ex: Mothers of children born with congenital heart defects may recall more illnesses during their pregnancy. Can help minimize by decreasing time from exposure to follow-up, or using medical records. Measurement Bias * Also called Classification or Information Bias. Results from systematic and improper, inadequate or ambiguous recording of factors – either exposure or outcome variables. Ex: Using a blood glucose monitor that has not been calibrated. Ex: Not collecting follow up data on all patients in the same manner. Can minimize this bias by using objective, standardized methods that are planned ahead of time, and administer equally to cases and controls (exposed/unexposed). Hawthorne effect – participants who are aware that they are being observed change their behavior; can be minimized by blinding or hidden measurement techniques. Procedure Bias Subjects in different groups are not treated the same way. Ex: Patients in the treatment arm spend more time being followed up in clinic. EBM Bias, CI & Statistical Tests 2024 Laura Benjamins, MD, MPH Jason Booza, PhD Observer Bias * A researcher’s belief about the effect of a treatment may be more likely to document certain outcomes. Ex: A physician who believes ivermectin cures COVID may report better outcomes among patients who received this drug (also called the Pygmalion effect). Blinding, use of placebo, randomization and standardization of follow-up and data end points can help minimize these biases. Interpreting the Results Confounding Bias * A characteristic related to both the exposure and outcome (but not in the causal pathway) can distort the effect of an exposure on an outcome. Can be minimized by randomization, cross-over studies, matching and stratification. Can also be corrected after the study by statistical adjustment. Confounding vs. Effect Modification Effect modification (also known as interaction) occurs when the effect of an exposure on an outcome differs depending on the level of a third variable, called an effect modifier. In other words, the relationship between an exposure and an outcome changes depending on the presence or value of another variable. Effect modification is not a bias but rather a true biological phenomenon that provides insights into how different subgroups or conditions may modify the effect of a particular exposure. EBM Bias, CI & Statistical Tests 2024 Laura Benjamins, MD, MPH Jason Booza, PhD Lead-Time Bias * Early detection can appear as a gain in survival, but the course of the disease has not changed. Ex: Patients diagnosed early with colon cancer by colonoscopy appear to survive longer. Length-Time Bias * A screening test detects diseases with a long latency period, while those with a shorter latency become symptomatic sooner. Ex: A slowly progressive cancer is more likely to be detected by a screening test. Can adjust survival based on the severity of the disease at the time of diagnosis, or randomize subjects to be screened or not screened. Publication Bias A.K.A. Study Selection Bias. Studies with significant or positive results are more likely to be published than studies with non-significant or negative results. Authors may not want to submit Reviewers may not recommend publication Editors may not accept If published, negative studies are quoted less Studies that are not in English Can affect systematic reviews and meta-analyses EBM Bias, CI & Statistical Tests 2024 Laura Benjamins, MD, MPH Jason Booza, PhD Intention To Treat Analysis Subjects analyzed based on the group they were originally assigned to, whether they drop out or not. Maintains randomization. Minimizes effect of attrition and non-adherence, but may dilute the true effects of the intervention. As Treated Analysis All subjects are analyzed based on the treatment they actually received. May increase bias. Per Protocol Analysis Only analyzes data from those subjects who strictly adhered to the study protocol. Because randomization is lost, confounders (both known and unknown) may not be equally distributed. MEASUREMENT OF DATA (VARIABLES) Quantitative Variables (Numerical) * Continuous Variables These are variables that can take any value within a range. They are measured on a continuous scale, meaning they have infinite possibilities within a given range. Examples: height, weight, and temperature. Discrete Variables These are quantitative variables that take on specific, countable values. They represent distinct categories or units that cannot be subdivided meaningfully. Examples: the number of children in a family, the number of hospital visits, or the number of patients. Qualitative Variables (Categorical) * Ordinal Variables Have a set order or ranking, but the intervals between categories are not necessarily equal. Ex. education level (high school, bachelor's, master's, PhD) or pain severity (mild, moderate, severe). Nominal Variables Represent categories with no inherent order or ranking. The values are purely labels. Ex. gender (male, female), blood type (A, B, AB, O), or race/ethnicity. EBM Bias, CI & Statistical Tests 2024 Laura Benjamins, MD, MPH Jason Booza, PhD Binary (dichotomous) Variables Can only take two distinct values. These values are often coded as 0 and 1, representing two mutually exclusive categories or outcomes. Commonly used in statistical models, especially in logistic regression, to represent outcomes or classifications. Ex. Yes/No questions, Presence/Absence of a condition, Success/Failure in an experiment. STATISTICAL DISTRIBUTION Measures of Central Tendency * Mean: The arithmetic average of a set of values. Calculated by adding all the numbers in a dataset and then dividing by the total number of values. The mean provides a general idea of the "center" of the data but can be influenced by extreme values (outliers). Median: The middle value of a dataset when the values are arranged in ascending or descending order. If the dataset has an odd number of values, the median is the exact middle value. If the dataset has an even number of values, the median is the average of the two middle values. The median is less sensitive to outliers compared to the mean. Mode: The value or values that appear most frequently in a dataset. There can be more than one mode if multiple values appear with the same highest frequency (bimodal or multimodal datasets). The mode is often used for categorical data but can also be applied to numerical data. Range: The difference between the largest and smallest values in a dataset. It provides a measure of the spread or dispersion of the data. However, the range only considers the two extreme values, so it may not fully reflect the variability of the entire dataset. EBM Bias, CI & Statistical Tests 2024 Laura Benjamins, MD, MPH Jason Booza, PhD EBM Bias, CI & Statistical Tests 2024 Laura Benjamins, MD, MPH Jason Booza, PhD Normal Distribution * A.K.A. Gaussian - a type of continuous probability distribution. Characterized by its symmetric, bell-shaped curve, which shows that data near the mean are more frequent in occurrence than data far from the mean. The key features Symmetry: The distribution is symmetric around the mean, meaning that the left and right halves of the graph are mirror images of each other. Mean, Median, and Mode are equal: In a perfectly normal distribution, the mean, median, and mode all fall at the same point in the center of the distribution. Bell-shaped curve: The shape of the distribution is often referred to as a "bell curve" because of its distinctive shape, with the highest point occurring at the mean, and the curve tapering off symmetrically on both sides. 68-95-99.7 Rule (Empirical Rule): * Approximately 68% of the data falls within one standard deviation (σ) of the mean. About 95% of the data falls within two standard deviations. Almost 99.7% of the data falls within three standard deviations. Non-Normal Distribution Important to recognize in data analysis as they affect the choice of statistical methods and interpretations, particularly when assumptions of normality are not met. Bimodal Has two distinct peaks or modes. These peaks represent the most frequent values in the dataset, and they may occur due to the presence of two different subgroups within the data. Ex. a distribution of human heights might be bimodal if the data includes both male and female individuals, as their heights tend to cluster around two different ranges. EBM Bias, CI & Statistical Tests 2024 Laura Benjamins, MD, MPH Jason Booza, PhD Positive (Right) Skew * Occurs when the tail on the right side of the distribution is longer or extends further than the left side. The majority of the data points are clustered toward the lower end of the range, while fewer data points are found at the higher values. As a result, the mean is typically greater than the median. Ex. income distributions, where most people earn lower incomes, but a small number earn significantly higher incomes, stretching the tail to the right. Negative (Left) Skew * Occurs when the tail on the left side of the distribution is longer or extends further than the right side. Most of the data points are concentrated at the higher end of the range, with fewer data points at lower values. As a result, the mean is typically less than the median. Ex. the distribution of ages at retirement, where most people retire at an older age, but a smaller number retire early, creating a left-skewed distribution. For data with a normal or bell shaped (gaussian) distribution, MEAN is the best measure of central tendency. For data that is skewed, MEDIAN is a better measure. EBM Bias, CI & Statistical Tests 2024 Laura Benjamins, MD, MPH Jason Booza, PhD MEASURES OF DISPERSION Standard deviation (SD) Measures the dispersion of a dataset relative to its mean. The standard error of the mean (SEM) Measures how much discrepancy is likely in a sample’s mean compared with the population mean. The SEM takes the SD and divides it by the square root of the sample size. The SEM will always be smaller than the SD. Quadrupling the sample size cuts the SEM in half. The larger sample size produces a smaller standard error of the mean. Variance (SD)2 A statistical measure that represents the spread or dispersion of a set of data points around their mean. It quantifies how much the values in a dataset vary from the average (mean) value. Variance is the square of the standard deviation (SD). The higher the variance, the more spread out the data points are. The lower the variance, the more closely packed the data points are around the mean. Since it is the square of the standard deviation, variance is expressed in the units of the data squared (e.g., if you're measuring height in meters, variance would be in square meters). CONFIDENCE INTERVALS * For a given statistic calculated for a sample of observations, the Confidence Interval is a range of values around that statistic that are believed to contain, within a certain probability (e.g., 95%), the true value of that statistic. The larger your sample size is, the narrower this range will be, as you get closer and closer to the “truth” about that population. EBM Bias, CI & Statistical Tests 2024 Laura Benjamins, MD, MPH Jason Booza, PhD The reason we generate confidence intervals is that it is usually impossible to sample an entire population. Therefore, we generate confidence intervals to estimate where the true value may lie. You can pick a 90% confidence interval, 99%, etc., but by convention most people choose a 95% confidence interval. This tells you that you can be 95% confident that the true value lies somewhere within the range of values given. HYPOTHESIS TESTING * Hypothesis Testing – Crucial in EBM Informs Clinical Decision-Making: provides a structured framework to evaluate the effectiveness of medical interventions, guiding practitioners to choose treatments backed by statistical evidence rather than anecdotal experience. Controls for Type I and Type II Errors: These errors are tightly controlled in clinical research to minimize the risk of making inappropriate treatment decisions. In EBM, avoiding Type I errors is particularly critical when approving new therapies, as false positives can lead to harmful clinical practices. Statistical Rigor: By setting predefined thresholds (p-values), hypothesis testing helps standardize the process of determining whether observed effects are statistically significant or due to random chance. This ensures that conclusions drawn from studies are based on quantifiable evidence. Null Hypothesis, HO Hypothesis that two possibilities are the same. No statistical difference exists in a set of observations. An observed difference is only due to chance. This hypothesis is deemed “true” until proven wrong by experimental data. Alternative Hypothesis, H1 Establishes a relationship between two variables. Directly contradicts the Null Hypothesis. P-Value The measured probability rejecting the null hypothesis, by chance alone, given that the null hypothesis is actually true. By convention, a p-value < 0.05 is often considered significant. ("There is less than a 5% probability that the finding was due to chance alone.") Power The probability of detecting a difference. Typical power probabilities are 80%. 1 – β. The higher the power the larger the sample size will need to be. Ex: "The study had a power of 80% to detect a difference of 5 mm Hg in diastolic blood pressure between the treatment and control groups." EBM Bias, CI & Statistical Tests 2024 Laura Benjamins, MD, MPH Jason Booza, PhD Type I Error (α) Mistakenly rejecting the null hypothesis when it is actually true. The maximum probability of making a Type I error that the researcher is willing to accept is call alpha. Smaller α = less chance of Type I Error. Alpha is determined before the study begins, commonly set at to 1 in 20 (=0.05). Type II Error (β) Mistakenly accepting (not rejecting) the null hypothesis when it is false. The probability of making a Type II error is called beta. For trials, the probability of a β error is usually set at 0.20 or 20% probability. A 20% chance of missing a true difference. A larger sample size decreases the chance of making a Type II Error. Great Video: https://www.youtube.com/watch?v=985KQG-8QV8 HO is rejected (and results ARE significant) when: - 95% CI for mean difference excludes O (zero) - 95% CI for OR or RR excludes 1 (one) - CIs between two groups DO NOT overlap HO is NOT rejected (and results are NOT significant) when: - 95% CI for mean difference includes O (zero) - 95% CI for OR or RR includes 1 (one) - CIs between two groups DO overlap CI = 1 - α - As sample size increases, CI narrows (becomes more precise) EBM Bias, CI & Statistical Tests 2024 Laura Benjamins, MD, MPH Jason Booza, PhD STATISTICAL TESTS Statistical tests are the backbone of hypothesis testing and are essential in analyzing data across various types of research. They help quantify relationships between variables, assess the effectiveness of interventions, and provide evidence to either support or refute hypotheses in scientific inquiry. Understanding the type of variables involved ensures that the right statistical tests are applied, making the conclusions of the research robust and reliable. Statistical Tests and Hypothesis Testing: Statistical tests are used to determine whether there is enough evidence to reject the null hypothesis (which typically states that there is no effect or no difference). Depending on the type of data and distribution, various statistical tests are applied (e.g., t-tests, chi-square tests, ANOVA, etc.). These tests provide p-values that help assess the probability that any observed difference is due to chance. If the p-value is below a predetermined significance level (commonly 0.05), the null hypothesis is rejected, indicating statistical significance. Statistical Tests in Research: In observational studies, statistical tests can determine the association between variables (e.g., correlation, regression). In experimental research, such as clinical trials, statistical tests are used to compare outcomes between groups to evaluate the effect of an intervention or treatment. Descriptive statistics (mean, median, mode, etc.) help summarize and describe the basic features of data, while inferential statistics use data from a sample to make inferences about the larger population. EBM Bias, CI & Statistical Tests 2024 Laura Benjamins, MD, MPH Jason Booza, PhD Statistical Assumptions Independence of observations (a.k.a. no autocorrelation): The observations/variables you include in your test are not related (for example, multiple measurements of a single test subject are not independent, while measurements of multiple different test subjects are independent). Homogeneity of variance: the variance within each group being compared is similar among all groups. If one group has much more variation than others, it will limit the test’s effectiveness. Normality of data: the data follows a normal distribution (a.k.a. a bell curve). This assumption applies only to quantitative data. If your data do not meet the assumptions of normality or homogeneity of variance, you may be able to perform a nonparametric statistical test, which allows you to make comparisons without any assumptions about the data distribution. If your data do not meet the assumption of independence of observations, you may be able to use a test that accounts for structure in your data (repeated-measures tests or tests that include blocking variables). The most common types of parametric test include regression tests, comparison tests (t-tests and ANOVA), and correlation tests Pearson’s r). One of the most common types of non-parametric test is the Chi Square test of Independence. Parametric Tests Parametric tests are statistical tests that assume underlying parameters about the population distribution from which the data is drawn. Typically, these tests require the data to follow a normal distribution (or at least approximately normal), and they often assume homogeneity of variance (equal variance among groups). These assumptions allow parametric tests to have higher statistical power when the conditions are met, meaning it can more easily detect differences or effects. Provides more detailed information about data relationships. Common Assumptions of Parametric Tests: -Normal distribution of the data. -Equal variances across groups being compared (homoscedasticity). -Interval or ratio level data (continuous data). Examples of Parametric Tests: -t-test:* Compares the means between two groups. -ANOVA (Analysis of Variance): Compares the means between three or more groups. -Pearson's correlation: Measures the strength of a linear relationship between two continuous variables. -Linear regression: Assesses the relationship between a dependent variable and one or more independent variables. EBM Bias, CI & Statistical Tests 2024 Laura Benjamins, MD, MPH Jason Booza, PhD Nonparametric Tests Do not rely on strict assumptions about the population distribution. They are used when the data does not meet the assumptions required by parametric tests (such as normal distribution or equal variances). Nonparametric tests are more flexible and can be used with ordinal data or when the sample size is small. Advantages: Fewer assumptions about the underlying data, making them more robust in cases of non-normality or heteroscedasticity. Suitable for analyzing ordinal data or non- continuous variables. Characteristics of Nonparametric Tests: -No assumption of normality in the population distribution. -Can handle ordinal, ranked, or categorical data. -Can be used when sample sizes are small, when the data has outliers or non-constant variance. Examples of Nonparametric Tests: -Chi-Square:* Tests the association between two categorical variables. -Fisher’s Exact Test: Tests association between two categorical variables in small sample sizes. -Mann-Whitney U test: A nonparametric equivalent of the t-test, used to compare differences between two independent groups. -Wilcoxon signed-rank test: A nonparametric alternative to the paired t-test. -Kruskal-Wallis test: A nonparametric equivalent to ANOVA, comparing three or more independent groups. EBM Bias, CI & Statistical Tests 2024 Laura Benjamins, MD, MPH Jason Booza, PhD Good videos explaining which test to use: https://www.youtube.com/watch?v=NRw8pNn-WmM https://www.youtube.com/watch?v=g_-SJiMjvo8 Statistical Significance Refers to the likelihood that an observed effect in a study is due to something other than chance, usually determined using a p-value (e.g., p < 0.05). Helps determine if the results of a study are meaningful from a purely mathematical standpoint, but does not reflect the practical impact of the findings. A statistically significant result means there is strong evidence against the null hypothesis, suggesting the effect observed is unlikely to occur randomly. Example: A new drug reduces blood pressure by 2 mmHg compared to a placebo, with a p-value of 0.02. This reduction is statistically significant, but the clinical relevance of such a small reduction may be questionable. Clinical Significance Refers to the practical importance of a treatment effect, specifically how meaningful the results are in real-world, patient-centered outcomes. Focuses on whether the effect size has enough magnitude to have a noticeable or beneficial impact on a patient's health or quality of life. EBM Bias, CI & Statistical Tests 2024 Laura Benjamins, MD, MPH Jason Booza, PhD A clinically significant result leads to a meaningful improvement in a patient's symptoms, function, or overall health, regardless of whether it’s statistically significant. A result can be statistically significant but not clinically significant, and vice versa. Example: A weight loss program results in an average 10% reduction in body weight. While the p-value is 0.07 (not statistically significant), a 10% weight loss is considered clinically significant as it can improve health outcomes, like reducing the risk of diabetes. Example Combining Both: A cholesterol-lowering medication decreases LDL cholesterol by 50 mg/dL with a p-value of < 0.001 (statistically significant) and a clinically significant effect because reducing LDL by this magnitude lowers the risk of heart disease. Contact information for questions: Laura Benjamins, MD, MPH, FAAP (she/her/hers) Professor, Adolescent Medicine Office: 313-448-9600 Cell: 832-419-4738