BA Reviewer: Application of Data Analysis in Business PDF
Document Details
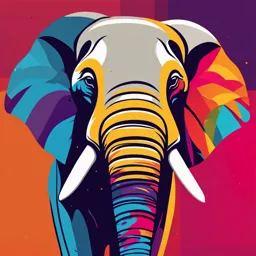
Uploaded by LavishEarth
Tags
Related
Summary
This document discusses the application of data analysis in business, highlighting its importance in decision-making, especially in e-commerce, with examples of companies like Shopee. The document also explores various types of data analysis and how they help improve business objectives in different departments.
Full Transcript
**[BA REVIEWER]** *Unit 4:Application of Data Analysis and Its Significance to Business* **Why Use Data Analytics?** - The importance of precisely interpreting the data could not be emphasized sufficiently. Your website, company, etc., [must have skilled professionals who distinguish ho...
**[BA REVIEWER]** *Unit 4:Application of Data Analysis and Its Significance to Business* **Why Use Data Analytics?** - The importance of precisely interpreting the data could not be emphasized sufficiently. Your website, company, etc., [must have skilled professionals who distinguish how to take data plus interpret the outcomes correctly]. For example, your company must examine data from two of the most prevalent social media, Facebook and Twitter. It\'s [not feasible to rely on an untrained individual to handle customer interactions on social media platforms.] - This is where a **Social Media Manager** comes in. [These professionals are adept at representing your brand in their responses and have a deep understanding of each social platform, making them an invaluable asset to your company. ] [Hiring professionals trained to transform unstructured and random data into a coherent structure is crucial.] This transformation can significantly alter the decision-making process based on the data and the operational strategies of your company, highlighting the value of their expertise. Trained professionals can trace the customers\' behavior concerning the use of your product and take all of your clients\' \"likes\" on your business Facebook page. Follow the decision-making procedure of these customers. If the customers like your product, then what? Do they reap the profits of using your product? Do they read the product description? What makes your firm\'s product better than the rest of the competition? Is your product sensibly priced in contrast to your competitor\'s values? - **Trained data analysts** [can analyze the outline your customers will take and trace these questions. They will follow the trace that the customers make. They can investigate the data from your customers\' unique \"likes\" to the buying on your website. ] The right persons with the training to follow and analyze this process [can aid your company in generating increased product sales]. They tool this info and disseminate it to the suitable team members through the company. Having meaningful and correctly interpreted data may be the difference between your company expanding its effect or shutting down owing to misinterpreted statistics. *An example of studying tweets is interpreting ancient tweets and distinguishing the considerable \"tweet\" from the casual \"tweet.\"* Data interpreters can analyze the effect of such \"tweets\" on customer buying habits and analyze historical data from preceding company \"tweets.\" These experts can translate which \"tweets\" are just social and which \"tweets\" are considerable. The analyst is competent in tracing the impact on the customer\'s initial mindset as to whether they will aid in achieving the company\'s core objective of buying the product from the initial root message texted on Twitter. *Which text is more considerable than others? Why is it more effective? Do images with the \"tweets\" tend to convince your customer base to purchase your product? Which \"tweets\" work finest in what areas of the world? Which \"tweets\" work most excellently with whatever age group? * [These are essential questions that data could answer. ] They identify what marketing strategies are working the best on each platform, and they show why it is significant to have analysts review them. [Analysts can understand large amounts of data using visual graphs showing statistical figures.] These can be given to suitable departments so they can make decisions to improve the general sales experience for your clientele. **The significance of data analytics for your business:** Data can improve the efficacy of your business in several ways. Here is the essence of how data can play a significant role in improving your operations. * Improving Your Promotion Strategies* Based on the data collected, [it is easier for a company to develop inventive and attractive marketing approaches.] It is easier for a firm to alter current marketing strategies plus policies in such a style that they align with current trends and customer expectations. E-commerce shopee.ph, for example, as shown below. Of course, you would have heard of this business, visited its website, and purchased items from it. Shopee is one of the virtual space consumers of analytics. Shopee\'s personalization approach is based on their website\'s resources and user details. As you can see below, we have an example of what Shopee does for its customers. For example, suppose you\'re looking for a face mask because it\'s essential to protect yourself from being infected with COVID-19. In that case, Shopee gives you five suggestions for different types of face masks that you may be interested in depending on what you\'re looking for. This means that Shopee can provide robust, strictly data-driven recommendations. Now, if Shopee were a small store, if it were a single brick-and-mortar shop, it would be run by someone who is very experienced in face masks and can give a lot of advice to people who come to the store. But, since Shopee has been online. Their customers are distributed around the country and do hundreds of transactions every second of the day; a single individual or even a group can\'t sit behind the Shopee website and make suggestions to people when they visit it. It\'s got to be done in an automated way, and this is where the analytics come into the picture. Shopee has developed sophisticated tools to predict the other products the consumer will be interested in based on the first product the customer is looking for. When consumers are pleased with the suggestions, they will buy more products and return to Shopee\'s website, eventually increasing Shopee products. This is again an example of an analytics-based company being more competitive, enhancing marketing strategies, and offering more value to its customers. \* Classifying Pain Points * Data can [help you categorize deviations from the usual if prearranged processes and patterns drive your business.] These slight deviations might be the reason behind the sudden increase in customer complaints, decrease in sales, or decrease in productivity. You will be capable of catching these little accidents early and taking educative actions with the aid of data. * Detecting Scam * It will be [easier to notice fraud committed when you have the numbers]. For example, when you have the acquisition invoice of 100 units of tins and see from your sales reports that merely 90 tins have been sold, you misplaced ten tins from your list and know where to look. Most companies are unaware of the fraud being committed in the first place and are silent victims of deception. One significant reason is the lack of suitable data management, which might have helped prevent fraud easily in the initial stages. * Recognize Data Breaches * The blast of complex data streams in the previous few years has brought new difficulties in the region of fraudulent practices. They have become delicate and complete. Your company's retail, payroll, accounting, and other business systems are adversely impacted by their adverse effects. In other words, data hackers would become more devious in their attacks on business data systems. Your company can stop fraudulent data compromises in your system, which can strictly cripple your business by using data analytics plus triggers. **Data analytics tools** [allow your firm to develop data testing procedures to detect early signs of deceitful activity in your data system.] Standard fraud testing might not be feasible in certain conditions. Specially tailored tests can be developed to trace any probable fraudulent activity in your data procedures if this is the case with your company. Traditionally, to investigate fraud plus implement breach stoppage strategies, companies have waited till their operations have been impacted fiscally. This is no longer viable in today's rapidly altering data-saturated world. With info being disseminated globally so rapidly, undetected fraudulent action can negatively affect a firm and its subsidiaries in no time worldwide. Contrariwise, data analytics testing could stop potential fraudulent data annihilation by revealing indicators that deception has begun to seep into data systems. If these data analytic tests are applied occasionally, fraud can be stopped rapidly for a company and its partners worldwide. * Improving Client Experience * Data also comprises the feedback provided by clients, as I mentioned beforehand. You will be able to work on areas that can aid you in improving the excellence of your product or service based on their feedback and satisfy the customer. Similarly, [you can modify your product or service better when you have a repository of client feedback.] For instance, some firms send out customized private emails to their clientele. This sends a message that the firm genuinely cares about and wants to satisfy its clientele. This is possible exclusively owing to effective data management. * Decision Making * Data is crucial for creating important business choices. For instance, it is essential first to collect data about the current market trends, the competitors\' pricing, the size of the consumer base, etc., if you want to launch a novel product in the marketplace. If data do not drive the decisions made by the company, something other than that could cost the company a lot. For instance, there is an option that your product might be high-priced if your firm decides to launch a product without seeing the price of the contestant\'s product. As with most high-priced products, the company would be concerned about growing the sales figures. I do not just refer to the choices about the product or service presented by the company When I say decisions. Data can also be valuable in making decisions concerning the role of departments, workforce management, etc. For example, data can aid you in assessing the number of workers required for the operative functioning of a division that is in line with the business necessities. This info can help you decide whether a definite division is overstaffed or otherwise understaffed. * Hiring Procedure * Using data to choose the right personnel is a deserted practice in business life. It\'s critical to place the most competent person in the correct job in your company. You want your business to be highly successful in each facet of operation. Using data to employ the right person is a sure method to put the best individual in the job. What kinds of data would you use to appoint a professional? For instance, you can use data from job applications, performance reviews, and even social media profiles to assess a candidate\'s skills, experience, and cultural fit. Big companies with astral budgets use big data to locate and choose the most skilled persons for the correct jobs. Start-ups and small firms would benefit hugely from using big data to appoint the right group of persons to make their recruitment prosperous from the start. The process involves collecting and analyzing data from various sources, such as job applications, social media profiles, and performance metrics, to identify the best candidates. For hiring the correct fit for groups of all sizes, This Avenue has proven effective for collecting data for hiring drives. Yet again, companies can use their data scientists to excerpt and interpret the specific data required by human resource divisions. \* Using Social Media Platforms to Recruit * For finding high-profile applicants for the correct positions within firms, social media platforms (Twitter, Facebook, and LinkedIn, to name a few) are concealed gold mines as data sources. Take Twitter, for example; firm job recruiters can follow persons who tweet precisely about their business. A company can find and recruit the perfect candidates founded on their knowledge of a precise industry or job inside that industry through this process. Do their \"tweets\" motivate new thoughts and possibly new inventions for their business? If so, you have a whole pool of prospective job applicants. Facebook is an alternative option for data collecting for potential job applicants. Corporations might use them as part of a primary cost-effective strategy, and these avenues are virtually free. Facebook is about gathering social networking data for firms looking to expand their staff or replace an open position. Company recruiters could join industry niche groups or otherwise niche job groups. "Liking" and following group members' comments will allow highly motivated job ads to be posted within the group and establish the firm's presence. The company can upsurge views, spreading the pool of prospective job candidates. Engaging with friends and followers in your industry is not just about establishing your company\'s presence, it\'s about building a community. By doing so, you can promote your job advertisement for a minimal fee and significantly increase your reach among potential job seekers. This approach can attract more highly accomplished job searchers, increasing the chances of finding the perfect fit for your job if your firm issues highly functioning job data posts. * Niche Social Group * Niche social groups [are particular groups you can join on social plus web platforms that can aid you in finding precise skill sets.] For example, what better place to find a prospective recruit than by joining a precise human resources social group if you are seeking to hire a human resources manager? You may find the correct person to fit into your human resources place by Locating social connections inside that group and then posting expressive but appealing job data posts. Group members will undoubtedly have referrals Even if your firm needs help finding the right person. Again, approaching these crowds is a cost-effective way to promote your job posts. * Innovative Data Collecting Methods for the Appointment Process * Why not try new approaches to data collection to appoint the right professional and think outside the hiring process box? Use social collecting data websites that collect data, such as Google+, LinkedIn, Facebook, and Twitter. Your company can extract relevant data from posts made by prospective job candidates plus search on these sites. Such data can help your company connect with highly effective job applicants. Keywords are a perfect data pool for usage. Keywords are used on the internet for every type of exploration imaginable. Why not use the most noticeable keywords in your online job description? Your firm can widely upsurge the number of views that your job posting will entice by doing this. You can also use PCs and software to find the right job candidate for your firm. Traditionally, analyze whether a current employee is a correct fit for another job or whether these data sources have been used to dismiss a company\'s employee. Why not try a whole new data-gathering system? This system would be fixed in standards diverse from the usual IQ tests, skill testing, or physical exams. These are still valued tools to measure candidates; however, they are limiting. Another focus can be the sturdy personality traits an applicant may possess. Is the individual negative? Is the person an isolationist who doesn't get together with other people? Is the person argumentative? These people can be recognized through this personality trait database and filtered out as probable team members. This data type will save the firm time, resources, and training resources when correctly extrapolated. The company has the job and the individual who could fill the job by eliminating a gap between the anticipations. Another advantage of this data-gathering system is that the results will identify people with the right persona to fit in with the present company culture and skilled persons for the correct jobs. The system will do this by \[explaining the process or algorithm used to match skills with job requirements\]. A person must be able to engage other staff to produce the most effective working relations and be sociable. The healthier the working atmosphere, the more effective firm production is. \* Gamification * This is an exclusive data tool that isn't presently in extensive use. It motivates applicants to press in and put forth their finest effort in the job selection procedure. You provide persons with "badges" and additional virtual goods to inspire them to persevere. Their ability to perform the job necessities will be eagerly apparent. This also makes the job application a fun experience in place of a typical tedious task. * Job Previews * Pre-planning the job employing process with precise data about the job requirements will let the job seeker know what to expect if they are appointed for the position. It is said that lots of learning on the work is by trial and error, which extends the learning procedure. It takes more time to get the worker up to speed to function competently as a valuable resource inside the company. Including the job preview data into the appointment process decreases the learning curve and aids the employee in becoming effective much quicker. Companies can use inventive data-gathering approaches to streamline the hiring procedure. They also aid the human resource department choose the most skilled persons to fill their employment requirements. Hence, data is vital in aiding trades in making effective verdicts. These are some of the details for the effective functioning of a business and why data is crucial. Let us get into the other data analysis features in the upcoming chapters, as we have had a glimpse at the significance of data. *Unit 5: Types of Business Analytics* **[1. Descriptive Analytics]** - Descriptive analysis, [the most commonly used business analysis form, is also the oldest.] - It provides the necessary insights for future forecasting, similar to the role of intelligence organizations in governments. - This type of analysis, often called **business intelligence**, [involves examining past data through data aggregation and mining techniques, thereby, providing valuable insights for business planning and decision-making. ] - As the name suggests, [descriptive analysis describes previous events.] We can turn such data into facts and statistics that are understandable to humans, thereby permitting us to use this data to plan our future events by using various data mining methods and processing statistics. - Descriptive analytics allows us to learn from previous events, whether they happened a day or a year ago, as well as to use this data to predict how they might affect future behavior. - For instance, if we can see trends similar to rising or falling numbers and are aware of the regular number of product sales we made per month in the previous three years, we can expect how these trends would influence future sales. Otherwise, we can see that the quantities are going down. This means we must change somewhat to get the sales back up, whether re-branding, increasing our team, or presenting new products. - *A maximum of the statistics businesses use in their daily operations falls into the category of descriptive analysis.* - [Statisticians] would collect descriptive statistics from the past and then convert them into a language comprehensible to the management and employees. - Using descriptive analysis [permits businesses to see how much of the product sales proportion falls to expenses, how much they are expending on average on several expenses, and how much is perfect profit.] All of these permit us to cut corners and make more revenue, which is the term of the game in business. **Process Involved Using Business Analytics** *Data:* We need data to be collected and a repository to access essential data. *Analyze:* We need to analyze the data we collected and the Data Model to assess and query the data collected. *Generate Reports:* Business Users can choose the required reports. (Sales, Financial, Distributions, Inventory, and similar reports). *Smart Decisions:* Our analysis is not just a process, it\'s a game-changer. The ways we analyze the data can lead to smarter decisions. Analytics is the key that allows Managers to create a better and smarter future for our business. "In analyzing, we need to visualize the data, create a modeling structure, and make preparations for drilling different data. These steps work together to comprehensively understand the data, which is crucial for better decision-making. (Drilling imposes drilling up and drilling down) What kind of analysis? What kind of data? What kind of reasoning? There are no hard-and-fast answers; we contend that almost any analytical process can be good if provided seriously and systematically." (Davenport, Harris, and Morison, 2012). *[How Can We Use Descriptive Analysis? ]* - Descriptive statisticians typically transform data into understandable results, such as reports with charts that display what kind of patterns a business has seen in the past graphically and clearly, enabling the organization to predict the future by using a range of specialized solutions without compromising the capabilities of Microsoft Excel. The method is currently very open to students as a specialized solution and is very expensive. - **Microsoft Excel** has broad computational capabilities to conduct mathematical analysis with enhanced visibility. Today, Excel comes with in-memory technology that makes it easily compatible with millions of bytes of data, enabling businesses to perform the required analysis without relying on specialized solutions. [The Excel environment makes data exploration and results deriving relatively easy.] It includes features that perform robust data analytics processes with just one click instead of using complex formulas. **[Values in Descriptive Analysis ]** - There are two main ways of describing data: *measures of central tendency* and *measures of variability or dispersion.* - When **[measuring a central tendency]**, we mean [measuring data and finding the *mean value* or average from a given data set.] This mean is determined by summing up all the data and dividing it by the number of data units, getting an average value that can be used in various ways. - **Another unit used in measuring the central tendency,** which tendency -- which is even more helpful and valuable -- is the *[median]*. Unlike the mean, [the median considers only the middle value of a given data set]. For instance, the fifth number is considered the median in a string of nine numbers. If we arrange all our numbers from lowest to highest, the median will often be a more reliable value than the mean because there could be outliers at either end of the spectrum, which bends the mean into a wrong number. The outliers are extremely small or big numbers that will naturally make the mean unrealistic, and the median will be more useful in cases with outliers. - [**Measuring the dispersion or variability** allows us to see how spread out the data is from a central value or the mean]. The values used to measure the dispersion are range, variance, and standard deviation. - *The range is the simplest method of dispersion.* The [range is calculated by subtracting the smallest number from the highest.] This value is also susceptible to outliers, as you could have an extremely small or high number at the ends of your data spectrum. - **Variance** is the [measure of deviation that tells us the average distance of a data set from the mean.] Variance is typically used to calculate the standard deviation, and by itself, it would serve little purpose. *Variance is calculated by calculating the mean, subtracting the mean from each data value, squaring each value to get all positive values, and then finding the sum of these squares.* Once we have this number, we will divide it by the total number of data points in the set, and we will have our calculated variance. - ** Standard deviation** is the [most popular dispersion method as it provides the average distance of the data set from the mean.] The variance and standard deviation will be high when the data is highly spread out. *You will find the standard deviation by calculating the variance and then finding its square root.* The standard deviation will be a number in the same unit as the original data, which makes it easier to interpret than the variance. All of these values used to calculate the central tendency and the dispersion of data can be employed to make various inferences, which can help with future predictions made by predictive analytics. *[Inferential Statistics ]* Inferential statistics is the part of analysis that allows us to make inferences based on the data collected from descriptive analysis. These inferences can be applied to the general population or any larger group that is more significant than our study group. For example, if we conducted a study that measured stress levels in a high-pressure situation among teenagers, we could use the data to predict general stress levels among other teenagers in similar situations. By incorporating data from other studies, we could even predict stress levels in older or younger populations. While these predictions may not be perfect, they can still be used with a degree of credibility. **[2. Diagnostic Analytics ]** - Diagnostic analytics is characterized by drill-down, data mining, data discovery, and correlations. It is a form of advanced analytics that inspects data or content to respond to the question, *"Why did it occur?"* Diagnostic analytics deeply looks at data to understand the causes of events and behaviors. *[What Are the Benefits of Diagnostic Analytics? ]* - [Diagnostic analytics lets you understand your data faster to answer critical workforce questions.] - **Cornerstone View** [provides the fastest and simplest way for organizations to gain more meaningful insight into their employees and solve complex workforce issues.] - **Interactive data visualization tools** [allow managers to quickly search, filter, and compare people by centralizing information across the Cornerstone unified talent management suite.] For example, users can find the right candidate to fill a position, select high-potential employees for succession, and quickly compare succession metrics and performance reviews across select employees to reveal meaningful insights about talent pools. - Filters also allow for a snapshot of employees across multiple categories such as location, division, performance, and tenure analytics. **[3. Predictive Analytics ]** - In simple terms, predictive analytics [is the art of obtaining information from collected data and utilizing it to predict behavior patterns and trends.] With the help of predictive analytics, [you can predict unknown factors, not just in the future but also in the present and past.] - For example, predictive analytics can identify suspects of a crime that has already been committed. It can also be used to detect fraud as it is being committed. ***[Process Involved using Predictive Analytics]*** *Data:* The collection of data is crucial as it serves as the foundation for making smart decisions. A data repository is essential for accessing this data. *Analyze:* We need to analyze the data we collected. This involves examining the Data Model, a visual or mathematical representation of the data\'s structure, *Provides Prediction:* In this step, predictions are now provided so that business users can define and track the following steps in their business analytics before they happen. *Intelligent Decisions:* We need to decide how we analyze the data. Analytics can allow managers to make better and brighter decisions. Many approaches to analysis are fair game, from the latest optimization techniques to tried-and-true versions of root-cause analysis. Perhaps the most common is statistical analysis, in which data are used to make inferences about a population from a sample. Variations of statistical analysis can be used for a huge variety of decisions---from knowing whether something that happened in the past was a result of your intervention, to predicting what may happen in the future. Statistical analysis can be powerful, but it's often complex and sometimes employs untenable assumptions about the data and the business environment. ***[What are the Types of Predictive Analytics? ]*** Predictive analytics could be referred to as **predictive modeling.** In modest terms, it is the [act of combining data with predictive models and arriving at a conclusion]. Let us look at the three models of predictive analytics. *Predictive Models* Predictive models are nothing; however, they are models of the relationship between the precise performance of a definite element in a sample and a few known qualities of the sample. - This model evaluates the probability that a similar element from a diverse sample might exhibit a similar performance. It is extensively used in marketing. In marketing, predictive models classify subtle patterns and identify clients' preferences. - These models are proficient in performing calculations as plus when a definite transaction occurs, i.e., live dealings. For instance, they are accomplished by evaluating the chance or risk related to a particular deal for a given client, helping the client decide if he or she wants to enter the deal. - Given the developments in calculating speed, individual agent modeling schemes have been designed to simulate human reactions or conduct for specific situations. *Predictive Models in Relation to Crime Scenes* To help police departments and criminal investigators rebuild crime scenes without violating the reliability of the proof, 3D technology has brought big data to crime scene studies. There are two kinds of laser scanners used by crime scene specialists. *Time-of-flight laser scanner* The scanner shoots out a ray of light that bounds off the targeted thing. Diverse data points are measured as the light yields to the sensor. It's accomplished by gauging **50,000 points per second.** *Phase shift 3D laser scanners* These scanners, while more costly, are remarkably efficient, capturing **976,000 data points per second.** They operate using infrared laser technology. These data laser scanners make crime scene rebuilding much easier. The process takes a lot less time than customary crime scene rebuilding takes. - The benefit of 3D technology is that investigators could re-visit the corruption scene anywhere. Investigators could now do this at home, in their workplaces, or in the field. This makes their occupation more mobile, and they can visit crime scenes almost anywhere. They no longer have to rely on notes or their memories to recall the details of the crime scene. Furthermore, they visit the crime scene one time, and that is it. They have all the data imageries recorded on their scanner. Investigators can re-visit crime scenes by viewing the images on computers or iPads. The distance between objects will be reviewed (like weapons.) The beauty of this technology is that crime experts do not have to guess second the information gathered from crime scenes. The original crime scene is constructed right there on the scanner images. It is as if the crime was committed right there on the scanned images. The images tell the story of the perpetrators and how they committed the crime. Investigators can look at the crime scenes long after they are released. Nothing in the crime scene will be disturbed or compromised. Any compromised evidence is inadmissible in court and cannot be used. All evidence must be left in the original state and not be tampered with. This is fine when the evidence is recorded in the data scanners. Law enforcement engineers can reconstruct the whole crime scene in a courtroom. Forensic evidence is untouched and left intact, thus guaranteeing a higher rate of convictions. *Forensic Mapping in Crime Scene Rebuilding* The persistence of 3D forensic mapping is to rebuild every detail of the crime scene holistically. This is a pure way of rebuilding crime scene proof. None of the proof is touched or, by accident, thrown away. Investigators do not have to walk in or around the evidence, avoiding the option of anything being accidentally dropped or otherwise kicked. ***[Predictive Analysis Techniques ]*** *Regression Techniques* These methods form the base of predictive analytics. They object to establishing a mathematical equation, which would, in turn, serve as a model for signifying the interactions amongst the different variables in question. Different models could be applied for the execution of predictive analysis Founded on the circumstances. Let us look at several of them in detail nowadays below. *Linear Regression Model* \* * This model evaluates the relationship between the set of independent variables related to it and the dependent variable in a given state. This is usually uttered in the formula of an equation. The dependent variable is uttered as a linear function of diverse parameters. These parameters could be adjusted in such a way that it leads toward optimizing the measure of fit. The objective of this model is to select parameters to minimize the sum of the squared residuals. This is known as the ordinary least squares estimation. Once the model is estimated, the statistical significance of the different coefficients used in the model must be checked. This is where the t-statistic comes into play, whereby you test whether the coefficient differs from zero. The ability of the model to predict the dependent variable depending on the value of the other independent variables involved can be tested by using the R2 statistic. *Discrete Choice Models* Linear regression models are continuous and could be used in cases where the dependent variable has a limitless variety. However, there are definite cases where the dependent variable is not incessant. In such cases, the dependent variable is distinct. *Logistic Regression* A categorical variable has a static number of values. For example, if a variable can take two standards at a time, it is named a binary variable. Categorical variables with more than two values are mentioned as polytomous variables. One instance is the blood kind of a person. Logistic regression determines and measures the relationship between the categorical variable in the equation and the other independent variables associated with the model. This is done by utilizing the logistic function to estimate the probabilities. It is similar to the linear regression model. However, it has different assumptions associated with it. There are two significant differences between the two models. They are as follows: The linear regression model uses a Gaussian distribution as the conditional distribution, whereas the logistic regression uses a Bernoulli distribution. The predicted values arrived at in the logistic regression model are probabilities and are restricted to 0 and 1. The logistic regression model is capable and highly effective in predicting the probability of specific outcomes, providing a high confidence level in its predictions. *Probit Regression* Probit models are used on behalf of logistic regression for forthcoming models for categorical variables. This is used in the case of binary variables, namely, categorical variables, which could take only two values. This technique is used in economics to forecast models that use variables that are not simply continuous but also binary. *Machine Learning Techniques* Machine learning is an arena of artificial intelligence. This is initially used to develop methods that will ease computers to learn. Machine learning contains an array of statistical approaches for classification plus regression. This is now being hired in dissimilar fields, such as medical diagnostics, credit card fraud detection, face recognition, speech recognition, and stock market study. *Neural Networks* Neural networks are non-linear modeling methods and classy capable of modeling compound functions. These methods are extensively used in neuroscience, finance, cognitive psychology, physics, engineering, and medicine. *Multilayer Perceptron * The multilayer perceptron is prepared of an input layer plus an output layer. Besides these, one or more hidden layers are prepared of nonlinearly activating nodes, which are otherwise sigmoid. The weight vector plays a significant role in regulating the network weights. *Radial Basis Functions* A radial basis function has an inherent distance criterion in link with a center. These functions are usually used to interrupt and smooth out data. These functions have furthermore been used as a portion of neural networks on behalf of sigmoid functions. In such cases, the net has three layers: the hidden layer through the radial basis functions, the input layer, and the output layer. *Support Vector Machines* Support vector machines are hired to exploit and detect complex outlines in data. This is accomplished by classifying, clustering, and ranking the data. This learning machinery can be used to perform regression estimates and binary categorizations. There are numerous support vector machines, counting polynomial, linear, and sigmoid, to name a few. *Naïve Bayes* Naïve Bayes, a method based on the Bayes conditional probability rule, is a straightforward and easy-to-use tool. It employs pre-built classifiers to classify various tasks, leveraging the assumption of statistically independent predictors. This simplicity and ease of use make it a powerful tool for classification tasks. *K-Nearest Neighbors* The K-Nearest Neighbors algorithm, a part of the pattern recognition statistical methods, operates without any underlying assumptions about the sample\'s distribution. It consists of a training set with both positive and negative values. When a new sample is drawn, it is categorized based on its distance from the nearest neighboring training set, offering a non-restrictive and flexible approach to pattern recognition. *Geospatial Predictive Modeling* The underlying standard of this technique is the supposition that the incidences of the events being modeled are restricted in terms of circulation. In other words, the events of events are neither arbitrary nor uniform in circulation. Instead, additional spatial environment issues, such as infrastructure, socio-cultural, topographic, etc., are involved. *Deductive method.* This method is founded on a subject matter expert, a person with deep knowledge and expertise in a specific field, who provides qualitative data. Such data is then used for the drive of describing the association that exists among the occurrence of an occasion and the factors related with the atmosphere. *Inductive method.* This technique is based on the spatial association between the events\' happenings and the factors related to the atmosphere. Every happening of an event is first plotted in the topographical space. **[4. Prescriptive Analytics ]** The prescriptive analytics model is the state-of-the-art branch of the data world. Several are already disputing whether this is the single way to go in the future analytical world. Prescriptive analytics has not caught fire in the trade world. It will convert more widely in diverse industries. Companies will understand the profits of having a model that will propose answers to future questions. This is the most advanced constituent of this comparatively new technology. ***[Process Involved in Prescriptive Analytics]*** *Data:* We need data to be collected and a repository to access essential data. *Analyze:* We need to analyze the data we collected and the Data Model to assess and query the data collected. *Provides Prediction using Algorithms:* In this step, Prediction is provided so that business users can define and track the following steps in their business analytics before they happen with mathematical algorithms and equation processes. *Intelligent Decisions:* We need to decide how we analyze the data. Analytics can allow managers to make better and wiser decisions. The difference between predictive and prescriptive is that predictive does not generate reports; predictive only provides predictions to business users indicating what will happen; on the other hand, prescriptive has the prediction and the generated reports indicating why it happened. Business users use this approach for a more in-depth analysis of the business. The only downfall is that it is tedious work and needs more time to implement and build/create this **Unit 6: Data Collection & Mistakes to Avoid** **What Can Big Data Achieve?** The importance of using big data is [considering what will enable the company to achieve.] Although big data is often related to marketing and e-commerce, it would be misleading to assume that data is restricted to those small sectors. Businesses across industries will benefit from data in various ways with proper analysis to allow a business to stand out from its competition. These techniques can also be used to identify potential errors before they occur or to prevent fraud, especially in the financial sector. - E-commerce firms like Amazon and Walmart use data to their advantage. By carefully evaluating the browsing behavior of their users, these companies have a better understanding of their shoppers, their habits, and their needs. This information is then used to ensure that the business maximizes its profits. The data also allows the company to show items that individual customers are more likely to order and buy. [What to Understand First?] A long-term plan and goal are essential before a company collects large amounts of data. Storing data can be expensive, and review of information can be even more expensive. Defining the company\'s data objectives ahead of time is also essential. Ask questions like: * What do you want to do with the information?* \* Do you intend to learn more about your clients, or are you taking precautions to prevent fraudulent behavior?* *If you have decided the intent of your data, these six steps will allow you to use the data to meet your company\'s needs.* *1. Data Collection* You should know exactly how your business intends to collect consumer data. The possibility is almost infinite. Some businesses will rely on data from social media networks like Facebook and Twitter. Data can also be obtained from Radio Frequency Identification (RFID) chip readings and Global Positioning System (GPS) tests. Another good idea is to gather transaction information. If you sell goods and services online, collecting this information from your transactions will prove very helpful, offering a wealth of insights into consumer behavior and preferences. *2. Evaluate Data Relevance and Accuracy* Next, you will need to determine the actual value of your data. How has the information been collected? Information compiled haphazardly may need to be more accurate, complete with flaws, and simply worthless. Therefore, you must analyze the factual accuracy of your information regularly, as this is a critical factor in making informed decisions. Before investing an immense amount of money to analyze the data in the first place, ensure that the data will provide useful insights. If not, collect the data more accurately before moving forward. *3. Gain Better Insights* Most modern companies already store a sufficient amount of data regularly. To gain better insights, several issues need to be addressed first: How much do you know about your company\'s data and collection processes? How frequently is the information changed, and where is it stored? Is the information being processed daily? Are there any protection or confidentiality issues about the information stored? Getting answers to these questions will give you an advantage and ensure that you better understand your company\'s current practices -- including compliance with local and international law. Also, be sure to consult the team or the individual responsible for analyzing your company\'s data, as their insights can be valuable in improving data practices. *4. In-House Capabilities* Believe it or not, processing and analyzing big data can be vast and costly. Great skill and expertise are required to access the information and use the associated tools effectively. Many organizations have yet to be able to appoint data analysts for their teams, putting them a step behind their rivals. At the same time, many will need help to maintain and manage their in-house talents. While it may seem a good idea to devote resources solely to data analytics programs, this may be costly. In order to get the most out of your results, it is essential to combine data analytics and IT technology closely. Avoid compartmentalizing! Alternatively, aim to spread your capital between the two sectors. Investments in IT infrastructure should be compatible with data mining techniques and vice versa. Studies by McKinsey & Company have shown that 40% of businesses have only been able to raise their income by parallel and organized investment in both sectors. *5. Data Visualization* When you have learned to collect accurate data, it is time to get insights from the details. Visualization is a crucial component of this process because it allows you to portray knowledge in a more accessible way. Your team will likely have a few members who could be more comfortable with numbers. In order to ensure that your data is used efficiently, you need to display the information in a visually attractive manner. Using tools such as Google Charts or Microsoft Excel would make translating the data into graphs and maps possible. This is highly recommended. Charts are easily understood and help ensure every team member is active and engaged. *6. Turning Insights into Action* Access to big data and being able to analyze that data will only do you good if you can translate that effort into successful action. Having the resources required to evaluate the data is only one step in the right direction. If the ultimate objective is to improve protection or boost income, you must find out how to turn the information gained into successful action. The CEO should be able to build a marketing strategy focused on observations. If the CEO and other senior executives are not on board, a buy-in is completely necessary. In addition, customer feedback at all levels should be systematically collected, analyzed, and integrated into every decision-making phase. Whether it is setting out a new advertising strategy or strategizing elsewhere, it is important to ensure that insights are added to the equation. Learn how to use this information correctly, and your business will benefit greatly. **Starter Software to gather Data** Since you already know the steps in collecting data, you can explore different software that could be very useful in processing the data needed to make good decisions. There are also plenty of free, open source products accessible that you can get started by right now, for no money down. In addition, there is a wealth of commercial predictive analytic software available in the marketplace today. If you don't see the kind of software that you are in search of here, odds are a rapid online search will be capable to point you in the correct direction while the following list would give you a good place to start. *Apache Mahout:* Apache Mahout is a [free scalable algorithm connected to machine learning primarily focused on clustering classification and collaborative filtering.] The Apache Software Foundation created it. Regarding standard math processes, counting things like statistics plus linear algebra also allows users to access communal Java libraries for help. For those needing to use them instead, it offers access to primeval Java assortments. The algorithms that it uses for filtering, organization, and clustering have all been executed based on the Apache Hadoop map paradigm. As of September 2016, this is still a comparatively new product, which means there are still gaps in whatever it can and cannot offer, whereas it has a wide variety of algorithms now available. Apache Mahout does not need Apache Hadoop to function correctly. Apache Mahout can be found online for free at mahout.apache.org. *GNU Octave:* GNU Octave is a program language software for high-level use. It was initially intended to help with complex numerical calculations of linear and nonlinear diversity and other similar arithmetical-based experiments. It primarily uses a batch-oriented language that is mainly compatible with MATLAB. It was shaped as part of the GNU Project. It is free software by default and is founded on the GNU General Public License. The fact that it is usually compatible with MATLAB is notable since Octave is the leading free competitor to MATLAB. Using the command line to force Octave to try and comprehensive the file name, function otherwise variable in query Notable GNU Octave features comprise the aptitude to type a TAB character. It does this using the text previous to where the cursor is located as a standard for the requirements to be completed. You can also shape your data structures to a restricted degree and look into your command history. Furthermore, GNU Octave uses logical short-circuit Boolean kind operators, which are then assessed in the way that a short circuit will be. It also offers restricted support for exception handling based on the impression of unwinding and protecting. GNU Octave is accessible online at GNU.org/Software/Octave. \* KNIME:* KNIME is a platform emphasizing integration, reporting, and data analytics, also recognized as the Konstanz Information Miner. Also, it uses different constituents of various other jobs, including data mining plus machine learning, over a unique pipeline founded on modular data. It also claims a graphical user interface, making the nodes used in data preprocessing much less complex. Though it has grown in the past decade, KNIME is mainly used in medicine. It can also be helpful when studying financial statistics, business intelligence, and customer data. KNIME is founded on the Java platform using Eclipse. Moreover, it also utilizes an addition to add plugins for a better array of functionality than what the base program could offer. Regarding data visualization, transformation, analysis, database management, and integration, the free version comprises more than 200 modules of choices. You can even use KNIME reports to generate a wide diversity of clear and edifying charts spontaneously. You can also use the additional extension, which is available for free, to design reports. KINE can be found online at KNIME.org. *Open NM:* OPEN NM, for short, is a C++ software library that is authorized below the GNU Lesser General Public Certificate, which means it is accessible to those who use it in worthy faith. It is a software package covering a general resolve artificial intelligence system. This software is helpful because it combines several layers of processing units in a nonlinear style to increase supervised learning. Furthermore, its exclusive architecture allows it to work by neural networks containing universal approximation possessions. Furthermore, it permits programming through multiprocessing means, such as OpenMP, for those interested in growing computational performance. Open NM also uses data mining algorithms that are bundled as a function. Using the including interface for application programming, these can ultimately be embedded in other tools and software of your selection. This makes it much easier than it is to find novel ways to integrate predictive study into even more jobs when used correctly. Please note that it does not offer a customary graphical interface; however, some visualization riggings support it. *Orange Software:* Orange Software is data mining software in the prevalent Python programming language. It has a front with a visual constituent, making added visualization types much calmer than they are with program plus data analysis, unlike some free accessible options. It also doubles as a Python library for those interested in that kind of thing. The University of Ljubljana and the Bioinformatics Laboratory of the Faculty of Computer and Information Sciences shaped this program. The individual components available in Orange Software can be used for almost everything from basic data visualization toward selecting subsets to empirical assessment, predictive modeling, preprocessing, and algorithm generation. They are individually mentioned as widgets. Furthermore, visual programming can be effectively implemented through an interface. This allows users to directly generate workflows quickly and easily by linking together a predefined collection of widgets. As they are free to alter widgets and manipulate data through Python, this doesn't stymie forward-thinking users. The latest form of Orange Software includes numerous core components shaped in C++ with wrappers that were then shaped using Python. The installation defaults for the software comprise regression, visualization, classification, widget sets for supervised plus unsupervised data analysis, data collection, and other numerous algorithms plus preprocessing tools. Orange Software is obtainable online, for free, at Orange.biolab.si. *R:* A programming language recognized simply as R is an excellent environment for software connected to statistical computing and visualization options. It is commonly used by data miners plus statisticians working on forming statistical programs, otherwise data analysis, and was created by the R Foundation for Statistical Computing. When using numerous methods, be they graphical or statistical, R and the libraries it is connected to are helpful. These comprise linear modeling, nonlinear modeling, clustering, classification time series analysis, and statistical tests. It can create interactive graphics plus publication-quality graphs without additional tweaking. Additional strengths include its aptitude to generate static graphics. *Scikit-Learn,* a powerful machine-learning library written in Python, offers a diverse range of regression, clustering, and classification algorithms. These include some less common algorithms in the open source space, such as DBSCAN, k-means, random forests, gradient boosting, and support vector machines. It seamlessly integrates with the SciPy and NumPy Python libraries, providing access to scientific and numerical libraries. Scikit-Learn, Initially shaped by David Cournapeau as part of the Google Summer of Code, has seen its code base rewritten by other developers. Since 2015, it has been under active development, making it a promising choice for those seeking a robust solution in the open-source space. Despite a period of less frequent updates, Scikit-Learn is now back in active development, ensuring its continued relevance and availability for free at Scikit-Learn.org. *WEKA:* WEKA, for short is a software set that was written in Java through the University of Waikato in New Zealand. WEKA has manifold user interfaces that provide access toward a wide diversity of options unlike several open source analysis platforms. The preprocess panel permits users to import data from databases plus filtering data over the use of predetermined algorithms. It also makes it likely to delete attributes otherwise instances founded on predefined criteria. Additionally, the classification panel determines the correctness of the models that are shaped through the process and makes it easy for users to use either regression or classification algorithms based on diverse data sets. The associate panel is valuable for users who are fascinated by gaining access to numerous algorithms that are useful while it comes to determining the numerous relationships that certain data points have through one another. The cluster panel is valuable for those looking for more options. It provides an \'intensification algorithm \', a term used to describe the process of enhancing the accuracy and efficiency of clustering, particularly if you are interested in finding the normal distribution combination and optimizing clustering effectively. The panel also includes the k-means algorithm. Lastly, the select attributes panel would allow users to access even additional algorithms; this time, they will be connected to various analytical attributes found in an assumed dataset. The visualization panel is valuable for those interested in making scatter plot matrixes, making it easy for scatter plots to be analyzed based on the information they provide. **Mistakes to Avoid** Much like what you have done in the activity above, analytics needs careful research to eliminate errors. Here are just some tips on how to avoid mistakes in using analytics: *Choosing a data warehouse based on what you require at the moment* When selecting the correct data warehouse for you, it could be easy not to think about the long tenure. You are going to be cursing the limits you accidentally enforced on yourself as making longstanding data decisions with merely the short term in mind is an easy method to ensure that a few years down the line. This means that if you want the future in your approval, you will want to look five years down the road for wherever you want your firm to be at that time. Remember to focus as much on the business strategy and the technical features you would be using. *Considering metadata as a reflection* Failing to make the correct Metadata selections early on can have long-reaching plus disastrous implications in the long term, while it seems like you can quickly go back and tinker with them at a later date. It would help if you considered it the leading integrator in making different kinds of data models play nicely with one another Instead of thinking of it as an afterthought. This means considering longstanding data requirements while making choices and documenting everything. You should start by confirming that every column and table you generate has its own descriptions and key phrases to do this properly. By picking a tagging and naming convention early and sticking with it through your time in the data warehouse, it is astonishing how many problems you can rapidly and easily solve in the long term. *Need to overestimate the usefulness of ad hoc querying*. \* * For lack of a better word, simple, in reality it could easily expand significantly, causing bandwidth costs toward skyrocket and productivity to reduction overall due to the additional strain while generating a simple report appears as though it should be. By simply relying on the metadata layer to make the reports in its place this issue could be avoided. Without directly affecting the secure plus sanctified nature, which refers to the preserved integrity and reliability, of the original data this will make things go much more effortlessly. This can also make it easier for less familiar users to get wherever they need to and interrelate with the system appropriately. It can make it easier for it to gain a wider quantity of buy in from main individuals. *Letting for supersede function* You may be desirous of picking something visually appealing without considering the long-term implications of that decision when deciding on a reporting layer for the data warehouse that you will use positively for years to come. Specifically, it is significant that you do not select a visual presentation style. It causes the system to run more sluggish than it otherwise would. Take a minute to ponder how often this system will be used, plus how many people will understand why even a five-second difference in load time could be an enormous time waster. With so many multipliers, it will take only a short time for five seconds to become hours, if not days. Furthermore, Speedier systems will make it more likely for various group members to actively partake in the system because its ease of use will be greater, if not prettier. In addition, it will save you and your team time. Additionally, the faster data can be generated, the more probable it is to remain faithful to the source. It will ensure you have a higher general quality of data to work through than you else might. *Focusing on cleaning data after it has already been stored* You should confirm that any data you put into it will be as precise and clean as possible once your data warehouse is up and running. Team members are more likely to fill out pertinent information regarding themes the business cares about and notice errors immediately as opposed to later. There are multiple reasons that this is the right choice. Furthermore, it is out of mind as well, which is just as accurate with data as it is with anything else, the adage that once somewhat is out of sight. It is much easier to overlook it and move on to another job. Even if team members have the finest intentions regarding data, it has been filed away afterward. If too much unfiltered data gets over, then the data as an entire is much more likely to find differences when compared with what happened. The occasional piece of unfiltered data slipping through will not affect stuff only a little. Recall that everything is correct with the current scheme. That does not mean you want to add additional issues to the pile. Error-ridden data comprises some active observing system in your data management system. Quality control can furthermore be governed through common usage. The data coherence plan that your business now has in place. *Allowing data warehouse tasks to be purely the concern of those in IT* It is important that they do not control the total of the project when it comes to implementing your own data warehouse, while those in the IT department are probable going to be a great source of lawful knowledge. Because a crucial feature for your group is especially hard to use, they should be consulted while it comes to key features to confirm that the system is not lifeless in the water inside six months. *Treating only certain types of data as relevant* It can be easy to envision Big Data as just that, big, plus uniform, and capable of fitting easily into a one-size-fits-all bin While you are first starting. Realistically, big data comes in three primary groups, and there is a diversity of shapes and sizes. Each of these crowds is especially pertinent to a particular segment of business. You will need to distinguish how you plan to use them if you hope to create an effective management system. Data could be unstructured, likely audio images or video, and simple text can be unstructured. Instead, data can be structured, comprising mathematical models, actuarial modes, risk models, financial models, sensor data, and machine data. Lastly, in between is the semi-structured data comprising similar software modules, earnings report spreadsheets, and emails. *Focusing on data quantity over quality* With big data on everyone's minds and lips, it can be easy to get so focused on obtaining as much information as possible that the quantity of data matters more than the quality of the data you collect. However, this is a big mistake, as data of low quality, even if cleaned, can still skew analytics in unwanted ways. This means that not only is it essential to know how to process Big Data effectively and to seek out the most valuable and relevant information possible at every opportunity, but it is also essential to understand how to improve the quality of data that is being collected in the first place. When it comes to unstructured data, it is essential to note that if you are interested in improving the quality of the data that is being collected, this should be done by improving the libraries that are used for language correction prior to the data being uploaded to the warehouse. It is best to have a human hand in that process if translation is required, as the finer points of translation are still lost in most cases on automatic translation programs. When it comes to semi-structured data with either numeric or text values, it is essential to run it through the same process of correction that you would use for traditional text files. Additionally, you will need to plan for lots of user input to ensure the data comes out the other side in its most valuable and accurate state. Structured data should generally be in a helpful state already and should not require further effort. *Failing to think about granularity* Once again, because of its lumbering nature, it is easy for those just getting started with analytics to create a data warehouse without considering the level of granularity that will ultimately be required from the data in question. While you cannot determine precisely how granular you will need to go up-front, you will want to know that it will eventually be required and plan for it in the construction phase. You need to do so to be able to process the relevant metrics you are interested in and foggy on their relevant hierarchies and related metrics. The situation can grow out of control extremely quickly, especially when working with semi-structured or text-based data. As these are the two types of data you will come into contact with most, it is worth considering early. \* Contextualizing incorrectly* While it is essential to contextualize the data you collect, which means placing the data in a specific context or setting, it is also important to contextualize it correctly for later use. Not only will this make the data more beneficial in the long term when the original details have primarily faded, but it will also reduce the potential risk of inaccuracy, increasing the chance of skewed analytics further down the line. Especially when dealing with text from multiple businesses or disciplines, you must always plan to have a knowledgeable human on hand to ensure that this information makes it into the data warehouse in a manner that is useful and as accurate as possible. Finally, contextualizing is essential because, when tagged correctly, it can make it easier to pepper the database with additional interconnected topics.