Artificial Intelligence: Principles and Practice 2022 PDF
Document Details
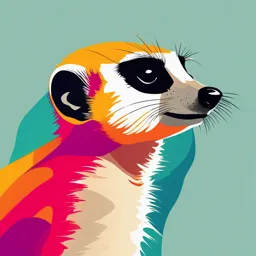
Uploaded by ExhilaratingDialogue
Menoufia University
2022
Dr. Sherif Eletriby
Tags
Summary
This textbook introduces the fundamental principles of artificial intelligence and applications including speech recognition, computer vision, and natural language processing. The book explores the historical context and various aspects.
Full Transcript
Menoufia University Faculty of Computers and Information Computer Science Department ___________________________________________ Artificial Intelligence: Principles and Practice _____________________________________________...
Menoufia University Faculty of Computers and Information Computer Science Department ___________________________________________ Artificial Intelligence: Principles and Practice _____________________________________________ Dr. Sherif Eletriby First Edition 2022 1 2 CHAPTER 1 INTRODUCTION 1.1 What is AI 1.2 Foundations of AI. 1.3 The Turing Test and Subfields of AI 1.4 History of AI. Artificial Intelligence (AI) is a field that has a long history but is still constantly and actively growing and changing. In this course, you’ll learn the basics of modern AI as well as some of the representative applications of AI. Along the way, we also hope to excite you about the numerous applications and huge possibilities in the field of AI, which continues to expand human capability beyond our imagination. The Industrial Revolution, which started in England around 1760, has replaced human muscle power with the machine. Artificial intelligence (AI) aims at replacing human intelligence with the machine. The work on artificial intelligence started in the early 1950s, and the term itself was coined in 1956. The academic theory of AI AI research tries to give machines the ability to reason, represent knowledge, plan, learn, communicate, perceive, move and manipulate objects. Current approaches to Artificial Intelligence include symbolic methods, such as knowledge based Artificial Intelligence, and sub-symbolic methods such as computational intelligence. 1.1 WHAT IS AI? There is no standard definition of exactly what artificial intelligence is. If you ask five computing professionals to define "AI", you are likely to get five different answers. The Webster's New World College Dictionary, Third Edition describes AI as "the capability of computers or programs to operate in ways to mimic human thought processes, such as reasoning and learning." This definition is an orthodox one, but the field of AI has been extended to cover a wider spectrum of subfields. 3 Definitions of artificial intelligence according to eight textbooks are shown in Figure 1.1. These definitions vary along two main dimensions. Roughly, the ones on top are concerned with thought processes and reasoning, whereas the ones on the bottom address behavior. The definitions on the left measure success in terms of fidelity to human performance, whereas the ones on the right measure against an ideal concept of intelligence, which we will call rationality. A system is rational if it does the "right thing," given what it knows. Figure 1.1 The parts of a nerve cell or neuron. Each neuron consists of a cell body, or soma, that contains a cell nucleus. Branching out from the cell body are a number of fibers called dendrites and a single long fiber called the axon. The axon stretches out for a long distance, much longer than the scale in this diagram indicates. Typically, an axon is 1 cm long (100 times the diameter of the cell body), but can reach up to 1 meter. A neuron makes connections with 10 to 100,000 other neurons at junctions called synapses. Signals are propagated from neuron to neuron by a complicated electrochemical reaction. The signals control brain activity in the short term and also enable long-term changes in the connectivity of neurons. These mechanisms are thought to form the basis for learning in the brain. Most information processing goes on in the cerebral cortex, the outer layer of the brain. The basic organizational unit appears to be a column of tissue about 0.5 mm in diameter, containing about 20,000 neurons and extending the full depth of the cortex (about 4 mm in humans). 4 The Field study of AI: Three Major Fields of Artificial Intelligence and Their Industrial Applications: Artificial intelligence is a new technical discipline that researches and develops theories, methods, technologies, and application systems for simulating the extension and expansion of human intelligence. The goal of artificial intelligence research is to let machines perform some complex tasks that require intelligent humans to complete. That is, we hope that the machine can replace us to solve some complicated tasks, not just repetitive mechanical activity but some that require human wisdom to participate in it. In this article, I will explain three major directions of artificial intelligence technology, that are speech recognition, computer vision, and natural language processing. Speech Recognition Speech recognition makes the computer listens, including Siri on the iPhone that we can access in daily life; and in Google voice input you can say a sentence, which turns into the text; speak to Google map says where I’m going, it can automatically generate navigation for you. These are some applications of speech recognition. Speech recognition can be divided into three aspects: Speech synthesis, including online and offline speech synthesis; Speech recognition, including speech dictation and other aspects; Semantic understanding is to use neural networks to extract the meaning of speech, including voice evaluation and some features of some of our commonly used machine translation. 5 Computer vision Computer vision makes the computer sees. We hope that computers can replace some of the functions of the human eye. For example, there is a very useful document analysis technology, called OCR. We can let the computer scan the document and read it. For example, we can get an invoice so that the computer can immediately scan it and then extract the information about the amount, tax rate and other information we care about from the invoice. There are some researches on computer vision in the field of intelligent medical diagnosis. Although it is not yet commercially available, I believe that there will be broad application scenarios in the future. At the same time, in the military field, drones are replacing human observation and measuring the trajectory for missiles. Popular directions for computer vision are: Object recognition and detection. The computer can quickly detect what we commonly see from the photos. For example, if we take out a landscape photo of a tourist area, we can immediately identify plants, people, animals, or vehicles on it, so does the computer. https://en.wikipedia.org/wiki/Object_detection#/media/File:Detected-with-YOLO-- Schreibtisch-mit-Objekten.jpg Object Movement Tracking. We have captured an image of an object on a certain frame. In the subsequent videos, we can continuously track the changes and conditions of this object. It is not an easy task. It is difficult to accurately identify an object because it will be subject to sunlight and light in the constant changes. 6 The other is the function of the computer to see pictures and speak. For example, given a picture, the computer can identify what it contains in the picture, and then tell some pre-made content. Now many exhibition halls have already used this technology. It can precast explanatory words and guide words. Visitors only need to use a mobile phone or other equipment to scan the exhibits or some designated locations in the exhibition area to hear the relevant guide words. Natural language processing From now on, our computer can listen to what we say and see what we see. But we want more. We prefer to interact with the computer, using natural language for communication, this is the aim of natural language processing. Natural language processing is now used in machine translation, information retrieval, and dialogue systems. Computer translation: It mainly includes machine simultaneous translation. Information Retrieval: For example, when I tell the computer what I am looking for, then it can search related things for me. Intelligent customer service: We interact with the computer through voice and let it answer our questions. Natural language processing is not that simple. It is relatively difficult. We have to solve the following problems. The first one is the ambiguity in the language, sometimes a sentence can be understood in two or more possible senses or ways. For example, “I went to the bank.” The bank could be a place where the money is kept, or it could be the edge of a river. 7 Photo by Museums Victoria on Unsplash; Photo by Fausto Vilca on Unsplash In addition, we need to solve the robustness of the language. We often say some typos in the daily speech, or say fewer words, or say more words than the original meaning, which will affect the language’s robustness. Also, there may be other nicknames which may refer to the same person. The other is knowledge dependence. We usually use a knowledge graph to solve the problem of knowledge dependence. Let’s say Da Yali (means big pear in Chinese), it is a fruit, and it is also the name of a very famous roast duck restaurant in Beijing. And like Seven Days, it can represent time, it can also represent a hotel’s name. These are all dependent on some background knowledge, we need to use a knowledge base or a knowledge map to solve this problem. The other is the context. Based on the context of the dialogue, we can accurately judge what is to be said. For example, “I want to eat Da Yali”, “Da Yali” may represent a fruit. “Let’s go to Da Yali”, then “Da Yali” represents a restaurant. In the context of different dialogues, different expressions show some different meanings. Summary 8 Before we moved to the industrial applications of artificial intelligence, let’s summarize what we have learned in the above part. We learned speech recognition. The computer can hear us and make some responses, such as translating our words into text. Then we studied computer vision, it let the computer sees. The computer can identify some objects in the image by looking at the image, and it can also track the changes of an object in continuous images. These are some of the hot topics that computers solve. Then, in the end, we learned about natural language processing, that is, not only do computers need to hear what we say, they also understand what I say, and then they can give us some feedback. Industry Applications of Artificial Intelligence Let's introduce the related applications of artificial intelligence from the fields of civil security, transportation, public security, self-driving cars, intelligent robots, and telecommunications industries. Civil Security First of all, let us tell you about the field of civil security. With the popularization of smart homes, artificial intelligence has gradually played a role in civil security. For example, a home security camera can learn from video and identify which belongs to our family through daily shooting. When our family enters the video surveillance range, it will not trigger the alarm. However, when an outsider enters illegally, it will immediately alert us, such as sending text messages to us or making loud alarming sounds. These are some simple applications of smart security cameras. 9 Photo by Sebastian Scholz (Nuki) on Unsplash Transportation In the field of transportation, we can analyze the video of traffic through artificial intelligence, and make use of the data to make decisions. We can analyze whether the current road is congested and how the situation is and then use artificial intelligence to make the decision automatically. For example, let AI adjust the time in the traffic signal to direct traffic, or implement large-scale traffic linkage scheduling to improve the operating efficiency of the entire city. Photo by chuttersnap on Unsplash 10 Public Security In the field of public security, artificial intelligence also has particularly obvious applications using image recognition and face recognition. For example, we found clues of suspects in massive video information; Or given certain characteristics, the AI extracted information from people or items that match the characteristics from the video, which is fast and accurate. Photo by chuttersnap on Unsplash Autopilot There are also many applications of artificial intelligence in the field of autonomous driving. Automated driving actually requires a lot of technology, including the perception of the environment. We perceive the surrounding environment through a series of devices such as relevant video cameras, laser rangefinders, microsensors, vehicle radars, etc., and then integrate this information together through artificial intelligence to determine how the surrounding environment is. After collecting all the information that is required for behavioral decision- making, based on the results of environmental perception, it is necessary to use artificial 11 intelligence to decide what the car should do next, whether to apply the brakes or the accelerator. The last one is the action control module. After the AI makes a decision, it has to pass that decision to the motion control module to control the car, such as actually pressing the brake down or actually pressing the accelerator down. Smart Robot Intelligent robots have great application potential in the service industry, education industry, and the medical industry. For example, many banks now have an automated question answering robots, which can guide the person who comes to the bank to conduct business, or queue up for numbers, or simply introduce some businesses, which has improved the efficiency of the bank and is also convenient for the customer, the majority of the people go to the bank to conduct business. Photo by Austin Distel on Unsplash 12 Application of Artificial Intelligence in the Telecommunications Industry What are the applications of artificial intelligence in the telecommunications industry? The telecommunications industry is also welcoming the arrival of the era of artificial intelligence. Many mobile companies have seized the opportunities of the era and developed and built a network platform for the core capabilities of artificial intelligence. This artificial intelligence network platform has big data analysis and machine learning functions. It automatically detects the condition of the mobile network, automatically troubleshoots, and performs traffic classification, anomaly detection, and prediction. At the same time, you can optimize the utilization of resources and perform related network optimization to enhance the intelligence of the mobile network and enhance the user experience through optimization. China Mobile also independently developed a smartphone customer service question-answering robot that uses artificial intelligence technologies such as machine learning, deep learning, and natural language processing to automate business processes, answer user questions more intelligently, and solve business consulting, business handling, and traffic Inquiries and other aspects of the problem, such as customer care, network coverage, billing, and other related services. In addition, in terms of subscribers, artificial intelligence and machine learning will also help telecom operators solve functions such as describing and analyzing user information, improving conversion rates, analyzing content usage trends, and network activities. With the help of artificial intelligence and data analysis, operators can also identify target customers at the right time and provide customers with various services. Mobile companies also focus on the five major areas: network, security, management, customer 13 service, and marketing, using artificial intelligence technology to expand the scale of applications. Photo by 7shifts on Unsplash In the network field, large-scale applications of artificial intelligence technology have been achieved in network self-service robots, intelligent VoLTE voice quality measurement, smart home broadband installation. In the security field, anti-fraud systems have been able to intercept scam calls, and the monthly interception volume is more than 14 million in China. In the management field, audit points for contracts and bills have also been implemented. Intelligent machines have replaced manual audits, which can save hundreds of millions of costs each year. In the field of customer service, the intelligent customer service question answering robot can answer more than 210 million times each month at present. Combining their own business advantages in vertical industries, telecommunications companies are actively deploying AI applications in various industries, including intelligent education, 14 intelligent medical treatment, intelligent transportation, intelligent industrial intelligent agriculture and other aspects. Summary Finally, We summarize the learning content of this part. In this part, we have learned and understood artificial intelligence security cameras in the field of civil security. Through video recognition, artificial intelligence in the transportation field can provide a basis for decision- making for our transportation with machine learning, and even make decisions automatically. In the field of public security, the identification of images can provide a quick basis for the detection of public security cases and the location of suspects. Self-driving cars not only use artificial intelligence technology to allow the car to perceive its surroundings, but also allow the car to make decisions about what to do next, and to manipulate the vehicle to achieve the purpose of self-driving. Intelligent robots are also very common in our lives. They usually play the role of customer service to help us. Finally, we briefly introduced the application and development of artificial intelligence in the telecommunications industry and mainly introduced the artificial intelligence network platform used by mobile companies and artificial intelligence customer service robots. Foundations of AI Philosophy (384 B.C.) Mathematics (George Boole 1815-1864) Economics (Adam Smith (1723-1790) published An Inquiry into the Nature and Causes of the Wealth of Nations) Neuroscience (Paul Broca's (1824-1880) study of aphasia (speech deficit) in brain-damaged patients) Psychology (Hermann von Helmholtz (1821-1894) applied the scientific method to the study of human vision, and his Handbook of Physiological Optics) 15 Computer engineering : (For artificial intelligence to succeed, we need two things: intelligence and an artifact. The computer has been the artifact of choice) Control theory and cybernetics: a water clock 250 B.C. and thermostat. Wiener's book Cybernetics (1948) be-came a bestseller and awoke the public to the possibility of artificially intelligent machines Linguistics: Noam Chomsky, wrote the book Syntactic Structures. He pointed out that the behaviorist theory did not address the notion of creativity in language-it did not explain how a child could understand and make up sentences that he or she had never heard before. 1.xx History of A The gestation of AI Warren McCulloch and Walter Pins (1943): build a neuron model to represent prepositional logic. SNARC: first neural network computer in 1950 by Marvin Minsky and Dean Edmonds at Harvard. The birth of AI McCarthy called for two months workshop at Dartmouth (1956). McCarthy invite Minsky, Claude Shannon, and Nathaniel Rochester. There were 10 attendees in all, including Trenchard More from Princeton, Arthur Samuel from IBM, and Ray Solomonoff and Oliver Selfridge from MIT. Newell and Simon stole the show by reasoning program, the Logic Theorist (LT), about which Simon claimed, "We have invented a computer program capable of thinking non-numerically, and thereby solved the venerable mind—body problem. Great expectations (1952-1969) Newell and Simon developed the GPS to imitate human. Arthur Samuel wrote a series of programs for checkers. 16 Minsky supervised a series of students who chose limited problems that appeared to require intelligence to solve which known by Microworlds McCarthy defined the high-level language Lisp A dose of reality (1966-1973) U.S. National Research Council funded an attempt to speed up the translation of Russian scientific papers in 1957. This project failed. Herbert Simon (1957) said: “It is not my aim to surprise or shock you but the simplest way I can summarize is to say that there are now in the world machines that think, that learn and that create. Moreover their ability to do these things is going to increase rapidly until—in a visible future—the range of problems they can handle will be coextensive with the range to which the human mind has been applied”. Knowledge-based systems: The key to power? (1969-1979) DENDRAL by Feigenbaum 1969 at Stanford: program to solve the problem of inferring molecular structure from the information provided by a mass spectrometer AI becomes an industry (1980—present) RI expert system: The program helped configure orders for new computer systems. And saved a company 40 million a year. The return of neural networks (1986—present) back-propagation learning algorithm first found in 1969 by Bryson AI adopts the scientific method (1987–present) The emergence of intelligent agents (1995—present) Allen Newell, John Laird. and Paul Rosenbloom on SOAR (Newell, 1990; Laird et al., 1987) is the best-known example of a complete agent architecture The availability of very large data sets (2001—present) 17 18 CHAPTER 2 INTELLIGENT AGENTS 2.1 Agents and Environments 2.1 Structure of Agents 2.1 Environment types 2.1 Agent types 2.1 Examples: (Vacuum world, …… ) AGENTS: An agent is anything that can be viewed as perceiving its environment through sensors and acting upon that environment through actuators Figure 2.1 Agents interact with environments through sensors and actuators. 19 Vacuum-cleaner world Figure 2.2 A vacuum-cleaner world with just two locations. Each location can be clean or dirty, and the agent can move left or right and can clean the square that it occupies. Different versions of the vacuum world allow for different rules about what the agent can perceive, whether its actions always succeed, and so on. 20 Problem Definition -Example vacuum world A simple agent function is: If current square is dirty then suck, else move to the other square Percepts: location and contents, e.g., [A, Dirty] Actions: Left, Right, Suck, No Op. Agent’s function →look-up table For many agents this is a very large table Figure 2.3 Partial tabulation of a simple agent function for the vacuum-cleanerworld shown in Figure xx. The agent cleans the current square if it is dirty, otherwise it moves to the other square. Note that the table is of unbounded size unless there is a restriction on the length of possible percept sequences. 21 Understanding PEAS in Artificial Intelligence We know that there are different types of agents in AI. PEAS System is used to categorize similar agents together. The PEAS system delivers the performance measure with respect to the environment, actuators, and sensors of the respective agent. Most of the highest performing agents are Rational Agents. Rational Agent: The rational agent considers all possibilities and chooses to perform a highly efficient action. For example, it chooses the shortest path with low cost for high efficiency. PEAS stands for a Performance measure, Environment, Actuator, Sensor. 1. Performance Measure: Performance measure is the unit to define the success of an agent. Performance varies with agents based on their different precepts. 2. Environment: Environment is the surrounding of an agent at every instant. It keeps changing with time if the agent is set in motion. There are 5 major types of environments: Fully Observable & Partially Observable Episodic & Sequential Static & Dynamic Discrete & Continuous Deterministic & Stochastic 3. Actuator: An actuator is a part of the agent that delivers the output of action to the environment. 4. Sensor: Sensors are the receptive parts of an agent that takes in the input for the agent. Performance Agent Environment Actuator Sensor Measure Hospital Patient’s health, Prescription, Symptoms, Hospital, Doctors, Management Admission process, Diagnosis, Scan Patient’s Patients System Payment report response The comfortable Steering wheel, Automated Car Roads, Traffic, Camera, GPS, trip, Safety, Accelerator, Drive Vehicles Odometer Maximum Distance Brake, Mirror 22 Performance Agent Environment Actuator Sensor Measure Maximize scores, Classroom, Desk, Subject Smart displays, Eyes, Ears, Improvement is Chair, Board, Staff, Tutoring Corrections Notebooks students Students Part-picking Percentage of parts Conveyor belt with Jointed arms and Camera, joint robot in correct bins parts; bins hand angle sensors Display Satellite image Correct image Downlink from Colour pixel categorization of analysis system categorization orbiting satellite arrays scene Environment types Fully observable (vs. partially observable) Deterministic (vs. stochastic) Episodic (vs. sequential) Static (vs. dynamic) Discrete (vs. continuous) Single agent (vs. multiagent): Fully observable (vs. partially observable) Is everything an agent requires to choose its actions available to it via its sensors? Perfect or Full information. If so, the environment is fully accessible If not, parts of the environment are inaccessible Agent must make informed guesses about world. In decision theory: perfect information vs. imperfect information. 23 Cross Word Poker Backgammon Taxi driver Part picking robot Image analysis Full Partially Partially Partially Full Full y y y Deterministic (vs. stochastic) Does the change in world state Depend only on current state and agent’s action? Non-deterministic environments Have aspects beyond the control of the agent Utility functions have to guess at changes in world Cross Word Poker Backgammon Taxi driver Part picking robot Image analysis Determin Stocha Stocha Stocha Stocha Determin istic stic stic stic stic istic ………. Agents in Artificial Intelligence Artificial intelligence is defined as the study of rational agents. A rational agent could be anything that makes decisions, as a person, firm, machine, or software. It carries out an action with the best outcome after considering past and current percepts(agent’s perceptual inputs at a given instance). An AI system is composed of an agent and its environment. The agents act in their environment. The environment may contain other agents. 24 An agent is anything that can be viewed as : perceiving its environment through sensors and acting upon that environment through actuators Note: Every agent can perceive its own actions (but not always the effects) To understand the structure of Intelligent Agents, we should be familiar with Architecture and Agent programs. Architecture is the machinery that the agent executes on. It is a device with sensors and actuators, for example, a robotic car, a camera, a PC. Agent program is an implementation of an agent function. An agent function is a map from the percept sequence(history of all that an agent has perceived to date) to an action. Agent = Architecture + Agent Program Examples of Agent: A software agent has Keystrokes, file contents, received network packages which act as sensors and displays on the screen, files, sent network packets acting as actuators. A Human-agent has eyes, ears, and other organs which act as sensors, and hands, legs, mouth, and other body parts acting as actuators. A Robotic agent has Cameras and infrared range finders which act as sensors and various motors acting as actuators. 25 Types of Agents Agents can be grouped into five classes based on their degree of perceived intelligence and capability : Simple Reflex Agents Model-Based Reflex Agents Goal-Based Agents Utility-Based Agents Learning Agent Simple reflex agents Simple reflex agents ignore the rest of the percept history and act only on the basis of the current percept. Percept history is the history of all that an agent has perceived to date. The agent function is based on the condition-action rule. A condition-action rule is a rule that maps a state i.e, condition to an action. If the condition is true, then the action is taken, else not. This agent function only succeeds when the environment is fully observable. For simple reflex agents operating in partially observable environments, infinite loops are often unavoidable. It may be possible to escape from infinite loops if the agent can randomize its actions. Problems with Simple reflex agents are : Very limited intelligence. No knowledge of non-perceptual parts of the state. Usually too big to generate and store. If there occurs any change in the environment, then the collection of rules need to be updated. 26 Model-based reflex agents It works by finding a rule whose condition matches the current situation. A model-based agent can handle partially observable environments by the use of a model about the world. The agent has to keep track of the internal state which is adjusted by each percept and that depends on the percept history. The current state is stored inside the agent which maintains some kind of structure describing the part of the world which cannot be seen. Updating the state requires information about : how the world evolves independently from the agent, and how the agent’s actions affect the world. Goal-based agents These kinds of agents take decisions based on how far they are currently from their goal(description of desirable situations). Their every action is intended to reduce its distance from the goal. This allows the agent a way to choose among multiple possibilities, selecting the one which reaches a goal state. The knowledge that supports its decisions is represented explicitly and can be modified, which makes these agents more flexible. They usually require search and planning. The goal-based agent’s behavior can easily be changed. 27 Utility-based agents The agents which are developed having their end uses as building blocks are called utility-based agents. When there are multiple possible alternatives, then to decide which one is best, utility-based agents are used. They choose actions based on a preference (utility) for each state. Sometimes achieving the desired goal is not enough. We may look for a quicker, safer, cheaper trip to reach a destination. Agent happiness should be taken into consideration. Utility describes how “happy” the agent is. Because of the uncertainty in the world, a utility agent chooses the action that maximizes the expected utility. A utility function maps a state onto a real number which describes the associated degree of happiness. 28 Learning Agent : A learning agent in AI is the type of agent that can learn from its past experiences or it has learning capabilities. It starts to act with basic knowledge and then is able to act and adapt automatically through learning. A learning agent has mainly four conceptual components, which are: 1. Learning element: It is responsible for making improvements by learning from the environment 2. Critic: The learning element takes feedback from critics which describes how well the agent is doing with respect to a fixed performance standard. 3. Performance element: It is responsible for selecting external action 4. Problem Generator: This component is responsible for suggesting actions that will lead to new and informative experiences. Figure 2.16 Three ways to represent states and the transitions between them. (a) Atomic representation: a state (such as B or C) is a black box with no internal structure; (b) Factored representation: a state consists of a vector of attribute values; values can be Boolean, realvalued, or one of a fixed set of symbols. (c) Structured representation: a state includes objects, each of which may have attributes of its own as well as relationships to other objects. 29 REFERENCES 1. Artificial Intelligence: A Modern Approach, 3rd ed. by Stuart Russell and Peter Norvig 2. Artificial Intelligence", by Elaine Rich and Kevin Knight, (2006), McGraw Hill companies Inc., Chapter 1-22, page 1-613. 3. Artificial Intelligence: Structures and Strategies for Complex Problem Solving", by George F. Luger, (2002), Addison-Wesley 30