ANCOVA and Factorial ANCOVA Assumptions and Procedure PDF
Document Details
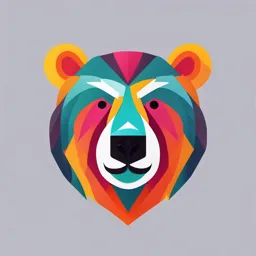
Uploaded by CourageousCyclops
Rizal Technological University
Tags
Summary
This document provides an overview of ANCOVA and factorial ANCOVA. It details assumptions and procedures. The document covers one and two-way ANOVA with continuous and categorical variables. The text also discusses how to test for assumptions using SPSS.
Full Transcript
ANCOVA and Factorial (Two-way) ANCOVA Assumptions and Procedure ONE WAY ANCOVA DEFINITION ? The one-way ANCOVA (analysis of covariance) can be thought of as an extension of the one-way ANOVA to incorporate a covariate. Like the one-way ANOVA, the one- way ANCOVA is used to...
ANCOVA and Factorial (Two-way) ANCOVA Assumptions and Procedure ONE WAY ANCOVA DEFINITION ? The one-way ANCOVA (analysis of covariance) can be thought of as an extension of the one-way ANOVA to incorporate a covariate. Like the one-way ANOVA, the one- way ANCOVA is used to determine whether there are any significant differences between two or more independent (unrelated) groups on a dependent variable. However, whereas the ANOVA looks for differences in the group means, the ANCOVA looks for differences in adjusted means (i.e., adjusted for the covariate). ? As such, compared to the one-way ANOVA, the one-way ANCOVA has the additional benefit of allowing you to "statistically control" for a third variable (sometimes known as a "confounding variable"), which you believe will affect your results. This third variable that could be confounding your results is called the covariate and you include it in your one-way ANCOVA analysis. Assumption #1: ? Your dependent variable and covariate variable(s) should be measured on a continuous scale (i.e., they are measured at the interval or ratio level). ? Examples of variables that meet this criterion include revision time (measured in hours), intelligence (measured using IQ score), exam performance (measured from 0 to 100), weight (measured in kg), and so forth. As stated earlier, you can have categorical covariates (e.g., a categorical variables such as "gender", which has two categories: "males" and "females"), but the analysis is not usually referred to as an ANCOVA in this situation. Assumption #2: ? Your independent variable should consist of two or more categorical, independent groups. Example independent variables that meet this criterion include gender (e.g., two groups: male and female), ethnicity (e.g., three groups: Caucasian, African American and Hispanic), physical activity level (e.g., four groups: sedentary, low, moderate and high), profession (e.g., five groups: surgeon, doctor, nurse, dentist, therapist), and so forth. Assumption #3 ? You should have independence of observations, which means that there is no relationship between the observations in each group or between the groups themselves. For example, there must be different participants in each group with no participant being in more than one group. This is more of a study design issue than something you can test for, but it is an important assumption of a one-way ANCOVA. If your study fails this assumption, you will need to use another statistical test instead of a one-way ANCOVA (e.g., a repeated measures design). Assumption #4: ? There should be no significant outliers. Outliers are simply data points within your data that do not follow the usual pattern (e.g., in a study of 100 students' IQ scores, where the mean score was 108 with only a small variation between students, one student had a score of 156, which is very unusual, and may even put her in the top 1% of IQ scores globally). The problem with outliers is that they can have a negative effect on the one-way ANCOVA, reducing the validity of your results. Fortunately, when using SPSS Statistics to run a one-way ANCOVA on your data, you can easily detect possible outliers. Assumption #5 ? Your residuals should be approximately normally distributed for each category of the independent variable. We talk about the ANCOVA only requiring approximately normal residuals because it is quite "robust" to violations of normality, meaning that the assumption can be violated to a degree and still provide valid results. You can test for normality using two Shapiro-Wilk tests of normality: one to test the within-group residuals and one to test the overall model fit. Both of these are easily tested for using SPSS Statistics. Assumption #6 ? There needs to be homogeneity of variances. You can test this assumption in SPSS Statistics using Levene's test for homogeneity of variances. Assumption #7: ? The covariate should be linearly related to the dependent variable at each level of the independent variable. You can test this assumption in SPSS Statistics by plotting a grouped scatterplot of the covariate, post- test scores of the dependent variable and independent variable. Assumption #8 ? There needs to be homoscedasticity. You can test this assumption in SPSS Statistics by plotting a scatterplot of the standardized residuals against the predicted values. Assumption #9 ? There needs to be homogeneity of regression slopes, which means that there is no interaction between the covariate and the independent variable. By default, SPSS Statistics does not include an interaction term between a covariate and an independent in its GLM procedure so that you can test this. REMINDER ? You can check assumptions #4, #5, #6, #7, #8 and #9 using SPSS Statistics. Before doing this, you should make sure that your data meets assumptions #1, #2 and #3, although you don't need SPSS Statistics to do this. Remember that if you do not run the statistical tests on these assumptions correctly, the results you get when running a one-way ANCOVA might not be valid. TWO-WAY ANCOVA DEFINITION ? The two-way ANCOVA (also referred to as a "factorial ANCOVA") is used to determine whether there is an interaction effect between two independent variables in terms of a continuous dependent variable (i.e., if a two-way interaction effect exists), after adjusting/controlling for one or more continuous covariates. ? In many ways, the two-way ANCOVA can be considered an extension of the one-way ANCOVA, which has just one independent variable (rather than two independent variables), or an extension of the two-way ANOVA to incorporate one or more continuous covariates. Assumption #1 ? Your dependent variable should be measured at the continuous level (i.e., it is an interval or ratio variable). Examples of continuous variables include revision time (measured in hours), intelligence (measured using IQ score), exam performance (measured from 0 to 100) and weight (measured in kg). ◦ Important: If your dependent variable is not measured on a continuous scale, but is either a count variable, ordinal variable, nominal variable or dichotomous variable, the two-way ANCOVA would not be an appropriate statistical test. Assumption #2 ? Your two independent variables should each consist of two or more categorical, independent groups. Categorical variables include both nominal variables and ordinal variables. Examples of nominal variables include gender (two groups: male or female) and ethnicity (three groups: Caucasian, African American and Hispanic) and profession (four groups: surgeon, doctor, nurse and dentist). ? Examples of ordinal variables include BMI (two levels: "normal" and "obese"), physical activity level (four levels: "sedentary", "low", "moderate" and "high"), Likert items (e.g., a 7-point scale from "strongly agree" through to "strongly disagree"), amongst other ways of ranking categories (e.g., a 3-point scale explaining how much a customer liked a product, ranging from "Not very much", to "It is OK", to "Yes, a lot"). Assumption #2 (Notes) ? Note 1: It is quite common for the independent variables to be called "factors" or "between-subjects factors", but we will continue to refer to them as independent variables. Furthermore, the two-way ANCOVA is also referred to as a "factorial ANCOVA" because ANCOVAs with two or more independent variables are all classified as factorial ANCOVAs. ? Note 2: A two-way ANCOVA can be described by the number of groups in each independent variable. For example, if you had a two-way ANCOVA with "gender" (2 groups: "male" and "female") and "transport type" (3 groups: "bus", "train" and "car") as the independent variables, and salary as a covariate, you could describe this as a 2 x 3 ANCOVA. This is a fairly generic way to describe ANCOVAs. Assumption #3: ? Your one or more covariates, also known as control variables, are all continuous variables (see Assumption #1 for examples of continuous variables). A covariate is simply a continuous independent variable that is added to an ANOVA model to produce an ANCOVA model. This covariate is used to adjust the means of the groups of the two categorical independent variables. ? In an ANCOVA the covariate is generally only there to provide a better assessment of the differences between the groups of the categorical independent variables in terms of the dependent variable. Assumption #4 ? You should have independence of observations, which means that there is no relationship between the observations in each group or between the groups themselves. For example, there must be different participants in each group with no participant being in more than one group. This is more of a study design issue than something you would test for, but it is an important assumption of the two-way ANCOVA. If your study fails this assumption, you will need to use another statistical test instead of the two-way ANCOVA (e.g., a repeated measures design). Assumption #5 ? The covariate should be linearly related to the dependent variable for each combination of groups of the independent variables (i.e., each cell of the design). When we refer to each cell of the design or each combination of groups of the independent variables, consider the following example where the independent variable, "diet", has two groups and the independent variable, "exercise", has three levels: Assumption #5 cont.… ? In this example there are six cells in the design (i.e., 2 groups x 3 levels = 6 cells of the design). You can test this assumption in SPSS Statistics by plotting a grouped scatterplot and adding loess lines to make the interpretation easier. LOESS ? LOWESS (Locally Weighted Scatterplot Smoothing), sometimes called LOESS (locally weighted smoothing), is a popular tool used in regression analysis that creates a smooth line through a timeplot or scatter plot to help you to see relationship between variables and foresee trends. What is Lowess Smoothing used for? ? LOWESS is typically used for: ◦ Fitting a line to a scatter plot or time plot where noisy data values, sparse data points or weak interrelationships interfere with your ability to see a line of best fit. ◦ Linear regression where least squares fitting doesn’t create a line of good fit or is too labor- intensive to use. ◦ Data exploration and analysis in the social sciences, particularly in elections and voting behavior. LOESS: Parametric and Non- Parametric Fitting ? LOWESS, and least squares fitting in general, are non- parametric strategies for fitting a smooth curve to data points. “Parametric” means that the researcher or analyst assumes in advance that the data fits some type of distribution (i.e. the normal distribution). Because some type of distribution is assumed in advance, parametric fitting can lead to fitting a smooth curve that misrepresents the data. In those cases, non-parametric smoothers may be a better choice. Non-parametric smoothers like LOESS try to find a curve of best fit without assuming the data must fit some distribution shape. In general, both types of smoothers are used for the same set of data to offset the advantages and disadvantages of each type of smoother. PROCEDURE ? LOESS is available from the Fit Line tab of the Properties panel when you edit a scatterplot in the chart editor. ? Produce a scatter plot: Graphs > Scatter/Dot menu. ? Select any option you like. ? Double-click the scatterplot to open it in the chart editor. ? Select Elements > Fit Line at total or possibly Elements > Fit Line at Subgroups. ? Check Loess: you can change default size of the smoothing window (expressed in % of the observations (neighborhood size of the slices) and you might also change the default "kernel method", i.e the way the smooth values are computed: the options are in fact different weighting schemes. Uniform gives the same weight to all values, other options weight observations towards the edge of the window less. ? Below you will find an example: with a loess curve added to a plot for each continent. Assumption #6 ? There should be homogeneity of regression slopes. This assumption checks that the relationship between the covariate and the dependent variable, as assessed by the regression slope, is the same in each cell of the design (i.e., for each combination of groups of the two independent variables). ? Simply put, the previous assumption assessed whether the relationships were linear; this assumption now checks whether these linear relationships are the same. This assumption can also be tested in SPSS Statistics, but it requires quite a few steps, including using the Compute Variable and Univariate procedures in SPSS Statistics. Assumption #7 ? There should be homoscedasticity. An important assumption of the two-way ANCOVA is that the variance of the error is identical for all combinations of the values of the independent variables and covariate. This can be tested in two parts, one of which can be referred to as testing for homoscedasticity; that is, there should be homoscedasticity of error variances within each combination of groups of the two independent variables (i.e., within each cell of the design) (Huitema, 2011). ? Homoscedasticity can be checked in SPSS Statistics by inspecting a plot of the studentized residuals against the predicted values for each cell of the design (i.e., each combination of groups of the independent variables). Assumption #8 ? There should be homogeneity of variances. To reiterate from Assumption #7 above, an important assumption of the two-way ANCOVA is that the variance of the error is identical for all combinations of the values of the independent variables and covariate. The second part to testing this assumption is referred to as testing for homogeneity of variances; that is, the variances of the residuals should be equal between each combination of groups of the two independent variables (i.e., between each cell of the design) (Huitema, 2011). If the variances are unequal, this can affect the Type I error rate. This can be tested in SPSS Statistics using Levene's test of equality of variances. Assumption #9 ? There should be no significant unusual points in any combinations of groups of your two independent variables. There can be certain data points that are, in some way, classified as unusual from the perspective of fitting a two-way ANCOVA model. These data points are generally detrimental to the fit or generalization (statistical inference) of the two-way ANCOVA. There are three main types of unusual point: outliers, leverage points and influential points. An observation can be classified as more than one type of unusual point. ? Whilst all are unusual, these different classifications of unusual point reflect the different impact they have on the two-way ANCOVA model. For example, you can have observations in your data set that have an unusual combination of values on the independent variables (i.e., leverage points) or affect the parameter estimates of the two-way ANCOVA in a detrimental manner (i.e., influential points). You can check for unusual points in SPSS Statistics by inspecting the values of the studentized residuals, the leverage values and Cook's distance values. Assumption #10 ? Your residuals should be approximately normally distributed for each combination of groups of the two independent variables. There are many different methods available to test this assumption, including numerical methods such as the Shapiro-Wilk test for normality, as well as graphical methods such as normal Q-Q plots. REMINDER ? You can check assumptions #5, #6, #7, #8, #9 and #10 using SPSS Statistics. Before doing this, you should make sure that your data meets assumptions #1, #2, #3 and #4, although you don’t need SPSS Statistics to do this. Just remember that if you do not run the statistical tests on these assumptions correctly, the results you get when running a two-way ANCOVA might be incorrect.