Artificial Intelligence in Medical Education PDF
Document Details
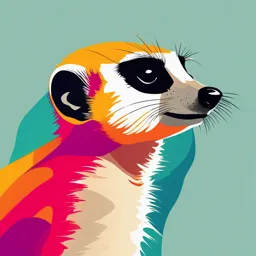
Uploaded by HardierOsmium
Sultan Qaboos University
2019
Ken Masters
Tags
Related
- Artificial intelligence PDF
- Readiness Towards Artificial Intelligence in Medical and Dental Students in Peshawar PDF
- Artificial intelligence in Imaging-1 PDF
- Artificial Intelligence (AI) Fact Sheet PDF
- A Short Guide for Medical Professionals in the Era of Artificial Intelligence PDF
- Medical Biochemistry and Artificial Intelligence PDF
Summary
This article discusses the ways artificial intelligence technologies are impacting medical education. It explores the history of thinking machines and AI, focusing on examples like AlphaGo and Microsoft's Tay. Lessons for educators, doctors, and the broader field are also explored.
Full Transcript
Medical Teacher ISSN: 0142-159X (Print) 1466-187X (Online) Journal homepage: https://www.tandfonline.com/loi/imte20 Artificial intelligence in medical education Ken Masters To cite this article: Ken Masters (2019): Artificial intelligence in medical education, Medical Teacher, DOI: 10.108...
Medical Teacher ISSN: 0142-159X (Print) 1466-187X (Online) Journal homepage: https://www.tandfonline.com/loi/imte20 Artificial intelligence in medical education Ken Masters To cite this article: Ken Masters (2019): Artificial intelligence in medical education, Medical Teacher, DOI: 10.1080/0142159X.2019.1595557 To link to this article: https://doi.org/10.1080/0142159X.2019.1595557 Published online: 21 Apr 2019. Submit your article to this journal Article views: 5 View Crossmark data Full Terms & Conditions of access and use can be found at https://www.tandfonline.com/action/journalInformation?journalCode=imte20 MEDICAL TEACHER https://doi.org/10.1080/0142159X.2019.1595557 AMEE GUIDE Artificial intelligence in medical education Ken Masters Sultan Qaboos University, Muscat, Sultanate of Oman ABSTRACT Artificial intelligence (AI) is a growing phenomenon, and will soon facilitate wide-scale changes in many professions, includ- ing medical education. In order for medical educators to be properly prepared for AI, they will need to have at least a fun- damental knowledge of AI in relation to learning and teaching, and the extent to which it will impact on medical education. This Guide begins by introducing the broad concepts of AI by using fairly well-known examples to illustrate AI’s implications within the context of education. It then considers the impact of AI on medicine and the implications of this impact for educators trying to educate future doctors. Drawing on these strands, it then identifies AI’s direct impact on the methodology and content of medical education, in an attempt to prepare medical educators for the changing demands and opportunities that are about to face them because of AI. Introduction Thinking machines and artificial intelligence: a Practice points brief history Artificial intelligence (AI) will have an impact on all professions, including those of medicine and The notion of thinking machines has existed for centuries, medical education. from Descartes’ Automata (Descartes 1637) to Charles Current experiments in AI indicate that the teach- Babbage’s Analytical Engine, of which Lady Lovelace said er’s role is crucial to good AI development. could only perform “whatever we know how to order it to The result of AI in medicine will not be to replace perform”(Menabrea 1843). In 1950, Alan Turing (Turing 1950) the doctor, but to replace and enhance many of asked, “Can machines think?” and constructed his Imitation the doctors’ roles, and create a range of Game (now called the Turing Test). In this test, a human sub- new roles. ject interacts with a machine and another human, and, if It is crucial that these changes be known in the subject cannot tell the difference between machine and advance, so that medical education can begin human, then the machine can be said to be “thinking.” preparing medical students for these new roles. In the twenty-first century, we have the first forays into artificial intelligence (AI). (For this Guide, I propose a work- ing definition of AI as the behaviors by computer software that are designed to mimic and extend human rational think- AI systems take the basics of what they have been taught, ing and actions, based loosely on general texts, such as grapple with them, get confused, attempt resolutions, Poole et al. (1998) and Russel & Norvig (2009)). extend, and apply. It is too simplistic to argue that good There have been AI failures, such as Microsoft’s Tay, an AI teachers automatically lead to good education, but we do system designed to learn from, and interact with, Twitter know that teachers influence students and their behavior; users. Unfortunately, Tay was taught by Twitter users to be Tay and AlphaGo illustrate that good teaching can provide a racist, sexist bigot, and was taken offline (Vincent 2016). the platform for good AI learning, and bad teaching can Contrastingly, there have been AI successes, such as harm AI. So, given that AI can learn, could we not use it Google’s AlphaGo, which, in 2016, stunned the world with to teach? a 4-1 victory over Go world champion Lee Se-dol (Sang- Hun 2016). Unlike Babbage’s Analytical Engine, both Tay and Teachers AlphaGo had entered the realm of the thinking machine, extending their knowledge and thinking beyond what they In 1980, Arthur C Clarke wrote “Any teacher who can be had been taught; unlike Tay, however, AlphaGo had been replaced by a machine should be!”(Clarke 1980). In a view taught well, and had behaved acceptably. of education as simple knowledge-transfer, replacing human teachers with machines has appeal. In reality, good teaching involves accessing information from many sour- Lessons for education ces, collating, prioritizing, adapting, and then using it Educational lessons can be learned from AI failures and within narrow (even unique) circumstances with extremely successes. Most important is that, just like human learners, subtle variations. In addition, creative teachers frequently CONTACT Ken Masters [email protected] Medical Education and Informatics Department, College of Medicine and Health Sciences, Sultan Qaboos University, Sultanate of Oman, Al-Khoud, Muscat 123, Oman. ß 2019 AMEE 2 K. MASTERS use materials that are not “educational” in any formal see this in perspective, we should reflect on salient issues. sense, but are useful to stimulate thinking, introduce These include the following: topics, or push boundaries beyond the narrow curriculum. This ability does not yet exist in computers. Appalling medical experiments performed around the AI will undoubtedly impact on medical education meth- world in the twentieth century (Masters 2018a); ods, including intelligent tutoring systems’ identifying and Declining empathy levels among medical students as responding to gaps in students’ knowledge, adaptable vir- they progress through their medical degree (Hojat et al. tual facilitators in constructivist learning approaches, data 2009; Neumann et al. 2011; Chen et al. 2012); mining (as opposed to using “search engines”), intelligent Pharmaceutical companies’ price gouging in pursuing feedback to students and teachers, and performing mun- financial profits (Hemphill 2010; Greene et al. 2016; dane tasks like assignment grading and attendance track- Wardle and Wood 2017) and fearing the Genomic ing. These will affect all education. Revolution because cures will eat into their profits To better see the potential of AI in medical education, (Kim 2018); we should consider the end of the process: good and com- Hospitals’ forcing doctors to rush through wards, giving petent doctors. Just as any change in medical education curt responses to patients, and schedules forcing doc- should be guided by this end goal, we need to ask, given tors to reduce consultations to 10 or 15 minutes; the potential that we have already seen for AI to learn, Insurance doctors’ overriding treating physicians and rather than replacing teachers, should we rather be aiming deciding on “best” treatments for patients without any at AI doctors? physical contact with those patients. A task for practic- ing doctors: consider some decisions made by those insurance doctors affecting your patients: on average, Doctors would an AI system do any worse? And, as AI systems Already, computers can perform prediction and diagnosis learn empathy (Huang and Rust 2018), their Human better than humans (Bogusevicius et al. 2002; Esteva et al. Touch will improve. Artificial Human Touch is better 2017; Litjens et al. 2017; Mobadersany et al. 2017; Betancur than no Human Touch at all. et al. 2018; De Fauw et al. 2018; Haenssle et al. 2018). But how far off is AI before society accepts an AI doctor? To Yes, there is a need for direct human interaction, espe- get some idea, we need to remember a few things: cially when breaking bad news, counselling and small pro- cedures like checking blood pressure, suturing, and so AlphaGo’s race to world champion was stunningly swift forth. But we do not need doctors for this. Breaking bad (Cho 2016), and we can expect rapid advances in all news and counselling is best performed by properly fields, including medicine. trained counsellors. Unless we move counselling from “also AlphaGo is a single system. A medical AI system would taught” to a major focus in the curriculum, we should leave be a global neural network utilizing hundreds of thou- this work to professionals. Small procedures like blood- sands of computers through the internet of things (IoT). pressure recording and suturing are best performed by Although evidence-based medicine (EBM) is the gold well-trained nursing staff. standard, there is no proven effective method of Can doctors be replaced by computers? While the research data dissemination and acquisition, and snappy answer is, “Any doctor who can be replaced by a research growth puts this ideal further from our grasp machine should be!”, reality is more subtle. Perhaps, rather every day. Early experiments show that AI is ideally than replacing doctors, we should look at using AI to suited to automatically accessing relevant data from replace or enhance some of the doctors’ roles. We need to published research and from electronic medical records understand these new roles so that we can adjust medical (EMRs), allowing systems and doctors to stay current education accordingly. (Tafti et al. 2017). To be a competent doctor, an AI system does not have to be the best doctor in the world. AI has to be better Replacing and teaching the new doctors’ roles than only the worst graduating student in your class. Identifying AI roles Further, assuming a standard distribution curve, if AI is better than your average student, it is better than 50% The literature cited above indicates that AI is able to per- of all doctors. Moreover, as seen from the references form some differential diagnosis and clinical reasoning above, there are already systems that can perform sev- more competently than humans (ironically, this ability eral tasks better than some of the best, so AI is on track would mean that the computer would “fail” the Turing test to achieve this. (Turing 1950)). Yet AI diagnostic systems carry warnings And finally, how confident are you in other aspects of that they should not replace qualified physicians, and even all your graduating students, such as their principles patients want diagnosis confirmation by physicians (Fink and ethics? et al. 2018). This is in spite of the fact that some AI systems have been designed precisely because trained and qualified physicians made mistakes (Isabel Healthcare 2018). In The human touch essence, we are deferring to a system that is proven to We may counter these contentions by considering the have inferior clinical reasoning skills, purely on the grounds Human Touch and empathy that all involved in healthcare that that system is human. Yet, perhaps unnoticed, we have for patients (Cayley 2006). Can AI do that? Again, to already have the early stages of role replacement: the EMR. MEDICAL TEACHER 3 Role replacement: early stages and psychological impact will be tremendous. In add- ition to technical training, wider questions around eth- EMRs herald great potential benefit to health services, yet ics, roles, protocols, and liability need to be addressed. there is so much criticism of EMRs by practicing doctors. Communicating with AI systems. Doctors need to be The most common is that data capture interferes with nor- taught how to inform AI systems of the relevant infor- mal health care workflow practice and procedures that spe- mation, so that EMRs can match new information to cifically require direct eye contact between doctor and information inside and outside the EMR, and prompt patient (Unger 2015; Gaither 2016; Sinsky et al. 2016; doctors to request further information on areas that Shanafelt et al. 2016). The solution seems to be to rectify appear unclear. This is an iterative process. In addition, these EMR design flaws, so that EMRs do fit in with normal doctors require careful training on verbal communica- workflow practice and procedures. tion, written communication, voice input, and translat- Unfortunately, this “solution” shows a misunderstanding ing tactile information, suspicions and hunches into of the EMR’s role in modern healthcare. Removing doctors’ digital information, and how to use data-mining tools eyes from patient to computer is not a design flaw; it is a within clinical environments. design feature. EMR systems are not designed fit in with Doctors also need to be taught how to engage with normal workflow practice; EMR systems are designed to AI systems that develop “personal” relationships with change normal workflow practice. In business terms, EMRs patients, watching for early warning signs, and simul- are not sustaining technologies, they are disruptive technol- taneously allowing patients to easily transfer between ogies (Christensen and Armstrong 1998). And, as EMR sys- providers, gathering and collating data, identifying tems increasingly interact with AI systems, and as EMR trends and anomalies, and triggering appropriate organ- systems increasingly become AI systems, so they will isational responses. increasingly disrupt normal workflow practice. Deeper counselling and related activities. Related to com- The frustration and anger that doctors feel now is munication skills, doctors need to be taught improved because they are at the initial stages of an important new counselling skills. Doctors without these skills would be role, largely characterised by gathering and entering more suitably employed away from patients, and coun- patient data into EMR and AI systems. This may be unwel- selling can be performed by qualified counsellors. This come news to doctors, as they may see themselves will grow in importance as AI opens new medical fields, reduced to data capturers, but it should not be surprising, such as enhanced medicine (medical procedures enhanc- in spite of what doctors may have been told about the ing the human mind and body beyond what is currently role of EMRs. In real terms, what doctors are experiencing considered “normal”). is merely the early stage of EMR usage while AI catches up Psychological reorientation of one’s roles. Some doctors’ and moves into the next stage: role change and reversal. roles will change so much that they will no longer be recognisable, and many roles might no longer fit the Role change, new roles, and teaching for them pattern of what we currently mean by the word “doctor.” This will have to be addressed at all levels, In practical terms, what does AI mean for future doctors’ especially in Continuing Medical Education (CME). roles? While it is impossible to foresee all implications, Teaching new medical AI. From Tay and AlphaGo, we some are obvious, and have been mentioned in preceding know that we need doctors who can teach AI properly. sections. This section looks at new possible roles and Initially, patient information will have to be gathered by changes to medical curricula content that will be required doctors and given to AI systems. But AI will have to be to meet these new roles. taught to gather this information itself, and how to work properly with it. Being proactive in AI system design. Successful disruptive We already have examples of medical AI system technologies frequently introduce simplification errors because of poor teaching—IBM’s Watson was (Christensen and Armstrong 1998), but that cannot be taught oncology skills through hypothetical cases only said about many current EMR systems. To balance (Ross and SwetlitzIke 2018), a practice considered change with maintained healthcare, doctors should unacceptable when teaching human doctors. It was not organise themselves into influencing groups and assist surprising that it made so many mistakes. Good AI directly in designing and trialing EMR and AI systems. needs teams of experts from medical, educational and Doctors should be taught at least basic medical inform- computing fields. atics, and have a sense of EMR and AI design principles Complex issues already inherent in medical informat- so that they can work directly with designers, ensuring ics’ ethics (Masters 2018a) will need to be built into that systems meet healthcare ethical, medical and prac- medical AI as guiding principles. Only by including tical requirements. these ethical principles into AI, can AI move from Working with AI diagnostic systems. Doctors need to Artificial Intelligence to Artificial Wisdom. know how to work constructively with AI diagnostic Individual EMR AI systems will need to be taught to and other systems to best serve their patients’ needs. communicate with greater, global AI systems, as smooth For this, they will require training on these systems to communication is crucial for effective system evolution. become as familiar with them as they are with any But the technical areas form only one aspect. A larger other medical tools. Training should start now. Waiting area requiring addressing is language. Medical jargon is until these systems are pervasive before beginning fraught with inconsistencies (Lee and Whitehead 2017), training will be counterproductive, as the learning curve and AI systems can already address these restrictions, 4 K. MASTERS and have communicated with each other far more effi- Some readers may be skeptical about the seemingly sci- ciently than humans can. The problem is that humans ence-fiction outlook of this Guide. One should remember, cannot understand machine-developed AI languages, however, that tens of billions of dollars are poured into AI and have responded by shutting down the systems research annually (Bughin et al. 2017), and the amount of (Wong 2016). We will need medical practitioners who data currently available to computer systems is unprece- can work with these new languages. dented; AI will impact directly on every aspect of our lives, Robotics. Although in its infancy, and mostly under and there is no reason to believe that medicine and med- human control, surgical robotics has already shown ical education will be spared. It is our responsibility to pre- potential in changing surgery, and the direct positive pare ourselves, our students and our doctors, for the future impact on healthcare will be profound (Nag et al. 2017; with AI, so that quality healthcare can be delivered. Schroerlucke et al. 2017; Porpiglia et al. 2018; Stiegler Those who ignore AI will have temporary comfort, but, and Schemmer 2018; Stravodimos et al. 2019). when they finally realise that it is all around them, they will Intelligent robots are simply robots with AI software, wonder how they missed it. and this software will eventually perform surgery with- Far from replacing doctors, students, or medical educa- out humans. Soon, these surgical methods will be tors, AI will open new horizons. It is not so much that standard, and medical schools that are not teaching those who can be replaced by AI will be; it is more that robotic surgery will fall behind rapidly. those who wish to be replaced by AI will be. For the rest, E-patients. Although the concept of e-patients (Masters the opportunities will be vast. Whatever the future, “We 2017; Masters 2018b) is beyond this Guide’s scope, we can only see a short distance ahead, but we can see plenty there that needs to be done” (Turing 1950). can note that AI will dramatically impact upon the e- patient. Patients will become accustomed to seeing, may expect to see, doctors’ using AI, and be judgemen- Acknowledgments tal of those who are not. I wish to thank Dr David Taylor, editor of the AMEE Guide series, and As AI improves, patients will frequently by-pass doc- anonymous reviewers who commented on earlier drafts of this Guide tors, preferring to interact with AI systems from their for their invaluable input. home or other remote locations through voice, wear- able and implanted computers. Combining these facets will change the very nature of the patient, as humans Disclosure statement evolve into Homo Nodus (Masters 2015), a node on a The author reports no conflicts of interest. The author alone is respon- vast Internet of Things. sible for the content and writing of this article. A particular difficulty will be AI companies’ wishing to prevent patients’ accessing personal AI systems directly, Notes on contributor or without paying a substantial fee. This discussion is already old, as the goal is to move beyond hospital- Dr Ken Masters, PhD, HDE, FDE, is Associate Professor of Medical Informatics, Medical Education and Informatics Department, Sultan owned and controlled EMR systems to encrypted, Qaboos University, Sultanate of Oman. He has been involved in educa- patient-owned and controlled systems, to which tion for over 30 years and in medical education for over a decade. His patient’s AI systems grant health personnel access only publications in medical education consider various theoretical peda- when needed, that access is authenticated and tracked, gogical perspectives, technologies, teaching strategies, and softer areas such as ethics. He teaches the concept of artificial intelligence to and data usage is tracked and audited. Doctors have to medical students as part of a broader medical informatics course. be taught how to interact with e-patients as part of the AI environment. ORCID It is inevitable that similar to the way in which industri- alisation led to “deskilling”(Frey and Osborne 2013), many Ken Masters http://orcid.org/0000-0003-3425-5020 health professionals’ tasks and roles will be taken over by AI, new roles will be necessary, and medical education must prepare for those new roles. Detailed curricula need References to be developed and updated as the technology and envir- onment evolve. Much material development will need to Betancur J, Commandeur F, Motlagh M, Sharir T, Einstein AJ, Bokhari S, Fish MB, Ruddy TD, Kaufmann P, Sinusas AJ, et al. 2018. Deep learn- be performed by development teams that include patients. ing for prediction of obstructive disease from fast myocardial perfu- sion SPECT. A multicenter study. JACC Cardiovasc Imaging. 11: 1654–1663. Conclusion Bogusevicius A, Maleckas A, Pundzius J, Skaudickas D. 2002. Prospective randomised trial of computer-aided diagnosis and con- This Guide has considered thinking machines from trast radiography in acute small bowel obstruction. Eur J Surg. 168: Descartes and Turing to Artificial Intelligence with AlphaGo. 78–83. As AI moves deeper into medical practice, we may have Bughin J, Hazan E, Ramaswamy S, Chui M, Allas T, Dahlstro €m P, Henke fears that the “real” work will be done by computers, and N, Trench M. 2017. Artificial Intelligence – The Next Digital Frontier? doctors will have nothing to do, and will know nothing. New York City. Available from: www.mckinsey.com/mgi. Cayley WE. 2006. Comfort always. Fam Pract Manag. 13:74. That is possible, if we want it to be. Just as likely, however, Chen DCR, Kirshenbaum DS, Yan J, Kirshenbaum E, Aseltine RH. 2012. new roles for health professionals will emerge, many roles Characterizing changes in student empathy throughout medical not yet foreseen, roles requiring new medical education. school. Med Teach. 34:305–311. MEDICAL TEACHER 5 Cho A. 2016. ‘Huge leap forward’: Computer that mimics human brain Masters K. 2018b. AMEE Guide 116: preparing medical students for the beats professional at game of Go. Science (80-) [Internet]. https:// e-patient. Dundee, (UK): Association for Medical Education in www.sciencemag.org/news/2016/01/huge-leap-forward-computer- Europe (AMEE). mimics-human-brain-beats-professional-game-go. Menabrea L. 1843. Sketch of the analytical engine invented by Charles Christensen C, Armstrong E. 1998. Disruptive technologies: a credible Babbage. London, UK: Richard & John Taylor. threat to leading programs in continuing medical education? Mobadersany P, Yousefi S, Amgad M, Gutman DA, Barnholtz-Sloan JS, J Contin Educ Health Prof. 18:69–80. Vega JEV, Brat DJ, Cooper L. 2017. Predicting cancer outcomes from Clarke AC. 1980. Electronic tutors. OMNI. 2:76–96. histology and genomics using convolutional networks. PNAS. Descartes. 1637. Discourse on the method. Descartes Key Philos bioRxiv:198010. writings. Nag H, Sisodia K, Sheetal P, Govind H, Chandra S. 2017. Laparoscopic Esteva A, Kuprel B, Novoa RA, Ko J, Swetter SM, Blau HM, Thrun S. excision of the choledochal cyst in adult patients: an experience 2017. Dermatologist-level classification of skin cancer with deep Hirdaya. J Minim Access Surg. 13:261. neural networks. Nature. 542:115–118. Neumann M, Edelh€auser F, Tauschel D, Fischer MR, Wirtz M, Woopen De Fauw J, Ledsam JR, Romera-Paredes B, Nikolov S, Tomasev N, C, Haramati A, Scheffer C. 2011. Empathy decline and its reasons: a systematic review of studies with medical students and residents. Blackwell S, Askham H, Glorot X, O’Donoghue B, Visentin D, et al. Acad Med. 86:996–1009. 2018. Clinically applicable deep learning for diagnosis and referral Poole D, Mackworth A, Goebel R. 1998. Artificial intelligence: a logical in retinal disease. Nat Med. 24:1342–1350. http://www.nature.com/ approach. New York: Oxford University Press. articles/s41591-018-0107-6. Porpiglia F, Bertolo R, Amparore D, Checcucci E, Artibani W, Dasgupta Fink C, Uhlmann L, Hofmann M, Forschner A, Eigentler T, Garbe C, Enk P, Montorsi F, Tewari A, Fiori C. 2018. Augmented reality during A, Haenssle HA. 2018. Patient acceptance and trust in automated robot-assisted radical prostatectomy: expert robotic surgeons’ on- computer-assisted diagnosis of melanoma with dermatofluoroscopy. the-spot insights after live surgery. Minerva Urol Nefrol. 70:226–229. J Dtsch Dermatol Ges. 16:854–859. Ross C, SwetlitzIke I. 2018. IBM’s Watson supercomputer recommended Frey CB, Osborne MA. 2013. The future of employment on technology ‘unsafe and incorrect’ cancer treatments, internal documents show. and employment. Oxford, UK. STAT [Internet] [cited 2018 Aug 30]. https://www.statnews.com/ Gaither C. 2016. 11 undeniable truths about EHRs and paper charts. 2018/07/25/ibm-watson-recommended-unsafe-incorrect-treatments/. MD [Internet]. [cited 2018 Sep 11]. http://www.mdmag.com/con- Russel SJ, Norvig P. 2009. Artificial intelligence: a modern approach. tributor/clark-gaither-md/2016/05/11-undeniable-truths-about-ehrs- 3rd ed. Upper Saddle River: Pearson. and-paper-charts/P-1. Sang-Hun C. 2016. Google’s computer program beats Lee Se-dol in go Greene JA, Anderson G, Sharfstein JM. 2016. Role of the FDA in afford- tournament. New York Times [Internet]. https://www.nytimes.com/ ability of off-patent pharmaceuticals. J Am Med Assoc. 315:461–462. 2016/03/16/world/asia/korea-alphago-vs-lee-sedol-go.html. Haenssle HA, Fink C, Schneiderbauer R, Toberer F, Buhl T, Blum A, Schroerlucke SR, Wang MY, Cannestra AF, Good CR, Lim J, Hsu VW, Kalloo A, Hassen ABH, Thomas L, Enk A, et al. 2018. Man against Zahrawi F. 2017. Complication rate in robotic-guided vs fluoro- machine: diagnostic performance of a deep learning convolutional guided minimally invasive spinal fusion surgery: report from MIS neural network for dermoscopic melanoma recognition in compari- refresh prospective comparative study. Spine J. 17:S254–S255. son to 58 dermatologists. Ann Oncol. 29:1836–1842. Shanafelt TD, Dyrbye LN, Sinsky C, Hasan O, Satele D, Sloan J, West Hemphill TA. 2010. Extraordinary pricing of orphan drugs: is it a CP. 2016. Relationship between clerical burden and characteristics socially responsible strategy for the U.S. pharmaceutical industry? of the electronic environment with physician burnout and profes- J Bus Ethics. 94:225–242. sional satisfaction. Mayo Clin Proc. 91:836–848. Hojat M, Vergare MJ, Maxwell K, Brainard G, Herrine SK, Isenberg G. a, Sinsky C, Colligan L, Li L, Prgomet M, Reynolds S, Goeders L, Veloski J, Gonnella JS. 2009. The Devil is in the third year: a longitu- Westbrook J, Tutty M, Blike G. 2016. Allocation of physician time in dinal study of erosion of empathy in medical school. Acad Med. 84: ambulatory practice: a time and motion study in 4 specialties. Ann 1182–1191. Intern Med. 165:753–760. Huang M-H, Rust RT. 2018. Artificial intelligence in service. J Serv Res. Stiegler P, Schemmer P. 2018. Robot-assisted transplant surgery - 21:1–18. vision or reality? A comprehensive review. Visc Med. 34:24–30. Isabel Healthcare. 2018. The Isabel Story. Isabel [Internet]. [cited 2018 Stravodimos K, Katafigiotis I, Pournaras C, Dimitroulis D, Kousournas G, Fragkiadis E, Constantinides C. 2019. Combined robot assisted right Dec 17]. https://www.isabelhealthcare.com/about-isabel-healthcare/ partial nephrectomy and cholecystectomy with single docking. isabel-story. J Robot Surg. 13:167–169. Kim T. 2018. Goldman Sachs asks in biotech research report: “Is curing Tafti AP, Badger J, LaRose E, Shirzadi E, Mahnke A, Mayer J, Ye Z, Page patients a sustainable business model?” CNBC. https://www.cnbc. D, Peissig P. 2017. Adverse drug event discovery using biomedical com/2018/04/11/goldman-asks-is-curing-patients-a-sustainable-busi- literature: a big data neural network adventure. JMIR Med Inform. 5: ness-model.html. e51. http://medinform.jmir.org/2017/4/e51/. Lee B, Whitehead MT. 2017. Radiology reports: what YOU think you’re Turing A. 1950. Computing machinery and intelligence. Mind. 59: saying and what THEY think you’re saying. Curr Probl Diagn Radiol. 433–460. 46:186–195. Unger JR. 2015. Med students: look up from your EMRs. J Fam Pract. Litjens G, Kooi T, Bejnordi BE, Setio AAA, Ciompi F, Ghafoorian M, van 64:517–518. der Laak J, van Ginneken B, Sanchez CI. 2017. A survey on deep Vincent J. 2016. Twitter taught Microsoft’s AI chatbot to be a racist learning in medical image analysis. Med Image Anal. 42:60–88. asshole in less than a day. The Verge [Internet]. https://www.the- Masters K. 2015. Homo Nodus: preparing for the next stage of the verge.com/2016/3/24/11297050/tay-microsoft-chatbot-racist. internet. J Med Educ. 5:1–4. https://print.ispub.com/api/0/ispub-art- Wardle N, Wood I. 2017. How drug prices are hiked and what is being icle/32411. done to tackle the problem price-hike methods employed by some Masters K. 2017. Preparing medical students for the e-patient. Med pharmaceutical companies. Pharm J. 7901:6–11. Teach. 39:681–685. Wong S. 2016. Google Translate AI invents its own language to trans- Masters K. 2018a. Health informatics ethics. In: Hoyt RE, Hersch WR, late with. New Sci [Internet]. https://www.newscientist.com/article/ editors. Heal informatics practice guide. 7th ed. Pensacola (FL): 2114748-google-translate-ai-invents-its-own-language -to-translate- Informatics Education; p. 233–251. with/.