Business Analytics PDF
Document Details
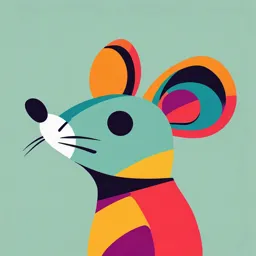
Uploaded by TopsVerse
Tags
Summary
This document provides an overview of business analytics, including its different phases, from descriptive to prescriptive. It explores the key components, and the significance in making better business decisions. It also introduces big data and AI’s role in business analytics.
Full Transcript
WHAT IS BUSINESS ANALYTICS Refers to the use of quantitative analysis to support managerial decision- making. A field which combines data, information technology, statistical analysis, quantitative methods and computer-based models, to provide decision makers of all poss...
WHAT IS BUSINESS ANALYTICS Refers to the use of quantitative analysis to support managerial decision- making. A field which combines data, information technology, statistical analysis, quantitative methods and computer-based models, to provide decision makers of all possible scenario to make a well sound and research decision (to help managers gain improved insight about their business As and make better, fact-based decisions). Used by company to provide data-driven decision making. Data analytics, business intelligence and computer programming when combined can analyze pattern that will be helpful in developing strategies a process of transforming data into actions through analysis and insights in the context of organizational decision making and problem solving.” EVOLUTION AND SCOPE OF BUSINESS ANALYTICS Operations Research (root) Descriptive Analytics (Early days): what has happened analyzing historical data to understand what has happened in the past through Data collection Basic reporting Reactive insights The Emergence of Diagnostic Analytics As technology advanced, businesses began to delve deeper into their data, leading to the development of diagnostic analytics. This phase introduced more sophisticated techniques to explore the reasons behind past performance: Data Integration: The integration of various data sources became more feasible, allowing for a comprehensive view of business operations. Data Mining: Techniques such as data mining and pattern recognition emerged, enabling businesses to identify trends, correlations, and anomalies in their data. Root Cause Analysis: Diagnostic analytics helped businesses move beyond surface- level insights to uncover the underlying causes of issues and trends. The Rise of Predictive Analytics: proactive approach Involve statistical models and machine learning techniques to predict future outcomes. Help anticipate market trends, customer behavior, and potential risks The next significant evolution in business analytics was the advent of predictive analytics, which uses historical data and statistical models to forecast future outcomes. This phase brought about: Advanced Statistical Techniques: Methods such as regression analysis, time series analysis, and machine learning algorithms became more prevalent. Scenario Modelling: Businesses began t use predictive models to simulate various scenarios and assess potential future outcomes. Proactive Decision-Making: Predictive analytics shifted the focus from understanding the past to anticipating future trends, enabling businesses to make more proactive and informed decisions. The Advent of Prescriptive Analytics Optimization and Algorithm: Best course of action The most advanced stage in the evolution of business analytics is prescriptive analytics, which not only predicts future outcomes but also suggests actions to achieve desired results. This phase is characterized by: Optimization Algorithms: The use of optimization techniques to recommend the best course of action based on specific objectives and constraints. Real-Time Analytics: The ability to analyze data in real-time and provide instant recommendations, allowing businesses to respond swiftly to changing conditions. Decision Automation: The integration of analytics with automated decision-making systems, reducing the need for human intervention in routine decisions. The Role of Big Data and Al The evolution of business analytics has been significantly accelerated by the proliferation of big data and advancements in artificial intelligence (AI). These technologies have transformed how businesses collect, process, and analyze data: Big Data: The vast volume, variety, and velocity of data generated today require advanced storage and processing capabilities. Technologies such as Hado and cloud computing have made it possible to handle big data efficiently. + Artificial Intelligence: Al and machine learning algorithms have revolutionized analytics by enabling more accurate predictions, deeper insights, and automated decision-making processes. SIGNIFICANCE AND USAGES OF BUSINESS ANALYTICS To make data-driven decisions Converts available data into valuable information Eliminate guesswork Get faster answer to questions Get insights into customer behavior Get key business metric reports when and where needed It impacts how the organization functions IMPLEMENTING BUSINESS ANALYTICS: KEY STRATEGIES To effectively leverage business analytics, organizations must adopt strategic approaches that encompass the following: 1. Data Governance and Quality: Ensuring the accuracy, consistency, and security of data is crucial. This involves: a. Data Governance Frameworks: Establishing policies and procedures for data management and usage. b. Data Quality Management: Implementing processes to maintain high data quality and address issues such as duplicates, errors, and inconsistencies. 2. Investment in Technology and Infrastructure: Building the necessary technological infrastructure to support advanced analytics capabilities is essential. This includes: a. Scalable Storage Solutions: Utilizing cloud- based storage to handle large volumes of data. b. Advanced Analytics Platforms: Investing in analytics tools and platforms that support complex analyses and real-time processing. 3. Developing Analytical Skills: Cultivating a workforce with the skills to harness the power of analytics is vital. Strategies include: a. Training and Education: Providing ongoing training programmes to develop analytical and technical skills. b. Cross-Functional Teams: Encouraging collaboration between data scientists, IT professionals, and business leaders to drive data-driven decision-making. 4. Embedding Analytics into Decision-Making Processes: Integrating analytics into the core decision-making processes of the organization is key to maximizing its impact. This involves: a. Creating a Data-Driven Culture: Fostering a culture that values data and encourages data-driven decision-making at all levels. b. Operationalizing Analytics: Embedding analytics into daily operations, strategic planning, and performance management processes. Conclusion The evolution of business analytics from descriptive to prescriptive has fundamentally changed the way businesses operate and make decisions. By leveraging the power of big data, Al, and advanced analytical techniques, organizations can gain deeper insights, anticipate future trends, and make more informed decisions. CHALLENGES FOR BUSINESS ANALYTICS Collecting meaningful data Selecting the right analytics tool Data visualization Data from multiple sources Low-quality data Data analysis skills challenges Scaling challenges Data security Budget limitations Lack of data culture Data inaccessibility BUSINESS ANALYTICS PROCESS 1. Define the business needs 2. Explore and collect data 3. Analyze the data 4. Predicts what’s likely to happen 5. Optimize –Find the best solution 6. Make a decision & measure the outcome 7. Update the system with the results of decisions COMPONENTS OF BUSINESS ANALYTICS (6 categories in analytics solution) 1. Data Mining: Create models by uncovering previously unknown trends and pattern in vast amount of data e.g. detect insurance claims frauds, Retail Market basket analysis. There are various statistical techniques through which data mining is achieved. Classification (when we know on which variables to classify the data e.g. age, demographics) Regression Clustering (when we don’t know on which factors to classify data) Associations & Sequencing Models 2. Text Mining: Discover and extract meaningful patters and relationship from text collections e.g. Understand sentiments of Customers on social media sites like Twitter, Face book, Blogs, Call centre scripts etc. which are used to improve the Product or Customer service or understand how competitors are doing. 3. Forecasting: Analyze and forecast processes that take place over the period of time E.g. Predict seasonal energy demand using historical trends, Predict how many ice creams cones are required considering demand 4. Predictive Analytics: Create, manage and deploy predictive scoring models E.g. Customer churn & retention, Credit Scoring, Predicting failure in shop floor machinery 5. Optimization: Use of simulations techniques to identify scenarios which will produce best results E.g. Sale price optimization, Identifying optimal Inventory for maximum fulfilment & avoid stock outs. 6. Visualization: Enhanced exploratory data analysis & output of modelling results with highly interactive statistical graphics. BUSINESS ANALYTICS USERS 1. Top Management: They require strategic insights and KPIs to make informed decisions and steer the company towards its objectives. 2. Data Analysts: They focus on data manipulation, analysis, and visualization to derive actionable insights for the organization. 3. IT Department: They manage data infrastructure, ensure data quality, and support analytics tools and platforms. 4. Students: They use business analytics for academic purposes, learning how to analyze data to make informed business decisions. 5. Investors: They rely on analytics to assess the financial health and potential of companies for investment decisions. 6. Accountants and Auditors: They utilize analytics for financial analysis, auditing processes, fraud detection, and compliance. 7. Planning and Operations Units: They use analytics for forecasting, resource allocation, supply chain management, and operational efficiency. 8. Human Resources: They apply analytics for talent management, workforce planning, performance evaluation, and employee engagement. 9. Sales and Marketing Units: They leverage analytics for customer segmentation, marketing campaign optimization, sales forecasting, and ROI analysis. 10. Competitors and Different Organizations: They use analytics for benchmarking, market analysis, competitive intelligence, and strategic positioning.