Difference-in-differences PDF
Document Details
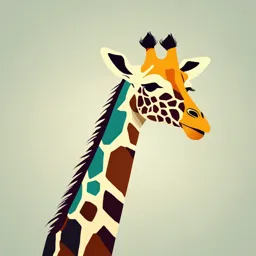
Uploaded by AppreciatedUranium
University of Bern
Blaise Melly
Tags
Summary
This document presents an overview of difference-in-differences (DiD) methodology. The author, Blaise Melly, discusses the concept for causal analysis, and details the method, including examples such as the Card and Krueger (1994) study on minimum wages and their effects on employment.
Full Transcript
Causal Analysis Parallel worlds: difference-in-differences Blaise Melly Blaise Melly Difference-in-differences 1 / 29 Difference-in-differences (DiD) What is it? Identifying assumptions Graphical and statistical...
Causal Analysis Parallel worlds: difference-in-differences Blaise Melly Blaise Melly Difference-in-differences 1 / 29 Difference-in-differences (DiD) What is it? Identifying assumptions Graphical and statistical analysis Sensitivity tests Potential concerns and limitations Alternative models Blaise Melly Difference-in-differences 2 / 29 Before / after estimator Average treatment effect for the treated E (Y1 |D = 1) − E (Y0 |D = 1) Unless randomized assignment to treatment, the outcome of the control group is biased by endogenous selection: E (Y0 |D = 1) ̸= E (Y0 |D = 0) Popular estimator: comparison of the outcome of the treatment group before and after the treatment. No selection bias, but the outcome before the treatment may not be a good estimate of the potential outcome without the treatment after the treatment: business cycle effects, increased experience, inflation,... Blaise Melly Difference-in-differences 3 / 29 Difference-in-differences methods DiD idea: mix these two estimators. In the simplest case: two groups (treatment and control): G = 1 and G = 0, two periods (before and after the treatment): T = t0 and T = t1. Only one group is treated during only one period. Population DiD E [Y |G = 1, T = t1 ] − E [Y |G = 1, T = t0 ] − (E [Y |G = 0, T = t1 ] − E [Y |G = 0, T = t0 ]) The first difference is the before-after estimand; the second eliminates common time-effects. Blaise Melly Difference-in-differences 4 / 29 Example: Card & Krueger (1994) Suppose you are interested in the effect of minimum wages on employment (a classic and controversial question in labor economics). In a competitive labor market, increases in the minimum wage would move us up a downward-sloping labor demand curve. =⇒ employment should fall. Blaise Melly Difference-in-differences 5 / 29 Example: Card & Krueger (1994) Card & Krueger (1994) analyze the effect of a minimum wage increase in New Jersey using a difference-in-differences methodology. In February 1992, NJ increased the state minimum wage from $4.25 to $5.05. Pennsylvania’s minimum wage stayed at $4.25. They surveyed about 400 fast food stores both in NJ and in PA both before and after the minimum wage increase in NJ. Blaise Melly Difference-in-differences 6 / 29 Distribution of wages Blaise Melly Difference-in-differences 7 / 29 Difference-in-Differences Strategy DiD is a version of fixed effects estimation using aggregate data. We do not need individual panel data; repeated cross-sections are enough. Potential outcomes: Y1ist : employment at restaurant i, state s, time t with a high wmin. Y0ist : employment at restaurant i, state s, time t with a low wmin. We then assume that: E [Y0ist |s, t ] = γs + λt In the absence of a minimum wage change, employment is determined by the sum of a time-invariant state effect γs and a year effect λt that is common across states. Let Dst be a dummy for high-minimum wage. Assuming E [Y1ist − Y0ist |s, t ] = β is the treatment effect, observed employment can be written: Yist = γs + λt + βDst + ε ist Blaise Melly Difference-in-differences 8 / 29 Difference-in-differences strategy (cont.) In New Jersey: Employment in February is: E [Yist |s = NJ, t = F ] = γNJ + λF Employment in November is: E [Yist |s = NJ, t = N ] = γNJ + λN + β The difference between November and February is E [Yist |s = NJ, t = N ] − E [Yist |s = NJ, t = F ] = λN − λF + β In Pennsylvania: Employment in February is: E [Yist |s = PA, t = F ] = γPA + λF Employment in November is: E [Yist |s = PA, t = N ] = γPA + λN The difference between November and February is E [Yist |s = PA, t = N ] − E [Yist |s = PA, t = F ] = λN − λF Blaise Melly Difference-in-differences 9 / 29 Difference-in-differences strategy (cont.) The difference-in-differences strategy compares the change in employment in NJ to the change in PA. The population difference-in-differences are E [Yist |s = NJ, t = N ] − E [Yist |s = NJ, t = F ] − (E [Yist |s = PA, t = N ] − E [Yist |s = PA, t = F ]) = β This is estimated using the sample analog of the population means. Blaise Melly Difference-in-differences 10 / 29 Card and Krueger: results Blaise Melly Difference-in-differences 11 / 29 Card and Krueger: graphical illustration Blaise Melly Difference-in-differences 12 / 29 Regression DiD We can estimate the difference-in-differences parameter in a regression framework. Advantages: It is easy to calculate standard errors. We can control for other variables that may reduce the residual variance (lead to smaller standard errors). It is easy to include multiple periods. We can also study treatments with different treatment intensities (e.g., varying increases in the minimum wage for different states). In the Card and Krueger case, the equivalent regression model is Yist = α + γNJs + λdt + β (NJs · dt ) + ε ist where NJs is an indicator for being from NJ and dt is an indicator for being from November. Blaise Melly Difference-in-differences 13 / 29 Regression DiD: graphical illustration Blaise Melly Difference-in-differences 14 / 29 Regression DiD: graphical illustration Blaise Melly Difference-in-differences 15 / 29 Regression DiD: graphical illustration Blaise Melly Difference-in-differences 16 / 29 Regression DiD: graphical illustration Blaise Melly Difference-in-differences 17 / 29 Key assumption of any DiD strategy: common trends The key assumption for any DiD strategy is that the outcome in the treatment and control group would follow the same time trend in the absence of the treatment. This assumption is never testable, but we can get some idea of its plausibility when several periods are available: check that the evolutions are the same in different groups Note that even if pre-trends are the same, one still has to worry about other policies changing simultaneously. Blaise Melly Difference-in-differences 18 / 29 Regression DiD including leads and lags Including leads in the DiD model is an easy way to analyze pre-trends. Lags can be included to analyze whether the treatment effect changes overtime after treatment; e.g., we might believe that the effects should grow or fade as time passes. The estimated regression is m q Yist = γs + λt + ∑ β−τ Ds,t −τ + ∑ βτ Ds,t +τ + ε ist τ =0 τ =1 where the sums on the right-hand side allow for m lags or post-treatment effects ( β −1 , β −2 ,..., β −m ) , q leads or anticipatory effects ( β 1 , β 2 ,..., β q ). Blaise Melly Difference-in-differences 19 / 29 Study including leads and lags - Autor (2003) Autor (2003) includes both leads and lags in a DD model analyzing the effect of increased employment protection on the firm’s use of temporary help workers. In the US, employers can usually hire and fire workers at will. Some state courts have made some exceptions to this employment-at-will rule and have thus increased employment protection. Different states have passed these exceptions at different points in time. The standard thing to do is to normalize the adoption year to 0. Autor then analyzes the effect of these exceptions on the use of temporary help workers. Blaise Melly Difference-in-differences 20 / 29 Study including leads and lags - Autor (2003) Blaise Melly Difference-in-differences 21 / 29 Study including leads and lags - Autor (2003) The leads are very close to 0. =⇒ no evidence for anticipatory effects (good news for the common trends assumption). The lags show that the effect increases during the first years of the treatment and then remains relatively constant. Blaise Melly Difference-in-differences 22 / 29 Misspecifying dynamic effects If the true treatment effects are heterogeneous with respect to time (either calendar or event time), a model imposing a unique treatment effect is misspecified. The two-way fixed effect estimator estimates a weighted average of the group treatment effects, but the weights can be negative! Therefore, the estimated model must allow for this heterogeneity. See e.g. Goodman-Bacon (2021, “Difference-in-differences with variation in treatment timing”), Callaway and Sant’Anna (2021, “Difference-in-differences with multiple time periods”), Wooldridge (2021, “Two-way fixed effects, the two-way Mundlak regression, and difference-in-differences estimators”). Blaise Melly Difference-in-differences 23 / 29 Standard errors in DiD strategies The variables of interest in many studies only vary at a group level (say state) and outcome variables are often serially correlated. In the Card and Krueger study, for example, it is very likely that employment in each state is not only correlated within the state but also serially correlated. Conventional standard errors often severely understate the standard deviation of the estimators. Solution: cluster at the group level and allow for arbitrary serial dependence. Problem: needs many groups. No simple solution for the case of only two groups. Blaise Melly Difference-in-differences 24 / 29 Meyer, Durbin, Viscusi (1995) in Stata Blaise Melly Difference-in-differences 25 / 29 Meyer, Durbin, Viscusi (1995) in Stata Change in the rate of wage compensation in case of injury: Does it impact on the length of sick leave? Identification strategy: comparison of low-earnings (E1-E2) workers (unaffected) and high-earnings workers (E3+) Blaise Melly Difference-in-differences 26 / 29 Alternative method 1: synthetic control methods In some cases, treatment and potential control groups do not follow parallel trends. The basic idea behind synthetic controls is that a combination of units often provides a better comparison for the unit exposed to the intervention than any single unit alone. Abadie & Gardeazabal (2003) pioneered a synthetic control method when estimating the effects of the terrorist conflict in the Basque Country using other Spanish regions as a comparison group. They assigned positive weights to each of the 16 regions. The weights are chosen so that the synthetic Basque country most closely resembles the actual one before terrorism. More about the synthetic control method. Blaise Melly Difference-in-differences 27 / 29 Alternative method 2: changes-in-changes DiD: the common trend assumption is not invariant to a change in the specification of the outcome (i.e., level vs linear). Athey and Imbens (2006) suggest an alternative model that is invariant to monotone reparametrization of the outcome. In addition, they can identify the treatment effect on the distribution of the outcome variable. Main identifying assumption: ranks do not change over time within groups. More about the changes in changes: section 5.3 in Frölich and Sperlich (2019) Blaise Melly Difference-in-differences 28 / 29 Blaise Melly Difference-in-differences 29 / 29