Prediction of Epidemic & Epidemic Threshold Curve PDF
Document Details
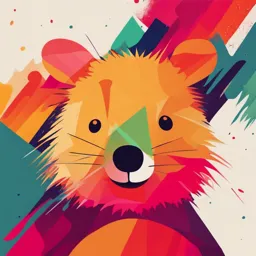
Uploaded by GleefulMagnesium
Dr. Nilanjan Dastidar
Tags
Summary
This document presents a comprehensive overview of epidemic prediction and the epidemic threshold curve. It explores the basic reproduction number (R0), related definitions, objectives of outbreak investigations, and machine-learning techniques for predicting the spread of infectious diseases.
Full Transcript
Prediction of epidemic & Epidemic threshold curve Dr. Nilanjan Dastidar MBBS, DPH, MPH (Chennai), MBA Introduction In the study of infectious disease spread, basic reproduction number (R0) is the average of the expected secondary infection cases caused by a primary infectious individual introduced...
Prediction of epidemic & Epidemic threshold curve Dr. Nilanjan Dastidar MBBS, DPH, MPH (Chennai), MBA Introduction In the study of infectious disease spread, basic reproduction number (R0) is the average of the expected secondary infection cases caused by a primary infectious individual introduced in a fully susceptible host population R0 : strongly correlated to the likelihood and extent of an epidemic R0 : depends on both disease & host population structure [Keeling and Rohani, 2008] Predicting whether a disease will die out or become an epidemic within a population is known as the epidemic threshold; a critical value beyond which an infection becomes an epidemic [Wang et al., 2003] Epidemic threshold (or Alert threshold): The minimum number of cases of a disease that starts an outbreak and requires an urgent response Related Definitions Outbreak: Sudden occurrence of a disease in relatively limited geographic area o Outbreak: usually limited to a small focal area, o Epidemic: covers larger geographical areas having more than one focal point Outbreak Epidemiology: Study of a disease cluster or epidemic in order to control or prevent further spread of the disease in the population Objectives of OB Investigation 1. Primary- to control the spread of disease 2. To determine the causes of disease, its source & mode of transmission 3. To determine who is at risk 4. To determine what exposures predispose to disease 5. To know magnitude of the problem 6. To identify new agent 7. To determine the effectiveness of control measures 8. To identify methods for present & future prevention & control 9. Research & training opportunities 10. Public, Political and legal concerns Trigger events & Warning Signals 1. Clustering of cases/deaths in time/space 2. Unusual increase in cases/deaths 3. Shift in age distribution of cases 4. High vector density 5. Unusual isolate 6. Public, Political and legal concerns Diseases requiring investigations 1. Endemic diseases with epidemic potential: malaria, cholera, measles, hepatitis, meningococcal meningitis etc. 2. Even a single case of diseases for which eradication/elimination goals have been set: polio, guineaworm, yaws 3. Rare but internationally important diseases with high case fatality rates: yellow fever 4. Outbreaks of unknown etiology OB: General lines of action Basic general lines of action during epidemics include – Preparedness and Interventions (investigations) Success in dealing with an epidemic depends largely on state of preparedness achieved in advance of any action It would be an error to consider a hitherto unrecognized endemic situation or a mere seasonal variation in incidence of a disease as an epidemic Confirmation of existence of OB: Epidemic Threshold Curve Are there cases in excess of the baseline rate for that disease & setting? Epidemic Threshold Curve: o To find out excess frequency o The periodic frequency for previous 3 years (at least) plotted on a graph and another graph of Mean + 2SD level superimposed on it - any fluctuations beyond these 2 graphs should be treated as epidemic fluctuations (method of moving averages) Epidemic Threshold Curve: Example 1 Ohio: New cases per day (epidemic curve) as of August 1st, 2021. Epidemic Threshold Curve: Example 2 The influenza positive rate, epidemic and intensity levels of school-aged children (left) and general population (right) in season 2018/2019 in Hubei Province, China. Machine Learning and Prediction of Infectious Diseases Machine Learning: Introduction Machine-learning algorithms can contribute to the control of infectious diseases by helping to both spatially and temporally predict the evolution and spread of infectious diseases Machine-learning algorithms are capable of analyzing large, complex data sets and identifying patterns and trends that may be difficult for humans to detect Makes them well suited for the prediction of infectious diseases, which often involve multiple factors such as population demographics, environmental conditions, and individual behaviours Many studies have applied machine-learning techniques to the prediction of infectious diseases, and the results have been promising Machine Learning: How to use A range of machine-learning algorithms employed Includes: Decision trees Random forests Support vector machines Deep-learning networks These algorithms have been applied to a variety of data sets, including electronic health records, genomic data, and social media posts Results of these studies have shown that machine-learning algorithms can accurately predict the spread and onset of infectious diseases, with performance comparable with or better than traditional statistical methods Machine Learning: Models Logistic Regression average (ARIMA) Random Forest Seasonal ARIMA (SARIMA) Naïve Bayes Ordinary differential equation K-nearest neighbor (KNN) mechanistic (ODE) MLP Generalized machine-learning model (GBM) Lib SVM Recursive neural network (RNN) Decision tree Long short-term memory (LSTM) & AdaBoost Attention-enhanced LSTM (LSTM-ATTN) Bagging Least absolute shrinkage & selection Radial basis function classifier operator (LASSO) Gaussian process formulation Autoregressive integrated moving Machine Learning: Use Use of machine learning in the prediction of infectious diseases is a promising area of research with potential applications in public health, epidemiology, and clinical practice Successfully applied: 1. To forecast the number of cases of a particular disease in a given region on the basis of historical data and current conditions 2. To identify the most likely sources of an outbreak on the basis of the genetic makeup of the pathogen and the patterns of infection 3. To predict the likelihood of an individual contracting an infectious disease on the basis of their personal characteristics and behaviors Machine Learning: Challenges 1. Availability of high-quality, comprehensive data. Infectious disease surveillance systems often collect data on a variety of factors which are often incomplete, biased, or noisy, which can affect the performance of machine-learning models 2. Require large amounts of data that may not be available in the early stages of an outbreak 3. Complexity of the underlying phenomena, such as transmission of infectious diseases, can make it difficult to develop accurate models 4. Some infectious diseases have long incubation periods - data on past outbreaks may not accurately reflect current conditions 5. Chance of bias and overfitting in machine-learning models, which can lead to inaccurate predictions References 1. UNHCR emergency handbook, Disease Surveillance Threshold. 2. O’Dea EB, Park AW, Drake JM. 2018 Estimating the distance to an epidemic threshold. J. R. Soc. Interface 15: 20180034. 3. Yuan Jiang et. al., Applying the Moving Epidemic Method to Establish the Influenza Epidemic Thresholds and Intensity Levels for Age-Specific Groups in Hubei Province, China; Int. J. Environ. Res. Public Health 2022, 19, 1677. 4. Claude Kanyou, Etienne Kouokam, Yves Emvudu. Epidemic threshold : A new spectral and structural approach of prediction. CARI 2022, Oct 2022, Yaoundé, Dschang, Cameroon. hal-03714202v1 5. Keegan Kresge et. al., Rochester Institute of Technology, Analyzing Epidemic Thresholds on Dynamic Network Structures. 6. Omar Enzo Santangelo et. al., Machine Learning and Prediction of Infectious Diseases: A Systematic Review; Mach. Learn. Knowl. Extr. 2023, 5, 175–198. https://doi.org/10.3390/make5010013 7. World Health Organization. Ethics and Governance of Artificial Intelligence for Health: WHO Guidance;World Health Organization: Geneva, Switzerland, 2021; Licence: CC BY-NC-SA 3.0 IGO. 8. Palaniappan, S.; V, R.; David, B.; S, P.N. Prediction of Epidemic Disease Dynamics on the Infection Risk Using Machine Learning Algorithms. SN. Comput. Sci. 2022, 3, 47. 9. Roy, S.; Biswas, P.; Ghosh, P. Spatiotemporal tracing of pandemic spread from infection data. Sci. Rep. 2021, 11, 17689. 10. Ghannam, R.B.; Techtmann, S.M. Machine learning applications in microbial ecology, human microbiome studies, and environmental monitoring. Comput. Struct. Biotechnol. J. 2021, 19, 1092–1107. Thank you