3003PSY Week 10 mini-transcript.pdf
Document Details
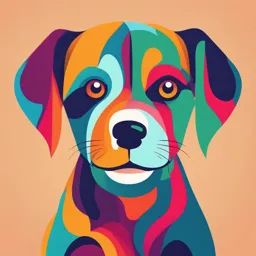
Uploaded by MesmerizedPeridot
Griffith University
Tags
Full Transcript
SPEAKER 1 Welcome back to the Mini Lecter syriza $3. SPEAKER 0 3 ps y I'm Dr Natalie Locks them and in SPEAKER 1 this mini lecture will look at the assumptions and limitations SPEAKER 0 of the Chi Square tests. SPEAKER 1 Just that regression has assumptions, so Disco Square is covered SPEAKER 0...
SPEAKER 1 Welcome back to the Mini Lecter syriza $3. SPEAKER 0 3 ps y I'm Dr Natalie Locks them and in SPEAKER 1 this mini lecture will look at the assumptions and limitations SPEAKER 0 of the Chi Square tests. SPEAKER 1 Just that regression has assumptions, so Disco Square is covered SPEAKER 0 in last week's topics. Non Parametric tests have fewer assumptions and perimeter tests, but SPEAKER 1 there is still some assumptions and limitations to the Chi Square. Unfortunately, no analysis has no limitations. The first assumption of Chi Square is that there's independence of observations. This means that a participant can only fall in one, SPEAKER 0 and in one and only one category they cannot be SPEAKER 1 counted twice as such. SPEAKER 0 You cannot use Pi Square or repeated measure designs. SPEAKER 1 They're special forms, a test that exist to test out SPEAKER 0 such relationships. But these air well outside the scope of freedom. Three PS way. SPEAKER 1 Second assumption is the size of the expected frequencies. SPEAKER 0 As it's all expected, cell frequency should be at least five. If they aren't, you shouldn't use the Chi Square test. SPEAKER 1 This's because small expected frequencies produced few possible values of SPEAKER 0 square, and we upped and we compare the obtain chi SPEAKER 1 square toe, a continuous distribution recall that the chi square SPEAKER 0 distribution is continuous. Note, though, that the greater the degrees of freedom. In other words, the more categories, the more lenient this SPEAKER 1 requirement. In the example, we had a least one cell with less than five expected frequencies, but in that example, we only had 20 participants. Usually you would have far more than this. The third assumption is the inclusions of none occurrences. This means that computations must be based on participants in the sample. Let's look at the example. Let's say you run a mirror suffering conditions study to test gender differences in this important developmental milestones. If you haven't done developmental psychology or forgot, this is SPEAKER 0 feline version of that test. This test cheques to see if a child were in this case, the kitty can distinguish themselves in the mirror. SPEAKER 1 As you can see, this poor little kitty found the test. Admit here that my seven month I'll pop still my box of himself in the mirror as well, and so SPEAKER 0 it is also clearly not passed this important milestone. SPEAKER 1 So in our study, we run a Mirror self Recognition Study with 42 year old Children, 20 boys and 20 girls 17 girls passed the test, but only 11 boys. Is there a significant gender difference here? According to our Car Square tests, there's no difference between the boys and girls and passing this milestone. SPEAKER 0 But we've only looked at the Children passed the test it did not include those have failed the test. SPEAKER 1 This is a violation of the inclusion of none occurrences. When you do Chi square, you must include all the data that you collected and clean. The Children did not pass the test. Now we have included them. We find we do have a significant result. We can conclude that passing the test was dependent upon gender. So here are three assumptions won independence of observations to expect a self frequencies of at least five and three inclusion of 90 clearances. They're also limitations to Chi Square one. SPEAKER 0 That's not really limitation per se, but it is something to be considered. Is that the close where is restricted to less than 22 or less variables to test like to design, she SPEAKER 1 would need to use a Multivariate generalisation off these approaches SPEAKER 0 that largely based on local Indian models as they sound, SPEAKER 1 they're actually quite complex and a best covered in their own course. We don't cover them. SPEAKER 0 In this particular course, Justus multiple regression is the simple regression log. Linear models are chi square sample size plays. A very strong role in significant testing in Chi Square has demonstrated here. SPEAKER 1 If he doubled the number of participants in your sample, you obtain close where value also doubles. And this example. SPEAKER 0 I've put the output from the two EKO Square test in the prison e letter on the left and ran SPEAKER 1 the same analysis with twice as many participants I used SPEAKER 0 the same portion fails and passes across the two attendants. Status is, and this is on the right hand side, SPEAKER 1 assuming in you can see that the obtained quite square for the original data set was 7.13 This is slightly different to the value we calculated by hand in the mini lecture. SPEAKER 0 But this is simple. To get around in the data set. SPEAKER 1 With twice as many participants obtain, value is exactly double SPEAKER 0 here. Both values exceeded the critical cut off and was significant SPEAKER 1 in other studies, though the sample sizes and made it influence on whether or not you find a significant result. SPEAKER 0 This is the course degrees a friend. Freedom is depending on the number of categories. SPEAKER 1 In this two by two case, there was one degree of freedom and so will not change. I often note, I often note this one. Using more advanced analysis, they used the Chi Square distribution to finish off the non parametric part of this module. SPEAKER 0 Let's look at the effects size of Chi Square. SPEAKER 1 One thing to remember is that a significant case where it does not indicate the strength of a relationship. And as I just demonstrate on the previous light, if SPEAKER 0 you have a sample sizes large enough, often very small effects will be detected as significant. SPEAKER 1 Luckily, we can convert co square toe, a measure of association that tells you how big the effect is. SPEAKER 0 This's fi. We use Fei as thie effect for the two by two Chi Square we interpret fired, just like Pearsons are SPEAKER 1 fire simply the correlation between two dichotomous variables. SPEAKER 0 If Christ were significant, then so will Chi will fight. SPEAKER 1 We can also interpret fly in the same terms as Cohen's covert covet conventions. Although fires a measure of association between two variables rather SPEAKER 0 than a sex eyes per se. SPEAKER 1 These concepts are related. SPEAKER 0 So, for example, if fires 0.5, you could say there SPEAKER 1 was a moderate level of association between the two variables SPEAKER 0 or if I was 0.65 you could say that there was a moderate to high level of association between the two variables. Here's the syntax with the request. If I added, we can also use Fei Square as SPEAKER 1 an index of variance Explained have placed inverted commas around the word variants as there's no actual variance at such SPEAKER 0 in categories. It's easy to think in terms of various Innogy ari SPEAKER 1 in Algeria. Ricky Sample. We obtained a five value of 50.592 If we square SPEAKER 0 five, we get 50.35 This is interpreted as the relationship between class attendance and pass fail has bean moderate and SPEAKER 1 class attendance as explaining 35% of the variance in passing or failing the exam here. I've also performed a correlation between attendances and economists, variable SPEAKER 0 and stats exam performance as a percentage in other words, a point by serial correlation. SPEAKER 1 Even though this is effectively addressing the same question, the effects sizes larger and the associated variance explain accounts from or variance. SPEAKER 0 This is because, as discussed in the non Parametric mini lecture, Parametric tests make Pearson's correlation and by extension, the point by serial association are more powerful tests. While the Chi Square is a very useful test for truly categorical data, Nusa Parametric tests went all the purpose assumptions admit appropriate assumptions are met opposite final point fires used for the two by two Table chi square. The chi squares of greater than two columns and or SPEAKER 1 coat or rose crane misfires use and interpreted the same SPEAKER 0 as five to summarise. SPEAKER 1 Chrysler has several assumptions and limitations. SPEAKER 0 These are independence of observation. Expected self frequencies of at least five, inclusion of non SPEAKER 1 occurrences and coast where can only be used with one SPEAKER 0 or two variables as degrees of freedom are based on SPEAKER 1 the number of cells. SPEAKER 0 Sample sizes have a strong effect on the obtain close square and by extension, significance. SPEAKER 1 Fire is the measure association between categorical variables in a SPEAKER 0 tube to Chi Square, Squaring five provides affair. It's accounted premise. Fire is used for case with with greater than two columns. All rose