3003PSY Regression Assumptions PDF
Document Details
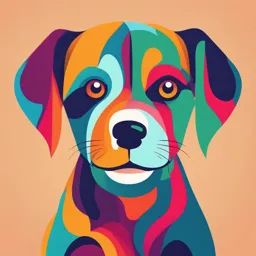
Uploaded by MesmerizedPeridot
Griffith University
Tags
Summary
This transcript discusses the assumptions of regression models, focusing on the residuals. It explains how significance testing, and considerations for linearity and independence of the errors, are critical in regression analysis.
Full Transcript
SPEAKER 0 Welcome back to the mini Lecter syriza 303 ps y on Dr Netley locks and in this mini lecture will look at the underlying assumptions of regression just as you had various assumptions that need to be met when using a T test run and over in wonderful, oh three or 2000 years immigration, we al...
SPEAKER 0 Welcome back to the mini Lecter syriza 303 ps y on Dr Netley locks and in this mini lecture will look at the underlying assumptions of regression just as you had various assumptions that need to be met when using a T test run and over in wonderful, oh three or 2000 years immigration, we also need to ensure that our data made a range of assumptions. SPEAKER 1 This is because their test of significance for overall model and the be weights are based on the normal distribution SPEAKER 0 records from the minute electrons. Significance testing is important to note that most the regression assumptions are made about the residuals, rather the data per se. SPEAKER 1 However, while looking at a residual to tell us if SPEAKER 0 there's any potential issues, we cannot do anything directly to SPEAKER 1 residuals as a residual is simply what is left over. Once we would befit a regression line. SPEAKER 0 We also see that we have a linear relationship between our predictors and the outcome, or criterion variable. We can still fit a regression line in non Ilya relationships, and not really linear relationship, for instance, could be SPEAKER 1 a curvilinear relationship. SPEAKER 0 But the output from S P SS will actually be. SPEAKER 1 Many lists recall that the regression equation is based on SPEAKER 0 a straight line. SPEAKER 1 So what are the regression assumptions that we need to SPEAKER 0 meet? SPEAKER 1 First, we sent the residuals and normal distributed or centred around zero second. SPEAKER 0 We assume I. SPEAKER 1 Miska, Justice Lee, that there is a constant variance of residual across all levels of predicted schools, which is the normality of residuals and high risk elasticity using plots from our regression of syntax. SPEAKER 0 Third, that there's independence of errors and other words that SPEAKER 1 the residuals aren't correlated with y and forth that the relationship between the predictors and the criteria is linear. SPEAKER 0 In other words, force along a straight line. Linearity is checked by looking at scatter plots from earlier SPEAKER 1 in the course. You may wonder why we go through all this trouble We do cause regression is very powerful analytic approach to testing quite interesting and complex questions. SPEAKER 0 I think that when you covered and over, you're pretty much limited in the number of independent variables that you SPEAKER 1 could actually use, usually at maximum three. In regression, you can have far more predictive variables in SPEAKER 0 an over. You could also any have categorical lives but in regression, you could have categorical and continuous variables. These benefits mean that we have to pay the cost SPEAKER 1 of insuring that I've gotta meet these assumptions. Recall that we partition people's actual scores into the part the weak and explain use the regression equation, and what SPEAKER 0 is left over is a residual a person school. SPEAKER 1 Why is that? Predicted school plus residuals also recall that everyone the data set has an actual score on the wide variable predicted score on the wide variable and a residual school. The residual school could be positive or negative, depending on whether the regression equation over or underestimates the person school. If the regression equation has underestimated the actual school, the residual B positive it. Instead, the true score is overestimated. The residual will be negative. It is the residual school. There's plotted by the various residual pots in the SS regression syntax that is used in the tutorial. SPEAKER 0 Let's return to our G R E key data to SPEAKER 1 obtain the predicted scores around CBss regression and asked for the predicted scores and the residuals. The predictor schools would lie on the line of best fit. The residual is the difference between the actual score and the predicted school. We looked at this previously, so hopefully this is old. News here have highlighted the first four participants in the data set, as you see here the residual so the first two participants are negative. There. Predicted scores overestimated their actual stats exam performance, the residuals SPEAKER 0 for the next two participants a positive. SPEAKER 1 And so they predicted scores underestimated this. That's exam performance, it predicted. Scores of independent why some scores are over or underestimated, in other words, have positive or negative residuals. Then the residuals should be fairly evenly spread across positive and negative values and across the range of predicted values. As such, the assumptions that we make about residuals rather than actual score that actual values. Next, the predicted and residual columns I've asked for the predicted and residual scores in standardised form record from the SPEAKER 0 mini lecture that standard I scored or simply said schools. SPEAKER 1 So said Predict is predicted. Score in sets four units and said resent is a residual score and sexual units. While the assumptions air about the residuals we used to standardise scores and regress the standards residuals on the standardised predicted school. We don't actually need to do this separately as we can simply use syntax and add this to our multiple SPEAKER 0 regression syntax. SPEAKER 1 It is the standardised predict the scores and residuals. They're going to the plot that you will be looking SPEAKER 0 at in the tutorials. SPEAKER 1 So what does white residuals matter? This is because residuals will take on distinctive patterns. SPEAKER 0 If there's something it's systematic and miss with the data. If there's only true school and ran an error in our data and we haven't omitted any particularly important predictors in our outcome, we would expect nice and neat knowledge SPEAKER 1 to residuals that are centred around zero. SPEAKER 0 Anything else means that there might be a problem that is, that something is affecting the Erin people scores apart from the predictors. SPEAKER 1 So first we need to cheque that the residuals are non distributed with a mean of zero. Here we used to standardise residual scores. SPEAKER 0 Although we only have 20 observations. The stand those residuals look pretty well centred around zero approximately half below 0.5 above zero. SPEAKER 1 The distribution also looks pretty normal, So I'd say that SPEAKER 0 that is such has been met. SPEAKER 1 This is created by your regression syntax by the way. SPEAKER 0 Okay. We also need to cheque your assumption off home, ask elasticity, and statistically refers to having a constant distribution of SPEAKER 1 residuals across the range of predicted schools. SPEAKER 0 On why, when a residual to correlated with predicted scores? SPEAKER 1 And we have random error in other words, an error. It's independent off the association with criteria and that we SPEAKER 0 haven't left out any important predictors. We should have a rectangular shape and can conclude that we have met the assumption of risk elasticity. SPEAKER 1 Well, we haven't discussed this in great debt. We want to try to include all the important predictors. Otherwise, well, we have what we call miss specified our SPEAKER 0 model. This is a concept that you will come across a more complex analysis for the time being. Let's just accept that we have specified and model correctly, SPEAKER 1 in other words, that we've included the important predictors. SPEAKER 0 When I got to meet the assumption of homos, get ecstasy. SPEAKER 1 The residual should look evenly scattered above and below zero, and the range of residual should around zero should be fairly narrow. SPEAKER 0 Effectively, you're looking at a rectangular shape to the data SPEAKER 1 points off your residuals. SPEAKER 0 If, however, a residuals look more like a funnel of fan than that suggest that we've not met the assumption of home scholastic city agency here. SPEAKER 1 The distribution of residuals across the range of predicted scores of why is not even such a pattern suggests that there is worst prediction at low predictive values of life that, at high predictive value, is away. Notice that the notice the greater variability of residuals at low levels are predicted. Schools on wife relative to the lower variability. Hi predicted schools in this diagram. Essentially, we just eyeball the spread of scores and look to see if we have more of a rectangular or SPEAKER 0 more of a rectangle. Or do we have more of a family or fan? There's actually no test of significance here if the spread looks more like a fan and we probably have skewed data in one or more of our predictors, although they SPEAKER 1 could also be other factors at play and the next SPEAKER 0 topic, we look at issues such askew. So how did the residuals and are G R E Q. Data look in terms of high risk elasticity. Again, we only have 20 observations, so it's hard to make out that rectangle. SPEAKER 1 The shape looks reasonably OK, but there may be issues with some evidence of greatest Fred A. Sports scores at minus one standard deviation off the predicted value. Why, in the data you will have in your assignment SPEAKER 0 dataset and in your own state, you'll have many more SPEAKER 1 observations, and a pattern will be clear. SPEAKER 0 The residual Parcell may also indicate nonlinear associations between the predictors and the criterion. Linearity is assessed by looking at the scatter plots. If you find nonlinear associations, then you may need to consider a different analytic approach. The following topic will deal with the situation in which inspection of residual plot suggests one on war regression assumptions has been violated. Note that in the assignment, even if you conclude that SPEAKER 1 residuals look OK, you will still need to run the SPEAKER 0 following procedures in order to answer some questions. So she's abusing a different, a different procedure entirely. SPEAKER 1 We have two courses of action open to us in SPEAKER 0 order to fix the data in order to meet the assumptions. First, we may find one or more outlined data points on one of more variables that is unduly influencing the regression analysis and potentially leading to potentially erroneous conclusions. Second, one or more predictors made Devi agree greatly from normality in terms of ski, eunice and ketosis. Again, this may lead to era. In this case, we generally apply one or more transformations to the data. We do this intern not concurrently, by the way. We then replaced the actual variable with the transport transform one. In the regression analysis, we'll look at some decision rules in the next topic. We use transformations because these because certain transformations may help alleviate SK Eunice, although your notice in the next topic this can be a little bit hit and miss that loss and transformations are covered in the next top again in the tutorials. There is very detailed information in the tutorial worksheet, and any company screen cast and additional worksheets to summarise Regression SPEAKER 1 is a powerful analytic approach that requires consideration of underlying SPEAKER 0 assumptions. SPEAKER 1 Assumptions are made, we forgot. With regard to the residuals, we assume normality of residuals, homos, Kostas city, independence of residuals and linearity. SPEAKER 0 These assumptions are tested by requesting additional information in their syntax. We'll cover this in the tutorials. Violations of assumptions may reflect skew out lies and or non linear associations