3003PSY Mini-lecture 2 Point biserial PDF
Document Details
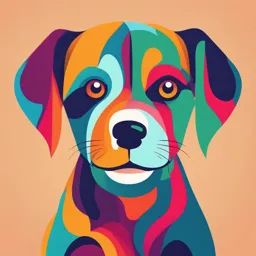
Uploaded by MesmerizedPeridot
Griffith University
Tags
Summary
This mini-lecture covers the point biserial correlation, a special case of correlation with one continuous and one dichotomous variable, in the context of survey design and analysis within psychology. It explores how this correlation is interpreted, its relationship to Pearson's correlation, and the equivalent t-test significance. The lecture also touches on using regression equations.
Full Transcript
3003PSY Survey Design and Analysis in Psychology WEEK 9: MINI-LECTURE 2 POINT BISERIAL CORRELATION POINTBISERIAL CORRELATION uPearson’s r is appropriate for describing linear relationships between two continuous variables uifone variable is genuinely dichotomous, score one level of that variab...
3003PSY Survey Design and Analysis in Psychology WEEK 9: MINI-LECTURE 2 POINT BISERIAL CORRELATION POINTBISERIAL CORRELATION uPearson’s r is appropriate for describing linear relationships between two continuous variables uifone variable is genuinely dichotomous, score one level of that variable as 0 and the other as 1 (or 1 and 2, or any two numbers) ucompute correlation using Pearson’s r formula ureferred to as the point-biserial correlation (rpb) INTERPRETATION OF POINTBISERIAL CORRELATION uabsolute value of rpb reflects strength of the relationship ubut the sign of the correlation depend on scoring (0 or 1; or 1 and 2) ur2pb interpreted the same way as r2 utest for significance in same way as for Pearson’s r REGRESSION var = Attendance Stats_Exam /dep = Stats_Exam /enter = Attendance. CORRELATIONS /VARIABLES=Stats_Exam Attendance /PRINT=TWOTAIL NOSIG /MISSING=PAIRWISE. rpb and t Alternatively, we could examine the relationship between a dichotomous and a continuous variable using an independent groups t-test Result of test of significance of rpb and t-test will be identical RECALL uPerson scoring 0 on attendance (i.e., did not attend every lecture) would have a predicted statistics exam score of 61.182 (61.182+ 14.37*0) uForevery scale point that a student scores on attendance (i.e., are a student who attended every lecture), their predicted score on statistics exam would increase by 14.37 uSo a student who attended every lecture has a predicted score of (61.182+14.37*1) = 75.552 uPerson scoring 0 on attendance (i.e., did not attend every lecture) would have a predicted statistics exam score of 61.182 (61.182+ 14.37*0) uForevery scale point that a student scores on attendance (i.e., are a student who attended every lecture), their predicted score on statistics exam would increase by 14.37 uSo a student who attended every lecture has a predicted score of (61.182+14.37*1) = 75.552 SUMMARY u A point biserial is a special case of a correlation with one continuous variable and one dichotomous variable uThe point biserial is interpreted the same a Pearson's correlation uThe t value and significance of a dichotomous predictor in the regression output is the same as if the analysis is tested as a t test. uYou can use the regression equation to calculate the mean of the two groups in the dichotomous variable just like in a t test