3 Module 3 Statistics Refresher Handouts.pdf
Document Details
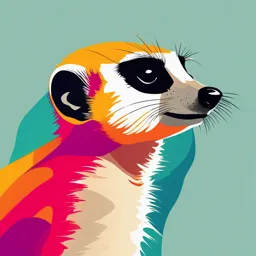
Uploaded by EyeCatchingOakland
Tags
Full Transcript
1/6/24 Module 3: A Statistics Refresher Bill Chislev Jeff J. Cabrera, M.Ed., RPm 1 Why is it important to study statistics in Assessment? Knowledge in psychologic...
1/6/24 Module 3: A Statistics Refresher Bill Chislev Jeff J. Cabrera, M.Ed., RPm 1 Why is it important to study statistics in Assessment? Knowledge in psychological statistics also help us in clinical decision making when we interpret quantitative assessment tools. 3-2 2 1 1/6/24 Issues of Measurement While we have definite ways to measure physical variables such as height, weight, length, volume, etc. ,measuring psychology related variables are challenging. Variable being Unit of Measurement Tools used to Measure Occupation Measured Engineer Distance Meters Meter stick Nurse Temperature Celsius Thermometer Dietician Weight Kilograms Weighing scale Psychometrician Happiness High/Low (Level) Test/Questionnaire 3-3 3 Issues of Measurement 3-4 4 2 1/6/24 Scales of Measurement Continuous scales – theoretically possible to divide any of the values of the scale. Typically having a wide range of possible values (e.g., height or a depression scale). Discrete scales – categorical values (e.g. male or female) Error – the collective influence of all the factors on a test score beyond those specifically measured by the test 3-5 5 Scales of Measurement Nominal Scales - involve classification or categorization based on one or more distinguishing characteristics; all things measured must be placed into mutually exclusive and exhaustive categories (e.g., apples and oranges, DSM-5 diagnoses, etc.). Ordinal Scales – Involve classifications, like nominal scales but also allow rank ordering (e.g. Olympic medalists). 3-6 6 3 1/6/24 Scales of Measurement (cont’d.) Interval Scales - contain equal intervals between numbers. Each unit on the scale is exactly equal to any other unit on the scale (e.g., IQ scores and most other psychological measures). Ratio Scales – Interval scales with a true zero point (e.g., Measures of length; periods of time, score in an exam). Psychological Measurement – Most psychological measures are truly ordinal but are treated as interval measures for statistical purposes. 3-7 7 Each of these four scale has distinct properties: 1. Magnitude- is the property of “moreness.” 2. Equal Interval- the difference between two points at any place on the scale has the same meaning as the difference between two other points 3. Absolute Zero- nothing of the property being measured exists 3-8 8 4 1/6/24 Scale Magnitude Equal Interval Absolute Zero Nominal No No No Ordinal Yes No No Interval Yes Yes No Ratio Yes Yes Yes 3-9 9 Describing Data Distributions - a set of test scores arrayed for recording or study. Raw Score - a straightforward, unmodified accounting of performance that is usually numerical. Frequency Distribution - all scores are listed alongside the number of times each score occurred 3-10 10 5 1/6/24 Describing Data Frequency distributions may be in tabular form as in the example above. It is a simple frequency distribution (scores have not been grouped). 3-11 11 Describing Data Grouped frequency distributions have class intervals rather than actual test scores 3-12 12 6 1/6/24 Describing Data A histogram is a graph with vertical lines drawn at the true limits of each test score (or class interval), forming a series of contiguous rectangles 3-13 13 Describing Data Bar graph - numbers indicative of frequency appear on the Y -axis, and reference to some categorization (e.g., yes/ no/ maybe, male/female) appears on the X -axis. 3-14 14 7 1/6/24 Describing Data frequency polygon - test scores or class intervals (as indicated on the X -axis) meet frequencies (as indicated on the Y - axis). 3-15 15 Types of Distributions 3-16 16 8 1/6/24 Measures of Central Tendency Central tendency - a statistic that indicates the average or midmost score between the extreme scores in a distribution. Mean - Sum of the observations (or test scores), in this case divided by the number of observations. Median – The middle score in a distribution. Particularly useful when there are outliers, or extreme scores in a distribution. Mode – The most frequently occurring score in a distribution. When two scores occur with the highest frequency a distribution is said to be bimodal. 3-17 17 Measures of Variability Variability is an indication of the degree to which scores are scattered or dispersed in a distribution. Distributions A and B have the same mean score, but Distribution A has greater variability in scores (scores are more spread out). 3-18 18 9 1/6/24 Measures of Variability Measures of variability are statistics that describe the amount of variation in a distribution. Range - The distance covered by the scores in a distribution, from smallest score to the largest score. Range = Xmax – Xmin 3-19 19 Measures of Variability Which of the following sets of scores has the greatest variability? A. 2,3,7,12 B. 13,15,16,17 C. 42,44,45,46 3-20 20 10 1/6/24 3-22 22 The three distributions of scores that appear very different have the same mean. Therefore, it is important to consider other characteristics of the distribution of scores beside the mean. The difference between the three sets lies in variability. 3-23 23 11 1/6/24 Measures of Variability Variance - the arithmetic mean of the squares of the differences between the scores in a distribution and their mean Standard deviation –It is the square root of the variance. Typical distance of scores from the mean. SD is the most used and the most important measure of variability. SD uses the mean of the distribution as a reference point and measures variability by considering the distance between each scores and the mean. 3-24 24 Measures of Variability Skewness - the nature and extent to which symmetry is absent in a distribution. Positive skew - relatively few of the scores fall at the high end of the distribution. Negative skew – relatively few of the scores fall at the low end of the distribution. 3-25 25 12 1/6/24 Measures of Variability Kurtosis – the steepness of a distribution in its center. Platykurtic – relatively flat. Leptokurtic – relatively peaked. Mesokurtic – somewhere in the middle. 3-26 26 The Normal Curve The normal curve is a bell-shaped, smooth, mathematically defined curve that is highest at its center. Perfectly symmetrical. Area Under the Normal Curve The normal curve can be conveniently divided into areas defined by units of standard deviations. 3-27 27 13 1/6/24 Standard Scores A standard score is a raw score that has been converted from one scale to another scale, where the latter scale has some arbitrarily set mean and standard deviation. Z-score - conversion of a raw score into a number indicating how many standard deviation units the raw score is below or above the mean of the distribution. (Mean is 0, SD is 1) T scores - can be called a fifty plus or minus ten scale; that is, a scale with a mean set at 50 and a standard deviation set at 10 Stanine - a standard score with a mean of 5 and a standard deviation of approximately 2. Divided into nine units. Normalizing a distribution - involves “stretching” the skewed curve into the shape of a normal curve and creating a corresponding scale of standard scores 3-28 28 Standard Scores 3-29 29 14 1/6/24 Exercise 3-30 30 3-31 31 15 1/6/24 Percentile Rank Percentile ranks replace simple ranks when we want to adjust for the number of scores in a group. A percentile rank answers the question, “What percent of the scores fall below a particular score (Xi)?” 3-33 33 3-34 34 16 1/6/24 Sample Question About how many cases fall between -2SD AND +2SD in the normal curve? A. 34% B. 68% C. 95% D. 99% 3-35 35 Sample Question For a population with µ= 80 and σ= 10, what is the z-score corresponding to X= 65? A. +0.25 B. -0.50 C. +1.00 D. -1.50 3-36 36 17 1/6/24 Sample Question Bill got a test result equivalent to percentile rank of 75 in ABC- IQ test. He also took XYZ-IQ test and got a deviation IQ of 115. What does the results mean? A. He performed well in both tests. B. He performed better in XYZ IQ test. C. He performed better in ABC-IQ test. D. He is a highly intelligent individual. 3-37 37 3-38 38 18 1/6/24 3-39 39 Correlation and Inference Cohen and Swerdlik (2018), maintained that central to psychological testing and assessment are inferences (deduced conclusions) about how some things (such as traits, abilities, or interests) are related to other things (such as behavior). Inferences are formulated by taking the information from your data (your sample) and using it to draw conclusions about the group you’re interested in (your population) (Statistics for Dummies, 2014). 3-40 40 19 1/6/24 Correlation and Inference A coefficient of correlation (or correlation coefficient) is a number that provides us with an index of the strength of the relationship between two things. Correlation coefficients vary in magnitude between -1 and +1. A correlation of 0 indicates no relationship between two variables. Positive correlations indicate that as one variable increases or decreases, the other variable follows suit. Negative correlations indicate that as one variable increases the other decreases. Correlation between variables does not imply causation but it does aid in prediction. 3-41 41 3-42 42 20 1/6/24 1. Strength of Relationship. The Pearson correlation coefficient (r) can range from 0.00 to +1.00 and 0.00 to -1.00. A correlation of 0.00 tells us that the two variables are not related at all. The closer a correlation is to 1.00, either +1.00 or -1.00, the stronger is the relationship. 2. Direction of Relationship Positive Correlation Coefficient (+) suggest that there is a positive linear relationship— high scores on one variable are associated with high scores on the second variable. A negative (-) linear relationship is indicated by a minus sign—high scores on one variable are associated with low scores on the second variable.. 3-43 43 Correlation and Inference Pearson r: A method of computing correlation when both variables are linearly related and continuous. Once a correlation coefficient is obtained, it needs to be checked for statistical significance (typically a probability level below.05). By squaring r, one is able to obtain a coefficient of determination, or the variance that the variables share with one another. Ex. r =.9, the r^2 would be equal to.81. Spearman Rho: A method for computing correlation, used primarily when sample sizes are small, or the variables are ordinal in nature. 3-44 44 21 1/6/24 Correlation and Inference The correlation coefficient (r) is a ratio between the covariance (variance shared by the two variables) and a measure of the separate variances (Dancey & Radey, 2017). For example, you correlate the number of hours spent on playing Mobile Legends ® online and the students’ grades. You have computed a correlation coefficient of r= -0.40. Hours r= -0.40, moderate negative correlation Playing GWA r^2= 16% ML 3-45 45 Correlation and Inference By squaring the correlation coefficient (r^2), you know how much variance, in percentage terms, the two variables share. Thus, -0.40 = 0.16 or 16%. So 16% of the variance has been accounted for by a correlation of -0.4. A Venn diagram will make this clearer. If two variables were perfectly correlated, they would not be independent at all. In our example, the shared variance between the number of hours playing mobile legends and grade is -0.4 or 16%. If 16% of the variance is shared by the two variables, then the remaining 84% is not shared (unique). r= -0.40, moderate negative correlation 42% 16% 42% r^2= 16% 3-46 46 22 1/6/24 Correlation and Inference Scatterplot – Involves simply plotting one variable on the X (horizontal) axis and the other on the Y (vertical) axis Scatterplots of no correlation (left) and moderate correlation (right) 3-47 47 Correlation and Inference Scatterplots of strong correlations feature points tightly clustered together in a diagonal line. For positive correlations the line goes from bottom left to top right. 3-48 48 23 1/6/24 Correlation and Inference Strong negative correlations form a tightly clustered diagonal line from top left to bottom right. 3-49 49 Correlation and Inference Outlier – an extremely atypical point (case), lying relatively far away from the other points in a scatterplot 3-50 50 24 1/6/24 Correlation and Inference Restriction of range leads to weaker correlations 3-51 51 Meta-Analysis Meta-analysis allows researchers to look at the relationship between variables across many separate studies. Meta-analysis- a family of techniques to statistically combine information across studies to produce single estimates of the data under study. The estimates are in the form of effect size, which is often expressed as a correlation coefficient. 3-52 52 25