Applying AI: Value Assessment of AI Products and Applications PDF
Document Details
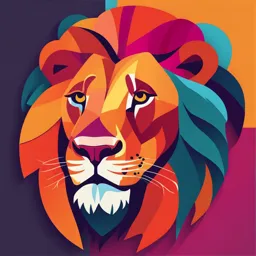
Uploaded by DelightedPolonium
Stanford University
Tags
Related
- 5-big-myths-of-ai-and-machine-learning-debunked.pdf
- AI in Business Unit 1 Lecture PDF
- Applied Artificial Intelligence Handbook for Business Leaders PDF
- Artificial Intelligence for Business MIS 272 Lecture Notes PDF
- Lecture 8: Managing Knowledge and Artificial Intelligence PDF
- AI Application in Business PDF
Summary
This document provides a systematic approach to quantifying the return on AI (ROAI) initiatives. It discusses the value assessment of AI products and applications, covering aspects like return drivers, investment expenditures, and prediction performance implications.
Full Transcript
Applying AI: Value Assessment of AI Products and Applications Value Assessment of AI Products and Applications: A Systematic Approach to Quantifying the ‘Return on AI (ROAI)’ Applying AI 4 Applying AI Introduction Companies of all sizes across various industries and geographies, as well as...
Applying AI: Value Assessment of AI Products and Applications Value Assessment of AI Products and Applications: A Systematic Approach to Quantifying the ‘Return on AI (ROAI)’ Applying AI 4 Applying AI Introduction Companies of all sizes across various industries and geographies, as well as members of parliaments and ministries, academia, regulatory agencies, and the media concur: As a general purpose technology and driving force behind the Fourth Industrial Revolution, artificial intelligence (AI), especially the subfield of machine learning (ML), holds tremendous value potential for businesses, the economy, and society. We gladly agree. At the same time, however, we acknowledge that quantifying and measuring the actual value contribution of AI initiatives presents a major challenge for companies, both for those that are gaining their first experience with AI and for the ones aspiring to become AI-first industry leaders: Besides the interweaving of data and learning algorithms – combined with the inherent result uncertainty of exploratory approaches – many of AI’s benefits are qualitative in nature, underpin a broader business initiative or series of projects, and have payouts that are uneven and increase non-linearly. An additional challenge lies in finding the right balance: When selecting the most promising use cases, decision-making bodies must consider that overemphasizing return expectations in early stages (i.e., at the proof of concept (PoC) level) hinders innovation and decelerates the development of viable products and/or applications, whereas not actively measuring value contribution entails the risk of focusing on inferior use cases or ‘doing AI for the sake of AI’. (Note that in this article, we employ the term ‘use cases’ to refer to projects in an ideation stage, whereas ‘products’ or ‘applications’ refer to use cases that have been brought to life and implemented already.) Against this backdrop, the appliedAI Initiative, its industry network, and its technology partners have teamed up to create a framework for the thorough value assessment of AI applications and a stringent ‘Return on AI (ROAI)’ calculation – all in a comprehensive, hands-on valuation tool accompanied by this publication. These complementary assets will benefit companies along their journey to AI maturity. From securing initial development resources to the cost-benefit analysis of stand-alone AI applications (especially when competing for a portion of a firm’s general technology or innovation budget) and subsequent AI portfolio management, this framework establishes a common basis for discussion among internal and external stakeholders regarding value-based AI implementation and scaling. Ultimately, using ROAI as a central evaluation metric in use case prioritization ensures an efficient utilization of limited resources and maximum return on the corporation’s AI portfolio. The organization of the publication and the tool are in sync. Both resources first evaluate return and investment components of individual AI products and applications (with the tool allowing for a detailed cataloging of use cases) before presenting a strategic perspective on early, short-term value capture and the foundations for long-term success and scaling. In the latter section, the whitepaper places additional emphasis on equipping readers with the criteria, process framework, and tools for the steering of AI application portfolios. 5 Applying AI Value assessment of AI products & applications: Return and investment Following the ROAI calculation logic, the subsequent section is divided as follows: Starting with the evaluation of return drivers, we then move on to the quantification of investment expenditures. We conclude by integrating both components under the consideration of prediction performance implications. After completing the initial, multi-stakeholder AI use case ideation and prioritization (please see appliedAI’s “How to Find and Prioritize AI Use Cases” publication for a detailed step-by-step guide), pre-selected pipeline candidates must be assessed in greater detail, including estimations of both the expected value as well as the expected investment costs. For the value assessment, one should not only clearly describe the issue to be addressed and the distinct opportunity arising from the use of AI (vs., e.g., ‘traditional’ solutions and digital approaches) but also define the time horizon and discount rate on which the ROAI evaluation is based. Regarding the investment expenditures, one has to differentiate between labor and infrastructure/toolstack/license costs, as well as additional expenses, e.g., for development partners or consultancies. Given that personnel costs tend to account for 70-80% of the total application development cost (these empirical values being, of course, subject to model complexity, infrastructure requirements, etc.), it is important to account for the required interdisciplinary roles across the application life cycle (i.e., ML engineers, data scientists, software engineers, and AI strategists/business analysts) early on. These values, together with estimates of the development and implementation time, will later be used to calculate the investment and operation/maintenance cost. 6 Ultimately, both dimensions are inextricably linked by the anticipated performance. AI product managers must estimate the minimum required model performance needed to reach the value threshold while accounting for the fact that increasing performance may disproportionately increase costs. Due to their probabilistic nature, ML systems will not achieve 100% accuracy, and timescales/costs for model development typically increase non-linearly with desired performance. Valuation of AI applications’ return AI is a versatile tool that can generate a rich set of benefits in regards to the financial, strategic, and operational outcomes that a corporation seeks to achieve (sometimes, in relation to their direct calculability, referred to as ‘hard’ and ‘soft’ benefits). Given this multifaceted nature of AI implementation, when calculating use case returns, decision makers are well advised to differentiate between internal and external value generation. When starting out, companies tend to use AI to automate internal processes, boost productivity, and improve staff and customer engagement. However, performance goals typically shift from efficiencies to strategic gains as businesses mature into AI leaders: More advanced enterprises report increasing benefits from faster revenue growth, greater market share, new business models, and accelerated time to market. Although AI applications and products may, in practice, unite both of these elements, it has Applying AI proven remarkably helpful to fundamentally differentiate between them across calculation stages, i.e., from the initial, ad hoc value estimation to a detailed post-implementation analysis. While AI applications’ main value drivers are naturally dependent on the industry and use case class, we’ve identified core levers to facilitate a thorough, transparent calculation. Internal value generation drivers Internal value is generated mostly through an optimization of core business processes and operational management. This is achieved through a reduction of manual/cognitive effort (automation of repetitive tasks), improved resource consumption (e.g., energy savings or quality optimization and consequent scrap reduction), increased speed of operations or time efficiency (e.g., increased Overall Operating Efficiency or Schedule Performance Index), as well as data-driven decision making based on more fine-grained information or more accurate risk assessment. The impact quantification chains shown in the following figure provide the calculation logic for five main return drivers as well as core questions and relevant examples. External value generation drivers For external product- or service-related AI applications, the quantification of benefits tends to be more challenging, as historical return data are often lacking and established mechanisms for crucial Key Performance Indicators (KPI) might not be applicable due to an application’s innovative nature. However, this shouldn’t discourage nascent AI initiatives, as the technology contributes significantly to the innovation performance of the (German) economy: Companies that use AI are more likely to produce sophisticated innovations with a high degree of novelty, and ~10% of German sales from world market innovations can be attributed to AI.1 Moreover, on an international level, a recent study reports that organizations exhibit a 6.3% increase in business unit revenue directly linked to their AI activities (on average) with companies in AI piloting and implementation phases exhibiting a 4-7% increase in revenue from specific AI efforts, and those in operation and optimization phases boasting an impressive 10-12% gain. 2 To assist in establishing a plausible and standardized way to calculate monetary impact, we have developed illustrative value chain calculation schemes. The table below provides examples of what potential calculation approaches might look like. Many applications could, of course, be motivated by more than one of these value generation drivers. In this case, in order to get a clear picture of the full value potential, it is important to calculate the value for the primary as well as the secondary drivers using the suggested respective calculation approaches. 1 B undesministerium für Wirtschaft und Energie (BMWi) / ZEW - Leibniz Centre for European Economic Research: “Auf Künstliche Intelligenz kommt es an: Beitrag von KI zur Innovationsleistung und Performance der deutschen Wirtschaft“ (2020) 2 IBM Institute for Business Value: “The business value of AI: Peak performance during the pandemic“ (2020) 7 Applying AI MAIN INTERNAL VALUE GENERATION DRIVERS Automation of (repetitive) manual and cognitive tasks • Does the algorithm take over a task that was previously done by a human or another machine? • How much time was previously spent on the task, and how costly was that time? How much money can be saved per event through the algorithm? • How does this differ depending on the performance of the algorithm? AI application automates the filing, documentation, and rediscovery of analogous invoices and delivery notes which are received in various formats (previously, considerable time was spent on trying to find old files in order to reproduce past procurement decisions) • High documentation quality with decreased human effort obtained through AI application Improved resource consumption • Does the algorithm improve resource consumption? • How much can be saved per event through data-based, improved resource consumption? • How does this differ depending on the performance of the algorithm? • Decreased energy consumption in the cooling of data centers through ML-based control (increased resource efficiency) • General-purpose framework to understand complex dynamics; potentially transferable to increasing plant conversion efficiency, reducing semiconductor manufacturing energy/water usage, and increasing manufacturing facilities’ throughput Increased speed of operations • Does the algorithm speed up the process (same output/shortened time frame) or increase operational efficiency (higher output/same timeframe)? • How much can be saved through the increased speed per event? • How does this differ depending on the performance of the algorithm? • More efficient machine utilization and reduced downtime through predictive/prescriptive maintenance • Potentially combined with (semi-)automated process steps, e.g., CV-aided quality control/inspection in series production Improved access to information/ decision making • Does the algorithm gather and evaluate data so that information on certain topics (e.g., prediction forecasts, etc.) is better than before? Does this result in an improved decision-making process? • How do these improved processes save costs? • How does this differ depending on the performance of the algorithm? • CV-aided retrieval of detailed information about specific models and suggestion of similar-looking watches based on customers’ in-store requests (with possibly different characteristics such as price) in short time frames • E.g., Google’s transformation of Cartier’s product search technology (case study) Risk reduction • Can operational risk be reduced through application of the use case? What implications does this have on the business? • How can this be translated into improved processes that save costs (and into additional profits)? • How does this differ depending on the performance of the algorithm? • Analysis of a large variety of documents to calculate credit scores or identify fraudulent patterns Figure 1: Main impact calculations across industries for internal value generation drivers, including indicative examples 8 Applying AI POTENTIAL CALCULATION APPROACHES Resource expenditure for cur- Reduction of effort through use Cost factor Scaling effect (e.g., number of per- rent process (time) cases (in %) (e.g., personnel costs) sons, processes, etc.) Current time spent (e.g., ~15% (potential) time savings Labor costs, [additional workload Could be extended to further handled in the freed up time/pro- administrative tasks based on ductivity boost; decreased error textual documents, e.g., claims, rate] regulatory requirements, etc. Cost factor (e.g., material costs) Scaling effect (e.g., number of pro- tracked/logged by the system) Resource expenditure for Reduction of effort through use current process (e.g., amount of cases (in %) cesses, etc.) material) Energy consumption for cooling ~40% reduction (15% reduction a particular data center (e.g., in overall PUE overhead after Electricity costs, e.g., in €/kWh Scaling across different data centers (or offering it as an AI-based Google/ DeepMind’s case study) accounting for electrical losses and service) other inefficiencies) Resource expenditure for cur- Reduction of effort through use rent process (time) cases (in %) Throughput per hour; cumulated ~10% (potential) savings in cumu- Machine hour rate (plus costs Geographical scaling across sites, production and inspection time lated manufacturing time per batch of production delays, repair or intra-site scaling across production replacement costs) lines Identified opportunities through Realized opportunity (reduction, Scaling effect (e.g., additional use case (in %) uplift) through use case decisions) Current information retrieval Reduction of sales associates’ Increased # of customers served in Scaling across boutiques (upselling time (mostly experience-based, answering time from several min- shorter time (increased sales and potential) manual process); customers utes to seconds (96.5% accuracy reduced personnel cost); increased served; customer satisfaction within three seconds) satisfaction & recurring purchase per batch Addressed KPI Cost factor (e.g., machine costs) Scaling effect (e.g., number of processes, etc.) probability (Estimated) probability of risk Risk reduction through use case occurrence (in %) (in %) Precision of ‘Probability of risk ~8% increase in early detection of occurrence’ and ‘Value at risk’ fraud intent Value at risk (in €) Scaling effect (e.g., additional areas where risk is apparent) Revenue lost per incident Potential extension to investment decisions, etc. estimations 9 Applying AI MAIN EXTERNAL VALUE GENERATION DRIVERS New products or services • How does the algorithm help the business to offer a new product or service, which results in increased (revenue or) profit? • How much additional profit will be generated? • How does this differ depending on the performance of the algorithm? • Mobile taxi ordering service • Exact pinpointing of destination through map integration • Route tracking, driver ratings, and shared rides with customers along the route New business models • How does the algorithm enable the creation of a new business model? How can (revenue or) profit be generated through this business model? • How much additional profit will be generated? • How does this differ depending on the performance of the algorithm? • Demand forecasting for comprehensive network of charging stations • Intelligent, resource-sensitive network planning through recommendations of charging station distribution based on an extensive variety of factors, such as energy grid, traffic routes, competing networks, etc. Improvements of existing products or services (increased customer satisfaction); higher volume • Does the application improve an existing product or service? Does it, e.g., improve the quality of customer experience with the product or service? • How much additional sales (e.g., through cross-selling) can be generated through the improved products or services, and what is the implication for profit? • How does this differ depending on the performance of the algorithm? • Online retailer with tailored product offerings • Recommendation engines used for personalized product suggestions and dynamic pricing Improvements of existing products or services (increased customer satisfaction); higher margin • Does the application improve an existing product or service? Does it, e.g., improve the quality of customer experience with the product or service? • How much additional profits (e.g., by increasing customers’ willingness to pay and imposing higher sales prices) can be generated by the improved products or services? • How does this differ depending on the performance of the algorithm? • Optimization of markdowns (and increasing efficiency in the supply chain) for fashion retailers • Reducing the amount of discounted stock using ML methods to optimize merchandising and mark-down effectiveness Improved customer retention • Does the algorithm help the business to improve customer retention? Can more customers be convinced to return to the product or service through the algorithm? Can more customers be prevented from leaving? • How can this be translated into additional profit? • How does this differ depending on the performance of the algorithm? • Convincing customers to return to the product/service • Prevention of customers leaving the company/service Figure 2: Main impact calculations across industries for external value generation drivers, including indicative examples 10 Applying AI POTENTIAL CALCULATION APPROACHES Current profit (in €) Profit per product or service sold Serviceable obtainable market Scaling effect (e.g., number of per- (in €) (SOM) sons, processes etc.) Current profit within the taxi AI-enabled (additional) profits per Tech-savvy, mobile, urban middle Initial focus on the US-American market ride, e.g., through higher vehicle to upper class within certain geo- market, now worldwide availability utilization graphical region in most big cities Profit per product or service sold Serviceable obtainable market Scaling effect (e.g., number of pro- (in €) (SOM) cesses, etc.) E.g., manufacturing of charging Development and operation of OEMs, car rental companies, gov- Geographical scaling stations charging stations based on AI-en- ernment agencies within certain abled prognosis geographical region Margin before use case or unit Volume increase achieved through Scaling effect (e.g., number of pro- (in €) improvement (in %) cesses, etc.) Volume of products currently (Unchanged) product-specific Additional products sold through Scaling across product offerings sold gross margin recommendations and additional services, utilization of Current profit (in €) Current volume (in units) customer insights Current volume (in units) Volume of products currently Margin before use case or unit Margin increase achieved through Scaling effect (e.g., additional (in €) use case (in %) decisions) Gross margin (e.g., per segment) Increased margin through setting Geographical scaling across seg- the optimum level of discount to ments/stores sold effect a sale and allocating stock to the most appropriate store Current number of customers Average value of customers lost Increase in customer retention Scaling effect (e.g., additional coun- (in €) (in %) tries, etc.) Current # of customers with Average (calculatory) value of a lost Increase in customer retention Scaling across service lines, busi- intention to quit the telecommu- customer ness units, or geographies nications provider 11 Applying AI Calculation of investment expenditures along the AI application life cycle Calculation of the ROAI After determining the expected value, organizations then need to realistically assess the investment expenditures with which they will be confronted throughout the application’s entire life cycle. In practice, it has proven eminently helpful to differentiate between AI use case development expenses (up to deployment) and the costs incurred for operation and maintenance (after deployment). Figure 4 shows an AI application life cycle from ideation to productive ML operation, the process diagrammed on page 13 provides practical guidance for ROAI calculation and incorporates operative as well as strategic and organizational learnings. While the terminology used for each phase may vary from company to company, the life cycle of an ML project is generally represented as a multi-component flow (in part with iterative components), as illustrated below. Each step is unique, which leads to variations in the resources, time, and team members required to complete each phase (as well as changing payoff profiles). We’ve identified central activities in the following figure to assist in the identification and quantification of the relevant cost categories. Figure 3: The ML lifecycle Core activities and cost categories Ideated, prioritized use case Go/no-go decision for further Technically & commercially validated, deployment-ready development Integrated, productive Operational self-learning; application/service subsequent scaling model • (Introductory AI workshop) • Market analysis and technology evaluation • Structured use case • Data analysis, prepa- • Data acquisition from ration, and validation • Deployment of selected (e.g., sensors, ERP systems, (cleaning, [labeling], model(s) for dynamic tenance, and reporting improvement (direct/ • (Automated) retraining indirect), considering • Infrastructure management incl. decisions on archi- the invocation mode and • Incremental cost tecture (environments/ latency requirements written notes) and external feature engineering) sources (e.g., data providers • Model building/training, departments or public databases) • Secure, structured, and units, prediction targets, efficient data processing workbenches) and learning • Integration into and success criteria (initial pipeline design) and (manual/automated existing systems/ model building) services (embedding; storage management • Exploratory data analysis; • Model evaluation, hyper- inference pipeline) initial modeling/testing parameter optimization • Required (personnel) (PoC development) • Model selection and versioning changes to existing processes for the integration of AI applications Required additional infrastructure Hardware costs (Cloud vs. on-premises vs. hybrid), licenses, etc. Miscellaneous External service providers, other factors 12 • Model monitoring/ relevant internal sources ideation across • Definition of analysis • Testing (QA/staging) supervision, main- increases for rollout/ scaling across processes, regions, sites, etc. Prioritized use case(s) (derived from structured ideation & multi-stakeholder pre-assessment) Identification of main source of (expected) value generation: EXTERNAL Identification of main source of (expected) value generation: INTERNAL 1. Identification of the main source of value Figure 4: A systematic approach to AI value assessment Set-up of representative, quantifiable KPIs (value chain factors) Strategic and organizational learning Definition of measurement methodology and frequence; assignment of central/decentral accountability Initial value estimation (based on domain know-how and/ or historical evidence) Deployment, measurement of operative perform. and target/ actual value comparison 3. Value hypothesis testing and refinement STRATEGIC AND ORGANIZATIONAL LEARNING • Collection of historical evidence on AI-based value creation and build-up of a benchmarking database to support future resource claims (e.g., for scaling/further rollout) • Development of a knowledge base regarding uncertainty factors per use case class • Collection of operative best practices in use case assessment and prioritization (e.g., number and frequency of meetings, processes, and collaboration tools) OPERATIVE LEARNING • Refinement of initial value estimation based on value contribution and cost data gathered after deployment • If required: Adjustment of previously defined budgets, timelines, etc. for scaling Representation of value hypothesis as logical value chain/ mathematically linked impact calculation 2. Value hypothesis formulation Applying AI 13 Applying AI Figure 5: Return on AI (ROAI) calculation Benefits from AI product/ application (internal/external value generation) Uncertainty of benefits Based on # of model predictions and value generation per prediction Quantification of frequency and impact/cost of errors RETURN INVESTMENTS Resources required for model development, operation, and maintenance Cost per resource (category) Similar to human decisions, machine predictions (i.e., outputs of data-based models) are unlikely to attain perfect accuracy, and the resulting uncertainty associated with the realization of benefits should be taken into account in the ROAI calculation, as illustrated here. It is, therefore, important to not only consider the costs saved through the application but to also think about the costs incurred through falsely predicted or classified events. False outputs can arise in a variety of forms, depending on the type of application. They may, for example, emerge in the form of false classification or clustering, as when a valid document has been falsely identified as fraudulent (a false negative) or a fraudulent document has been incorrectly assessed as valid (a false positive). A false output could also manifest as a deviation of the actual from the predicted value, e.g., material requirements may be estimated incorrectly, resulting in too much or too little available material. Similarly, false alarms in predictive maintenance cases can lead to unnecessary repair work. 14 As suggested above, the profitable application of AI technologies is considerably more complex than the implementation of ‘traditional’ digitalization initiatives (which often precede AI efforts and create the required data basis or rule-based software systems, which are later expanded to include an AI component). Although building first proofs of concept (exploration) may be comparatively easy, these do not yet bring returns on investment to the company and depend largely on initial investments. AI beginners, especially, tend to encounter high upfront costs in data preparation, IT infrastructure, technology adoption, and people development. Consequently, these early stages focus on technological knowledge expansion and transfer, as expertise, scale, and time are required to reach the break-even point and to create a significant return on investment. After successful deployment, however, the value contribution begins to grow and corporate returns bloom as the AI product/service is gradually scaled across the organization. Applying AI Figure 6: Cumulated value contribution and investment expenditures along the AI application lifecycle Ideated, prioritized use case Go/no-go decision for further Technically & commercially development validated, deployment-ready Integrated, productive Operational self-learning; application/service subsequent scaling model Structured use case ide- Data acquisition; exploratory ation; initial (qualitative) value data analysis and insight Data analysis and preparation; Review for deploy; testing Model monitoring and feature engineering; model (QA/staging); inference mainten. (incl. (automated) training, validation, pipeline design; model ser- retraining); reporting; infra- assessment and ease of generation; initial modeling implementation evaluation; and testing; (re-)evaluation of and testing; (automated) ving (deployment to appropri- structure mgmt.; further roll- connection of model perf. value and technical feasibility model selection; model ate runtime engine) metrics to business KPIs hypotheses versioning (exp. tracking) out/scaling across processes, regions, sites, etc. € (cumulative) Returns/ benefits Time 15 Applying AI Early, short-term value capture and foundations for long-term success With increasing AI maturity, the corporate perspective on AI is changing: While the focus at the beginning is on the assessment and implementation of individual use cases, AI portfolio management is becoming increasingly important with the successful operationalization of applications across technology domains. The following section thus provides guidance on the transition from early, short-term value capture and mid-term value realization to sustainable, long-term AI successes. Shifting priorities and use case prioritization criteria along the AI journey To utilize AI technologies’ full value potential and become a market leader, a company must experience a holistic AI transformation, a process which involves an integrated, multistep journey. Initially, organizations should thus strive to capture the short-term value pools which will most likely be found in the optimization of internal processes, while at the same time preparing to seize the longterm value potential of technologically more complex applications. Resources that were freed up in the short to mid-term need to be reinvested, and gained capital and knowledge should be leveraged to increase overall AI maturity. The first pilots, when implemented rapidly, can serve as early demonstrations of the type of value creation that AI applications are able to generate and will, therefore, help to build trust in and commitment to AI within the company. For each main department, companies should identify the top AI applications and implement them using a test-and-learn 16 logic. Building upon initial successes, companies then roll out promising AI applications across the organization. For example, AI applications that were first implemented in a single factory or region can be scaled to the entire or international factory ecosystem to lift the full value potential. Long-term success and value creation depends on building a solid foundation and procuring sufficient investment at these early stages. Simultaneously, based on the lessons learned from the initial use cases, companies use the established momentum to develop the required enabling factors, such as a standardized data ecosystem, adequate infrastructure set-up, and a trusted ecosystem of (implementation) partners. In parallel, a technologically- and strategically-versed AI core team (which may later evolve into an AI center of excellence) with formalized governance structures and processes should be established. (For more information, please refer to appliedAI’s “The Elements of a Comprehensive AI Strategy” and “Building the Organization for Scaling AI” publications.) Successful players have made significant headway in setting up business cases, implementation plans, and systems for measuring and monitoring AI performance. They are also far along in implementing AI platforms to gather, integrate, process, and manage data. Moreover, having already made major progress in developing basic AI capabilities, they are able to invest in a next-generation technology stack. Successful companies with high AI maturity as well as above-peer profitability and free cash flow from AI innovations may thus achieve compounding positive returns and the network-effect advantages resulting from their firm commitment to AI transformation. Applying AI Excursus: Elements of a comprehensive AI strategy There is little doubt that AI will become relevant for all companies, regardless of their industry or size. When it comes to creating value from AI, experience has demonstrated several potential pitfalls, including the isolation of AI products or applications, a lack of resources and capabilities, and a poor understanding of use cases and applications. To avoid such pitfalls, a systematic approach towards AI is needed. It is imperative, therefore, that from the very beginning a company is clear on the overarching objectives or purpose of the company’s use of AI. Furthermore, it is necessary to understand how AI can help the company achieve its broader objectives. A company’s AI ambition sets the highlevel goals for which any AI application is developed. The AI ambition incorporates an understanding of the current position of the company, its competitive stadium, and the industry dynamics, including potential changes to the industry’s business model. On this basis, it can be decided where the organization could benefit most from AI − whether within a specific product/service or by improving processes, or both. The AI ambition then needs to be translated into a portfolio of AI use cases. To build this portfolio, the company needs to identify and prioritize relevant use cases. Figure 7: appliedAI’s comprehensive AI strategy house The Elements of an AI Strategy Ambition Future Competitive Advantage Fields of Action Commitment AI Use Cases Discovery and Specification Make or Buy Portfolio Management Enabling Factors Execution Organization Expertise Research and Exploration Culture Data Development and Validation Technology Ecosystem Operationalization and Maintenance 17 Applying AI Before the use cases can be executed, however, numerous enabling factors must be addressed regarding the organizational structure of the company’s AI initiatives, the employees’ position vis-a-vis AI adoption, the technology involved, and the AI ecosystem: • First, a company needs to set up the right organization for its AI initiatives. One best practice is a hybrid approach: Here, a central AI team – often called the Center of Excellence (CoE) – bundles certain functions and expertise while maintaining strong links to decentralized units and the rest of the organization. An appropriate governance structure will also have to be established, a development that may even necessitate changes to the inventory of Board roles (see appliedAI’s “Artificial Intelligence for Boards” whitepaper for a comprehensive deep-dive). • Second, employees need to be prepared for AI adoption, and the necessary talent must be recruited. New roles are emerging: AI engineers, for example, are required to build learning systems from an engineering perspective. Acquiring and retaining the employees with the right skills is currently a major challenge, which is why reskilling the company’s existing data scientists or software engineers and qualifying the company’s business experts are important options to consider. • 18 But that is not enough. Other employees, including the executives, need to have a basic understanding of what AI will enable and how it will change their working lives. Everyone must be brought on board because silent resistance at various points within a company can be detrimental for the success of an AI initiative. Such acceptance of AI requires an adaptation of company culture and acceptance of the fact that the use of learning systems implies a certain degree of being comfortable with failure. Employees’ fears need to be addressed so as to create acceptance for the use of AI-based solutions, and the company will need to exhibit a very high level of trustworthiness in order to implement AI use cases successfully. This includes explainability, fairness, transparency, safeguarding data privacy, and robustness against adversarial threats and potential incursions. In order to manage risk and regulatory requirements, as well as to protect their brand reputations, companies are recognizing the importance of a comprehensive approach to governed data and AI technologies. • Moreover, the company needs to build up the required technology for adoption of AI, including both the necessary AI infrastructure and the data. The latter is key, as the training of AI models requires a great deal of data, and if a corporation does not already have well-defined data governance, it is unlikely that the company will possess readily usable data. In that case, data sources will need to be identified, data pipelines built, data cleaned and prepared (including, e.g., anonymization in order to fulfill GDPR requirements), potential signals in the data detected, and results measured. An adequate IT infrastructure is also required: A principal question is whether to use the company’s own servers and GPUs or rely on the cloud. The answer to this question hinges not only on data security but also on cost and economic feasibility. • Ultimately, the company’s ecosystem must be addressed. At present, hardly any company has truly comprehensive experience when it comes to applying AI. Therefore, it is beneficial for a company to exchange knowledge externally: with startups, academia, and other companies. (Helpful guidance on partnering approaches, make-or-buy decisions with regards to AI, and contract design/structure, including data appropriation and IP buy-out rights, may be found in applied AI’s “Enterprise Guide for Make-or-Buy Decisions”.) Finally, the use cases can be executed. But keep in mind that AI isn’t like traditional software: An AI system learns continuously as new data are fed into the system. Thus, an AI system needs to be monitored to ensure that the model is still delivering the expected results. For this, a company needs to put in place the right (automated) processes. (MLOps best practices and practitioners’ experiences may be found in appliedAI’s Enterprise Guide to ML.) Applying AI AI portfolio management, assessment criteria, and evaluation process AI portfolio management approaches With high upfront expenditures in data preparation, technology adoption, as well as personnel recruiting and employee qualification, reaching the break-even point and generating significant returns from AI requires time and scale, as was noted earlier. Rather than computing the success or failure of AI initiatives use-case-by-use-case, a portfolio approach to ROAI and a value-based operative process for the management of the corporate AI portfolio have proven to be particularly effective. This approach enables a joint realization of the defined return targets (e.g., in terms of VC-financing) while acknowledging ML-specific failure probability, balancing potential risks, utilizing synergies in scaling and learning effects, and detecting white-spots in the AI strategy. Similar to the calculation of ROAIs for singular AI applications and products, however, no universally agreed upon industry standards for managing AI portfolios have yet emerged. In striving for higher degrees of sophistication in the balancing of their AI portfolios, decision makers find inspiration from multinational pharmaceutical corporations’ (and venture capital firms’) test-andlearn approach, modern portfolio theory’s risk-return tradeoff (widely used in financial services), and the innovation-focused competitiveness-investment tradeoff. fail clinical trials and once promising startup ideas fail to stand the test of time and market, a select handful succeed, and some even go on to become blockbusters that more than compensate for the portfolio’s other entries. Similarly, in the case of startups, the distribution of returns tends to be heavily skewed, as described by the power law (i.e., a small percentage of companies capture a large share of industry returns). To complement this approach, AI decision makers can adapt three key characteristics of modern portfolio theory (MPT) to manage AI initiatives: a portfolio approach to assessing returns, the inclusion of risk and the risk-return tradeoff as a key concern, and the availability of diverse AI ‘asset classes’ with variable risk-return profiles (e.g., based on experience gained from previously realized applications). Rather than focusing solely on singular AI applications (an approach which keeps current costs comparatively low and does not overburden the organization with change, but comes at the expense of a higher risk of an individual initiative failing and reduced speed by having to look at ideas sequentially), companies should thus strive to build a portfolio of AI applications over time. Moreover, decision makers should weigh additional factors, such as model fairness, explicability, and safety, in their holistic assessment. Ultimately, corporations may differentiate between three sorts 3 of AI investments: • Pharmaceutical companies and, similarly, venture capitalists, frequently employ a portfolio approach, as they often find it difficult to predict whether or not a specific medication candidate or startup will be successful. While many prospective pharmaceuticals 3 Stay in Business (SIB) investments are for fundamental infrastructure or non-discretionary legal/regulatory mandates. Such investments should be judged on their ability to meet regulatory or technological criteria while reducing risk and expense. Adapted from: Chunka Mui: “3-Point Plan for Balancing Your Innovation Portfolio” (2014) 19 Applying AI • • (Classical) Return on Investment (ROI) opportunities are pursued in order to generate predictable, short-/mid-term financial returns. Here, standard measurements like net present value (NPV), return on investment (ROI), and other well-known metrics are most commonly used. Option Creating Investments (OCI) are sought in order to develop company choices that could lead to future ‘killerapp’-type opportunities. OCI investments do not produce immediate cash returns. Instead, they develop skills and knowledge that can be used to take advantage of future ROI possibilities. OCIs, like financial options, typically have a high level of risk and provide extremely high rewards. Organizations that strive for competitive differentiation in a particular (sub-)sector or AI technology field are typically overweight in OCI, while those that want to stay on par with the competition are usually overweight in ROI projects, and players who have just launched their AI activities are usually overweight in SIB initiatives so as to prevent losses in competitiveness. As the respective industry’s overall AI maturity increases and organizations become more proficient in AI implementation and scaling, certain AI technologies tend to shift from OCI to ROI initiatives, and eventually evolve into table stakes or SIB projects (e.g., many data transformation, data quality, and business intelligence (BI) initiatives have gradually morphed into SIB projects across industries). Analyzing the AI portfolio according to these investment categories provides a unique complementary perspective and allows a company to filter as appropriate – for example, by eliminating ROAI opportunities that do not meet the firm-specific standard hurdle rate or OCI opportunities that do not provide exceptional option value. (The valuation tool may primarily be used for this type of investment. For SIB, decisions are sometimes made independently of ROAI considerations, especially when complying with regulations. For Option Creating Investments (OCI), profitability and ROAI play a role when viewed over the long term; however, due to increased uncertainty and a long time horizon, the cash flows are sometimes difficult to forecast and seldomly constant.) 20 First- versus second-order assessment criteria In order to optimize the models and data that inform AI applications, as well as to avoid inefficient resource utilization, counter the risk of ‘reinventing the wheel’ across departments, and reduce complications caused by competing methods and multiple vendors, the need for a comprehensive review of the individual use cases’ application domains arises. Additionally, such a review will facilitate a more holistic, long-term vision for the application of AI and diminish the risk of realizing cases that do not support the company’s strategy due to simply being driven forward by management pressure. The following figure further illustrates the differences between these first- and second-order criteria. As noted earlier, at first companies might want to focus on low-risk, mid- to high-value use cases that can be rapidly implemented, as required data and expertise are already present and will thus generate value very quickly. However, once a company has established a basic portfolio of viable AI use cases, we recommend investing in use cases which at first glance are not the easiest to implement and will not generate significant value in the short term. Such use cases, however, support a greater objective and broaden the organization’s AI approach, thus preparing the company for long-term strategic goals. Ideally, value generated through the first use cases will cross-finance investments in the subsequent ones. First- and second-order criteria may thus be considered at different times in holistic AI portfolio prioritization and steering. Although the selection and prioritization of particular first- and second-order criteria depend on a company’s unique background (size, industry, data, infrastructure set-up, AI competencies, granularity of existent and planned investment decision and monitoring processes, etc.), all companies should track the business value, implementation effort, and risk (equivalent to the implied likelihood of success) right from the start of each AI initiative. Irrespective of the ‘AI’ label, the same principles and scrutiny that apply to all investment decisions should be followed as well, which includes being conservative with projections: Undervaluing an AI use case is preferable Applying AI to overpromising, and it is crucial to ensure that estimates are derived as accurately as possible from consultations with technological implementers, AI strategists, domain experts, and finance and accounting professionals. Moreover, it is crucial to address the fundamental issues in an iterative manner: Even the best model will fail if the underlying data are inaccurate, which is why use case development and data management have to go hand in hand. While proof-of-concept initiatives can help with company buy-in, it must be ensured that data are reliable, consistent, and updated automatically; otherwise, the targets of AI initiatives will not be reached and systems will be destined to fail at the point of their operational application. Figure 8: First- and second-order criteria for AI portfolio prioritization and steering High VALUE FOR OVERALL AI STRATEGY ADDITIONAL VALUE COMPONENTS Economic value • Absolute/relative return • Time-to-value (short- VALUE vs. long-term) • Risk reduction potential • Value chain: focused vs. balanced approach • Department: lead vs. follower • Geography: selective vs. broad • Top-/bottom-line contribution Strategic alignment • Competition aspect (indus- Low try-wide necessity vs. differ- LEARNING EFFECTS • Operational learnings • Strategic knowledge building entiation opportunity Low EASE OF IMPLEMENTATION High REALIZATION • Building vs. partnering vs. buying, and hybrid forms • Maintenance effort • Assess use case ideas along core dimensions of value EASE OF IMPLEMENTATION Value and implementation effort • Availability and quality • Decompose highly strategic, • Effort for integration/ complex use cases into smaller solutions to make them manageable as intermediate viable products • Cluster use cases with similar underlying technology (capabilities/data) • Execution of one UC can have positive implementation benefits for other UCs • Implied change in the prioritization due to collective required updates Required know-how • Existing technical know-how • Existing domain knowledge Processes & systems • Affected processes & systems, required changes Technology clusters • Existing implement. of UC/algorithm • Selective/focused vs. broad approach implementation speed-up 21 Applying AI AI portfolio management process From an operational perspective towards value-based AI portfolio management, we propose an iterative, fivestep process (see below) that is based on our partner companies’ best practices. This process begins with use case ideation and initial prioritization, followed by a detailed assessment (i.e., quantification of value hypothesis) through a centralized unit (e.g., the AI CoE and departments), given that building upon a uniform evaluation logic is important to ensure transparency and comparability. This is followed by implementation along with continuous monitoring of the technical application and evaluation of the KPI-based target performance. Once a company has successfully implemented and scaled multiple AI products and services, clustering these by technological focus areas (or, e.g., by business units and geographical regions) helps to identify over- and underperforming clusters and to derive associated strategic implications (e.g., increase in investment). Ultimately, success factors (and failures) across the use case life cycle phases must be identified and collected centrally. It is also good practice for the company to monitor the amount of time between ideation and prototype development, and between the prototype and deployment, plus the use case Figure 9: Systematic AI portfolio management Iterative approach CORE CONTENTS • UC ideation and initial prioritization • Definition of measurement • Detailed assessment of prioritized use cases (quantification • Efficient UC implementation (with organiza- families, i.e, geographical/ tion-wide roll-out of business clusters or technology focus areas methodology & responsi- of value hypothesis) single use case and data/ bility sharing btw. CoE & BUs through CoE & BUs resource sharing in mind) • Budget structuring • Detailed, yet pragmatic, implementation effort estimation • Exploration phase for Top 5 UCs • Grouping of UC into • Continuous technical monitoring • Continuous KPI-based target-performance • Assessment of over- • Knowledge-building with increasing experience and AI maturity • Centralized tracking of success factors (and and underperforming failures) for discovery, clusters and derivation of exploration, deployment, strategic implications (e.g., increase in investments) and operation phases • Sharing of knowledge & data artifacts comparison OUTPUTS • UC database incl. first ranking (e.g., Top 5, 10) • Unified understanding re: (final) decision compet. • Joint budget (CoE/ BU) for UC implementation & monitoring • Unified basis for discussion across BUs • Objective basis of decisionmaking for internal or external implementation • Approved UCs for • Implemented applications • Systematic value contribution analyses at UC level • Thorough tracking • Aggregated value • Efficient UC implemen- assessment of AI tation through intra-or- solution clusters ganizational learnings and • Aggregated implementation ideal resource utilization of implementation cost overview per category • Culture that embraces efforts at UC level (e.g., FTE, local hardware, learning from failure cloud hosting, etc.) development and implementation AAI ASSESSMENT TOOL • Introduction to BUs & Finance/Accounting through CoE (tool owner) • Illustration of multiple factors to consider for AI 22 • Enabler for standardized • Provision of calculation comparison of (expected) logic for main value driver UC value contribution analysis at UC level • Increasing sophisti- • Enabler for detailed • Aggregation of single UC to clusters possible • Direct assessment of clusters possible (e.g., cation of implemen- implementation cost through consideration of tation cost estimation factor analysis at UC level total infrastructure cost) • Unified language across departments (CoE, BUs Finance/Accounting, etc.) • Accessible educational tool for non-AI experts Applying AI AI portfolio management tools success rate (i.e., the ratio of applications deployed to production versus requests from business teams or other teams within the organization). When administering a structured valuation process, introducing the valuation tool and framework to all relevant stakeholders (e.g., through the AI CoE) is crucial to ensure sustainable success. Furthermore, having clear and transparent investment criteria defined has been shown to substantially increase the quality of ideated use cases. Given limited budget and development resources, an objective, data-based evaluation process also mediates between the sometimes competing interests of departments or business units. This is especially relevant as corporations have progressively moved towards joint financing between the business unit or subsidiary implementing the use case and the parent corporation, with the latter’s budget contingent on pre-defined investment criteria. To monitor the AI portfolio and to visualize progress over time, a radar perspective has proven to be a particularly powerful view in practice. Use cases can be structured in several dimensions, such as technology clusters (e.g., computer vision, planning, and discovery) or core functions (e.g., product development, manufacturing, and marketing and sales) (see below). Furthermore, the concentric spheres of the radar illustrate the life cycle phases in which the individual use cases are contained. This can be useful when investigating how well various AI capabilities have been developed and how mature different business units are in terms of AI application, thus facilitating the identification of focus areas and weak links. Making this unified overview available to all involved stakeholders ensures that the whole organization has knowledge of the breadth and depth of its AI journey. Figure 10: Exemplary overview of use cases by technology cluster Foreca st i n g Di sc ov er y Pl Co au m pu d i te t io r n r te pu t ic s m s Co g u i il n n ced Ad v a s a n d c i ot rob ntrol co an nin Operation, monitoring, maintenance, and scaling Deployment Development, validation, and testing Exploration Ideation & scoping t ion C o m p u te r Crea vi sio n g Use cases 23 Applying AI Figure 11: Exemplary use case detail view from the insurance industry: Deep dive on use case ‘AI-enhanced lead management’ (use case 17) Exemplary insurance value chain: AI-ENHANCED LEAD MANAGEMENT: PROVISION OF NEW SALES LEADS BASED ON SOCIAL MEDIA AND PURCHASE DATA • • • 24 Identification of promising prospects/potential quality leads through enrichment of internal information with data from a variety of sources, e.g., from social media campaigns or weblog clickstreams Creation of cross-/upselling opportunities through product personalization (prediction, e.g., of potential spend, and suggested campaigns) Personalized lead interaction also applicable in call centers Low High Low High Low High Low High Applying AI Figure 12: Exemplary overview of use cases by business functions CO RE FU T NC u an f NS t ac ur i ng a nd ra t i o n O p e a g e m e nt ma n Marketi ng s a l es a n d Fi n an ce SU M IO PP O FU Su ma ppl na y c ge ha m in en t RT NC TI O IT NS Operation, monitoring, maintenance, and scaling Deployment Development, validation, and testing Exploration Ideation & scoping Produ develop ct men er n d ot h H R a t fu n c t i o n s po r t su p Use cases 25 Authors About appliedAI Jannik Seger, Senior AI Strategist at appliedAI Dr. Philipp Hartmann, Director of AI Strategy at appliedAI Dr. Andreas Liebl, Managing Director at appliedAI appliedAI is Europe’s largest initiative for the application of leading-edge, trustworthy AI technology, with the vision of shaping Europe’s innovative power in AI. appliedAI was formed as an objective, reliable initiative that acts both as enabler and innovator. Based on our ecosystem, we promote value creation by helping to build global AI champions. The authors would like to thank Clara Laufenberg, Clara Mehler, and Christian Wender for their invaluable contributions in writing this report and in the development of the associated tool, as well as Henrike Noack for designing this publication. Contributors The AI use case assessment tool and this report are the result of appliedAI’s ‘Value Assessment of AI Use Cases’ Working Group. We have drawn on the experience of the following leading experts from appliedAI partner companies and are grateful for their contributions: Matthias Neuenhofer (BayWa AG) Helfried Binder, Christian Funke, Olaf Niebisch, Daniela Rittmeier (BMW AG) Philipp Stähle (EnBW Energie Baden-Württemberg AG) Anant Nawalgaria (Google LLC) Simon-Pierre Genot, Nico Kelling (Infineon Technologies AG) Matthias Weber (Sandoz Deutschland / Hexal AG) You can find more information about appliedAI at: www.appliedai.de appliedAI Initiative GmbH Freddie-Mercury-Str. 5 80797 Munich Germany www.appliedai.de