Attention Approaches Module 5 (2024)
Document Details
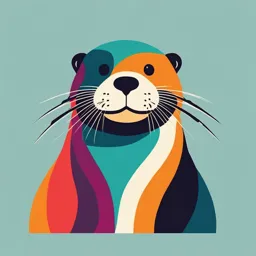
Uploaded by EasedJasper3951
Vellore Institute of Technology
2024
Tags
Summary
This document is a syllabus for Module 5: Region Based CNN, focusing on Attention Approaches, including Encoder-Decoder Models, Attention approaches, RCNN, Yolo, data collection, image labeling, building custom models, comparative analysis, various applications, and challenges in encoding long sentences.
Full Transcript
Module -5 Region Based CNN - Syllabus Encoder-Decoder Models, Attention approaches, RCNN, Yolo and its versions Data Collection, Image labeling and Training. Build Custom models, Comparative analysis. Various Applications Attention Approaches Introduction to attention mechanisms Trad...
Module -5 Region Based CNN - Syllabus Encoder-Decoder Models, Attention approaches, RCNN, Yolo and its versions Data Collection, Image labeling and Training. Build Custom models, Comparative analysis. Various Applications Attention Approaches Introduction to attention mechanisms Traditional Machine Translation systems typically rely on sophisticated feature engineering based on the statistical properties of text. In short, these systems are complex, and a lot of engineering effort goes into building them. Neural Machine Translation systems work a bit differently. In NMT, we map the meaning of a sentence into a fixed-length vector representation and then generate a translation based on that vector. NTM systems are much easier to build and train, and they don’t require any manual feature engineering. Most NMT systems work by encoding the source sentence (e.g. a German sentence) into a vector using a Recurrent Neural Network, and then decoding an English sentence based on that vector, also using a RNN. ce e n ng n t di Se bed Em Challenges Encoding all information about a potentially very long sentence into a single vector and then have the decoder produce a good translation based on only that. For example, assume the sentence is of 100 words long, and the first word of the English translation is probably highly correlated with the first word of the source sentence. But that means decoder has to consider information from 100 steps ago, and that information needs to be somehow encoded in the vector.\ Recurrent Neural Networks are known to have problems dealing with such long-range dependencies. LSTMs should be able to deal with this, but in practice long-range dependencies are still problematic. Solutions Reversing the source sequence (feeding it backwards into the encoder) produces significantly better results because it shortens the path from the decoder to the relevant parts of the encoder. Similarly, feeding an input sequence twice also seems to help a network to better memorize things. In a few cases(German/French, this technique might work) In a few other cases, there are languages in which the last word might be the root cause to predict the first word in the translation In that case, reversing the input would make things worse Attention mechanism The attention mechanism was introduced to improve the performance of the encoder- decoder model for machine translation. With an attention mechanism there is no need to encode the full source sentence into a fixed-length vector. Rather, we allow the decoder to “attend” to different parts of the source sentence at each step of the output generation. Importantly, we let the model learn what to attend to based on the input sentence and what it has produced so far The idea behind the attention mechanism was to permit the decoder to utilize the most relevant parts of the input sequence in a flexible manner, by a weighted combination of all the encoded input vectors, with the most relevant vectors being attributed the highest weights. The attention mechanism was introduced by Bahdanau et al. (2014) to address the bottleneck problem that arises with the use of a fixed-length encoding vector, where the decoder would have limited access to the information provided by the input Bahdanau et al.’s attention mechanism is divided into the step-by-step computations of the alignment scores, weights, and context vector also known as Additive attention as it performs a linear combination of encoder states and the decoder states all the encoder hidden states, along with the decoder hidden state are used to generate the Context vector 1. Producing the Encoder Hidden States - Encoder produces hidden states of each element in the input sequence 2. Calculating Alignment Scores between the previous decoder hidden state and each of the encoder’s hidden states are calculated (Note: The last encoder hidden state can be used as the first hidden state in the decoder) 3. Softmaxing the Alignment Scores - the alignment scores for each encoder hidden state are combined and represented in a single vector and subsequently softmaxed 4. Calculating the Context Vector - the encoder hidden states and their respective alignment scores are multiplied to form the context vector 5. Decoding the Output - the context vector is concatenated with the previous decoder output and fed into the Decoder RNN for that time step along with the previous decoder hidden state to produce a new output 6. The process (steps 2-5) repeats itself for each time step of the decoder until an token is produced or output is past the specified maximum length Producing the Encoder Hidden States After passing the input sequence through the encoder RNN, a hidden state/output will be produced for each input passed in. Instead of using only the hidden state at the final time step, we’ll be carrying forward all the hidden states produced by the encoder to the next step. Calculating Alignment Scores After obtaining all of our encoder outputs, decoder is used to produce outputs. At each time step of the decoder, alignment score of each encoder output with respect to the decoder input and hidden state at that time step is computed. The alignment score is the essence of the Attention mechanism, as it quantifies the amount of “Attention” the decoder will place on each of the encoder outputs when producing the next output. Bahdanau Attention are calculated using the hidden state produced by the decoder in the previous time step and the encoder outputs 1 2 3 Global Attention The term “global” Attention is appropriate because all the inputs are given importance. Originally, the Global Attention (defined by Luong et al 2015) had a few subtle differences with the Attention concept we discussed previously. The differentiation is that it considers all the hidden states of both the encoder LSTM and decoder LSTM to calculate a “variable-length context vector ct, whereas Bahdanau et al. used the previous hidden state of the unidirectional decoder LSTM and all the hidden states of the encoder LSTM to calculate the context vector. When a “global” Attention layer is applied, a lot of computation is incurred. This is because all the hidden states must be taken into consideration, concatenated into a matrix, and multiplied with a weight matrix of correct dimensions to get the final layer of the feedforward connection. To solve this we can prefer local attention Soft Attention is the global Attention where all image patches are given some weight; but in hard Attention, only one image patch is considered at a time. Hard means that it can be described by discrete variables while soft attention is described by continuous variables. In other words, hard attention replaces a deterministic method with a stochastic sampling model. starting from a random location in the image tries to find the “important pixels” for classification Roughly, the algorithm has to choose a direction to go inside the image, during training. Cannot use SGD. To train we need RL Luong Attention and Bahdanau Attention The two main differences The way that the alignment score is calculated The position at which the Attention mechanism is being introduced in the decoder Application They use a Convolutional Neural Network to “encode” the image, and a Recurrent Neural Network with attention mechanisms to generate a description. By visualizing the attention weights (just like in the translation example), we interpret what the model is looking at while generating a word: SELF ATTENTION The Transformer – a model that uses attention to boost the speed with which these models can be trained. The Transformer outperforms the Google Neural Machine Translation model in specific tasks. The biggest benefit, however, comes from how The Transformer lends itself to parallelization. It is in fact Google Cloud’s recommendation to use The Transformer as a reference model to use their Cloud TPU offering. The encoder’s inputs first flow through a self-attention layer – a layer that helps the encoder look at other words in the input sentence as it encodes a specific word. The outputs of the self-attention layer are fed to a feed-forward neural network. The exact same feed-forward network is independently applied to each position. The decoder has both those layers, but between them is an attention layer that helps the decoder focus on relevant parts of the input sentence (similar what attention does in seq2seq models). The embedding only happens in the bottom-most encoder. The abstraction that is common to all the encoders is that they receive a list of vectors each of the size 512 – In the bottom encoder that would be the word embeddings, but in other encoders, it would be the output of the encoder that’s directly below. one key property of the Transformer, which is that the word in each position flows through its own path in the encoder. There are dependencies between these paths in the self-attention layer. The feed-forward layer does not have those dependencies, however, and thus the various paths can be executed in parallel while flowing through the feed-forward layer. an encoder receives a list of vectors as input. It processes this list by passing these vectors into a ‘self-attention’ layer, then into a feed-forward neural network, then sends out the output upwards to the next encoder. The animal didn’t cross the street because it was too tired As the model processes each word (each position in the input sequence), self attention allows it to look at other positions in the input sequence for clues that can help lead to a better encoding for this word. Maintaining a hidden state allows an RNN to incorporate its representation of previous words/vectors it has processed with the current one it’s processing. Self-attention is the method the Transformer uses to bake the “understanding” of other relevant words into the one we’re currently processing. The first step in calculating self-attention is to create three vectors from each of the encoder’s input vectors (in this case, the embedding of each word). So for each word, we create a Query vector, a Key vector, and a Value vector. These vectors are created by multiplying the embedding by three matrices that we trained during the training process. The second step in calculating self-attention is to calculate a score. Calculating the self-attention for the first word in this example, “Thinking”. Compute score for each word of the input sentence against this word. The score determines how much focus to place on other parts of the input sentence as we encode a word at a certain position. The score is calculated by taking the dot product of the query vector with the key vector of the respective word we’re scoring. So if we’re processing the self-attention for the word in position #1, the first score would be the dot product of q1 and k1. The second score would be the dot product of q1 and k2. The third and fourth steps are to divide the scores by 8 (the square root of the dimension of the key vectors used in the paper – 64. This leads to having more stable gradients. There could be other possible values here, but this is the default), then pass the result through a softmax operation. Softmax normalizes the scores so they’re all positive and add up to 1. The fifth step is to multiply each value vector by the softmax score (in preparation to sum them up). The intuition here is to keep intact the values of the word(s) we want to focus on, and drown-out irrelevant words (by multiplying them by tiny numbers like 0.001, for example). The sixth step is to sum up the weighted value vectors. This produces the output of the self-attention layer at this position (for the first word). Final result Matrix calculation INPUT : "I love to play basketball." Word vectors: "I" -> [1, 0, 0] "love" -> [0, 1, 0] "I" -> [0.1, 0.2, 0.3] "to" -> [0, 0, 1] "play" -> [1, 1, 0] "love" -> [0.2, 0.3, 0.4] "basketball" -> [0, 1, 1] "to" -> [0.3, 0.4, 0.5] "." -> [1, 0, 1] "play" -> [0.4, 0.5, 0.6] Suppose to compute self attention for the word play "basketball" -> [0.5, 0.6, 0.7] Three vectors: a query vector, a key vector, and a value vector. "." -> [0.6, 0.7, 0.8] These vectors are learned parameters of the self- attention mechanism, and they are used to determine how much attention to pay to each word in the input sentence. Step 2: Query, Key, and Value Next, we use the embedding vectors to compute the query, key, and value vectors. Let's say we choose a simple linear transformation to compute these vectors: Query("play") = 0.5 * Embedding("play") = [0.5, 0.5, 0] Key("play") = 0.5 * Embedding("play") = [0.5, 0.5, 0] Value("I") = 0.5 * Embedding("I") = [0.5, 0, 0] Value("love") = 0.5 * Embedding("love") = [0, 0.5, 0] Value("to") = 0.5 * Embedding("to") = [0, 0, 0.5] Value("play") = 0.5 * Embedding("play") = [0.5, 0.5, 0] Value("basketball") = 0.5 * Embedding("basketball") = [0, 0.5, 0.5] Value(".") = 0.5 * Embedding(".") = [0.5, 0, 0.5] Step 3: Scoring We then compute the dot product of the query vector with the key vector for each word to obtain the score: Score("play", "I") = 0.5 * 0.5 + 0.5 * 0 + 0 * 0 = 0.25 Score("play", "love") = 0.5 * 0 + 0.5 * 0.5 + 0 * 0 = 0.25 Score("play", "to") = 0.5 * 0 + 0.5 * 0 + 0 * 0.5 = 0 Score("play", "play") = 0.5 * 0.5 + 0.5 * 0.5 + 0 * 0 = 0.5 Score("play", "basketball") = 0.5 * 0 + 0.5 * 0.5 + 0 * 0.5 = 0.25 Score("play", ".") = 0.5 * 0.5 + 0.5 * 0 + 0 * 0.5 = 0.25 Step 4: Attention Weights softmax function to the scores to obtain the attention weights: Attention("play", "I") = e^Score("play", "I") / (e^Score("play", "I") + e^Score("play", "love") + e^Score("play", "to") + e^Score("play", "play") + e^Score("play“, “basketball“) + e^Score("play“, “.”) softmax([0.02, 0.26, 0.12, 0.46, 0.12, 0.02]) = [0.067, 0.215, 0.107, 0.367, 0.107, 0.067] Then we compute the weighted sum of the value vectors for each word, using the attention weights as the weights: Output("play") = weighted sum of the values for each word = [0.067 * 1 + 0.215 * 1 + 0.107 * 0 + 0.367 * 1 + 0.107 * 1 + 0.067 * 1, 0.067 * 0 + 0.215 * 1 + 0.107 * 0 + 0.367 * 1 + 0.107 * 1 + 0.067 * 0, 0.067 * 0 + 0.215 * 0 + 0.107 * 1 + 0.367 * 0 + 0.107 * 1 + 0.067 * 1] = [0.5, 0.582, 0.386] If the self-attention mechanism is part of a larger transformer model, the output of the self-attention layer is usually passed through a feedforward neural network layer, followed by additional self-attention and feedforward layers. Attention with Multi Heads It expands the model’s ability to focus on different positions. Yes, in the example above, z1 contains a little bit of every other encoding, but it could be dominated by the actual word itself. If we’re translating a sentence like “The animal didn’t cross the street because it was too tired”, it would be useful to know which word “it” refers to. It gives the attention layer multiple “representation subspaces”. Multi-headed attention have not only one, but multiple sets of Query/Key/Value weight matrices (the Transformer uses eight attention heads, so we end up with eight sets for each encoder/decoder). Each of these sets is randomly initialized. Then, after training, each set is used to project the input embeddings (or vectors from lower encoders/decoders) into a different representation subspace. If we do the same self-attention calculation as done earlier, just eight different times with different weight matrices, we end up with eight different Z matrices The feed-forward layer is not expecting eight matrices – it’s expecting a single matrix (a vector for each word). So we need a way to condense these eight down into a single matrix. How do we do that? We concate the matrices then multiply them by an additional weights matrix WO. Cross attention The English sentence "I love music" is represented as a sequence of vectors, with each vector representing a word in the sentence. Similarly, a French sentence "J'aime la musique" is represented as a sequence of vectors. The cross-attention mechanism is used to compute attention scores between each position in the English sentence and all positions in the French sentence. For example, when attending to the word "love" in the English sentence, the cross- attention mechanism may assign high weights to the words "aime" and "musique" in the French sentence, since these words are most likely to be relevant for the translation of the word "love". The output of the cross-attention mechanism is a weighted sum of the vectors representing the French sentence, with the weights determined by the attention scores. This weighted sum is concatenated with the output of the self-attention layer in the same position and passed through a feedforward neural network to generate the final translation of the English sentence into French.