20240906T123845373.att.3924172291147747.pdf
Document Details
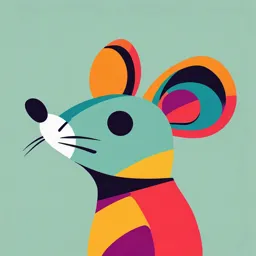
Uploaded by ComfyKhaki
Tags
Related
- PASS biostats.pdf
- Epidemiology & Biostatistics Lecture 4 (2024) PDF
- Jekel’s_Epidemiology,_Biostatistics,_Preventive_Medicine,_and_Public-19-30.pdf
- Jekel’s_Epidemiology,_Biostatistics,_Preventive_Medicine,_and_Public-32-39.pdf
- Association and Causality ملف اضافي 2.pdf
- 20240906T123845373.att.3924172291147747.pdf
Full Transcript
BIOSTATISTICS RETROSPECTIVE STUDY LABORATORY In a retrospective study, an outcome is identified after the...
BIOSTATISTICS RETROSPECTIVE STUDY LABORATORY In a retrospective study, an outcome is identified after the data have already been collected. Retrospective studies are often conducted when the outcome is not very common and when it would require a long time to follow subjects STATISTICS prospectively. In public, health, retrospective studies are usually conducted as Statistics is a mathematical science pertaining to the collection, classification, case-control studies. In a case-control study, case subjects (those having the analysis, interpretation or explanation, and presentation of data or facts, for outcome) and control subjects (those not having the outcome) are identified. drawing inferences based on their quantifiable likelihood (probability). Existing data are obtained to determine what factors were related to subjects Statistics helps in business forecasting, decision making, quality control, becoming either a case or a control. (Further study of an identified outcome) search of new ventures, study of market, study of business cycles, useful for planning, useful for finding averages, useful for bankers, brokers, insurance, CROSS-SECTIONAL STUDY etc. In a cross-sectional study, data are collected at a particular time point and represent a cross-section time. The outcome and the variables of interest are all BIOSTATISTICS measured at the same time. Surveys that measure the responses of subjects at BIO means life, while STATISTICS refers the collection, classification, analysis, a particular point in time are typically conducted as part of a cross-sectional interpretation or explanation and presentation of data. The term study. Many times, cross-sectional studies are limited in their conclusions BIOSTATISTICS, therefore, refers to the application of statistical methods to the because the data re only collected at a single point in time. (Result/outcome are life sciences like biology, medicine, and public health. being compared to the other time form a same respondent) BIOSTATISTICS AND EPIDEMIOLOGY The discipline of epidemiology and biostatistics are essential to achieving the VARIABLES goals of public health, and combining these two disciplines in a single - An observable characteristics or phenomena of a person or object whereby department creates synergies for both training and research. the members of the group or set vary differ from one another. Epidemiologists study the distribution and determinants of health and - Are considered raw data or materials gathered by a researcher or disease in populations. investigator for statistical analysis usually expressed by the symbols X,Y,Z, Biostatisticians develop and apply statistical theory, methods and etc. (The immediate data) techniques to public health research data and the planning, CATEGORICAL/DISCRETE – variables whose measurements represent implementation and evaluation of public health programs. a limited set of possible values, such as the child being defined as Given the string overlap, epidemiologists and biostatisticians often obese or non-obese. (Categorical = words; Discrete = whole number) collaborate to work toward the shared goal of generating and analyzing ORDINAL VARIABLES – are categorical variables with different data to advance the public’s health. levels or categories whose order matter. (e.g. rankings, positions) SUBJECT is the who or what needs to be studied. DATA are obtained by NOMINAL VARIABLES – are categorical variables with different measuring the characteristics of the subjects. A POPULATION is the entire levels or categories whose order does not matter. group of individuals you want to study. And a SAMPLE is a subset of that group. DICHOTOMOUS VARIABLES – are a very special kind of A SURVEY is a data collection activity involving a sample of the population. A categorical variable that can have only two levels (e.g. Yes or CENSUS collects information about every member of the population. no, True or False). CONTINOUS – variables whose measurements represent an unlimited set of possible values, such as the child’s BMI measured in kg/m2. (e.g. SAMPLE height, weight, etc). It is also numerical but can and cannot be a whole If only certain members of the population are chosen so that the sample number. systematically represents the population, the sample is biased sample and may - Can have only a numeric variable. They are called continuous not be effective for investigating the research question. because there are no natural gaps between the numbers. And the level of detail measure by a continuous variable is limited only by Voluntary Response – allowing subjects to select themselves for the sample. the level of detail of the measuring instrument. Convenience sample – selecting subject that are convenience to the COUNT VARIABLES – are variables that can take only positive whole investigator. number values. Count variables are considered discrete because values in between whole numbers count does not occur. RANDOM SAMPLE The strategies for choosing a random sample depend on the research question and how feasible it is to implement the randomization. NUMERICAL SUMMARIES SIMPLE RANDOM SAMPLE – means that every case of the population For categorical variables or for variable with a limited number of possible has an equal probability of inclusion in sample. values, numerical summaries include: STRATIFIED SAMPLING – is where the population is divided into strata COUNTS: For each level or category, a subject either belongs in a or subgroups, and a random sample is taken from each subgroup. category or not. SYSTEMATIC SAMPLING – is where every nth case after a random start PROPORTIONS: The proportion is simply the count for a category is selected. (e.g. before selecting there is a chosen certain number, divided by the total number of subjects. then the person who entered chronologically into that specific number PERCENTAGE: The percentage is the proportion times 100. will be interviewed). Each type of random sample requires a list of the population known as the EXAMPLE: sampling frame. COUNT: 50 (total number of respondents) PROPORTION: 26/50 (fractions; subgroup/total number of respondents) PERCENTAGE: 52% (percentage of the category/subgroup over the total STUDY DESIGNS respondents) The study design is how information on the subjects will be collected. For continuous variables, there are may options for summarizing the responses PROSPECTIVE STUDY numerically. Typically, numerical summaries of continuous variables are the Subjects are identified and followed for a specific period of time. Data collection mean, standard deviation, median, first and third quartiles, and minimum and starts at the beginning of the study and continues as the subjects are followed maximum. during the study. The investigator controls what variables are measured and how they are measured. In public health, prospective studies are often conducted when the goal is to compare groups. COHORTS – in public health, cohorts are the groups with particular characteristics are identified at the start of the study, then followed to determine whether a particular outcome occurs. COHORT-STUDY – is a prospective study involving the comparison of cohorts is called a cohort-study. CLENT J. JANDONGAN Indicates a set of observations with lower values compared to the majority. NUMERICAL SUMMARIES FOR DESCRIBING CONTINOUS VARIABLES - Right Skewed Numerical Symbols Measures How to find it The distribution has a longer tail to the right. Summary Indicates a set of observations with higher values compared to the Mode x Center Most common response majority. Mean Center Sum of responses divided by the X number of responses POISSON DISTRIBUTION Median x Center Middle value of the responses - A discrete probability distribution whose possible values are whole Variance S2 Spread Mean squares distance of numbers from 0 to infinity, which is not necessarily symmetric like the responses from the mean normal distribution. Standard √𝑆 Spread Square of the variance Deviation - When the mean is small, the distribution is somewhat skewed. However, as Range R,r Spread Maximum minus minimum the mean increases, the distribution becomes more symmetric. First Quartile Q1 Spread Middle value of the first half of the responses Third Quartile Q3 Spread Middle value of the last half of the responses VARIABILITY WITHIN AND BETWEEN SUBJECTS Interquartile IQR Spread Third quartile minus first quartile Range VARIABILITY Minimum Min Spread Lowest value Variability can be defined and described in many ways. Maximum Max Spread Highest value Variability in measurements occurs when multiple measurements are taken on a subject. If there is little measurement variability, the measurement has reliability. PARAMETERS AND STATISTICS Variability also exists between subjects. In any study, the subjects are Parameters are actual numerical summaries that exist. The specific value is generally not the same. Subject-to-subject variability is one of the just not known, which is why a study was conducted in the first place. This primary reasons numerical summaries are necessary, because not describes the numerical summaries of the population. every subject is the same. Researchers need a systematic approach to A statistic is the same numerical summary as the parameter but provides a describe average measurements on subjects as well as how similar summary of the sample. This describes the numerical summaries of the these measurements are. sample. VARIABILITY BETWEEN SAMPLES GRAPHICAL SUMMARIES Variability between samples refers to the differences or fluctuations in the The distribution of a variable consists of a summary of the possible values the characteristics of different samples drawn from the same population. This variable can have and the number of subjects with each of these values. variability can arise due to several factors, including natural differences within Frequency distribution – is a distribution that uses count to distribute population, sampling methods, and random errors. Understanding this concept the number of subjects with a particular value. is crucial in statistics and research as it impacts the reliability and Probability distribution – is a distribution that uses proportions to generalizability of the results. describe the number of subjects with a particular value. The Central Limit Theorem Categorical If the means obtained from all possible samples are summarized in a histogram, - Because categorical variables have only limited number of possible values, The shape will be unimodal and asymmetric or normally distributed. the distribution can be displayed with either a table or a picture. This table The center of the sampling distribution will be the true population can be converted to a picture, which is more meaningful than the table of mean. values. The distance between the center and the point of curvature will - A pie chart, as the name suggests, looks like a pie. Each level of categorical represent one standard deviation, which is equal to the standard error variable is a slice of pie, and the size of the slice represents the number of of the mean. the subject in that category. A bar graph is a graph whose vertical axis The characterization of all sample means is known as the central limit theorem. represents the number of times a value occurs, and whose horizontal axis represents the possible values of the categorical variables. ESTIMATION - Variables with only two levels are very common and have nice properties. The probability distribution that occurs when dichotomous variables are measured on subjects in a sample given a particular name, the BINOMIAL Margin of Error DISTRIBUTION. - The method for creating the interval estimate so that the interval has a good chance of hitting the parameter of interest involves statistical inference. - Bernoulli distribution – is when a variable has only possible outcomes, only The statistic from a single sample can be made bigger by adding and has two possible outcomes. subtracting an amount, the margin of error, on either side of the point estimate. The sampling distribution can be used to determine how much CONTINOUS need to be added or subtracted on either side of the estimate to hit the true - Histogram provides a quick picture of the distribution of a variable like the parameter. graphs of the discrete variable. - Like discrete variables, when counts are used on the vertical axis, the Confidence Intervals distribution is referred to as a frequency distribution. - The sampling distribution consists of all possible statistics and how often - The histogram is called a probability distribution when a vertical axis these statistics occur. consists of proportions. Unlike the distribution of the discrete variable, - Because the sampling distribution is a normal distribution, the empirical however, there are no gaps between the bars of the histogram. rule can be used to determine the number of observations, that are 1, 2, and 3 standard error from the mean. DISTRIBUTION 68% of all sample means are within one standard error of the parameter 95.4% of all sample means re within 2 standard errors of the parameter NORMAL DISTRIBUTIONS 99.7% of all sample means re within 3 standard errors of the center - Distributions with bell-shaped, unimodal, and symmetric. parameter - Common in many data sets. - Accordingly, these interval estimates are often known as confidence - The most common value, the middle of the distribution, and the mean are intervals, where the level of comfort is called the confidence level. all the same. Common confidence levels are 90%, 94%, and 99% for a normally distributed distribution. SKEWED DISTRIBUTIONS - Left Skewed The distribution has a longer tail to the left. HYPOTHESIS TESTING CLENT J. JANDONGAN A research hypothesis is a statement of expectation or prediction that will be tested by research. - A hypothesis can be defined as a tentative explanation of the research problem, a possible outcome of the research, or an educated guess about the research outcome. (Sarantokas, 1993) ----- TOPIC 3 ----- - Hypothesis is a formal statement that represents the expected relationship between an independent and dependent variable. (Cresswell, 1994) Null Hypothesis – denoted as (H0)(µ0) it is the hypothesis that state CONTINUOUS DATA: CORRELATION AND REGRESSION equality statement. Alternative hypothesis – denoted (H1)(µ1) it is the hypothesis that state GRAPHICAL SUMMARIES non-equality statement. - An association between two variables is a measure of how much one - The P-value for any given hypothesis test is the probability of getting a variable change when the other variable changes. sample statistics at least as extreme as the observed value. The proportion The association is positive if, one variable increases as the other of statistics that are even farther from the null parameter than the observed variable increases. statistic is called the p-value. The association is negative if one variable decreases as the other When the p-value is small, the observed statistic is rare and provides variable increases. evidence against the null hypothesis. Scatterplot - is used to describe the relationship when investigating the When the p-value is large, the observed statistic is common and does association between two variables. In a scatterplot, each observation is not provide sufficient evidence against the null hypothesis. presented by a single point. The point represents a subject’s value for each of the variables. If there are two variables, a point represents the measurement of the subject in variable 1 and variable 2. The plot of all the TYPE 1 ERROR pairs of measurements for each observation result in a scatter plot. Even when a small p-value is obtained, the null hypothesis may still be really true. When a statistic provides evidence against the null parameter, but the null NUMERICAL SUMMARIES: CORRELATION parameter is really the true parameter, a Type 1 error is made. - When two variables can be described by a line, their relationship is referred A type 1 error occurs when the null hypothesis is rejected even though to as being linear. To describe the strength of a linear relationship, a it is really true. numerical summary called correlation is used. The strength of the linear relationship between two variables is measured by the correlation. A correlation ranges from [-1 to 1] TYPE 2 ERROR Strongest positive relationship results in a correlation of 1. If the research hypothesis is true and the null hypothesis is not rejected, a Strongest negative relationship results in a correlation of -1. mistake is made. This mistake is called a type 2 error. When the significant level Twi variables that have no linear association at all gave a is set to a very small value, then it is more likely that a type 2 error will be made. This, as the chance of a type 1 error decreases, the chance of type 2 error correlation of 0. increases. INFERENCES FOR THE CORRELATION COEFFICIENT A type 2 error occurs when the null parameter is not the true parameter, Scatterplot and correlation coefficients can be very helpful in describing the but the null hypothesis is not rejected. relationship between two continuous variables. However, these summaries describe only the sample. To determine whether there is evidence of an association between the variables, hypothesis tests and confidence intervals POWER can be used. The goal of any hypothesis test is to have a sample with good power. That is, a sample with a good chance of supplying enough evidence so that an incorrect Hypothesis testing - takes into account the variability that occurs between null parameter can be rejected as the true parameter. The probability that the different samples of the same size and helps in determining whether the null hypothesis will be rejected when it is indeed false is called power. Power is statistics merely happened by chance or happened because the true the opposite of the probability of a type 2 error. A study with good power has power of 80 to 90%. If a study has 90% power, this means that there is a 90% correlation is not zero. chance that a false null parameter will be rejected as the true parameter. SIMPLE LINEAR REGRESSION Although determining whether two variables are correlated and estimating the PLANNING STUDIES direction of the association is helpful, generally, an investigator is trying to explain how one variable impact another. In public health, research questions If the purpose of the study is estimation, then the goal is to provide a precise often arise when the goal is to try to understand why one person is different from estimate of the outcome, and confidence intervals can be used to determine one another. sample size. This is often the case in exploratory or observational studies. If, however, the purpose of the study is testing, then the goal is to test some hypotheses, significant levels, and power are necessary for estimating the DEFINING THE LINEAR RELATIONSHIP When discussing correlations, the relationship between two variables was sample size. It is often in the case of clinical trials, experiments, and described as linear, meaning that a line could be used to represent how the two confirmatory studies. variables were associated. When two variables appear to demonstrate a linear relationship, a line can be used to model how the response variable related to the explanatory variables. ESTIMATION STUDY When the goal of the study is estimation, the study is likely to involve only one THE ANOVA TABLE group of interest. In these studies, the objective is generally to estimate mean, In an ANOVA analysis, the goal is to divide the variability of the outcome into the proportion, or rate. Providing an estimate of the parameter means providing a confidence interval. The precision of the confidence interval is the same as its variability that can be explained by the explanatory variable and the variability that cannot be explained. The partitioning of the variability with linear regression width. A parameter is estimated with high precision when the confidence is very similar to the partitioning of the variability when comparing multiple interval is narrow. A parameter is estimated with low precision when the confidence interval is wide. groups. The term “between” is replaced by “model”. OVERALL HYPOTHESIS If the model does a good job of explaining the variability in the response, the TESTING STUDY When the goal of the study is to test a hypothesis, the study is likely to involve variability attributable to the model is larger than the variability of the error, more than one group. In public health, testing hypotheses often entails suggesting the explanatory variable has a linear relationship with the response. To determine whether the variability explained by the model is large enough to comparing groups. In these studies, the objective is generally to perform a test claim that there is a linear relationship, the ratio of the mean-squared model to determine whether a parameter is different from some value. The difference in means is not zero or a ratio is not one. Calculations for the sample size and the mean-squared error is calculated. depend on the research question and the parameter of interest. CLENT J. JANDONGAN SPECIFIC HYPOTHESIS TEST FOR SLOPE MULTIPLE LINEAR REGRESSION After establishing that there is a linear relationship between variables and the When multiple variables might explain differences in the response variable, specific slope associated within the variables can be investigated, a positive multiple linear regression can be used. The underlying concepts of multiple slope suggests that increases in variable 2 are related to the increase of variable linear regression are very similar to those of simple linear regression. The main 1, whereas a negative slope would suggest that the decrease in variable 2 are difference is the number of explanatory variables. Simple linear regression related to the decrease in variable 1. A slope of 0 would suggest no linear involves only one explanatory variable, but multiple linear regression involves relationship. multiple variables that can help explain differences in the response. Often, the multiple variables in the multiple linear regression are referred to as regressors. SPECIFIC CONFIDENCE INTERVAL FOR SLOPE A regressor is any variable that appears on the right-hand side of the model, The point estimates indicate the slope of a sample, but to use this point equation, or any variable that is associated with a slope in a linear regression estimate in estimating the true population slope, confidence intervals are model. needed. Confidence intervals are based on the idea of sampling distributions and the fact that not all samples result in the same statistic. The sampling SPECIFIC HYPOTHESIS TEST FOR SLOPES distribution is a t-distribution with the same degrees of freedom as the mean In a multiple linear regression, the model connects multiple regressors to a squared error and can be used to obtain confidence intervals. single response, and a datapoint is comprised of all three variables. All three of these variables are used in the least square regression simultaneously. So, all PREDICTION EQUATION three variables are used to obtain estimates of the slopes and intercepts. The regression line is appropriate only for predicting values in the range used to create it. REGRESSORS DO NOT HAVE TO BE CONTINUOUS When thinking of a linear regression, some tend to think that all the variables must be continuous or ordinal. This is due in part to the interpretation of the To avoid unreasonable predictions, possible explanatory values must be limited slopes. As the regressor increases by one unit, the change in the response is the to one similar to those used to find the regression line. slope. However, multiple linear regression is more flexible than this. In fact, all that is required is that the response comes from an approximately normal DIAGNOSTICS distribution. The regressors can be any kind of variable, continuous, ordinal, Statistical models are functions that define how the explanatory variable can nominal, dichotomous. Although the interpretation of the slope corresponding explain changes in the response variable. Models provide a way for investigators to these different types of variables may be different, each type of variable is to understand how the data might have been produced. Therefore, a model is allowed to be considered in the model. useful only if it is appropriate for the data or if the data fits well. To determine whether a linear regression is the most appropriate model, check the DUMMY VARIABLES assumptions. When a categorical variable is converted into multiple dichotomous variables, they are known as dummy variables. A dummy variable only takes on values of 1. The subjects are independent. only 0 or 1. The slope associated with a dummy variable can be interpreted as 2. The errors come from a normal distribution that has a mean of zero and the mean change in the response between two groups. When a categorical a constant variance. variable is converted to dummy variables, the slope associated with each dummy variable is interpreted as the mean change in the response between a Assumptions on these error terms can be checked by considering the residuals. group and a reference. The reference group is a group that was not converted to a dummy variable and that was not included in the model. VIOLATIONS TO CONSTANT VARIANCE Constant variance means that the response follows the regression line equally CONFOUNDING well for different values of the explanatory variable. If a scatterplot of the Some regressors are included in the regression model not because they are residuals versus explanatory variable exhibits a fan shape, the assumption of particularly interesting, but because they might interrupt the relationship constant variance has been violated. A fan shape results when the variability of between response and explanatory variables. Including these variables in the the residuals is different depending on the value of the explanatory variable. model allows the investigator to control or adjust their effects. Variables that One way to lessen the fan shape in the residuals is to stabilize the variance by are not of primary importance but that are collected and included in the model transforming the response variable. Some common transformations include a because they could potentially influence the relationship between the response natural log and a square root. and explanatory variables are called confounding variables. VIOLATIONS OF NORMAILITY VARIABLE SELECTION The best way to verify whether the assumption of normality has been violated is If performing a primary data analysis, the problem is a little more to investigate plots of the errors. A histogram of the residuals provides an straightforward because the data are collected to answer a particular question. indication of whether the distribution of the residuals is skewed and not However, if performing a secondary data analysis, the problem can be normally distributed. A skewed distribution is possible when the data contains considerably more complicated. There are two main reasons for performing a outliers, which are points that lie outside the pattern of other datapoints. If an regression analysis, forecasting or prediction, and covariate adjustment. The outlier is suspected, run the regression analysis with and without the suspicious strategies employed in variable selection depend on the purpose. data point. Although removing data points from the analysis is never desirable, seeing what the analysis would be when outliers are removed can be helpful. Another type of extreme point that may impact the normality assumption are influence points, which are points with extreme explanatory values. They are particularly troublesome because the least square regression line is created by minimizing squared errors. If a point influences the regression line by pulling it away from the other data points and closer to itself, the resulting regression line may not be as accurate as it could be in describing the linear relationship between the explanatory and response variables. VIOLATIONS OF LINEARITY Simple linear regression is, by definition, the process of using a line to summarize the relationship between the response and explanatory variables. If a line does a poor job of describing the relationship, then the assumption of linearity has been violated. The assumption of linearity can be checked simply by viewing the scatter plot of the response and the explanatory variables to see whether it demonstrates a linear pattern. If it does, then the points should line up well along the line. If, however, the relationship is not linear, then a line may do a poor job of explaining the relationship between the explanatory and response variables. CLENT J. JANDONGAN