Computational Molecular Microbiology (MBIO 4700) PDF
Document Details
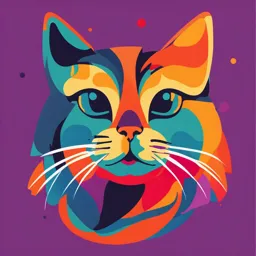
Uploaded by ArticulateBowenite6305
University of Manitoba
Abdullah Zubaer
Tags
Summary
This document is a lecture presentation on computational molecular microbiology (MBIO 4700 at the University of Manitoba). It covers topics like working with sequences, phylogenetic concepts, evolutionary rates, and different models of evolution, as well as examples illustrating the principles and approaches.
Full Transcript
Computational Molecular Microbiology (MBIO 4700) ABDULLAH ZUBAER UNIVERSITY OF MANITOBA Working with sequences Sequence databases Sequence comparison • Pairwise alignment • Multiple sequence alignment Phylogenetic tree Phylogenetics Leaf Leaf Leaves Branches Branch length Nodes (ancestors) Cl...
Computational Molecular Microbiology (MBIO 4700) ABDULLAH ZUBAER UNIVERSITY OF MANITOBA Working with sequences Sequence databases Sequence comparison • Pairwise alignment • Multiple sequence alignment Phylogenetic tree Phylogenetics Leaf Leaf Leaves Branches Branch length Nodes (ancestors) Clades (share a common ancestors) Root (or outgroup) https://socratic.org/questions/what-is-represented-by-the-base-root-of-a-phylogenetic-tree Branch length (and scale bars) Genetic change (substitutions/site) = Evolutionary rate x (substitutions/site/year) Divergence time (years) D – low evolutionary rate A diverged from B recently from a common ancestor https://www.ebi.ac.uk/training/online/course/introduction-phylogenetics/what-phylogeny/relating-distance-rate-and-time Phylogenetics • Evolution of life (tree of life) • Origin of infections diseases • Function based on homology • Evolution of protein / gene families • Gain understanding of genomes and their composition (evolution) etc. Phylogenetic inference: A “simplistic overview” DNA sequence analysis Assumptions: 1. each site evolves independently 2. different “lineages” evolve independently (accumulation of Neutral mutations – no phenotype) 3. rate of change for each site should be the same What are we looking for? Change over time: Gradual accumulation of nucleotide substitutions within each lineage Change: Different types Of Substitutions http://users.ugent.be/~avierstr/principles/phylogeny.html Substitutions Are all “changes” visible? Are all changes equal? (noncoding, coding, position of change within a codon etc.) Any possibility of homoplasy? -> simplest approach “count differences” OR use Models (ML) OR use Cladistics (PARS) Nemesis: homoplasy A potential problem with sequence data “convergent evolution” ◦ Some amino-acids at some position but not due to ancestry (function is a strong driver towards similar or identical amino-acids) ◦ Structural constraints (for ribozymes or RNA structures) Change and probabilities Protein coding sequences ◦ Codon: XYZ are all positions in a codon “equal” And non-protein coding sequences ◦ rDNA Independence of all “sequence” characters Example rDNA “compensatory substitutions” ◦ Change at one location “promotes” change at another location to maintain function ◦ i.e., not all positions evolve independently 5.8S gene ITS1 and ITS2 Think in “3D” --------------N------------------------------A G Change (events): 6 possibilities – for any one “spot” BUT are they all equally “likely” Probabilities vs likelihoods Need evolutionary models and “priors” – C T Priors = information about the sequences that may help in predicting what is more likely (based on probabilities) Two “possible” or likely explanation Two different probabilities Likelihood And Probabilities “prior” – Gremlins do not exist p = 0 For N = (if A is ancestral - ?) A to A what are the probabilities ? A to C A to G A to T Evolutionary rates Gene's “rate” of sequence evolution (a fundamental evolutionary quantity): Evolutionary rates determined by what? (NOT Obvious) Different genes evolved at different rates. Scientific debate: the following “seven predictors are studied” Seven predictors (protein coding genes) gene expression level dispensability (essential or redundancy alternative genes available) protein abundance codon adaptation index (~codon usage and regulation) gene length number of protein-protein interactions gene's centrality in the interaction network What determines the evolutionary rate: No easy answers! Comparative genomics and comparing rates of evolution among orthologs: Translation efficiency is an important factor (codon bias/usage) Duplication Orthologs A B Paralogs Homologous Genes Duplication HGT = horizontal gene transfer HGT A Xenologs B C D Codon usage and GC content maybe different for Xenologs Early globin gene Gene Duplication -chain gene mouse human cattle ß-chain gene cattle ß Orthologs Paralogs Homologs human ß mouse ß paralogs Mitochondrial Porins (GENE TREE) (Voltage-dependent anionselective channel ->VDAC) Example: Zea has several VDACs (showing up in different parts of the tree) Young MJ, Bay DC, Hausner G, Court DA. The evolutionary history of mitochondrial porins. BMC Evol Biol. 2007 Feb 28;7:31. doi: 10.1186/1471-2148-7-31. paralogs (Young et al. 2006) “recent paralogs” Species tree vs Gene tree Orthologous and paralogous sequences -examples globin genes, EF-TU and EF-G, VDACs (porins) -upon duplication paralogs may either degenerate (drift) or gain a new function or become a more specialized version of the original (either way paralogs evolve in their own lineage) Phylogenetic inference summary: The “simplistic overview” is NOT realistic. Assumptions: 1. each site evolves independently 2. different “lineages” evolve independently (accumulation of Neutral mutations – no phenotype) 3. rate of change for each site should be the same Models of evolution There are many! Essentially there are biases in what happens over time: GC biases or transitions vs transversions; “trends” to suggest a shift towards A’s, codon positions etc. Coding (protein coding) vs rDNA regions DNA segments that are not transcribed Repetitive DNA etc. Substitution models are statistical models that are supposed to correct for biases in the way sequences evolve/change over time. DNA – substitution models Common mutational models for DNA (applied in phylogenetics): Jukes-Cantor (JC): all mutations equally likely Kimura 2-parameter (K2P): transitions more likely than transversions Felsenstein 84 (F84): K2P plus unequal base frequencies Generalized Time Reversible (GTR): most general usable model http://evomics.org/resources/substitution-models/nucleotide-substitution-models/ Yang Z, Rannala B. 2012. Molecular phylogenetics: principles and practice. Nat Rev Genet. 13(5):303-314. doi: 10.1038/nrg3186. GTR Rate matrix – for the 6 possible events with regards to nucleotide sequences: Consider the overall number of substitutions per unit time. A G C T Calculate the number of parameters*: Allows for back mutations, convergent parallel – and multiple substitutions. *Consider: 6 substitution rate parameters and 4 equilibrium base frequency parameters Amino acid sequences vs nucleotide sequences Evolutionary changes in amino-acid sequences: #1: #2: G G G A A A V K R H G A A V V A V K R W Invariant position (Identity) Variable residues Conservative replacement (G = gly; A = ala; V = val; K = lys; R = arg; H = histidine; W = trp) general properties: G, A, V, = non-polar; ~ low MW, aliphatic R groups; K, R, H, = polar, + charged R groups; W = aromatic; ~ non-polar; high MW; Amino acid sequences encoded by DNA sequences: (Genetic code - “degenerate”) #1: G GGU G GGC G GGA A GCU A GCC A GCG V GUU K AAA R AGG H CAC #2: G GGG A GCC A GCA V GUU V GUC A GCC V GUU K AAG R CGA W UGG Example of synonymous substitution Example of nonsynonymous substitution Need a scoring matrix to evaluate distances or probabilities Generating phylogenetic trees Phylogenetic tree building methods ▪ Distance methods (calculate a distance matrix) ▪ Character state methods (Cladistics and Parsimony) sharing of derived characters ▪ Maximum Likelihood - probability of characters evolving (from ancestral sequences to “leaves”) ▪ Bayesian Analysis - probability of characters evolving (but “inverse” or posterior probabilities; we know the end result but how did we get there?) Yang Z, Rannala B. 2012. Molecular phylogenetics: principles and practice. Nat Rev Genet. 13(5):303-314. doi: 10.1038/nrg3186. Yang Z, Rannala B. 2012. Molecular phylogenetics: principles and practice. Nat Rev Genet. 13(5):303-314. doi: 10.1038/nrg3186. Phylogenetic analysis (in the real molecular evolution world): Simplistic “models”: NCBI/Clustal-X (tree options) If you want to include a phylogenetic tree in a publication – more sophisticated methodologies are expected. PHYLIP/PAUP/MEGA etc. : Distance Methods -pairwise comparisons - genetic distances Maximum likelihood methods (-probabilities) Parsimony Methods -the tree that requires the least number of evolutionary changes -character states & “Bootstrapping” https://isogg.org/wiki/Phylogeny_programs http://evolution.genetics.washington.edu/phylip.html PHYLIP Distance and “Probability (ML)” based methods -pairwise comparisons - genetic distances -all differences are used (in distance methods) what biases (for ML) can be detected? -What model (for ML)- calculation/formula - to use? -DNA (only 4 possible nucleotides) -transitions vs transversions -back mutations? -homoplasy How to account for these? (ML tries to address these) ML = maximum likelihood Distance and ML for ML – “ancestral sequences are inferred” (character states) probabilities for changes from the nodes to the leaves are calculated -> Modeltest (Bioinformatics 1998; 14: 817-818. -> FINDmodel (online); Best model - MEGAX -> ProtTEST (Bioinformatics 2005; 21: 2104-2105 Distance: -”clusters” sequences based on genetic distances (counting differences) MOST popular: NJ (neighbor joining) – fast For ML: tree with overall best probability is assumed to be correct Ancestral nodes Sequences 4 mutations ML 7 mutations Which tree is correct or the “best”/more likely? Lesk, A.M. (2005) Introduction to Bioinformatics 2nd Ed. Maximum Likelihood (ML) Maximum Likelihood (ML) - apply evolutionary models -complex - sequences added separately to the tree and for each site (change) a probability is generated to evaluate how likely such a mutation would have occurred (based on an evolutionary model) at the ancestral node. (~character state) -sum of all probabilities (multiplied together) gives the overall probability for the tree -> Find the tree that fits the data! - the tree with the ML - highest probability is viewed as best possible tree - VERY SLOW - Many trees are built during this process and evaluated based on the ML criterion - get one ML tree Parsimony Methods -Character states- differences are examined for their degree of being informative (informative or non informative). -Program tries to reconstruct ancestral nodes - based on shared derived sequence characters (~ Cladistic approach). -Many possible trees can be constructed but the tree(s) that requires the least number of evolutionary changes (substitutions) is (are) assumed to be correct. Criticism: - “ Simplistic” and what is “non informative”? Maybe has some information that is getting ignored. Reality check – need at least 4 sequences A B C D aat ... ... ... 1 tcg ..a ..a ..a 2 ctt ..g ..c ..a 3 cta ..a ..c ..g 4 gga .t. ... ..g atc ... ..t ..t 5 tgc ... ... ... cta t.. ... t.t 6 atc ... ... ..t ctg ..a t.a t.. 7 1 invariable 2 not informative (only unique to one member) 3 highly variable (not informative) 4 not very informative 5 6 7 all informative these suggest clear relationships with respect to all 4 members in the analysis Looking for shared derived characters [synapomorphies] Ancestral nodes Sequences 4 mutations If based on informative sites? Lesk, A.M. (2005) Introduction to Bioinformatics 2nd Ed. 7 mutations Which tree is correct or the “best”/more likely? OR most parsimonious? Summary – tree methods • *“Distance based methods (eg. Neighbour Joining (NJ)): find a tree such that branch lengths of paths between sequences (species) fit a matrix of pairwise distances between sequences. • Maximum Parsimony: find a phylogenetic tree that explains the data, with as few evolutionary changes as possible. • Maximum likelihood : find a tree that maximizes the probability of the genetic data given the tree.” http://www.ihes.fr/~carbone/MaximumLikelihood2.pdf Bootstrapping Testing a tree topology How “good or reliable” is my tree or parts of my tree? Phylogenetic tree is a “representation” of the data (alignment); i.e. - the genetic variation within your alignment suggests certain relationships. How much “information” (characters) is actually present in your data set to support your tree? MORE bootstrapping -“randomize the data set” to generate pseudoreplicates of the original data set. -“pull characters from original data set randomly - some characters get pulled more then once others get missed, final data set = in size to original -keep going till you made ~ 1000 “bootstrap replicates” NOW analyze them all - collect all the trees (1000 or more) and obtain the “consensus tree” Example: Original data set: 1 2 3 4 1 C T T T 2 G G A T 3 G T A A 5 A T T 6 A T T Resample: (usually 1000 times or more) 5 possible bootstrap replicates: 1 2 3 1 2 3 66 AA TT TT 33 TT AA AA 45 TA TT AT 22 TT GG TT 55 AA TT TT 61 AC TG TG 33 TT AA AA 66 AA TT TT 12 CT GG GT 44 TT TT AA 11 CC GG GG 23 TT GA TA 55 AA TT TT 22 TT GG TT 34 TT AT AA etc.......(each sample has same number of characters as original sample) Argument: if there is a trend in a data set, then resampling should not “dilute” the trend. So, each bootstrap replicate now has to be analyzed and all “trees are then compared”. (--->Consense program) Usually, to be significant 95 % of bootstrap replicates should show the trend in order to be significant (but lower numbers are also accepted ~ “moderate support”) Majority rule consensus tree Phylogenies and bootstrap support (node support) NODE support Values: Branch length based on NJ and Kimura 2-parameter distance model Peng et al. 2009. BMC Genomics 10:247 doi:10.1186/1471-2164-10-247. Tree based on various nuclear markers.