Data Science Methodology - Fall 2024 PDF
Document Details
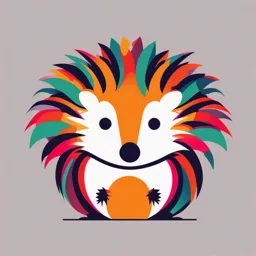
Uploaded by JoyousChrysanthemum
2024
Mohamed Kholief
Tags
Summary
This document is lecture notes on data science methodology, focusing on the data science pipeline, CRISP-DM, and various data acquisition methods. The Fall 2024 lecture notes include topics like data understanding, data acquisition methods, and common pitfalls.
Full Transcript
Data Science Methodology Prof. Dr. Mohamed Kholief ([email protected]) Fall 2024 Lecture 2: Understanding the Data Science Pipeline Business Understanding, Data Acquisition, and CRISP-DM Learning Objectives Gr...
Data Science Methodology Prof. Dr. Mohamed Kholief ([email protected]) Fall 2024 Lecture 2: Understanding the Data Science Pipeline Business Understanding, Data Acquisition, and CRISP-DM Learning Objectives Grasp the phases of business understanding and data understanding. Understand different data acquisition methods and data types. Learn about the CRISP-DM methodology and its significance in data science projects. Recognize the importance of aligning data science projects with business goals. Recap of Lecture 1 Brief overview of Data Science Methodology. Data Science Life Cycle stages introduced. Importance of problem formulation and the role of a data scientist. Business Understanding Phase Definition: Translating business objectives into data science goals. Key Activities: Identifying business problems and opportunities. Defining project objectives and success criteria. Assessing the feasibility of the project. Importance: Ensures that data science efforts are aligned with organizational goals. Data Understanding Phase Definition: Initial exploration and analysis of data to understand its quality and relevance. Key Activities: Data collection from various sources. Data exploration to identify patterns, anomalies, and relationships. Assessing data quality and completeness. Importance: Provides insights into the data's suitability for addressing the business problem. Data Acquisition Methods Primary Methods: APIs (Application Programming Interfaces): Accessing data from web services. Web Scraping: Extracting data from websites. Databases: Querying structured data from SQL/NoSQL databases. Public Datasets: Utilizing available datasets from repositories (e.g., Kaggle, UCI). Considerations: Data accessibility and permissions. Data format and structure. Frequency of data updates. Structured vs. Unstructured Data Structured Data: Organized in fixed formats (e.g., tables, spreadsheets). Easily searchable and analyzable. Examples: SQL databases, CSV files. Unstructured Data: No predefined format or organization. Requires more processing to extract meaningful information. Examples: Text documents, images, videos, social media posts. Semi-Structured Data: Contains some organizational properties (e.g., JSON, XML). Challenges in Data Acquisition Data Quality Issues: Missing values Inconsistent data formats Duplicates and errors Data Integration: Combining data from multiple sources Ensuring compatibility and consistency Scalability: Handling large volumes of data Ensuring efficient data processing Introduction to CRISP-DM Definition: Cross-Industry Standard Process for Data Mining. Phases of CRISP-DM: Business Understanding Data Understanding Data Preparation Modeling Evaluation Deployment Significance: Provides a standardized framework for managing data science projects. CRISP-DM cyclical diagram Detailed Look at CRISP-DM Phases Business Understanding: Define objectives and requirements from a business perspective. Data Understanding: Collect initial data and become familiar with it. Data Preparation: Clean and transform data for modeling. Modeling: Apply various modeling techniques to the prepared data. Evaluation: Assess the model's performance and ensure it meets business objectives. Deployment: Implement the model in a production environment. Benefits of Using CRISP-DM Flexibility: Applicable across different industries and projects. Structured Approach: Ensures all critical aspects of a project are addressed. Reusability: Phases can be revisited as needed, supporting iterative improvements. Communication: Provides a common language and framework for teams. Case Study: Applying CRISP-DM Scenario: Retail company wants to optimize inventory management. Application of CRISP-DM: Business Understanding: Define goals to reduce stockouts and excess inventory. Data Understanding: Collect sales data, inventory levels, supplier information. Data Preparation: Clean data, handle missing values, create relevant features. Modeling: Develop predictive models for demand forecasting. Evaluation: Validate model accuracy and its impact on inventory metrics. Deployment: Integrate the model into the inventory management system. Tools for Data Acquisition and Understanding Data Acquisition: APIs: Postman, Insomnia Web Scraping: BeautifulSoup, Scrapy Databases: SQL, MongoDB Data Understanding: Exploratory Tools: Pandas Profiling, Tableau, Power BI Visualization: Matplotlib, Seaborn Best Practices in Data Acquisition Plan Ahead: Understand data requirements based on business objectives. Ensure Data Quality: Implement validation checks during data collection. Automate Processes: Use scripts and tools to streamline data acquisition. Document Sources: Keep track of data sources and acquisition methods for transparency. Common Pitfalls in Data Science Projects Poor Problem Definition: Misalignment between business and data science goals. Inadequate Data Quality: Making decisions based on incomplete or inaccurate data. Overlooking Data Privacy: Failing to comply with data protection regulations. Ignoring Iterative Processes: Not revisiting earlier phases based on new insights. Summary Business and Data Understanding: Critical for aligning data science projects with business goals. Data Acquisition: Involves various methods and understanding data types. CRISP-DM: A robust methodology for managing data science projects effectively. Best Practices: Essential for successful data acquisition and project execution. Discussion Questions How can misalignment between business objectives and data science goals impact a project? What are some strategies to handle unstructured data effectively? How does CRISP-DM facilitate better project management in data science? Next Week Topic: Data Collection and Wrangling Techniques for collecting data from various sources. Methods for cleaning and transforming raw data for analysis.