Inferential Statistics (Chi-Square Test) PDF
Document Details
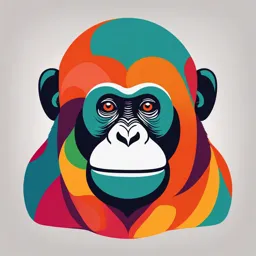
Uploaded by CourteousAlbuquerque5836
Tags
Summary
This document provides an overview of inferential statistics, focusing on the chi-square test. It covers objectives, definitions, and applications. This document is likely lecture notes or study material for a course on statistics.
Full Transcript
Inferential statistics (Chi-square test) Outlines Objectives Differentiate between descriptive & inferential statistics Types inferential statistical Tests Differentiate between null and alternative hypothesis The concept of “Hypothesis Te...
Inferential statistics (Chi-square test) Outlines Objectives Differentiate between descriptive & inferential statistics Types inferential statistical Tests Differentiate between null and alternative hypothesis The concept of “Hypothesis Testing” Define level of significance Definition of chi-square Uses and application of Chi-square test Principles of Chi-square Interpret the significance of chi-square test χ2 test. Exercises Objectives By the end of this lecture each student will be able to: Define hypothesis and level of significance Differentiate between null and alternative hypothesis Describe the concept of “Hypothesis Testing” Define chi-square Identify uses and application of Chi-square test Mention principles of Chi-square Interpret the significance of chi-square test χ2 test. Types of Statistics/Analyses Descriptive Statistics Inferential Statistics Frequencies Hypothesis Testing Basic measurements Correlation Confidence Intervals Significance Testing Prediction Describing a phenomena Proving or disproving theories Associations between How many? How phenomena much? If sample relates to the larger BP, HR, BMI, IQ, etc. population Summarize of data E.g., Diet and health through Graph or table Descriptive Statistics Descriptive statistics can be used to summarize and describe a single variable (uni- variate) Frequencies (counts) & Percentages Use with categorical (nominal) data Levels, types, groupings, yes/no, Drug A vs. Drug B Means & Standard Deviations Use with continuous (interval/ratio) data Height, weight, cholesterol, scores on a test Inferential Statistics Inferential statistics can be used to prove or disprove theories, determine associations between variables, and determine if findings are significant and whether or not we can generalize from our sample to the entire population Statistical Tests Parametric tests Non-parametric tests Continuous data Categorical or Ordinal normally distributed data The types of inferential statistics we will go over: - Parametric test T-tests ANOVA Correlation - Nonparametric test Chi-square test for independence Inferential Statistics Inferential statistics are used to draw conclusions about a population by examining the sample Accuracy of inference depends on representativeness of sample from population Random selection: equal chance for anyone to be selected makes sample more representative Researchers set the significance level for each statistical test they conduct by using probability theory as a basis for their tests, researchers can assess how likely it is that the difference they find is real and not due to chance Hypothesis Testing H0: There is no association between the exposure and disease of interest H1: There is an association between the exposure and disease of interest Hypothesis testing for independence variable (Chi Square) An inferential statistics technique designed to test for significant relationships between two categorical variables organized in a bivariate table Data organized in a contingency table – cross tabulation containing counts (frequency data) for number of observations in each category When to use it? When you want to know if there is an association between two categorical (nominal) variables (i.e., between an exposure and outcome) Ex) Smoking (yes/no) and lung cancer (yes/no) Ex) Obesity (yes/no) and diabetes (yes/no) The Chi-Square Test of Independence determines whether there is an association between categorical variables (i.e., whether the variables are independent or related). It is a nonparametric test. Chi square requirement One or more categories Independent observations A sample size of 30 observation ( people) in the table Random sampling Each cell must contain a count of 5 or more Hypothesis The null hypothesis (H0) and alternative hypothesis (H1) of the Chi-Square Test of Independence can be expressed in two different but equivalent ways: H0: "[Variable 1] is not associated with [Variable 2]“ "[Variable 1] is independent of [Variable 2]" H1: "[Variable 1] is associated with [Variable 2]“ H1: "[Variable 1] is dependent of [Variable 2]" P value Measure of probability that a result is due to chance. If the.05 level is achieved (p is equal to or less than.05), that means rejects the H0 and accepts the H1 Calculating Expected Frequencies To obtain the expected frequencies for any cell in any cross-tabulation in which the two variables are assumed independent, multiply the row and f = (column marginal)(row marginal) e column totals for that cell and divide the product by the total number of cases in the table. Determining the Degrees of Freedom df = (r – 1)(c – 1) Where r = the number of rows c = the number of columns Calculating Degrees of Freedom How many degrees of freedom would a table with 3 rows and 2 columns have? (3-1)(2-1) = 2 degrees of freedom Example Suppose we want to test for an association between smoking behavior (nonsmoker, current smoker, or past smoker) and gender (male or female) using a Chi-Square Test of Independence Based on the results, we can state the following: No association was found between gender and smoking behavior (Χ2(2)> = 3.171, p = 0.205). Limitations of the Chi-Square Test The chi-square test does not give us much information about the strength of the relationship or its substantive significance in the population. The chi-square test is sensitive to sample size. The size of the calculated chi- square is directly proportional to the size of the sample, independent of the strength of the relationship between the variables. The chi-square test is also sensitive to small expected frequencies in one or more of the cells in the table. To create a crosstab and perform a chi-square test of independence, click Analyze > Descriptive Statistics > Crosstabs.