Critical Thinking and Decision-Making with Data and AI PDF
Document Details
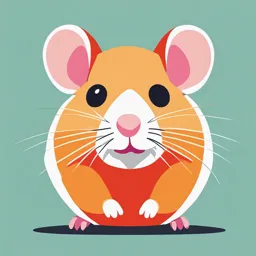
Uploaded by FlawlessFantasy4551
Tags
Related
- HCA 301 Leadership and Management in Healthcare PDF
- Critical Thinking, Clinical Judgement, and Decision Making PDF
- Critical Thinking, Clinical Judgment, and Decision Making PDF
- PPT Slides: Attention & Memory, Decision Making, Critical & Creative Thinking - Psych 172
- Critical Thinking in Nursing - PDF
- Critical Thinking & Logic Mastery - 3 Books in 1 PDF
Summary
This document provides an overview of critical thinking principles and data-driven decision-making, particularly within a contexts of artificial intelligence (AI). The text explains the importance of different facets of critical thinking, such as analytical, curious, and research-minded skills, which then influences logical & better judgments in problem solving.
Full Transcript
Critical Thinking and Decision-Making with Data and AI.. Critical thinking and data-driven decision-making are fundamental skills that help you work ethically and effectively with artificial intelligence (AI). In this module, you explore these fundamental skills, learn about decision-making and AI m...
Critical Thinking and Decision-Making with Data and AI.. Critical thinking and data-driven decision-making are fundamental skills that help you work ethically and effectively with artificial intelligence (AI). In this module, you explore these fundamental skills, learn about decision-making and AI models, and see how AI is helping organizations make data-driven decisions across diverse industries. Let’s get started. So What Is Critical Thinking?. Critical thinking is the process of analyzing, evaluating, and synthesizing information, evidence, and arguments to make well-informed, reasoned decisions. It involves the ability to think clearly, objectively, and independently, and to consider various perspectives and potential outcomes. Critical thinking leads to wise decisions and effective problem solving. Analytical. Critical thinkers break down complex issues or problems into smaller components, making them easier to understand and address. To develop this skill try to understand the problem before thinking about the potential solution. It might help to break down the problem into small chunks. Curious. Critical thinkers ask great questions (a cornerstone of critical thinking) and seek to know all components of a problem to understand how the components fit together in the big picture before thinking about solutions. Asking questions is the best way to explore potential solutions. To develop this skill seek to understand root causes of a problem and ask questions that help you discover important information. Research-minded. Critical thinkers assess the credibility, relevance, and quality of information and arguments, distinguishing between fact and opinion. To develop this skill try to understand the context in which information is presented. Consider the source, the purpose of the information, and the audience it is intended for. Open-minded. Critical thinkers are open to different viewpoints and are willing to adjust their views in the face of new information or persuasive arguments. To develop this skill be conscious of your own biases while evaluating and problem-solving. Recognizing your biases can aid in approaching information with an open and critical mindset. Logical. Critical thinkers employ sound logic and reasoning to reach conclusions and make decisions.To develop this skill try to distinguish between objective facts and subjective opinions. Facts can be verified, while opinions are personal beliefs or interpretations. The Role of Critical Thinking in Decision-Making.. Critical thinking is like having a trusted guide on your decision-making journey. It helps you gather evidence, think logically, and weigh your options before choosing a path. The qualities of being analytical, curious, open-minded, research-focused, and logical prepare you to practice critical thinking. When you apply critical thinking in decision-making, you usually do the following activities. Gather information.: Critical thinking begins with the collection of information. When you face a decision, seek out relevant data, facts, and evidence to understand the problem or opportunity at hand. Evaluate information.: Examine the gathered information closely. Distinguish between credible and unreliable sources, separate fact from opinion, and evaluate the quality of the data. This analysis enables you to make a decision based on a solid foundation. Identify assumptions.: Identify any underlying assumptions or beliefs that might influence your decision-making. Question your own preconceptions and biases and consider how these factors might affect the choice. Evaluate pros and cons.: Engage in a process of weighing the advantages and disadvantages of each option. Consider potential benefits, risks, and consequences to make a decision that aligns with your goals. Problem solve.: Decision-making often involves solving a problem or addressing a challenge. Critical thinking, with its blend of analytical and creative skills, gives you the problem-solving tools you need to break down a complex issue into manageable parts. It helps you determine which elements to focus on, and it inspires creativity in the process. This creative approach allows you to identify potential solutions and choose the most appropriate course of action. The art of asking questions is involved in each of these activities. In fact, asking questions is the cornerstone of critical thinking, as it paves the way for clarity, objectivity, and the pursuit of the most rational and ethical choices. Questions enable you to probe deeper, challenge assumptions, and uncover hidden truths. Through skillful questioning, you can dissect complex issues, separate facts from opinions, and evaluate the validity of arguments. This process not only uncovers valuable insights but also ensures that decisions are well-informed and based on a thorough understanding of the matter at hand. What Is Data-Driven Decision-Making?. You just learned that gathering and analyzing information is an important part of decision-making for critical thinkers. Data lets you make decisions based on facts rather than a best guess. To make data-driven decisions, you follow a process: Understand your goals, ask questions, gather data, study the data to find patterns, make a choice based on the patterns and trends, and evaluate the results. As you can see, critical thinking is involved at every step. Critical thinking is the ability to evaluate information, understand it, and make informed decisions based on facts. Critical thinkers are curious, ask questions, and think outside the box. They’re open to different ideas and don’t jump to conclusions. For example, an ecommerce company collects data on customer behavior, analyzes it to understand shopping trends, and uses this information to improve the user experience. It uses the information to personalize product recommendations, streamline the checkout process, offer targeted promotions, and enhance website navigation. This data-driven approach not only caters to individual customer preferences but also optimizes the website’s overall performance, leading to increased customer satisfaction and higher conversion rates. Use Critical Thinking, Data, and AI Together.. Critical thinking helps you ask the right questions, data provides critical facts and information, and AI processes the data quickly. AI is like a supercharged assistant for critical thinking. It can quickly analyze tons of data, spot patterns, and make predictions to support your decision-making. While this assistant can speed up your decision-making process, you need to be aware of potential ethical implications. When using data and AI, you need to consider ethical questions like privacy, fairness, and transparency. Critical thinking helps you make ethical choices. You’ve learned the basics about critical thinking and data-based decision-making. And you’ve seen how critical thinking plays a key role when you work with data and AI. Next, let’s delve into the art of asking great questions. Explore the Art of Asking Great Questions.. In the realm of data-driven decision-making, the skill of asking great questions is fundamental and transformative. The ability to formulate effective questions is not just an art but a science that can greatly influence the quality and depth of insights you gain from data and AI analysis. In this unit, you explore how effective questioning can help you uncover information, reveal new perspectives, and navigate the ethical complexities of decision-making with AI. “If I had an hour to solve a problem I’d spend 55 minutes thinking about the problem and five minutes thinking about solutions.” –Albert Einstein The Power of Effective Questioning.. Effective questioning is a cornerstone of critical thinking. It’s your most valuable tool to delve deeper into issues, challenges, and opportunities. In the context of data-driven decision-making, effective questioning is paramount. It allows you to go beyond surface-level information and access the hidden gems within data sets. Effective questions have the power to unlock fresh insights, challenge assumptions, and stimulate innovative thinking. Your questions act as catalysts, enabling you to explore complex issues from various angles, and ultimately, to make well-informed decisions. One of the key roles of effective questioning is in surfacing information that might not be readily apparent. In data analysis, this is particularly relevant. By asking the right questions, you can uncover correlations, trends, and patterns in data that can guide strategic decision-making. Types of Effective Questions.. Not all questions are created equal. There are various types of effective questions that you can employ to enhance your critical thinking and data analysis skills. These include open-ended questions that encourage thoughtful and detailed responses, closed-ended questions that are typically answerable with a simple one-word answer, probing questions that seek further clarification or additional details, and leading questions that subtly influence the respondent’s perspective. In the table, explore the strengths and weaknesses of these question types and when to use them. Quality Description Open-ended These questions encourage thoughtful and detailed responses, questions allowing respondents to express their viewpoints more fully. Use this type of question when you need detailed explanations behind the concept. Closed-ended Typically answerable with specific numerical answers or a simple “yes” questions or “no”, “Blue.”, or “The Great Fire of London”. These questions are useful for gathering specific, quantifiable data. Closed-ended questions are often used in multiple-choice tests or surveys, especially when computers are used to process the test or survey. Probing Probing questions seek further clarification or additional details, and questions help you delve deeper into a topic or issue. This type of question usually comes up after initial understanding of the topic. Leading These questions subtly influence the respondent's perspective, often questions steering them toward a specific answer or viewpoint. The way that you structure questions is equally important. You can frame a question in a way that encourages deeper reflection, generates a more comprehensive response, and ultimately provides a greater depth of insight. Effective structuring can turn a seemingly ordinary question into a powerful tool for uncovering hidden information. Frame Questions for Data and AI Analysis.. For data and AI analysis, the art of questioning takes on a specific and strategic role. In this context, it’s essential to formulate questions that are directly aligned with your data collection and analysis goals. That means always keeping your research or business objectives in the forefront. Without a clear link between the questions you pose and your desired outcomes, data analysis can become aimless and unproductive. The skill of framing questions for data and AI analysis has become critical in the modern data-driven landscape. Consider the world of ecommerce, where data and AI play a pivotal role in decision-making, particularly in optimizing the online shopping experience for customers. Let’s say you’re a part of a data analysis team working for a well-known ecommerce platform. The company is keen to improve its product recommendation system to boost customer engagement and sales. The current system relies on collaborative filtering, but the company is eager to harness the power of AI and data analytics to take personalization to the next level. It wants to provide customers with recommendations that go beyond the basics. By using AI and data analytics, the company aims to deliver highly tailored product suggestions that match each customer’s unique preferences and shopping habits. To achieve these tailored suggestions, analysts need to ask the appropriate questions that pertain to the specific product needs and frame those questions to align with messaging. This enhanced recommendation system not only can increase customer engagement but also lead to a significant boost in sales as customers discover products they genuinely love. Ethical Decision-Making with AI.. AI technologies have brought a new dimension of decision-making to the forefront–careful consideration of ethical implications. Now let’s look at how ethical questioning helps you navigate the complex ethical dilemmas that often arise when employing AI in decision-making processes. Ethical questioning techniques let you explore the moral dimensions of choices and actions. They allow you to comprehensively examine the ethical consequences of various decisions and can be instrumental in ensuring morally responsible decision-making. By asking the right ethical questions, you can mitigate potential harm, protect privacy, and uphold principles of fairness and justice. Picture a situation where a hospital introduces AI to help doctors diagnose complex health issues, even those that are rarely seen. This AI learns from a huge collection of medical data, including patient records, images, and clinical studies. The developers should consider ethical questions about the data the AI system learns from, how it ensures fair and unbiased decisions, and how it aligns with the current ethical standards and values in healthcare. In this scenario, the hospital wants the AI to present its reasoning to doctors so they can comprehend and have faith in its recommendations. But it understands that while AI is a useful tool that supports decision-making, it’s not a substitute for the knowledge and judgment of healthcare professionals. In the world of data-driven decision-making, where AI systems have the power to influence and automate choices, ethical questioning becomes a safeguard against unintended consequences and ethical breaches. It provides a structured and methodical approach to assess the potential impact of AI decisions on individuals, society, and the environment. In this unit, you explored the art of asking great questions and how questions are pivotal in data-driven decision-making and ethical AI usage. Next, take a look at decision-making frameworks, data-driven strategies, and AI models. Discover Decision-Making Frameworks and AI Models.. Decision-Making Models.. There are a diverse array of decision-making models and theories. These frameworks are designed to help organizations and individuals make more informed, objective, and effective decisions based on empirical evidence. Here are some of the primary models. Rational decision-making model.: This model assumes that individuals make logical and consistent decisions by weighing all possible alternatives and selecting the one with the highest utility. Rationality is the cornerstone of this model, and it seeks to make the optimal choice. Bounded rationality model.: Bounded rationality recognizes that in real-life scenarios, decision-makers often operate with limited information and cognitive constraints. They make decisions that are “good enough” rather than seeking the optimal choice. Intuitive decision-making.: Intuitive decision-making relies on gut feelings and personal experiences. It is often used when quick decisions are required or when information is incomplete. Data-Driven Decision Frameworks.. Data-driven decision frameworks provide a structured approach to decision-making, using data as a primary driver. Here are two major decision frameworks in the industry. Tool What Is it? Decision These graphical representations help you visualize and evaluate decision trees choices, outcomes, and probabilities. Pugh This decision matrix helps you compare multiple alternatives by quantifying matrix criteria and evaluating their impact. Decision Tree.. A decision tree gives you a systematic approach to making choices or decisions based on a set of criteria. It provides a structured way to evaluate different options and their potential outcomes, helping you select the most suitable course of action. Decision trees consist of nodes and branches, where nodes represent decisions or choices, and branches represent possible outcomes or consequences. For example, a decision tree can help you determine whether to play a sports game (yes or no) based on weather conditions. It can show you that if it’s sunny, first check humidity, and that if humidity is high, no, do not play, but if it’s low, yes, play. Or, if it’s cloudy, yes, play, and so on through different conditions. This framework is commonly used in business, finance, healthcare, and machine learning to model decision-making processes and optimize choices based on data and logic. Pugh Matrix.. The Pugh matrix, also known as the Pugh method or decision matrix, is a decision-making tool you use to evaluate and compare multiple alternatives or options based on a set of criteria. It gives you a systematic approach to make informed decisions by quantifying and ranking different options. The method was developed by Stuart Pugh, a British engineer and design theorist. The Pugh matrix helps you make rational decisions by considering multiple factors and their relative importance in a structured manner. The example Pugh matrix below shows criteria that start with a baseline of 0 with alternatives compared to the baseline as positive (+), negative (-), or equal (0). These comparisons are totaled to rank and optimize solutions. This method is a valuable tool in fields such as engineering, product design, and business management. These data-driven decision frameworks give you the ability to make objective and evidence-based choices. In logistics and supply chain management, data-driven decision frameworks can be applied to optimize the movement of goods. Companies can use data on inventory levels, demand forecasts, transportation costs, and lead times to make decisions about the most cost-effective shipping routes, storage locations, and reorder points. This minimizes costs, reduces stockouts, and ensures that products reach customers efficiently. Introduction to AI Models.. Now let’s look at AI models. As you’ve learned, artificial intelligence and machine learning (AI/ML) are powerful tools that can support your decision-making processes. Types of AI Models.. There are three primary types of AI models: supervised learning, unsupervised learning, and reinforcement learning. Supervised machine learning models are trained with labeled data sets, which allow the models to learn and grow more accurate over time. For example, an algorithm is trained with pictures of dogs and other things, all labeled by humans, and the machine learns ways to identify pictures of dogs on its own. Supervised machine learning is the most common type used today. In unsupervised machine learning, a program looks for patterns in unlabeled data. Unsupervised machine learning can find patterns or trends that people aren’t explicitly looking for. For example, an unsupervised machine-learning program can look through online sales data and identify different types of clients making purchases. Reinforcement machine learning trains machines through trial and error to take the best action by establishing a reward system. Reinforcement learning can train models to play games, or train autonomous vehicles to drive, by telling the machine when it made the right decisions, which helps it learn over time what actions it should take. Generative AI models use neural networks to identify the patterns and structures within existing data to generate new and original content. One of the breakthroughs with generative AI is its ability to leverage different learning approaches, including unsupervised or semi-supervised learning for training. Generative AI uses all three techniques together. Click the use case tabs to see how various industries use these AI models in decision-making processes. Bias and Fairness in AI.. The integration of artificial intelligence into decision-making processes across various domains has brought about unprecedented advancements in efficiency and accuracy. But as AI increasingly becomes an integral part of our lives, we need to address the issue of bias and fairness in AI systems. The importance of ensuring fairness and upholding ethical guidelines cannot be overstated. Bias, discrimination, and ethical dilemmas can arise when AI systems are not developed and used responsibly. Recognizing Bias and Ensuring Fairness in Data and AI Models.. Bias refers to the presence of systematic and unfair distinctions or preferences that can lead to discriminatory outcomes. Recognizing bias is the first step in addressing and mitigating its impact–once bias is identified, developers, data scientists, and business users can take corrective measures. Click each tab to learn actions organizations can take to recognize bias and promote fairness in data and AI models. In this unit, you explored various decision-making models, data-driven frameworks, and AI models, and the ethical imperative of mitigating bias and promoting fairness. In the next unit, take a look at real-world applications of AI in diverse scenarios. Apply AI in Decision-Making.. From business and healthcare to government and public policy, AI is transforming decision-making processes, optimizing business operations, and enhancing healthcare and public governance. In this unit, you explore AI's pivotal role in decision-making across various sectors. AI in Business Decision-Making.. Integrating AI into Business Strategy and Decision-Making.. Businesses have entered an era where data-driven decision-making is predominant. And AI is at the heart of this transformation. Using AI, organizations can extract valuable insights from data, streamline operations, and drive growth. In this section, let’s explore how businesses are integrating AI into their decision-making processes. AI scours through vast amounts of information to help businesses make informed choices. Companies big and small have embraced AI to augment their decision-making capabilities. For example, the world’s largest ecommerce platforms use AI algorithms to predict what products customers are likely to buy. By analyzing past purchase patterns and browsing history, AI assists in optimizing inventory management and enhancing customer satisfaction. This results in a seamless shopping experience for customers while maximizing the ecommerce company’s efficiency. Another excellent example is AI-driven chatbots and virtual assistants. Companies can utilize these AI tools to provide instant customer support. When you chat with a customer service representative online and receive quick, helpful responses, you’re likely interacting with an AI chatbot. These chatbots are trained to handle routine inquiries, resolve issues, and improve the overall customer experience by reducing response times. Have you used an AI co-pilot? These are artificial intelligence systems that assist or collaborate with a human user in various tasks or activities. AI co-pilots enhance productivity, provide guidance, and improve decision-making across a range of domains. Developers are rapidly adopting AI co-pilots to help with writing and fixing code. Another area where AI shines is personalized marketing. For example, streaming services harness AI algorithms to recommend content tailored to individual preferences. When you see recommended movies or TV shows that match your taste, it’s the result of AI analyzing your viewing history and suggesting content you’re likely to enjoy. This not only keeps you engaged but also increases your loyalty to the platform. Business Process Optimization with AI.. AI is also a process optimizer. It helps businesses streamline their operations, enhance efficiency, and reduce costs. For example, retailers use AI to predict customer demand. By analyzing vast amounts of data, including historical sales, seasonal trends, and even weather forecasts, retailers using AI can optimize their inventory to ensure that the right products are in stock at the right time, minimizing waste and preventing stockouts. Imagine you're shopping for groceries at your local supermarket. You may not notice it, but AI is working behind the scenes to ensure that products are available, prices are competitive, and the store layout is optimized for your convenience. The result? A smoother and more pleasant shopping experience for you, and greater operational efficiency and profitability for the store. AI has become an indispensable tool in business decision-making, offering valuable insights, enhancing customer experiences, and driving operational efficiency. It empowers organizations to adapt to ever-evolving market dynamics, make data-informed choices, and remain competitive in a fast-paced world. Using AI in a targeted way, businesses can navigate complexity, uncover opportunities, and chart a path toward sustained success. AI in Healthcare Decision-Making.. In the realm of healthcare, the integration of artificial intelligence has brought about a transformative shift in how medical professionals make critical decisions. AI applications have emerged as invaluable tools for improving diagnostic accuracy, treatment planning, and patient care. The Impact of AI on Healthcare Decision-Making.. AI is like a brilliant assistant for healthcare professionals. It can process vast amounts of patient data, analyze it for patterns and abnormalities, and offer valuable insights that assist doctors, nurses, and medical practitioners in making well-informed decisions. The use of AI in healthcare spans various domains, including medical imaging, patient data analysis, and drug discovery. For example, when a patient visits a hospital with symptoms of a disease, AI can rapidly analyze the patient's medical history, laboratory results, and medical images, such as X-rays or CT scans. By comparing this data with a vast repository of medical knowledge, AI can help clinicians make more accurate diagnoses. This can lead to earlier interventions, timely treatment, and improved patient outcomes. AI in Medical Diagnosis and Treatment Planning.. Imagine AI as a medical detective with an incredible memory. It can study thousands of medical cases and detect patterns that may not be immediately apparent to human observers. In the field of medical imaging, AI algorithms are employed to detect anomalies and support radiologists in interpreting complex scans. For example, the Google DeepMind research team has developed AI algorithms capable of detecting diabetic retinopathy from retinal images. Diabetic retinopathy is a condition that can lead to blindness if not detected and treated in its early stages. By analyzing retinal images, AI can identify signs of the condition, allowing for early intervention and better patient outcomes. Furthermore, AI’s capabilities extend to treatment planning. In oncology, AI-powered systems like IBM Watson for Oncology analyze vast amounts of medical data and research to provide oncologists with treatment recommendations. These recommendations are based on the latest medical literature, patient-specific information, and clinical guidelines. By leveraging AI, healthcare professionals can access cutting-edge information and make more personalized treatment decisions. Ethical Considerations in AI-Powered Healthcare Decisions.. While the integration of AI in healthcare holds great promise, it also raises ethical considerations. You’ve learned that AI-driven decisions can have significant consequences. This is especially true in a field as critical as healthcare. AI-driven recommendations and decisions must align with a patient’s best interests. Additionally, AI systems must respect patient privacy and obtain informed consent for interventions. For example, the use of AI in telemedicine and remote patient monitoring can raise concerns about data security and patient privacy. Healthcare organizations and AI developers must implement stringent security measures to protect patient data and ensure compliance with relevant regulations like the Health Insurance Portability and Accountability Act (HIPAA). Ethical AI in healthcare extends to issues of fairness and bias. AI algorithms must be carefully designed to avoid discriminatory practices and ensure that recommendations are based solely on clinical evidence. Ongoing research and development in ethical AI aim to create safeguards against bias and to promote fairness and transparency in healthcare decision-making. The integration of AI in healthcare decision-making represents a significant step toward more accurate diagnoses, improved treatment plans, and enhanced patient care. By working in tandem with healthcare professionals, AI ensures that decisions are data-driven, timely, and aligned with the best interests of patients. As AI continues to evolve, it will play an increasingly vital role in revolutionizing healthcare decision-making and improving patient outcomes. AI in Policy and Government Decision-Making.. The adoption of AI in the public sector is revolutionizing policy and government decision-making. AI has the potential to enhance the quality, efficiency, and fairness of government policies. Let’s see how it’s already informing government policies and decisions today. AI Application Key Benefits Data analysis for policy Data-driven policy development and improved outcomes insights Predictive analytics for Enhanced decision-making through trend forecasting decision-making Automation in public Streamlined services and cost reduction services Public opinion analysis Real-time public sentiment analysis for policy adjustment AI's Role in Informing Government Policies and Decisions.. AI is an invaluable resource for government bodies seeking to enhance their decision-making processes. By analyzing vast datasets, AI can identify trends, assess public sentiment, and predict future outcomes. This data-driven approach ensures that government policies are evidence-based and responsive to emerging challenges. For instance, some large cities are using AI in their traffic management systems. These systems analyze real-time traffic data and adjust traffic signals accordingly, reducing congestion, improving urban mobility, and enhancing the daily lives of citizens. Challenges and Considerations in AI Adoption in the Public Sector.. The adoption of AI in government decision-making is not without its challenges. Ethical considerations, data privacy, transparency, and regulatory compliance are essential areas of focus when integrating AI into public policies. AI used in government must adhere to ethical standards, ensuring that AI-driven decisions are made in the best interest of citizens. Transparency and accountability are critical, as the public should have visibility into how AI is used in government policies and decisions. The integration of AI in policy and government decision-making represents a significant advancement in making governance more efficient, data-driven, and responsive to citizens’ needs. Review Real-World Examples.. As you can see, AI has rapidly transformed the way we make decisions across many industries and domains. Click the tabs to review eight compelling examples. AI is enhancing decision-making, improving efficiency, and providing personalized experiences. By using critical thinking, data, and artificial intelligence together, organizations have incredible potential to make ethical, well-informed decisions that foster success and innovation.