Nonlinear Programming PDF
Document Details
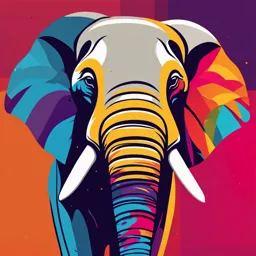
Uploaded by ResoluteConnemara508
Tags
Summary
This document provides an overview of nonlinear programming, discussing its applications to business problems. It introduces different models used in multicriteria decision-making and covers the solution methodologies. The document includes example problems, such as a profit analysis example from the Western Clothing Company. The document also features learning outcomes and course materials.
Full Transcript
Exhibit 9.16 Activities/Assessments 1. What is Goal Programming? 2. What is the importance of Goal Programming? 3. What is Graphical Interpretation of Goal Programming? 4. How is Graphical Interpretation applied? 5. In a Computer Solution of Goal Programming Problems which program i...
Exhibit 9.16 Activities/Assessments 1. What is Goal Programming? 2. What is the importance of Goal Programming? 3. What is Graphical Interpretation of Goal Programming? 4. How is Graphical Interpretation applied? 5. In a Computer Solution of Goal Programming Problems which program is better to use QM for Windows or Excel? Explain your answer. 6. What are Scoring Models? How is it used? 7. Identify the different models used in Multiciteria Decision Making and provide one example each. Provide your situations or data and use the model presented here to find a solution CHAPTER 10: NONLINEAR PROGRAMMING Overview More attention has been devoted to linear programming in this text than to any other single topic. It is a very versatile technique that can be and has been applied to a wide variety of problems. We have also presented several variations of linear programming, integer and goal programming, and unique applications of linear programming for transportation and assignment problems. In all these cases, all the objective functions and constraints were linear; that is, they formed a line or plane in space. However, many realistic business problems have relationships that can be modeled only with nonlinear functions. When problems fit the general linear programming format but include nonlinear functions, they are referred to as nonlinear programming problems. Nonlinear programming problems are given a separate name because they are solved in a different manner than are linear programming problems. In fact, their solution is considerably more complex than that of linear programming problems, and it is often difficult, if not impossible, to determine an optimal solution, even for a relatively small problem. What is difficult in nonlinear programming is determining if the point at the top of a peak is just the highest point 167 in the immediate area (called a local optimal, in calculus terms) or the highest point of all (called the global optimal). In this chapter, we present the basic structure of nonlinear programming problems and use Excel to solve simple models. Learning Outcomes At the end of this chapter , students must be able to 1. Understand the basic strucutre of non-linear programming 2. Apply excel to non-linear programming problems COURSE MATERIALS Nonlinear Profit Analysis To demonstrate the solution procedure, we will use a profit function based on break-even analysis. In Chapter 1 we used break-even analysis to begin our study of model building, so it seems appropriate that we return to this basic model to complete our study of model building. Recall that in break-even analysis the profit function, Z, is formulated as One important but somewhat unrealistic assumption of this break-even model is that volume, or demand, is independent of price (i.e., volume remains constant, regardless of the price of the product). It would be more realistic for the demand to vary as price increased or decreased. For our Western Clothing Company example from Chapter 1, let us suppose that the dependency of demand on price is defined by the following linear function: This linear relationship is illustrated in Figure 10.1. The figure illustrates the fact that as price increases, demand decreases, up to a particular price level ($60.98) that will result in no sales volume. 168 Figure 10.1 Linear relationship of volume to price Now we will insert our new relationship for volume (v) into our original profit equation: Substituting values for fixed cost (cf = $10,000) and variable cost (cv = $8) into this new profit function results in the following equation: Because of the squared term, this equation for profit is now a nonlinear, or quadratic, function that relates profit to price, as shown in Figure 10.2. Figure 10.2 The nonlinear profit function In Figure 10.2, the greatest profit will occur at the point where the profit curve is at its highest. At that point the slope of the curve will equal zero, as shown in Figure 10.3. Figure 10.3 Maximum profit for the profit function 169 In calculus, the slope of a curve at any point is equal to the derivative of the mathematical function that defines the curve. The derivative of our profit function is determined as follows: Given this derivative, the slope of the profit curve at its highest point is defined by the following relationship: Now we can solve this relationship for the optimal price, p, which will maximize total profit: The optimal volume of denim jeans to produce is computed by substituting this price into our previously developed linear relationship for volume: The maximum total profit is computed as follows: The maximum profit, optimal price, and optimal volume are shown graphically in Figure 10.4. Figure 10.4 Maximum profit, optimal price, and optimal volume 170 An important concept we have yet to mention is that by extending the break-even model this way, we have converted it into an optimization model. In other words, we are now able to maximize an objective function (profit) by determining the optimal value of a variable (price). This is exactly what we did in linear programming when we determined the values of decision variables that optimized an objective function. The use of calculus to find optimal values for variables is often referred to as classical optimization. Constrained Optimization In the preceding section, the profit analysis model was developed as an extension of the breakeven model. Recall that the total profit function was and the demand function (i.e., volume as a function of price) was By substituting this demand function into our total profit equation, we developed a nonlinear function: Then, by substituting values for cf ($10,000) and cv ($8) into this function, we obtained We then differentiated this function, set it equal to zero, and solved for the value of p ($34.49), which corresponded to the maximum point on the profit curve (where the slope equaled zero). 171 This type of model is referred to as an unconstrained optimization model. It consists of a single nonlinear objective function and no constraints. If we add one or more constraints to this model, it becomes a constrained optimization model. A constrained optimization model is more commonly referred to as a nonlinear programming model. The reason this type of model is designated as a form of mathematical programming is because all types of mathematical programming models are actually constrained optimization models. That is, they all have the general form of an objective function that is subject to one or more constraints. Linear programming has an objective function and constraints that happen to be linear. A nonlinear programming model has the same general form as a linear programming model, except that the objective function and/or the constraint(s) are nonlinear. Nonlinear programming differs from linear programming, however, in one other critical aspect: The solution of nonlinear programming problems is much more complex. In linear programming a particular procedure is guaranteed to lead to a solution if the problem has been correctly formulated, whereas in nonlinear programming no guaranteed procedure exists. The reason for this complexity can be illustrated by a graph of our profit analysis model. Figure 10.5 shows the nonlinear profit curve for the example model. Figure 10.5 Nonlinear profit curve for the profit analysis model As stated previously, the solution is found by taking the derivative of the profit function, setting it equal to zero, and solving for p (price). This results in an optimal value for p that corresponds to the maximum profit, as shown in Figure 10.5. Now we will transform this unconstrained optimization model into a nonlinear programming model by adding the constraint In other words, because of market conditions, we are restricting the price to a maximum of $20. This constraint results in a feasible solution space, as shown in Figure 10.6. 172 Figure 10.6 A constrained optimization model In Figure 10.6, point A is the optimal solution. It corresponds to the maximum value of the portion of the objective function that is still feasible. However, the difficulty with nonlinear programming is that the solution is not always on the boundary of the feasible solution space formed by the constraint. For example, consider the addition of the following constraint to our original nonlinear objective function: This constraint also creates a feasible solution space, as shown in Figure 10.7. Figure 10.7 A constrained optimization model with a solution point not on the constraint boundary But notice in Figure 10.7 that the solution is no longer on the boundary of the feasible solution space, as it would be in linear programming. Point C represents a greater profit than point B, and it is also in the feasible solution space. This means that we cannot simply look at points on the solution space boundary to find the solution; instead, we must also consider other points on the surface of the objective function. A number of different solution approaches to nonlinear programming problems are available. As we have already indicated, they typically represent a convergence of the principles of calculus and mathematical programming. However, as noted earlier, the solution techniques can be very complex. Thus, we will confine our discussion of nonlinear programming solution methods to several different model applications and the Excel solution. 173 Solution of Nonlinear Programming Problems with Excel Exhibit 10.1 shows an Excel spreadsheet set up to solve our initial Western Clothing Company example. The demand function contained in cell C4 is =1500-24.6*C5. The formula for profit is contained in cell C3 and is shown on the formula bar at the top of the spreadsheet. Exhibit 10.1 The Solver Parameters window for this problem is shown in Exhibit 10.2. It is important tomake sure that the ―Nonlinear‖option has been selected. Exhibit 10.3shows the Excel solutionfor the Western Clothing Company example. Exhibit 10.2 Exhibit 10.3 Exhibit 10.4 shows an Excel spreadsheet set up to solve a nonlinear version of the Beaver Creek Pottery Company example from Chapter 2 that is formulated as Exhibit 10.4 174 The numbers of bowls and mugs produced are contained in cells C5 and C6, respectively. The profit formula for a bowl is contained in cell D5. The total profit contained in cell C11 is computed using the formula =SUMPRODUCT(C5:C6,D5:D6) and is shown on the formula bar at the top of the spreadsheet. The formula for labor, =C5+2*C6, is contained in cell C9. The Solver Parameters window for this problem is shown in Exhibit 10.5, and the final solution is shown in Exhibit 10.6. Excel will also provide the value of the Lagrange multiplier, which provides the dual value of the labor resource. To derive the Lagrange multiplier, after you click on ―Solve‖ in Solver, the Solver Results screen shown in Exhibit 10.7 will appear. On this screen, under ―Reports,‖ select ―Sensitivity.‖ This will generate the sensitivity report shown in Exhibit 10.8. Note that in addition to the problem solution, the value of the Lagrange multiplier is also provided for the labor constraint. The Lagrange multiplier value of.33 is analogous to the dual value in a linear programming problem. It reflects the approximate change in the objective function resulting from a unit change in the quantity (right-hand-side) value of the constraint equation. For this example, if the quantity of labor hours is increased from 40 to 41 hours, the value of Z will increase by $0.33— from $70.42 to $70.75. Exhibit 10.5 Exhibit 10.6 Exhibit 10.7 175 Exhibit 10.8 A Nonlinear Programming Model with Multiple Constraints Now that we have shown how Excel can be used to solve nonlinear problems, we can look at more complex problems—for example, a problem with more than one constraint. Consider the 176 Western Clothing Company example presented earlier, except now the company produces two kinds of jeans, designer and straight-leg, and production is subject to resource constraints for denim cloth, cutting time, and sewing time. The company sells its jeans to several upscale clothing store chains, and sales demand is dependent on the price at which the company sells the jeans. The demand for designer jeans (x1) and the demand for straight-leg jeans (x2) are defined by the following relationships: The cost of producing designer jeans is $12 per pair, and the cost of producing straight-leg jeans is $9 per pair; thus, the objective function for profit is The production of jeans is subject to the following resource constraints for available cloth, cutting time, and sewing time: The complete nonlinear programming model formulation is summarized as follows: Notice that the decision variables for this problem are p1 and p2, not x1 and x2 The demand variables x1 and x2, are functions of price and, thus, are dependent variables. Also notice that we did not substitute the functional relationships for x1 and x2 into the objective function, creating a quadratic function. The model is still nonlinear, but we can solve it directly with Excel in its present form. Exhibit 10.9 shows an Excel spreadsheet set up to solve our Western Clothing Company example with constraints. The decision variables for price are contained in cells D5:D6. The formula for demand for designer jeans is contained in cell C5 and is shown on the formula bar at the top of the screen. The formula for total profit, =SUMPRODUCT(C5:C6,E5:E6) is contained in cell E7. The formulas for the resource constraints are contained in cells C11, C12, and C13, and the resource availabilities for each constraint are in cells D11:D13. The Solver Parameters window and solution are shown in Exhibit 10.10 and Exhibit 10.11, respectively. 177 Exhibit 10.9 Exhibit 10.10 178 Exhibit 10.11 Nonlinear Model Examples In our previous profit analysis examples, the models followed the traditional mathematical (i.e., linear) programming formulation, with an objective function, decision variables, and constraints. However, many types of problems employ well-known nonlinear functions but are not typically thought of in the traditional math programming model framework. In this section we consider several additional examples that include familiar nonlinear functions—the facility location model and the investment portfolio selection model. Facility Location In a facility location problem, the objective, in general, is to locate a centralized facility that serves several customers or other facilities to minimize distance or miles traveled between the facility and its customers. This problem uses as a measure of distance the formula for the straight-line distance between two points on a set of x, y coordinates, which is also the hypotenuse of a right triangle: Consider, for example, the Clayton County Rescue Squad and Ambulance Service, which serves five rural towns, Abbeville, Benton, Clayton, Dunning, and Eden. The rescue squad wants to construct a centralized facility and garage to minimize its total annual travel mileage to the towns. The locations of the five towns in terms of their graphical x, y coordinates, measured in miles relative to the point x = 0, y = 0 and the expected number of annual trips the 179 squad will have to make to each town are as follows: The objective of the problem is to determine a set of coordinates (x, y) for the rescue squad facility that minimizes the total miles traveled to the town, according to the following function: The Excel solution to this problem is shown in Exhibit 10.12. The coordinates (x, y) for the new rescue squad facility are in cells C14:C15. The formulas for the distances between the rescue squad facility and each of the towns (d1) are in cells E6:E10. For example, the formula for the distance, dA, between the rescue squad facility and Abbeville in cell E6 is =SQRT((B6- C14)^2+(C6-C15)^2). The formula for the total annual distance in cell D18 is =SUMPRODUCT(E6:E10,D6:D10), which is also shown on the formula bar at the top of the spreadsheet. In the Solver Parameters window for this problem, D18 is the target cell that is minimized, and the changing cells (i.e., decision variables) are C14:C15. There are no constraints. Exhibit 10.12 The location of the rescue squad facility is shown graphically in Figure 10.8. 180 Figure 10.8 Rescue squad facility location Investment Portfolio Selection A classic example of nonlinear programming is the investment portfolio selection model developed by Harry Markowitz in 1959. This model is based on the assumption that most investors are concerned with two factors—return on investment and risk. Thus, the objective of the portfolio selection model is to minimize some measure of portfolio risk while achieving a minimum return on the total portfolio investment. Risk is reflected by the variability in the value of the investment, and in this model, variance in the return on investment is the measure of risk. Covariance, which is a measure of correlation, is also used to reflect risk. Individual investment returns within a portfolio typically exhibit statistical dependence. Over time, the returns of any two stocks may exhibit positive or negative correlation; that is, two stocks of the same general type will go up or down together. To reflect the risk associated with not diversifying, the model includes covariance. The minimization of portfolio risk, as measured by the portfolio variance, is the model objective. The variance, S, on the annual return from the portfolio is determined by the following formula: In the preceding formula for S, the first part is a measure of variance, and the second part is a measure of the covariance. In many cases, the parameters in this equation may be estimates or sample values (the sample variance, sample covariance, etc.). The general formulation of the portfolio selection model is as follows. The investor desires to achieve a minimum expected annual return from the portfolio, which is formulated as a model 181 constraint as follows: A second constraint specifies that all the money is invested: The following example and Excel solution will demonstrate how this model is applied. Jessica Todd has identified four stocks she wants to include in her investment portfolio. She wants a total annual return of at least.11. From historical data, she has estimated the average returns and variances for the four investments as follows: She has also estimated the covariances between the stocks, as follows: The nonlinear programming model formulation for this problem is as follows: The Excel spreadsheet for Jessica Todd’s portfolio is shown in Exhibit 10.13. The decision variables representing the proportion of each stock in the portfolio (xi) formula for total return, =SUMPRODUCT(B6:B9,E6:E9), is in cell B19. The objective is to minimize the total portfolio variance formula, which is shown on the formula bar at the top of the spreadsheet. This is a complicated formula and difficult to type in. It has been shortened slightly by doubling the covariance terms in the last part of the equation to reflect all the different investment pairs that are included in B14:E17 (i.e., 2 times AB, AC, AD, BC, BD, and CD will include BA, CA, DA, CB, DB, and DC). In other words, all the covariance terms to the right of the diagonal in the matrix B14:E17 are doubled to include the same values to the left of the diagonal. Exhibit 10.13 182 The Solver Parameters window is shown in Exhibit 10.14. Notice that nonnegativity constraints for the variables are included—by clicking on ―Options.‖ The model solution is shown in Exhibit 10.15. It indicates that Jessica should invest 36.0% of her funds in Altacam (x1), 27.2% in Bestco (x2), 31.5% in Com.com (x3), and 5.3% in Delphi (x4). This will result in her desired Exhibit 10.14 Exhibit 10.15 minimum portfolio annual return of.11 and a variance of.0104. What does this variance mean? A variance of.0104 equals a standard deviation of.102. If returns are normally distributed (which is likely), then there is a.95 probability that the annual portfolio return will be between -.8099 [.11 – (1.96)(.102)] and.3099[.11 + (1.96)(.102)]. 183