BEHL 2005/2019 Introductory Research Methods PDF
Document Details
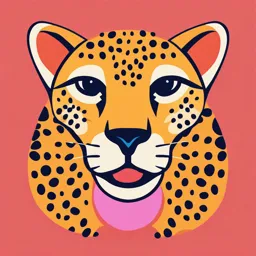
Uploaded by LuckiestForethought
BEHL
Hannah Keage
Tags
Summary
This document is an introductory lecture on research methods, focusing on parametric vs. non-parametric data. It covers what each type of data represents, how to identify it, and appropriate tests. It also discusses outliers and degrees of freedom.
Full Transcript
BEHL 2005 / BEHL 2019 (UO) Introductory Research Methods How to determine if you have parametric or non-parametric data Professor Hannah Keage What are we going to cover? What is parametric data? How to determine if you have parametric data Parametric v non-parametric tests Content from this lec...
BEHL 2005 / BEHL 2019 (UO) Introductory Research Methods How to determine if you have parametric or non-parametric data Professor Hannah Keage What are we going to cover? What is parametric data? How to determine if you have parametric data Parametric v non-parametric tests Content from this lecture references: What is parametric data? • • • • Parametric data = normal data. Non-parametric data = not normal or non-normal. In this course, we will really only assess the normality of continuous data. So, what’s normal? • • • • Bell curve. Not too skewed (sway to left or right). Not too kurtotic (flat or peaky). No outliers (extreme values). • Why do we care? • Normality is an assumption of some statistical models, mathematically. • If we violate normality and use a parametric test, we may not be able to trust the model estimates. For continuous data… NO Has an outlier(s) YES Can you remove the outlier(s)? NO Non-parametric test (with non-normal data) YES YES Data is skewed or kurtotic? NO Parametric test (with normal data) For continuous data… NO Has an outlier(s) YES Can you remove the outlier(s)? NO Non-parametric test (with non-normal data) YES YES YES Another option here is transforming the data, to change its shape; we will not cover transformations in IRM. Notably, sometimes this option doesn’t suit psychological data. Data is skewed or kurtotic? NO Parametric test (with normal data) Testing for outliers • Box plot, very easy in jamovi. • The thick line in the middle of the box = median. • The box itself spans from the 25th percentile to the 75th percentile (or inter quartile range). • Whiskers indicate acceptable values (not outliers). • Any observation whose value falls outside this acceptable range is plotted as a dot and is not covered by the whiskers = outlier. • Common alternative: 3 standard deviations (SD) from the mean (+/-). I have an outlier…! • There’s no absolute right or wrong way to proceed. Be transparent and justify your approach. You can… • Run a non-parametric test. • Commonly done if it’s a “true” value. E.g. testing went well, the participant understood task instructions, but scored very low; this performance represents that participants ability. • Remove the value and leave as missing. • Commonly done when working with big data sets, where you’re not going to check participant records and have plenty of statistical power. • Remove the value and replace with nearest acceptable value. • Commonly done in psychological studies. • Remove value and replace with mean. • Historical, not commonly done these days. Testing skew and kurtosis • Shapiro-Wilk test. Very easy in jamovi. • Takes into account both skew and kurtosis. • W statistic. • Maximum value of 1 = data looks “perfectly normal”. • The smaller the value of W the less normal the data are. • p value (of W statistic). • Typically, <.05 = non-normal data. • Therefore, ≥.05 = normal data. Parametric tests Non-parametric tests Pearson correlation Spearman correlation T-test (between groups or within groups) Wilcoxon test (2 groups/conditions) ANOVA (IRM, we’ll work with between groups) Kruskall-Wallis test (3 or more groups) Parametric v non-parametric tests • There are generally non-parametric versions of all parametric tests. • We do non-parametric tests when our data are not normally distributed. • We do parametric tests when our data are normally distributed. • Parametric tests have more statistical power, so, they are preferred and are generally the default set of tests. Degrees of freedom • Important to the mathematical calculations of parametric and nonparametric tests. • Based on the quantities of data in your model, e.g. participants or factors. In the models we will use in IRM, degrees of freedom (df) will mostly be the number of participants - 1. • For the most part, a higher df = more statistical power. I don’t get a choice! BEHL 2005 / BEHL 2019 (UO) Introductory Research Methods How to determine if you have parametric or non-parametric data Professor Hannah Keage