Introduction to Statistics PDF
Document Details
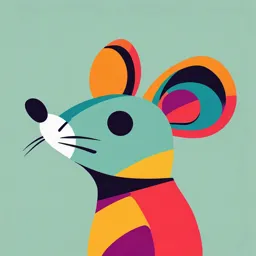
Uploaded by CohesiveForest9347
Central Bicol State University of Agriculture
Tags
Summary
This document provides an introduction to fundamental statistical concepts, including definitions of key terms like population, sample, variable, and data. It outlines different levels of measurement (nominal, ordinal, interval, and ratio) and provides examples to illustrate these concepts.
Full Transcript
UNIT 1: INTRODUCTION Learning Outcomes: At the end of this unit, you will be able to define the basic terms in statistics differentiate the fields of statistics: mathematical/ theoretical statistics and applied statistics enumerate the steps in statistical inquiry A. Basic Concepts Defin...
UNIT 1: INTRODUCTION Learning Outcomes: At the end of this unit, you will be able to define the basic terms in statistics differentiate the fields of statistics: mathematical/ theoretical statistics and applied statistics enumerate the steps in statistical inquiry A. Basic Concepts Definition of Statistics o Plural sense – set of numerical figures e.g. shooting averages of basketball players, vital statistics in a beauty contest o Singular sense – the branch of science that deals with the collection, presentation, organization, analysis, and interpretation of data (COPAI) Why is it important to learn how to collect, present, organize, analyse, and interpret data? Statistics provides tools to convert massive data volumes into pertinent information that can be used to make BETTER and MORE SENSIBLE decisions. Information empowers us to make INTELLIGENT choices. Key Terms o Population – (denoted by N) the collection of all elements under consideration in a statistical inquiry o Sample – (denoted by n) a subset of the population o Elements of the population can be individuals, objects, animals, geographic areas, etc. o E.g. set of farmers in Negros Occidental, set of instructors in CPSU, collection of papaya harvested in Kabankalan, etc. o Variable – a characteristic or attribute of the elements in a collection that can assume different values for the different elements. Example: Age, Weight, Height o Experimental unit is the individual or object on which a variable is measured. Example: Age (in years) of a freshman student Weight (in kilograms) of mangoes harvested Height (in inches) of a sugarcane plant in 3 months o Observation – realized value of a variable o Data – collection of observations Example: Variable Possible observation a. S = sex of a student Male, Female b. E = employment status of an employee Temporary, Permanent, Contractual c. I = monthly income of a person in pesos 𝑖≥0 d. N =number of children of a teacher 𝑛 = 0, 1, 2, 3, … e. H = height of a basketball player h > 0 cm. Example. Let’s identify the population and sample under study and variable/s of interest and observation. a. The Office of Admissions is studying the relationship between the score in the entrance examination during application and the general weighted average (GWA) upon graduation among graduates of the university from 2000 to 2005. Population: collection of all graduates of the university from 2000 to 2005 Sample: all of the graduates of the university from 2000 to 2005 in a particular department Variable of interest: score in the entrance examination and general weighted average (GWA) Observation: the individual scores in the entrance exam and the individual GWA of the students o Parameter – a summary measure describing specific characteristic of the population usually denoted by Greek letters: μ (mu), σ (sigma), ρ (rho), λ (lambda), τ (tau), θ (theta), α (alpha) and β (beta) e.g. population mean, population variance o Statistic – a summary measure describing specific characteristic of the sample e.g. sample mean, sample variance o Proportion – the quotient obtained when the magnitude of the part is divided by the magnitude of the whole 𝑛𝑜. 𝑜𝑓 𝑒𝑙𝑒𝑚𝑒𝑛𝑡𝑠 𝑝𝑜𝑠𝑠𝑒𝑠𝑠𝑖𝑛𝑔 𝑎 𝑐𝑒𝑟𝑡𝑎𝑖𝑛 𝑐ℎ𝑎𝑟𝑎𝑐𝑡𝑒𝑟𝑖𝑠𝑡𝑖𝑐 𝑃= 𝑛𝑜. 𝑜𝑓 𝑒𝑙𝑒𝑚𝑒𝑛𝑡𝑠 𝑖𝑛 𝑡ℎ𝑒 𝑐𝑜𝑙𝑙𝑒𝑐𝑡𝑖𝑜𝑛 Example: Population: 20 students in a Statistics course Variable under study: X = whether or not the student owns a cellphone Possible values: 0 – the student does not own a cellphone 1 – the student owns a cellphone Suppose, among 20 students, 15 own a cellphone, the proportion of students in the population with cellphones is 𝑛𝑜. 𝑜𝑓 𝑠𝑡𝑢𝑑𝑒𝑛𝑡𝑠 𝑤𝑖𝑡ℎ 𝑐𝑒𝑙𝑙𝑝ℎ𝑜𝑛𝑒 15 𝑃= = = 0.75 𝑛𝑜. 𝑜𝑓 𝑠𝑡𝑢𝑑𝑒𝑛𝑡𝑠 𝑖𝑛 𝑡ℎ𝑒 𝑝𝑜𝑝𝑢𝑙𝑎𝑡𝑖𝑜𝑛 20 Suppose, a sample of ten students was taken in this class, Among the 10 students, 7 have cellular phones. We cannot compute for P but we can compute for 𝑃̂ (P hat). 𝑃̂ = proportion of students in the sample with cellphones 𝑛𝑜. 𝑜𝑓 𝑠𝑡𝑢𝑑𝑒𝑛𝑡𝑠 𝑤𝑖𝑡ℎ 𝑐𝑒𝑙𝑙𝑝ℎ𝑜𝑛𝑒𝑠 𝑖𝑛 𝑡ℎ𝑒 𝑠𝑎𝑚𝑝𝑙𝑒 7 𝑃̂ = = = 0.7 𝑛𝑜. 𝑜𝑓 𝑠𝑡𝑢𝑑𝑒𝑛𝑡𝑠 𝑖𝑛 𝑡ℎ𝑒 𝑠𝑎𝑚𝑝𝑙𝑒 10 Note that 𝑃 (population proportion) is a parameter while 𝑃̂ (sample proportion) is a statistic. Example: In order to estimate the true proportion of students at a certain college who smoke cigarettes, the administration polled a sample of 200 students and determined that the proportion of students from the sample who smoke cigarettes is 0.12. Identify the a) population, b) sample, c) parameter, and d) statistic. a) Population: The set of students at a certain college. b) Sample: The set of 200 students who were interviewed. c) Parameter: The population proportion of students in a certain college who smoke cigarettes. d) Statistic: (0.12) the proportion of students in the sample who smoke cigarettes. Example: A politician who is running for the office of mayor of a city with 25,000 registered voters commissions on a survey. In the survey, 48% of the 200 registered voters interviewed say they plan to vote for her. a) What is the population of interest? The group of 25,000 registered voters b) What is the sample? The group of 200 registered voters who were interviewed c) Is the value 48% a parameter or a statistic? Statistic B. Fields of Statistics 1. Statistical Methods of Applied Statistics - procedures and techniques used in the collection, presentation, analysis and interpretation of data. 2. Statistical Theory of Mathematical Statistics - deals with the development and exposition of theories that serve as bases of statistical methods. a. Descriptive Statistics – includes all the techniques used in organizing, summarizing, and presenting the data on hand. b. Inferential Statistics – includes all the techniques used in analyzing the sample data that will lead to generalizations about a population from which the sample came from Descriptive Inferential 1. A bowler wants to find his bowling 1. A bowler wants to estimate his chance of average for the past 12 games. winning a game based on his current season averages and the averages of his opponent. 2. A housewife wants to determine the 2. A housewife would like to predict based on last average weekly amount she spent on year's grocery bills, the average weekly amount groceries in the past three months. she will spend for this year. 3. A politician wants to know the exact 3. A politician would like to estimate, based on an number of votes he received in the last opinion poll, his chance of winning in the election. upcoming election. C. Steps in a Statistical Inquiry Statistical Inquiry o A designed research that provides information needed to solve a research problem. o Identifies the problem, plans the study, collects the data, explores the data, analyzes the data, and interprets the results Steps in a Statistical Inquiry Step 1: Identify the problem Step 2: Plan the study Step 3: Collect the Data Step 4: Explore the Data Step 5: Analyze data and interpret the results Step 6: Present the results Step 1: Identify the Problem Must begin with a clearly stated research problem A review of related literature is necessary at this stage After stating the general problem, researchers identify specific information needed to answer the problem. Example: A researcher wants to determine if there is association between the price and production of lumber. 1. What kind of lumber will be included in the study? Will all types of lumber be included or just one specific type? 2. Will the study include the whole production of lumber or only lumber produced for sale? 3. What price for lumber will be used, the market price or the factory price? 4. What is the scope of the study? Are all the regions of the country included or just a specific region or province only. 5. What period is covered in the study? Problem: What is the relationship between the total mahogany production of Mindanao and the market price of mahogany in the past 10 years? How to specify the scope and definition of the population: Population of interest: collection of students in the universities a) What is a university? b) Who are the students in the university? Should “students” refer only to college students or should it also include students in the graduate programs. c) Are all types of students included or only the regular ones? Population of interest: collection of fishponds in Central Luzon a) What is a fishpond? b) What is the minimum area of the fishpond considered in the study? c) What type/s of fish is included in the study? Population of interest: collection of residents in Quezon City a) What is a resident? b) Is student staying in a dormitory considered a resident? What about household help, are they also considered as residents? Different ways of writing the research problem 1. Form of a question What are the factors affecting the job performance of an employee? What is the relationship between wheat yield and amount of fertilizer? 2. Statement or general aim This study proposes to describe the relationship among job satisfaction, salary, quality of relationship with the supervisor, and job performance This study aims to predict next month’s supply/demand for rice. To further refine the statement of the problem, a researcher formulates a hypothesis Hypothesis – educated guess by the researcher, a possible answer to the research problem based on his study of related literature, own experiences and previous observations. Example: The average expenditures of households in district I is higher than that of district II. Sodium content in cereals produced by a certain company exceeds the required daily limit The researchers must list down all the specific objectives after stating the problem. Step 2: Plan the study In coming up with a plan, the researchers need to take into consideration the stated research problem and the specific objectives. The concrete output in step 2 is the research design Research design is the detailed discussion of the methods and strategies for data collection and analysis that the investigators plan to use order to meet the specific objectives stated in step 1. Effective research design ◦ Simple – to avoid complications and errors ◦ Cost efficient – to be confident of completing the study within the allotted budget and time, without sacrificing the quality of data Basic elements of a research design ◦ List of variables in the study, the design of the instrument that will be used to measure them ◦ Data collection method ◦ Sampling design if data will be collected from a sample ◦ Experimental design if data will be collected through experiment. ◦ Methods for data analysis Step 3: Collect the Data Investigators carry out the plans specified in the research design on data collection Investigators take extra measures to ensure the quality of the data collected Step 4: Explore the data Investigators need to explore and understand the essential features of their data Determine if data satisfy the assumptions made in the derivation of the statistical technique to be used for analysis Step 5: Analyze and interpret the results The investigators do data analysis. Examine all of the results, tables, charts, estimated summary measure, and tests of hypotheses Check if the analysis answers the research problem and give recommendations Check if the results contradict an existing theory or the hypothesis made. Step 6: Present the results Present results in a clear and concise manner to the users of the research Presentation must include discussion of the whole research process. Three ways of presenting results are textual, tabular, and graphical TERMINOLOGIES IN STATISTICS Population: The entire group that is the subject of a study or the group from which a sample is drawn. It includes all members of the group being studied. Sample: A subset of the population selected for analysis. Samples are used to estimate characteristics of the population. Parameter: A numerical value that summarizes a characteristic of the population, such as the population mean or population proportion. Statistic: A numerical value that summarizes a characteristic of the sample, such as the sample mean or sample proportion. Statistics are used to estimate parameters. Mean (Average): The sum of all values in a data set divided by the number of values. It provides a measure of central tendency. Median: The middle value in a data set when the values are arranged in ascending or descending order. It is also a measure of central tendency. Mode: The value that occurs most frequently in a data set. It can be used to identify the most common value. Variance: A measure of the dispersion or spread of a data set. It calculates the average of the squared differences from the mean. Standard Deviation: The square root of the variance. It provides a measure of how spread out the values in a data set are from the mean. Descriptive Statistics: Methods for summarizing and describing the important features of a data set, such as measures of central tendency and dispersion. Inferential Statistics: Methods for making inferences and predictions about a population based on a sample of data. This includes hypothesis testing, confidence intervals, and regression analysis. Understanding these terminologies is crucial for effectively analyzing and interpreting data in statistics. TYPES OF DATA The data is classified into four major categories: 1. Nominal data 2. Ordinal data 3. Discrete data 4. Continuous data Further, we can classify these data as follows: Types of Data Categorical or Numerical or Qualitative Data Quantitative Data Discrete Continuous Nominal Ordinal Data Data Data Data Qualitative or Categorical Data Qualitative data, also known as the categorical data, describes the data that fits into the categories. Qualitative data are not numerical. The categorical information involves categorical variables that describe the features such as a person’s gender, home town etc. Categorical measures are defined in terms of natural language specifications, but not in terms of numbers. Sometimes categorical data can hold numerical values (quantitative value), but those values do not have a mathematical sense. Examples of the categorical data are birthdate, favorite sport, school postcode. Here, the birthdate and school postcode hold the quantitative value, but it does not give numerical meaning. Nominal Data Nominal data is one of the types of qualitative information which helps to label the variables without providing the numerical value. Nominal data is also called the nominal scale. It cannot be ordered and measured. But sometimes, the data can be qualitative and quantitative. Examples of nominal data are course, hair color, nationality, sex, name of people, etc. The nominal data are examined using the grouping method. In this method, the data are grouped into categories, and then the frequency or the percentage of the data can be calculated. These data are visually represented using the pie charts. Ordinal Data Ordinal data/variable is a type of data that follows a natural order. The significant feature of the ordinal data is that the difference between the data values is not determined. This variable is mostly found in surveys, finance, economics, questionnaires, and so on. The ordinal data is commonly represented using a bar chart. These data are investigated and interpreted through many visualization tools. The information may be expressed using tables in which each row in the table shows the distinct category. Examples of ordinal data are year level, educational level, income category, sleep quality, etc. Quantitative or Numerical Data Quantitative data is also known as numerical data which represents the numerical value (i.e., how much, how often, how many). Numerical data gives information about the quantities of a specific thing. Some examples of numerical data are height, length, size, weight, and so on. The quantitative data can be classified into two different types based on the data sets. The two different classifications of numerical data are discrete data and continuous data. Discrete Data Discrete data can take only discrete values. Discrete information contains only a finite number of possible values. Those values cannot be subdivided meaningfully. Here, things can be counted in whole numbers. We can easily count the variables in a discrete data. Example: Number of students in the class, number of players in a team Continuous Data Continuous data is data that can be calculated. It has an infinite number of probable values that can be selected within a given specific range. Example: Temperature range, height, weight, age KINDS OF VARIABLES A variable (usually denoted by letters or symbols) is a characteristic, number, or quantity that takes different values in different situations. 1. Independent variable A variable is independent if it may vary freely and does not depend upon changes in other variables. It is usually denoted by x. 2. Dependent variable A variable is dependent if it varies according to changes in other variables. It is usually denoted by y. Independent variable Dependent variable Time spent reviewing for an exam The marks (grade/score) from an exam How many spoonfuls of sugar you put in your tea How sweet your tea is MEASUREMENT SCALES In Statistics, the variables or numbers are defined and categorized using different scales of measurements. Each level of measurement scale has specific properties that determine the various use of statistical analysis. In this article, we will learn four types of scales such as nominal, ordinal, interval and ratio scale. What is the Scale? A scale is a device or an object used to measure or quantify any event or another object. Levels of Measurements There are four different scales of measurement. The data can be defined as being one of the four scales. The four types of scales are: 1. Nominal Scale 2. Ordinal Scale 3. Interval Scale 4. Ratio Scale 1. Nominal Scale A nominal scale is the 1st level of measurement scale in which the numbers serve as “tags” or “labels” to classify or identify the objects. A nominal scale usually deals with the non-numeric variables or the numbers that do not have any value. Characteristics of Nominal Scale A nominal scale variable is classified into two or more categories. In this measurement mechanism, the answer should fall into either of the classes. It is qualitative. The numbers are used here to identify the objects. The numbers don’t define the object characteristics. The only permissible aspect of numbers in the nominal scale is “counting.” Example: What is your gender? M- Male F- Female Here, the variables are used as tags, and the answer to this question should be either M or F. 2. Ordinal Scale The ordinal scale is the 2nd level of measurement that reports the ordering and ranking of data without establishing the degree of variation between them. Ordinal represents the “order.” Ordinal data is known as qualitative data or categorical data. It can be grouped, named and also ranked. Characteristics of the Ordinal Scale The ordinal scale shows the relative ranking of the variables It identifies and describes the magnitude of a variable Along with the information provided by the nominal scale, ordinal scales give the rankings of those variables The interval properties are not known The surveyors can quickly analyze the degree of agreement concerning the identified order of variables Example: Ranking of school students – 1st, 2nd, 3rd, etc. Ratings in restaurants Evaluating the frequency of occurrences Very often Often Not often Not at all Assessing the degree of agreement Totally agree Agree Neutral Disagree Totally disagree 3. Interval Scale The interval scale is the 3rd level of measurement scale. It is defined as a quantitative measurement scale in which the difference between the two variables is meaningful. In other words, the variables are measured in an exact manner, not as in a relative way in which the presence of zero is arbitrary. Characteristics of Interval Scale: The interval scale is quantitative as it can quantify the difference between the values It allows calculating the mean and median of the variables To understand the difference between the variables, you can subtract the values between the variables The interval scale is the preferred scale in Statistics as it helps to assign any numerical values to arbitrary assessment such as feelings, calendar types, etc. Example: Likert Scale Net Promoter Score (NPS) Bipolar Matrix Table 4. Ratio Scale The ratio scale is the 4th level of measurement scale, which is quantitative. It is a type of variable measurement scale. It allows researchers to compare the differences or intervals. The ratio scale has a unique feature. It possesses the character of the origin or zero points. Characteristics of Ratio Scale: Ratio scale has a feature of absolute zero It doesn’t have negative numbers, because of its zero-point feature It affords unique opportunities for statistical analysis. The variables can be orderly added, subtracted, multiplied, divided. Mean, median, and mode can be calculated using the ratio scale. Ratio scale has unique and useful properties. One such feature is that it allows unit conversions like kilogram – calories, gram – calories, etc. Example: What is your weight in Kgs? Less than 55 kgs 55 – 75 kgs 76 – 85 kgs 86 – 95 kgs More than 95 kgs