07_Data%20literacy%20and%20strategy.pdf
Document Details
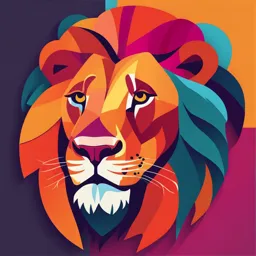
Uploaded by DelightedPolonium
2023
Tags
Full Transcript
Data literacy and strategy MGT001354: AI for Innovation and Entrepreneurship Dr. Peter Hofmann | November 27th, 2023 | Munich 2023 Agenda 1 What makes data valuable? 2 Which data to collect? And how much is enough? 3 How to succeed as a chief data officer? ‹#› What makes data valuable? 4...
Data literacy and strategy MGT001354: AI for Innovation and Entrepreneurship Dr. Peter Hofmann | November 27th, 2023 | Munich 2023 Agenda 1 What makes data valuable? 2 Which data to collect? And how much is enough? 3 How to succeed as a chief data officer? ‹#› What makes data valuable? 4 829 billion euros could be generated in the European data economy by 2025 European Commission SOURCE: European Union (2020) The European Data Strategy. Shaping Europe’s Digital Future. 5 Among leading AI teams, many can likely replicate others’ software in, at most, 1–2 years. But it is exceedingly difficult to get access to someone else’s data. Thus data, rather than software, is the defensible barrier for many businesses. Andrew Ng Former Google Brain 6 Picture: unsplash.com/de/@filmlav 7 Data is the new oil Pictures unsplash.com/de/@robineero 8 Picture: /unsplash.com/de/@luigir 9 Digital vs. analog signals 10 There are a lot of shapes of data and taxonomies classifying it Text Boolean Transactions Integer Images String Audio Array Sensor output Float ... … … 11 What about synthetic data? Perception Analysis Language Planning Generation Recognize structure and patterns in sensory data Find trends, associations and make predictions Understand language and computer code Make tactical or strategic decisions Synthesize new data samples Is the input structured data? E.g., tabular data, graphs Is input or output text or code? Is the output a sequence of steps? Is the output similar to raw sample inputs? E.g., images, sounds Is the input raw data? E.g., images, sensors, video 12 The ontological reversal: Data is not only a representation of the real-world Classical view Information systems represent and reflects physical reality Proposition Digital technologies create and shape physical reality SOURCE: Baskerville et al. (2019) Digital First. MIS Quarterly. 13 Even with excellent information or predictions you only made it halfway Information value chain* data → information → knowledge → action Machine learning* Alternativ 1: data → predictions → knowledge → action Alternative 2: data → predictions → automated/autonomous action FOOTNOTE: The activities required to move forward in the value chain are not strictly sequential, but require an iterative (experimental) approach. 14 There is no value in data unless it can be applied in a use case Traditional reporting and dashboarding to improve internal decision-making Process or service-/ product-centric AI to improve or create new processes or services/products with AI Selling data or data-based services to generate sales revenue 15 Data literacy is the ability to use data productively and to think about it in a critically reflective way SOURCE: Sternkopf and Mueller (2018) Doing Good with Data. HICSS. 16 Processing data involves tools Picture: https://twitter.com/kareem_carr/status/1187010839603232768 17 What can go wrong with no data literacy? 18 False, biased, or misinterpreted output leading to ill-informed decisions 19 Low quality data 20 What can go wrong scanning a document? Picture: https://www.xerox.de/de-de/office-produkte/multifunktionsdrucker/workcentre-pro-232-238 21 It is important to question information Original Scan Sources including pictures: https://www.dkriesel.com/en/blog/2013/0802_xerox-workcentres_are_switching_written_numbers_when_scanning 22 What are the consequences? Pictures: Xerox; unsplash.com/de/@syinq; unsplash.com/de/@jamestarbotton 23 The story behind is worth a watch SOURCE: https://www.youtube.com/watch?v=c0O6UXrOZJo 24 A typical reason why machine learning applications fail Production data differing from training data Train-serving skew SOURCE: CS 329S: Machine Learning Systems Design. Data shifts 25 Biases are diverse and human Confirmation bias Run-to-solve bias Outlier bias Availability bias Selection bias Anchor bias … 26 Why you should keep attention when you process data Grades Average 27 Irrelevant output wastes investments 28 How key business questions lead to actionable knowledge High potential to impact Pipe dreams High-value key business questions Low ability to activate High ability to activate Curiosities Incremental improvements Low potential to impact SOURCE: https://hbr.org/2020/02/use-data-to-answer-your-key-business-questions 29 Missing innovation opportunities 30 Data and business imagination make the difference Data imagination Business imagination Pictures: unsplash.com/de/@xavi_cabrera; unsplash.com/de/@mourimoto; unsplash.com/de/@omaralbeik 31 Data and business imagination makes the difference SOURCE: https://brickit.app/; https://www.youtube.com/shorts/T4WAi5ykQyc 32 Vulnerability to misinformation and data misuse 33 Generative AI could be abused for disinformation campaigns “Degrees of trust will go down, the job of journalists and others who are trying to disseminate actual information will become harder” Ben Winters Electronic Privacy Information Center SOURCE: https://www.theguardian.com/us-news/2023/jul/19/ai-generated-disinformation-us-elections 34 Avoid being fooled with charts SOURCE: https://handsondataviz.org/detect.html 35 Avoid being fooled with charts SOURCE: https://handsondataviz.org/detect.html 36 This does not mean that you should not tell convincing stories Picture: Wiley 37 Limitation of career growth 38 The benefits of data literacy 3/5 74% fastest-growing skill sets across the UK and the US were data skills of respondents agreed or strongly agreed that those with data literacy skills outperformed those with inadequate data skills SOURCE: datacamp (2023) The State Of Data Literacy 2023. 39 What can go wrong with no data literacy False, biased, or misinterpreted output leading to ill-informed decisions Vulnerability to misinformation and data misuse Limitation of career growth Irrelevant output wastes investments Missing innovation opportunities Pictures: Flaticon/geotatah; Flaticon/Color Cods. 40 Which data to collect? And how much is enough? 41 Qualitative data 42 What makes an apple qualitative? Picture: unsplash.com/de/@amit_lahav 43 Its ability to meet your requirements - Picture: unsplash.com/de/@amit_lahav Picnic snack Apple pie … 44 Recap: Relevance High potential to impact Pipe dreams High-value key business questions Low ability to activate High ability to activate Curiosities Incremental improvements Low potential to impact SOURCE: https://hbr.org/2020/02/use-data-to-answer-your-key-business-questions 45 Feature accuracy Degree to which data correctly captures the “real-life” objects/phenomena they are intended to represent Ground truth SOURCE: Adapted from Pipino et al. (2002) Data Quality Assessment. Measurements 46 Target class balance Many ML approaches assume a relatively equal number of samples per target class Fraud detection example Instances of fraud happen once per 200 transactions in this data set SOURCES: https://developers.google.com/machine-learning/data-prep/construct/sampling-splitting/imbalanced-data?hl=en https://arxiv.org/pdf/2207.14529.pdf 47 Completeness Feature 1 The problem of missing values exists in many real-world datasets. SOURCE: https://arxiv.org/pdf/2207.14529.pdf Feature 2 Feature 3 2 2 12 3 NaN 10 NaN NaN NaN 1 23 0 48 Consistency A data set is consistent in its representation if no feature has two or more unique values that are semantically equivalent. I.e., each real-world entity or concept is referred to by only one representation City … New York … NY … New York City … … … Example: List with city names SOURCE: https://arxiv.org/pdf/2207.14529.pdf 49 Uniqueness Redundant data does not provide additional information to the ML-model for the training process. Thus, de-duplication is a common step in ML pipelines to avoid overfitting. DOI* … 10.1007/s12599-023-00842-7 … 10.1016/j.is.2023.102246 … 10.1007/s12599-023-00842-7 … … … Example: List of papers in your literature review *DOI = Digital object identifier SOURCE: https://arxiv.org/pdf/2207.14529.pdf 50 Timeliness The extent to which the data is sufficiently up-to-date for the task SOURCES: https://towardsdatascience.com/data-drift-part-1-types-of-data-drift-16b3eb175006 Adapted from Pipino et al. (2002) Data Quality Assessment. 51 Harnessing the potential of data is not free of charge Data collection costs e.g., data labeling costs, data access fees Data storage costs e.g., standard vs. nearline vs. coldline vs. archive storage Data processing costs Compute requirements depend on data volume 55 Data resources can become a honey pot for attacks SOURCE: https://www.nytimes.com/2017/10/03/technology/yahoo-hack-3-billion-users.html 56 The need to meet regulatory requirements: Principles relating to processing of personal data Lawfulness, fairness and transparency Accuracy Purpose limitation Storage limitation Data minimisation SOURCE: https://eur-lex.europa.eu/legal-content/EN/TXT/HTML/?uri=CELEX:32016R0679&from=EN#d1e1807-1-1 Pictures: Flaticon/Hexagon075; judanna; Freepik; herikus Accountability Integrity and confidentiality 57 There is also an ethical perspective on data collection and use Picture: Profile Books 58 The ambition should be to just jump as high as necessary Picture: unsplash.com/de/@devine_images 59 How to succeed as a chief data officer? 60 What to consider in a data strategy? People, roles & responsibilities Performance management Data architecture Data applications Data lifecycle Processes & methods SOURCE: Legner et al. (2020) Accumulating Design Knowledge with Reference Models. JAIS. 61 What is your data strategy? Defense Ensure data security, privacy, integrity, quality, regulatory compliance, and governance SOURCE: https://hbr.org/2017/05/whats-your-data-strategy Offense Improve competitive position and profitability 62 Recap: Leverage data’s potential Information value chain* data → information → knowledge → action Machine learning* Alternativ 1: data → predictions → knowledge → action Alternative 2: data → predictions → automated/autonomous action FOOTNOTE: The activities required to move forward in the value chain are not strictly sequential, but require an iterative (experimental) approach. 63 Certifications processes not only foster compliance but can create trust ISO/IEC 29100:2011 ISO/IEC 27001 provides a privacy framework which is a standard for information security management systems ● specifies a common privacy terminology; ● defines the actors and their roles in processing personally identifiable information (PII); ● describes privacy safeguarding considerations; and ● provides references to known privacy principles for information technology. Conformity with ISO/IEC 27001 means that an organization or business has put in place a system to manage risks related to the security of data owned or handled by the company SOURCES: https://www.iso.org/standard/85938.html; https://www.iso.org/standard/27001 64 Some data challenges that needs every department’s attention Reproducibility Transparency Traceability I want to reproduce the training of a specific model. Which data set has been used in the initial training? Data has to be used according to the data policies of a company. How can someone else audit my data usage? Data and data sets continuously develop. How can I understand and trace the data development? Accessibility Scalability Specialized data sets are created by one department with a lot of effort. How can these data sets be shared with others? The amount of data increases steadily. How can I organize the large amount of data most efficiently? 65 Data lineage tools make the flow of data from transparent and inspectable Data lineage is the process of tracking the flow of data over time, providing a clear understanding of ● where the data originated, ● how it has changed, and ● its ultimate destination within the data pipeline. SOURCE: https://www.ibm.com/topics/data-lineage 66 An exemplary tradeoff between defense and offense Data access restriction Data democratization 67 Privacy must not be only a corporate burden SOURCE: https://www.apple.com/newsroom/2023/01/apple-builds-on-privacy-commitment-by-unveiling-new-efforts-on-data-privacy-day/ 68 “Skywise connects the aviation industry’s in-flight, engineering, and operations data in a secure ecosystem and is used by suppliers, as well as over 100 airlines. 10.500+ aircrafts 26.900+ users 27+ apps & datasets Third-party analysis estimates that the platform creates a revenue opportunity exceeding $850mn/annum and enables cost savings of greater than $1.7bn/annum.“ SOURCES: https://aircraft.airbus.com/en/services/enhance/skywise/skywise-solutions; https://www.palantir.com/assets/xrfr7uokpv1b/7uEHPTEM0MkKtBFcx2zh63/9d75da5b76439717ac95135b5012479e/Palantir-Airbus-Partnership_Overview.pdf 69 “This project allows the pharma partners for the first time to collaborate in their core competitive space, invigorating discovery efforts through efficiency gains.” Hugo Ceulemans, Project Leader, Janssen Pharmaceutica NV SOURCE: https://www.melloddy.eu/ 70 Data sharing ecosystems “Data sharing ecosystems arise when organizations agree to share data and insights under locally applicable regulations to create new value for all participants.” ● Data brokerage and aggregation ecosystem ● Reciprocal data-sharing ecosystem ● Federated analytics ecosystem ● Collaborative data-supply-chain ecosystem SOURCE: https://www.capgemini.com/wp-content/uploads/2021/09/Final-Web-Version-of-Report-Data-Ecosystems-1.pdf 71 63% of the companies surveyed in Germany do not yet exchange data SOURCE: https://www.bitkom.org/sites/main/files/2022-05/Bitkom-Charts_Datenökonomie_04_05_2022_final.pdf 72 Key challenges in exchanging data in industrial ecosystems Culture and mindset The need for ontologies Technological challenges Management/ administration challenges SOURCE: https://www.mckinsey.de/~/media/mckinsey/locations/europe%20and%20middle%20east/deutschland/publikationen/data%20sharing%20in%20industrial%20e cosystems/mckinsey_article_data_sharing_in_industrial_ecosystems.pdf 74 Offense wins games (but) defense wins championships Picture: unsplash.com/de/@24ameer 76