Transportation, Transshipment, and Assignment Problems PDF
Document Details
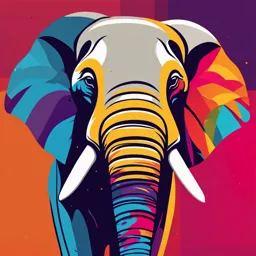
Uploaded by ResoluteConnemara508
Tags
Summary
This document discusses transportation, transshipment, and assignment problems as special types of linear programming. It gives examples of transportation models applied to wheat shipments. The mathematical formulations of the models are highlighted.
Full Transcript
CHAPTER 6: TRANSPORTATION, TRANSSHIPMENT, AND ASSIGNMENT PROBLEMS Overview In this chapter, we examine three special types of linear programming model formulations— transportation, transshipment, and assignment problems. They are part of a larger class of linear programming problems known as netwo...
CHAPTER 6: TRANSPORTATION, TRANSSHIPMENT, AND ASSIGNMENT PROBLEMS Overview In this chapter, we examine three special types of linear programming model formulations— transportation, transshipment, and assignment problems. They are part of a larger class of linear programming problems known as network flow problems. These problems have special mathematical characteristics that have enabled management scientists to develop very efficient, unique mathematical solution approaches to them. These solution approaches are variations of the traditional simplex solution procedure. Like the simplex method, we have placed these detailed manual, mathematical solution procedures—called the transportation method and assignment method—on the companion Web site that accompanies this text. Learning Outcomes At the end of this lesson, students must be able to; 1. Differentiate the three special types of linear programming model formulations ( transportation, transhipment and assignment models) 2. Apply the computer solution for these types of linear programming models COURSE MATERIALS The Transportation Model 67 The transportation model is formulated for a class of problems with the following unique characteristics: (1) A product is transported from a number of sources to a number of destinations at the minimum possible cost; and (2) each source is able to supply a fixed number of units of the product, and each destination has a fixed demand for the product. Although the general transportation model can be applied to a wide variety of problems, it is this particular application to the transportation of goods that is most familiar and from which the problem draws its name. The following example demonstrates the formulation of the transportation model. Wheat is harvested in the Midwest and stored in grain elevators in three different cities—Kansas City, Omaha, and Des Moines. These grain elevators supply three flour mills, located in Chicago, St. Louis, and Cincinnati. Grain is shipped to the mills in railroad cars, each car capable of holding 1 ton of wheat. Each grain elevator is able to supply the following number of tons (i.e., railroad cars) of wheat to the mills on a monthly basis: The cost of transporting 1 ton of wheat from each grain elevator (source) to each mill (destination) differs, according to the distance and rail system. (For example, the cost of shipping 1 ton of wheat from the grain elevator at Omaha to the mill at Chicago is $7.) These costs are shown in the following table: The problem is to determine how many tons of wheat to transport from each grain elevator to each mill on a monthly basis to minimize the total cost of transportation. A diagram of the different transportation routes, with supply and demand, is given in Figure 6.1. 68 Figure 6.1 Network of transportation routes for wheat shipments The linear programming model for this problem is formulated as follows: In this model the decision variables, xij, represent the number of tons of wheat transported from each grain elevator, i (where i = 1, 2, 3), to each mill, j (where j = A, B, C). The objective function represents the total transportation cost for each route. Each term in the objective function reflects the cost of the tonnage transported for one route. For example, if 20 tons are transported from elevator 1 to mill A, the cost ($6) is multiplied by x1A(=20), which equals $120. The first three constraints in the linear programming model represent the supply at each elevator; the last three constraints represent the demand at each mill. As an example, consider the first supply constraint, x1A + x1B + x1C = 150. This constraint represents the tons of wheat transported from Kansas City to all three mills: Chicago (x1A), St. Louis (x1B) and Cincinnati (x1C) The amount transported from Kansas City is limited to the 150 tons available. Note that 69 this constraint (as well as all others) is an equation (=) rather than a (≤) inequality because all the tons of wheat available will be needed to meet the total demand of 600 tons. In other words, the three mills demand 600 total tons, which is the exact amount that can be supplied by the three grain elevators. Thus, all that can be supplied will be, in order to meet demand. This type of model, in which supply exactly equals demand, is referred to as a balanced transportation model. Realistically, however, an unbalanced problem, in which supply exceeds demand or demand exceeds supply, is a more likely occurrence. In our wheat transportation example, if the demand at Cincinnati is increased from 300 tons to 350 tons, a situation is created in which total demand is 650 tons and total supply is 600 tons. This will result in the following change in our linear programming model of this problem: One of the demand constraints will not be met because there is not enough total supply to meet total demand. If, instead, supply exceeds demand, then the supply constraints will be ≤ Sometimes one or more of the routes in the transportation model may be prohibited. That is, units cannot be transported from a particular source to a particular destination. When this situation occurs, we must make sure that the variable representing that route does not have a value in the optimal solution. This can be accomplished by assigning a very large relative cost as the coefficient of this prohibited variable in the objective function. Computer Solution of a Transportation Problem Because a transportation problem is formulated as a linear programming model,it can be solvedwith Excel and using the linear programming module of QM for Windows Computer Solution with Excel We will first demonstrate how to solve a transportation problem by using Excel. A transportation problem must be solved in Excel as a linear programming model, using Solver, as demonstrated in Chapters 3 and 4. Exhibit 6.1 shows a spreadsheet setup to solve our wheat-shipping example. Notice that the objective function formula for total cost is contained in cell C10 and is shown on the formula bar at the top of the spreadsheet. It is constructed as a SUMPRODUCT of the decision variables in cells C5:E7 and a cost array in cells K5:M7. Cells G5:G7 in column G and cells C9:E9 in row 9, labeled ―Grain Shipped,‖contain the constraint formulas for supply and demand, respectively. For example, the constraint formula in cell G7 for the grain shipped from Des Moines to the three mills isand the right-hand-side quantity value of 275 available tons is in cell F7. 70 Exhibit 6.1 Exhibit 6.2 shows the Solver Parameters window for our example. Cell C10,containing the objective function formula, is minimized. The constraint formula includes all three demand constraints, and the constraint formula includes all three supply constraints. Before solving this problem, remember to select ―Simplex.LP‖ to invoke the linear programming solution approach. Exhibit 6.2 Exhibit 6.3 shows the optimal solution,with the amounts shipped from sources to destina-tions and the total cost. Figure 6.2 shows a network diagram of the optimal shipments. 71 Exhibit 6.3 Figure 6.2 Transportation network solution for wheat-shipping example Computer Solution with Excel QM Excel QM, which was introduced in Chapter 1, includes a spreadsheet macro for transportation problems. When Excel QM is activated, clicking on ―Transportation‖ from the drop-down window will result in the Spreadsheet Initialization window, as shown in Exhibit 6.4. In this window, we set the number of sources (i.e., origins) and destinations to 3, select ―Minimize‖ as the objective, and type in the title for our example. To exit this window, we click on ―OK,‖ and the spreadsheet shown in Exhibit 6.5 is displayed. The transportation problem is completely set up as shown, with all the necessary formulas already in the cells. However, initially the data values in cells B10:E13 are empty; the spreadsheet in Exhibit 6.5 includes the values that we have typed in. Exhibit 6.4 72 Exhibit 6.5 To solve the problem,we follow the instructions in the box superimposed on the spreadsheetin Exhibit 6.5—click on ―Data‖at the top of the spreadsheet,then click on ―Solver,‖and whenSolver appears,click on ―Solve.‖We have not shown the Solver Parameters window here; how-ever,it is the same Excel Solver as shown in all previous chapters. It already includes all thedecision variables and constraints required to solve the problem; thus, all that is needed is to clickon ―Solve.‖The spreadsheet with the solution is shown in Exhibit 6.6. You will notice that although the total cost is the same as in the solution we obtained with Excel in Exhibit 6.3,the decision variable values in cells B17:D19are different. This is because this problem has mul-tiple optimal solutions,and this is the alternate solution to the one we got previously in Excel. 73 QM for Windows Solution To access the transportation module for QM for Windows, click on ―Module‖ at the top of the screen and then click on ―Transportation.‖ Once you are in the transportation module, click on ―File‖ and then ―New‖ to input the problem data. QM for Windows allows for any of three initial solution methods—northwest corner, minimum cell cost, or VAM—to be selected. These are the three starting solution procedures used in the mathematical procedure for solving transportation problems. Because these techniques are not included in the text (but are included on the Web site Module B transportation and assignment problems), it makes no difference which starting procedure we use. Exhibit 6.7 shows the input data for our wheat-shipping example. Exhibit 6.7 Once the data are input, clicking on ―Solve‖ at the top of the screen will generate the solution, as shown in Exhibits 6.8 and 6.9. QM for Windows will indicate if multiple optimal solutions exist, but it will not identify alternate solutions. This is the same optimal solution that we achieved earlier in our Excel QM solution. Exhibits 6.8 and 6.9 show the solution results in two different tables, or formats. Exhibit 6.8 shows the shipments in each cell (or decision variable) plus total cost, and Exhibit 6.9 lists the individual shipments from each source to destination and their costs. 74 Exhibit 6.8 Exhibit 6.9 Sensitivity Analysis Once a transportation problem is set up in any of the software formats—Excel, Excel QM, or QM for Windows—it is simple to perform sensitivity analysis by changing the model size or parameters. For example, we’ll change our wheat-shipping example to reflect the unbalanced conditions, where the demand at Cincinnati is 350 tons, resulting in demand exceeding available supply. We’ll also increase the shipping cost from Des Moines to St. Louis from $5/ton to $7/ton. The new Excel solution to this revised version of our example isshown in Exhibit 6.10. Note that we could have handled the extra demand in two ways:Wecould have made the demand constraintsconstraints in Solver,or we could create an extrarow with ―slack‖variables to take up the amount demanded but not supplied. We chose thesecond method,as shown in cells C8:E8 in Exhibit 6.10. 75 Exhibit 6.10 The Transshipment Model The transshipment model is an extension of the transportation model in which intermediate transshipment points are added between the sources and destinations. An example of a transshipment point is a distribution center or warehouse located between plants and stores. In a transshipment problem, items may be transported from sources through transshipment points on to destinations, from one source to another, from one transshipment point to another, from one destination to another, or directly from sources to destinations, or some combination of these alternatives. We will expand our wheat-shipping example to demonstrate the formulation of a transshipment model. Wheat is harvested at farms in Nebraska and Colorado before being shipped to the three grain elevators in Kansas City, Omaha, and Des Moines, which are now transshipment points. The amount of wheat harvested at each farm is 300 tons. The wheat is then shipped to the mills in Chicago, St. Louis, and Cincinnati. The shipping costs from the grain elevators to the mills remain the same, and the shipping costs from the farms to the grain elevators are as follows: The basic structure of this model is shown in the graphical network in Figure 6.3. 76 Figure 6.3 Network of transhipments routes As with the transportation problem, a linear programming model is developed with supply-and- demand constraints. The available supply constraints for the farms in Nebraska and Colorado are The demand constraints at the Chicago, St. Louis, and Cincinnati mills are Next we must develop constraints for the grain elevators (i.e., transshipment points) at Kansas City, Omaha, and Des Moines. To develop these constraints we follow the principle that at each transshipment point, the amount of grain shipped in must also be shipped out. For example, the amount of grain shipped into Kansas City is x13 + x23 and the amount shipped out is x36 +x37 + x38 Thus, because whatever is shipped in must also be shipped out, these two amounts must equal each other: x13 + x23 = x36 + x37 + x38 The transshipment constraints for Omaha and Des Moines are constructed similarly: 77 x14 + x24 + x46 + x47 + x48 = 0 x15 + x25 – x56 + x57 + x58 = 0 The complete linear programming model, including the objective function, is summarized as follows: Computer Solution with Excel Because the transshipment model is formulated as a linear programming model, it can be solved with either Excel or QM for Windows. Here we will demonstrate its solution with Excel. Exhibit 6.11 shows the spreadsheet solution and Exhibit 6.12 the Solver for our wheatshipping transshipment example. A network diagram of the optimal solution is shown in Figure 6.4. The spreadsheet is similar to the original spreadsheet for the regular transportation problem in Exhibit 6.1 except that there are two tables of variables—one for shipping from the farms to the grain elevators and one for shipping grain from the elevators to the mills. Thus, the decision variables (i.e., the amounts shipped from sources to destinations) are in cells B6:D7 and C13:E15. The constraint for the amount of grain shipped from the farm in Nebraska to the three grain elevators (i.e., the supply constraint for Nebraska) in cell F6 is =SUMB6:D6, which sums cells B6+C6+D6. The amount of grain shipped to Kansas City from the farms in cell B8 is =SUM(B6:B7). Similar constraints are developed for the shipments from the grain elevators to the mills. 78 Exhibit 6.11 Exhibit 6.12 Two cost arrays have been developed for the shipping costs in cells I6:K7 and cells J13:L15,which are then multiplied by the variables in cells B6:D7 and C13:E15 and added together. The objective function, =SUMPRODUCT(B6:D7,16:K7)+SUMPRODUCT(C13:E15,J13:L15), is shown on the toolbar at the top of Exhibit 6.11. Constructing the objective function with cost arrays like this is a little easier than typing in all the variablesand costs in a single objective function when there are many variables and costs. A networkdiagram of the optimal solution is shown in Figure 6.4. Figure 6.4 Transshipment network solution for wheat-shipping example 79 The Assignment Model The assignment model is a special form of a linear programming model that is similar to the transportation model. There are differences, however. In the assignment model, the supply at each source and the demand at each destination are each limited to one unit. The following example will demonstrate the assignment model. The Atlantic Coast Conference (ACC) has four basketball games on a particular night. The conference office wants to assign four teams of officials to the four games in a way that will minimize the total distance traveled by the officials. The supply is always one team of officials, and the demand is for only one team of officials at each game. The distances in miles for each team of officials to each game location are shown in the following table: The linear programming formulation of the assignment model is similar to the formulation of the transportation model, except all the supply values for each source equal one, and all the demand values at each destination equal one. Thus, our example is formulated as follows: This is a balanced assignment model. An unbalanced model exists when supply exceeds demand or demand exceeds supply. Computer Solution of an Assignment Problem The assignment problem can be solved using the assignment modules in QM for Windows and Excel QM, and with Excel spreadsheets. We will solve our example of assigning ACC officials 80 to game sites first by using Excel, followed by Excel QM, and then by using QM for Windows. Computer Solution with Excel As is the case with transportation problems, Excel can be used to solve assignment problems, but only as linear programming models. Exhibit 6.13 shows an Excel spreadsheet for our ACC basketball officials example. The objective function in cell C11 was developed by creating a mileage array in cells C16:F19 and multiplying it by the decision variables in cells C5:F8. The model constraints for available teams (supply) are contained in the cells in column H, and the constraints for teams of officials at the game sites (demand) are contained in the cells in row 10. Exhibit 6.13 Exhibit 6.14 shows the Solver Parameters screen for our example. Before clicking on ―Solve,‖ remember to invoke ―Simplex LP‖ to solve as a linear programming model. The optimal solutionis shown in Exhibit 6.15. A network diagram of the optimal solution is shown in Figure 6.5. Exhibit 6.14 Computer Solution with Excel QM Excel QM also includes a spreadsheet macro for assignment problems. It is very similar to the ExcelQM macro demonstrated earlier in this chapter for the transportation problem,and the 81 spreadsheetsetup is very much like that of the Excel spreadsheet in Exhibit 6.15. The assignment macro isaccessed (from the ―Excel QM‖menu) and applied similarly to the transportation problem macro. Italready includes all the cell and constraint formulas necessary to solve the problem. The solution toour ACC basketball example with Excel QM is shown in Exhibit 6.16. Exhibit 6.15 Figure 6.5 Assignment network solution for ACC officials example Exhibit 6.16 Computer Solution with QM for Windows The data input for our example is shown in Exhibit 6.17, followed by the solution in Exhibit 6.18. 82 Exhibit 6.17 Exhibit 6.18 Activities/Assessments Business Problem A concrete company transports concrete from three plants to three construction sites. The supply capacities of the three plants, the demand requirements at the three sites, and the transportation costs per ton are as follows: Solution: The Linear Programming Model Formulation 83 Excel Solution Given the above information: 1. Determine the decision variables, objective function and constraints of this problem. 2. Using your own excel Solver, show how you arrive on the solution. (Include steps and screenshot) Note: If you don’t have a laptop/computer or Excel Solver for No.2, create (write) the steps based on the previous discussions and modules and use the above Excel spreadsheet as reference for the cell numbers etc. 84