AI Use Cases PDF
Document Details
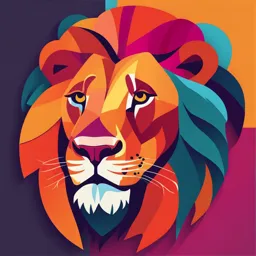
Uploaded by DelightedPolonium
Technische Universität München
Tristan
Tags
Related
Summary
This document provides an overview of AI use cases, focusing on ideation, evaluation, and prioritization techniques. It explores AI capabilities and offers insights into making informed decisions about AI application, whether for startup validation or organizational implementation.
Full Transcript
AI Use Cases AI for Innovation and Entrepreneurship Tristan | November 20th, 2023 | TUM The Ideation Process ‹#› AI Use Case: Ideation, Evaluation, Priorization 20th November Module Overview The "AI Use Case: Ideation, Evaluation, Prioritization" module is meticulously designed to guide...
AI Use Cases AI for Innovation and Entrepreneurship Tristan | November 20th, 2023 | TUM The Ideation Process ‹#› AI Use Case: Ideation, Evaluation, Priorization 20th November Module Overview The "AI Use Case: Ideation, Evaluation, Prioritization" module is meticulously designed to guide students through the multifaceted process of identifying, evaluating, and prioritizing AI use cases. This session will explore the diverse capabilities of AI, offering techniques to ideate potential AI applications. Students will learn how to critically assess and prioritize AI use cases, focusing on value delivery and ease of implementation. Additionally, discussions on 'make or buy' strategies will provide insights into resource allocation for optimal outcomes. This module is a comprehensive guide to strategically navigating the landscape of AI use case development, whether for startup validation or organizational implementation. Why Attend This Session This session is crucial for anyone looking to validate AI startup ideas or decide which AI use cases to develop and allocate budget to within an organization. It provides practical knowledge and techniques for ideating, evaluating, and prioritizing AI use cases effectively, ensuring the selection of the most valuable and feasible options. Attendees will gain insights into making informed decisions on AI application, whether it’s about validating the viability of startup ideas or optimizing resource allocation for AI development in organizations. This module empowers students to strategically leverage AI capabilities for maximum impact and value creation. ‹#› AI Use Case: Ideation, Evaluation, Priorization Learning Objectives 1. Understanding of AI Capabilities: Explore the diverse capabilities of AI, gaining insights into what AI enables us to achieve and how it can be leveraged for various applications. 2. Skills in AI Use Case Ideation: Learn techniques for ideating AI use cases, enhancing the ability to conceptualize potential AI applications and solutions effectively. 5. Strategic Decision-Making in AI Application: Develop the skills to make strategic decisions on AI use case development and budget allocation, whether for validating startup ideas or for organizational implementation. . 3. Proficiency in Evaluating and Prioritizing AI Use Cases: Acquire the knowledge to critically assess and prioritize AI use cases, focusing on high-value and feasible options for optimal outcomes. 4. Insights into 'Make or Buy' Strategy: Understand the principles of 'make or buy' strategy in AI, gaining the ability to make informed decisions on resource allocation and AI development. ‹#› Agenda 1 Lorem Ipsum Inspiration 3 Make or Buy 1.1. Lorem Ipsum 2 1.2. AI Knowledge AI Use Case Lorem Ipsum 3 Idea Generation 2.1. Lorem Ipsum 2.2. Lorem Ipsum 4 2.3. Lorem Ipsum Prioritization Lorem Ipsum 2.1. Lorem Ipsum 2.2. Lorem Ipsum 2.3. Lorem Ipsum ‹#› The Ideation Process ‹#› ‹#› Inspiration ‹#› The Ideation Process ‹#› Machine Capabilities Perception Capabilities Analytical Capabilities • Comp. Vision • Discovery • Comp. Audition • Forecasting • Comp. Linguistics • Planning & Optimization Motoric Capabilities Generating Capabilities • Advanced Robotics & Control • Creation ‹#› Chaban, M. A. V. (2022, September 16). Speedy recovery: How AI research is helping insurers boost claims response. Google Cloud Blog. Retrieved from https://cloud.google.com/transform/insurance-ai-disaster-recovery-claims-response-munichre-google-research?hl=en and Mueller, H., Groeger, A., Hersh, J., Matranga, A., & Serrat, J. (2021). Monitoring war destruction from space using machine learning. Proceedings of the National Academy of Sciences, 118(23), e2025400118. https://doi.org/10.1073/pnas.2025400118​ ‹#› Satellite image analysis being used for damage detection of natural catastrophes (left) and war aftermath (right) ‹#› Dual Use: "The capability of AI technologies to serve both civilian and military applications." ‹#› AI Use Case ‹#› AI Use Cases perception language planning Clearly defined set of activities designed to reach a specific goal from a business or costumer perspective, in which one or more AI solution are involved in reaching the perspective goal Use Cases use AI capabilities like tools in a toolbox generation analysis ‹#› AI Use Cases Clearly defined set of activities designed to reach a specific goal from a business or costumer perspective, in which one or more AI solution are involved in reaching the perspective goal ‹#› Janssen, S. (2019, December 16). Sports Video Analysis using Machine Learning. LinkedIn. Retrieved from https://www.linkedin.com/pulse/sports-video-analysis-using-machine-learning-stephan-janssen/ ‹#› ‹#› Conceptualising Use Cases ‹#› Idea Generation ‹#› The Ideation Process ‹#› ‹#› Ideating and Evaluating AI ‹#› Design Thinking Process ‹#› Ideating and Evaluating AI ‹#› The Costumer Journey ‹#› Costumer Journey at Parkinglot ‹#› The Process Map ‹#› ‹#› Ideating and Evaluating AI ‹#› Ideating and Evaluating AI ‹#› Ideating and Evaluating AI ‹#› ‹#› ‹#› ‹#› Emotion Recognition Siam, Ali & Soliman, Naglaa & Algarni, Abeer & Abd El-Samie, Fathi & Sedik, Ahmed. (2022). Deploying Machine Learning Techniques for Human Emotion Detection. Computational Intelligence and Neuroscience. 2022. 10.1155/2022/8032673. 3D Reconstruction Saikia, T. (2023). 3D-R2N2: A Unified Approach for Single and Multi-view 3D Object Reconstruction. [PowerPoint slides]. University of Freiburg. ‹#› Ideating and Evaluating AI ‹#› ‹#› Use Case Evaluation ‹#› The Ideation Process ‹#› The Market exploded ‹#› Assessing an UC in two Dimensions Assessing an UC in two Dimensions Assessing an UC in two Dimensions Assessing an UC in two Dimensions Assessing an UC in two Dimensions ‹#› Assessing an UC in two Dimensions Example: Smart Parking Haponik, A. (2022, August 18). How can AI help optimize smart parking? Addepto Blog. Assessing an UC in two Dimensions Assessing an UC in two Dimensions Assessing an UC in two Dimensions AI Red Flags AI red flags are critical issues such as regulatory compliance, ethical dilemmas, cybersecurity vulnerabilities, and human-centric challenges that can significantly impede the successful implementation of AI systems. Navigating complex and evolving regulatory frameworks and ethical considerations presents significant challenges in AI implementation. Ensuring robust cybersecurity measures is critical, as AI systems are increasingly targeted by sophisticated cyber threats. Addressing the human factor, including workforce adaptation and public perception, is essential for successful AI integration and acceptance. ‹#› Prioritization ‹#› The Ideation Process ‹#› ‹#› The Pareto Principle ‹#› Prioritization Matrix ‹#› Prioritization Matrix ‹#› Prioritization Matrix ‹#› Prioritization Matrix ‹#› Clustering and Selecting UCs ‹#› Clustering and Selecting UCs ‹#› Clustering and Selecting UCs ‹#› Clustering and Selecting UCs ‹#› Prioritization Matrix ‹#› Prioritization Matrix ‹#› Prioritization Matrix ‹#› An Iterative Process ‹#› Li, Y. (April 7). Meta: segment 'anything anywhere all at once'. WIRED. Retrieved from https://wired.me/technology/segment-anything-all-at-once/. ‹#› ‹#› The Ideation Process ‹#› Return on AI ‹#› Deepc.ai ‹#› AI Value Assessment ‹#› ‹#› ‹#› ‹#› ‹#› Lorem Ipsum ‹#› ‹#› ‹#› Make or Buy ‹#› Development Choices ‹#› Airbus.com Make or Buy ‹#› ‹#› Data: Thinking, Literacy, Strategy 27th November Module Overview The "Data: Thinking, Literacy, Strategy" module is designed to delve deep into the essence of data as the driving force behind the AI Age. This session will explore the importance of data thinking and data literacy, essential components in leveraging data effectively. Participants will learn how to formulate robust data strategies, understanding the pivotal role of data in fueling AI innovations. This module aims to provide a comprehensive insight into the multifaceted nature of data and its strategic utilization in the realm of AI. Why Attend This Session This session is crucial for anyone aspiring to master the art and science of data in the context of AI. It provides invaluable insights into data thinking and literacy, empowering participants to harness the full potential of data. Attendees will learn how to craft effective data strategies, enhancing their ability to navigate the data-driven landscape of AI. Whether you are a data enthusiast, a budding AI innovator, or a strategic thinker, this module will equip you with the knowledge and skills to think, understand, and strategize data effectively in the AI Age. ‹#› Data: Thinking, Literacy, Strategy Learning Objectives 1. Mastery of Data Thinking: Develop a profound understanding of data thinking, enhancing the ability to conceptualize and leverage data effectively in AI applications. 5. Enhanced Strategic Utilization of Data: Delve deep into the strategic utilization of data, acquiring the knowledge to harness data effectively for optimal outcomes in AI. . 2. Proficiency in Data Literacy: Acquire essential skills in data literacy, gaining the ability to interpret, manage, and utilize data efficiently in the AI domain. 3. Insights into Data Strategy Formulation: Learn the intricacies of crafting robust data strategies, understanding the strategic alignment of data in driving AI innovations. 4. Understanding of Data’s Pivotal Role in AI: Gain insights into the importance of data as the fuel for the AI Age, developing a comprehensive perspective on the multifaceted nature of data in AI. ‹#›