Week 5 Lecture 5 PDF
Document Details
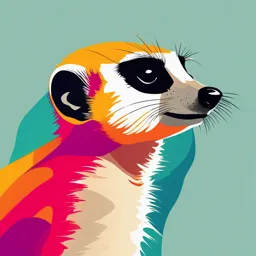
Uploaded by VictoriousElf1785
Bournemouth University
Bryan L
Tags
Summary
This document is a lecture on t-tests and hypothesis testing. It covers different types of t-tests, such as independent samples t-test and repeated measures t-test.
Full Transcript
Week 5: Testing differences between two groups or conditions What are t-tests? Today’s objectives 01 Differences between two groups: Independent Sample/Between subject 02 Differences between two variables: Repeated Measures/W ithin subject 03 How to write-up th...
Week 5: Testing differences between two groups or conditions What are t-tests? Today’s objectives 01 Differences between two groups: Independent Sample/Between subject 02 Differences between two variables: Repeated Measures/W ithin subject 03 How to write-up the ‘Results’ section? Recap: Hypothesis testing How to examine differences between two groups Confidence interval (95% CI): Remember, we often estimate (with 95% confidence) that the population mean is within a specific range/interval. Recap: Hypothesis testing How to examine differences between two groups Confidence interval (95% CI): Remember, we often estimate (with 95% confidence) that the population mean is within a specific range/interval. If 95% CI error bars do not overlap, you can be relatively certain that there are differences between the conditions Recap: Hypothesis testing How to examine differences between two groups Confidence interval (95% CI): Remember, we often estimate (with 95% confidence) that the population mean is within a specific range/interval. If 95% CI error bars do not overlap, you can be relatively certain that there are differences between the conditions However, we may not always obtain zero overlaps between error bars… Recap What are the types of ‘hypothesis testing’? 1. Differences 2. Correlations 3. Interactions Recap What are the types of ‘hypothesis testing’? 1. Differences 2. Correlations 3. Interactions Differences between two groups: Independent Sample Attention in ‘coffee drinkers’ vs. ‘non-coffee drinkers’ Memory in ‘individuals with dementia’ vs. ‘healthy typical adults’ Effectiveness of treatment in ‘placebo’ vs ‘cognitive therapy’ Sex differences, e.g., ‘male’ vs ‘female’. To examine such differences, you will need a between-subject design. Differences between two groups: Independent Sample Attention in ‘coffee drinkers’ vs. ‘non-coffee drinkers’ Remember, a between-subject design means you separate your sample into distinct groups! In our case, two different groups were used based on their coffee drinking habit. Coffee drinkers Non-Coffee drinkers Differences between two groups: Independent Sample Attention in ‘coffee drinkers’ vs. ‘non-coffee drinkers’ Let’s imagine, we recruited 24 participants, one half of the participants had to drink 2 cups of coffee at the start of the experiment, the other half only had water. Differences between two groups: Independent Sample Attention in ‘coffee drinkers’ vs. ‘non-coffee drinkers’ Let’s imagine, we recruited 24 participants, one half of the participants had to drink 2 cups of coffee at the start of the experiment, the other half only had water. We then gave them a task to find Waldo! We recorded their detection duration. Differences between two groups: Independent Sample Attention in ‘coffee drinkers’ vs. ‘non-coffee drinkers’ If coffee actually improved attention, we would observe a faster detection speed. To do this, we will need to compare between the two groups. i.e., if I would measure all the population (without measuring error), would their mean be different? Differences between two groups: Independent Sample Attention in ‘coffee drinkers’ vs. ‘non-coffee drinkers’ H0: No differences in detection speed H1: Differences in detection speed You found that ‘coffee drinkers’ had a mean reaction time of 3 seconds, while ‘non coffee drinkers’ had a mean reaction time of 5 seconds… can we reject the null hypothesis? Differences between two groups: Independent Sample Attention in ‘coffee drinkers’ vs. ‘non-coffee drinkers’ H0: No differences in detection speed H1: Differences in detection speed You found that ‘coffee drinkers’ had a mean reaction time of 3 seconds, while ‘non coffee drinkers’ had a mean reaction time of 5 seconds… can we reject the null hypothesis? NO! Because we don’t know if we discovered something or what we measured only happened by chance? (e.g., hypothesis testing) Recap: Hypothesis testing Probability density function Under a set of assumptions, we can determine the probability of an effect of probability density coffee, i.e., s, in case there is no effect (null hypothesis: H0). We use the probability density function to determine whether the effect s we unlikely unlikely observed is likely or not, under H0. likely all possible values of s Differences between two groups: Independent Sample How to examine differences between two groups Independent sample t-test: Created by William Sealy Gosset (a scientist in Guinness) Since Guinness did not want their competitors to know their measurements for their brew, he published the article under the pseudonym “Student”. Hence, you might also see that this test termed as Student’s t-test. Differences between two groups: Independent Sample How to examine differences between two groups Independent sample t-test: ഥ𝐁 𝐀 ഥ t = standardized measure of the difference between the sample means 𝐴ҧ − 𝐵ത 𝑡= Variance 2 2 𝑆𝐴 + 𝑆𝐵 σ 𝑛 𝑖=1 (𝐵𝑖 − ത 𝐵) 2 𝑆𝐵2 = 𝑁 𝑁−1 Differences between two groups: Independent Sample How to examine differences between two groups Systematic variance ഥ𝐁 𝐀 ഥ Unsystematic variance 𝐴ҧ − 𝐵ത 𝑆𝐵2 𝑡= 𝑆𝐴2 2 2 𝑆𝐴 + 𝑆𝐵 𝑁 Differences between two groups: Independent Sample How to examine differences between two groups Systematic variance ഥ𝐁 𝐀 ഥ Variation as consequence of the differences between the two groups, e.g., manipulation of your IV Unsystematic variance 𝑆𝐵2 Variation due to uncontrolled factors, e.g., 𝑆𝐴2 measuring noise, individual differences. t = how many times the systematic variance is larger than the unsystematic variance (ratio) Differences between two groups: Independent Sample How to examine differences between two groups Let’s say we obtained t = 0.5168… ഥ𝐁 𝐀 ഥ Is this big enough to reject H0? 𝑆𝐵2 𝑆𝐴2 Differences between two groups: Independent Sample How to examine differences between two groups Let’s say we obtained t = 0.5168… Is this big enough to reject H0? No. Assuming H0, it is likely (more than 5% chance) to observe the t value we observed, therefore we cannot reject H0. E.g., We failed to show a significant difference between the mean of A and B t = 0.5168 𝛂=0.05 Differences between two groups: Independent Sample How to examine differences between two groups Let’s say we obtained t = 2.3 Is this big enough to reject H0? t = 2.3 𝛂=0.05 Differences between two groups: Independent Sample How to examine differences between two groups Let’s say we obtained t = 2.3 Is this big enough to reject H0? Yes! Assuming H0, it is unlikely (less than 5% chance) to observe the t value we observed, therefore we can reject H0. E.g., There is a significant difference between the mean of A and B t = 2.3 𝛂=0.05 Differences between two groups: Independent Sample How to examine differences between two groups What is “unlikely”? 𝛂: probability of rejecting H0 when it is true, i.e., the probability of the unlikely range We arbitrarily define 𝛂 = 0.05. We want 𝛂 small, but not too small. If not, we will never find an effect! 𝛂=0.05 Differences between two groups: Independent Sample How to examine differences between two groups The ‘p-value’ We associate t to a probability P-value: probability of obtaining t at least as extreme as the t actually observed 𝛂=0.05 Differences between two groups: Independent Sample How to examine differences between two groups The ‘p-value’ If p <.05 (p is less than 0.05), it means t is within the unlikely range. t>2 p < 0.05 𝛂=0.05 Differences between two groups: Independent Sample How to examine differences between two groups The ‘p-value’ If p >.05 (p is more than 0.05), it means t is within the likely range. t = 0.5 p > 0.05 𝛂=0.05 Differences between two groups: Independent Sample How to examine differences between two groups The ‘p-value’ If p >.05 (p is more than 0.05), it means t is within the likely range. This suggest that the ‘shape’ of t distribution is important, as it affects where p will lie. t = 0.5 p > 0.05 𝛂=0.05 Differences between two groups: Independent Sample How to examine differences between two groups The “shape” of t is determined by degrees of freedom (df) Differences between two groups: Independent Sample How to examine differences between two groups The “shape” of t is determined by degrees of freedom (df) Number of observations free to vary when estimating the mean in the population df = N – 1 You can often obtain your df from the sample size! Differences between two groups: Independent Sample How to examine differences between two groups The “shape” of t is determined by degrees of freedom (df) Number of observations free to vary when estimating the mean in the population Since independent sample t-tests consist of two independent samples, the df = N-2 (i.e., df = (N1 – 1) + (N2 – 1)) If you have 20 participants across two groups, your df is 18! Differences between two groups: Independent Sample What is degrees of freedom??? First + Second number = 10 Degrees of freedom (df) Let's imagine that you can choose 2 numbers without constraints, you will have df of 2. Differences between two groups: Independent Sample What is degrees of freedom??? 8 + Second number = 10 Degrees of freedom (df) However, if we say the sum must be 10, and your first number was 8, your second choice 8 is fixed (i.e., 2), you have no choice in that matter. ? Thus, the degree of freedom is reduced by 1 (due to the imposed constraint). Now the df is 1, because you only have one choice. Differences between two groups: Independent Sample What is degrees of freedom??? Degrees of freedom (df) If we imposed more constraints (more variables), df becomes smaller, and this makes it easier to find an effect (e.g., see yellow line). The probability of obtaining t > 2 becomes ‘wider’ as my df becomes lesser. Logically, if you have more constraints, it will be easier to identify the effect of any one of these constraints. Differences between two groups: Independent Sample The assumptions to be met in Independent t-tests H0 is true Before we can conclude this, we have to assume that: 1. H0 is true We have to assume that not finding an effect as our default hypothesis The assumptions to be met in Independent t-tests H0 is true Before we can conclude this, we have to assume that: 1. H0 is true 2. Data are normally distributed This is also called as the Normality test of normality Because, if our data is skewed, it means the mean is not the best measure of central tendency! To test for normality, you need to use Shapiro-Wilk test Shapiro-w ilk test (normality test) Also based on null-hypothesis testing 0.5 1 H0: data are normally distributed 0.4 0.8 Probability Density Probability Density (normality not significantly different, p >.05) 0.3 0.6 H1: normality assumption is violated (normality significantly different, p <.05) 0.2 0.4 The default (H0) is that normality is 0.1 0.2 met, so if p is less than 0.05, it means 0 0 -4 -2 0 2 4 -4 -2 0 2 4 data is NOT normally distributed or a dependent variable dependent variable Data are normally distributed Data are not normally distributed deviation from normality. Shapiro-w ilk test (normality test) 𝐀 ഥ ഥ 𝐁 ഥ ഥ 𝐁 𝐀 What if the assumption is not met? 0.5 1 You should then conduct a ‘Mann- 0.4 0.8 Probability Density Probability Density Whitney t-test’ (an alternative to t- 0.3 0.6 test, interpreted the same way). 0.2 0.4 In this alternative t-test, the median (instead of mean) of each groups are 0.1 0.2 compared instead. 0 0 -4 -2 0 2 4 -4 -2 0 2 4 dependent variable dependent variable Data are normally distributed Data are not normally distributed Why normality is important? If your data is not normally 0.955 distributed, the mean (used to calculate both our systematic and 0.95 unsystematic variances) will not be a power 0.945 good representation of our data! As the distribution becomes more 0.94 skewed, the probability of detecting 0.935 an effect when it is there (i.e., power) skewness decreases (Type 2 error) The assumptions to be met in Independent t-tests H0 is true Before we can conclude this, we have to assume that: 1. H0 is true 2. Data are normally distributed 3. Equal variance (homoscedasticity) Normality Data in the two groups must have equal (similar) variance To test for equal variance, you need to use Levene’s test Homoscedasticity Levene’s test (homoscedasticity) Also based on null-hypothesis testing 0.5 2 H0: variance are equal 0.4 1.5 (variance not significantly different, p >.05) Probability Density Probability Density H1: variance are different 0.3 1 (variance significantly different, p <.05) 0.2 𝑆𝐵2 What if the assumption is not met? 0.1 𝑆𝐴2 0.5 You should then conduct a 0 0 ‘Welch’s t-test’ (an alternative to t- -4 -2 0 2 4 -4 -2 0 2 4 dependent variable dependent variable test, interpreted the same way) Variance are equal Variance are not equal Levene’s test (homoscedasticity) ഥ𝐁 𝐀 ഥ t = how many times (ratio) the systematic variance is larger than the unsystematic variance When your data has different variances, the systematic variance may stay the same, but not the unsystematic variance. Therefore, if the variances are 𝑆𝐴2 significantly different, this ‘ratio’ will be inaccurate! 𝑆𝐵2 Why is equal variance important? If variance are unequal, this can lead to: 1. Differences found when indeed there is none (Type 1 error). 2. Differences not found when indeed there is an effect (Type 2 error) Our default is that variances are equal. If p is less than 0.05, it means there is a significant difference between the two variances. This means they are NOT equal. The assumptions to be met in Independent t-tests H0 is true Before we can conclude this, we have to assume that: 1. H0 is true 2. Data are normally distributed 3. Equal variance (homoscedasticity) Independence Normality 4. Independence Data in one group must be independent of data in the other. Homoscedasticity The assumptions to be met in Independent t-tests H0 is true Metric data Before we can conclude this, we have to assume that: 1. H0 is true 2. Data are normally distributed 3. Equal variance (homoscedasticity) Independence Normality 4. Independence 5. Metric data Measures are in interval or ratio scale (properties to allow calculating mean, variance, etc.) Homoscedasticity One-sample t-test However, sometimes we might not have two groups of sample per se, but rather one group against the population mean, 𝛍 (e.g., a study reported the average height of people in UK 20 years ago) 𝛍 One-sample t-test 𝛍 could be a theoretical value, e.g., the average height of people in UK 20 years ago. More often 𝛍 = 0, if we measure the effect of an experimental treatment, we want to know whether it is different from zero (i.e., there is an effect). 𝛍 One-sample t-test Similar logic to independent sample t-test: ത 𝛍 𝑋− 𝑡= 𝑆2 𝑁 Except of comparing the means between two groups, you are comparing the means (or median) of one group to a single fixed value, 𝛍 t=? Today’s objectives 01 Differences between two groups: Independent Sample/Between subject 02 Differences between two variables: Repeated Measures/W ithin subject 03 How to write-up the ‘Results’ section? Differences between two variables: Repeated measures Manipulation Manipulation Also called paired sample t-test Each sample have “a pair of results” from the two variables Differences between two variables: Repeated measures Attention before and after coffee Memory ‘before’ vs. ‘after’ 8 hours of sleep User-friendly testing of application in ‘old’ vs ‘new’ design Rating the best candy, ‘Haribo’ vs ‘Skittles’ To examine such differences, you will need a within-subject design. Differences between two variables: Repeated measures Attention before vs. after coffee Here, we used a within-subject design. Instead of separating your sample into groups, all your No coffee Drink coffee participants went through different conditions/same manipulations. Differences between two variables: Repeated measures Attention before vs. after coffee Let’s imagine we want to see if coffee increases our attention. We recruited 10 participants to conduct a visual search task (e.g., Find WALDO!) Differences between two variables: Repeated measures Attention before vs. after coffee All participants had their baseline reaction time measured (before coffee) while they find Waldo. After a short break, the same 10 participants had to drink coffee before conducting the task again. Before After Differences between two variables: Repeated measures Attention before vs. after coffee We found that the mean reaction Mb time before and after coffee were 365 ms and 331 ms, respectively Ma Before After Differences between two variables: Repeated measures Attention before vs. after coffee If we use the formula of independent t-test, we will find that the p value associated with our t is more than 0.05, hence we have to accept our null hypothesis (H0). 𝑀𝑎 − 𝑀𝑏 33.64 𝒕= = = 𝟎. 𝟕𝟖 𝑆𝑎2 + 𝑆𝑏2 43.37 𝑁 Differences between two variables: Repeated measures Attention before vs. after coffee However, we have to take into Mb account that the manipulation were done so on the same sample! Ma We should compare each individual to themselves instead… 𝒅 = 𝑿𝑨 −𝑿𝑩 = 𝑝1𝐴 − 𝑝1B, 𝑝2𝐴 − 𝑝2B, …, 𝑝𝑁𝐴 − 𝑝𝑁B Before After Differences between two variables: Repeated measures Attention before vs. after coffee We should compute the difference Mb between conditions for each participant, separately (i.e., d) Ma We then test whether the mean of d is different from zero ഥ 𝒅 ഥ 𝒅 𝒕= 𝒏 = 𝟏 σ𝒊 (𝒅𝒊 −𝒅)𝟐 𝑺𝑬𝒅 Before After 𝑵 𝑵−𝟏 Differences between two variables: Repeated measures Attention before vs. after coffee Here we can see that the probability was very unlikely! (e.g., p < 0.05) Independent t-test can’t reject H0 but the paired t-test can, why??? 𝑀𝑎 − 𝑀𝑏 33.64 𝒕= = = 𝟔. 𝟗𝟗 1 σ𝑛 (𝑑 −𝑑) 2 4.81 𝑖 𝑖 𝑁 𝑁−1 Standard deviation vs. standard error 𝑀𝑎 − 𝑀𝑏 33.64 𝑀𝑎 − 𝑀𝑏 33.64 𝑡= = = 6.99 𝑡= = 𝑆𝑎2 + 𝑆𝑏2 𝟒𝟑. 𝟑𝟕 = 0.78 vs 1 σ𝑛𝑖 (𝑑𝑖 −𝑑)2 𝟒. 𝟖𝟏 𝑁 𝑁 𝑁−1 The standard deviation (and standard error) of the differences are much smaller than the standard deviation of the two samples because it does not include individual differences. Before After Standard deviation vs. standard error 𝑀𝑎 − 𝑀𝑏 33.64 𝑀𝑎 − 𝑀𝑏 33.64 𝑡= = = 6.99 𝑡= = 𝑆𝑎2 + 𝑆𝑏2 𝟒𝟑. 𝟑𝟕 = 0.78 vs 1 σ𝑛𝑖 (𝑑𝑖 −𝑑)2 𝟒. 𝟖𝟏 𝑁 𝑁 𝑁−1 Since the same participants experienced all conditions, each participant serves as their own control/comparison. There will be no effects from variations caused by individual differences between conditions. Before After Standard deviation vs. standard error 𝑀𝑎 − 𝑀𝑏 33.64 𝑀𝑎 − 𝑀𝑏 33.64 𝑡= = = 6.99 𝑡= = 𝑆𝑎2 + 𝑆𝑏2 𝟒𝟑. 𝟑𝟕 = 0.78 vs 1 σ𝑛𝑖 (𝑑𝑖 −𝑑)2 𝟒. 𝟖𝟏 𝑁 𝑁 𝑁−1 E.g., one would assume that the black data points is slower than all other colours. But when the differences within each participant is calculated, the value is very similar to the other colours. Before After Standard deviation vs. standard error 𝑀𝑎 − 𝑀𝑏 33.64 𝑀𝑎 − 𝑀𝑏 33.64 𝑡= = = 6.99 1 𝑡= = 𝑆𝑎2 + 𝑆𝑏2 𝟒𝟑. 𝟑𝟕 = 0.78 vs 1 σ𝑛𝑖 (𝑑𝑖 −𝑑)2 𝟒. 𝟖𝟏 𝑁 𝑁 𝑁−1 0.8 0.6 In other words, it doesn’t matter where the points are ‘before’ and ‘after’, rather, Power 0.4 the importance lies in the difference between ‘before’ and ‘after’ 0.2 This implies much more power to show 0 an effect when there is one, i.e., higher 0 0.5 1 1.5 2 Effect Size (d) probability to reject H0, when H1 is true. Recap: Hypothesis testing Confidence interval If 95% CI error bars do not overlap, you can be relatively certain that there are differences between the conditions However, this is different depending on which of the two t-tests you use Recap: Hypothesis testing Confidence interval 16 p-value Ind. Paired 0.214 0.025 The 95% CI is estimated based on the 14 0.283 0.042 standard error of mean (SEM) 0.014 0.001 12 0.103 0.000 0.054 0.001 Most p-value obtained with the 10 0.157 0.018 independent samples t-test is smaller 0.050 0.023 sample # than 0.05 when the 95% CI intervals of 8 0.109 0.001 the two means do not touch. 0.277 0.017 6 0.156 0.050 0.130 0.013 However, the paired t-test can still give a 4 0.055 0.027 significant result, because it is based on 0.227 0.118 the SE of the differences. 2 0.337 0.103 0.228 0.076 0 2 3 4 5 6 7 dependent variable Differences between two variables: Repeated Measures How to examine differences between two variables The “shape” of t is determined by degrees of freedom (df) Number of observations free to vary when estimating the mean in the population df = N – 1 Since you have only one sample in paired t- tests, the df would then be N-1 (i.e., df = (N1 – 1)) The assumptions to be met in Repeated measures t-tests H0 is true Similar to independent t-test, the test also assumes: 1. H0 is true We have to assume there are no differences as our default hypothesis The assumptions to be met in Repeated measures t-tests H0 is true Similar to independent t-test, the test also assumes: 1. H0 is true 2. Independence there is no relationship between Independence the observations, with respect to the dependent variable E.g., the measurements taken on one condition should not be influenced by the measurements on another condition The assumptions to be met in Repeated measures t-tests H0 is true Similar to independent t-test, the test also assumes: 1. H0 is true 2. Independence 3. Data are normally distributed Independence Normality If Shapiro-Wilk test has p < 0.05 and small sample size (N