An Introduction to the T-Test PDF
Document Details
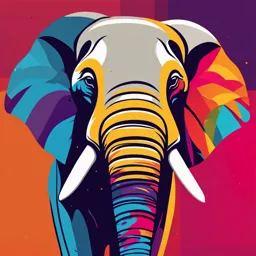
Uploaded by LargeCapacityAntigorite4770
Tata Memorial Centre
Tags
Summary
This document provides an introduction to the t-test, a statistical method for comparing two groups with independent samples, and introduces the Student's t-distribution. The text explains statistical hypotheses, the logic of t-tests, and covers topics from degrees of freedom to the assumptions needed for running an independent samples t-test. Includes keywords like t-test, statistics, hypothesis, and data to help readers understand the material better.
Full Transcript
An Introduction to the T-Test Comparing Two Groups w/ Independent Samples T-test A common objective we have in our research work is to compare groups of people. For example, we might wish to know: if PhD students are more anxious than undergrad students; if men are more likely to binge drink than wo...
An Introduction to the T-Test Comparing Two Groups w/ Independent Samples T-test A common objective we have in our research work is to compare groups of people. For example, we might wish to know: if PhD students are more anxious than undergrad students; if men are more likely to binge drink than women; or, if students in different school districts have different performance on standardized tests. The 𝑡 -test is one such test we can use, when the data calls for it! Let us say we have a random sample that we have split into two groups, 𝐺1 and 𝐺2. We have measured a numeric variable 𝑋 , which we assume is normally distributed. For example, 𝐺1 might represent men and 𝐺2 might represent women and 𝑋 might be systolic blood pressure. We are curious if group membership (𝐺1 𝑣𝑠𝐺2 ) is associated with different values of 𝑋. One way we can frame this as a scientific question is to ask if: “The mean value of 𝑋 is different between groups 𝐺1 and 𝐺2.” Let’s define 𝜇𝐺1 as the population-level mean of 𝑋 for 𝐺1 , and 𝜇𝐺2 as that for 𝐺2. What we are essentially trying to ask is, does 𝜇𝐺1 = 𝜇𝐺2 ? Statistical Hypotheses of the Independent Samples T-Test The null and alternate hypothesis of the t-test are typically presented as: 𝐻0 : 𝜇𝐺1 = 𝜇𝐺2 𝐻𝐴 : 𝜇𝐺1 ≠ 𝜇𝐺2 Importantly, 𝜇𝐺1 = 𝜇𝐺2 is the same as 𝜇𝐺1 − 𝜇𝐺2 = 0. Therefore, we can also consider the null and alternate hypothesis as follows: 𝐻0 : 𝜇𝐺1 − 𝜇𝐺1 = 0 𝐻𝐴 : 𝜇𝐺1 − 𝜇𝐺1 ≠ 0 So, the independent sample t-test will attempt to assess the probability of our observed data assuming that 𝐻0 is true. Logic of the t-test Before we learn how to run a t-test, we need to think through what our null hypothesis means and what it might mean to reject it. When we run a statistical test, we assume that our null hypothesis is true. If we assume that the null is true and that 𝜇𝐺1 − 𝜇𝐺2 = 0 at the population-level then it follows that if we were to sample a set of people from 𝐺1 and 𝐺2 and looked at the difference in their group means of 𝑋 , that 0 is the most likely value. While we use 𝜇𝐺1 and 𝜇𝐺2 to represent the population-level mean, we use 𝑥¯𝐺1 and 𝑥¯𝐺2 to represent the mean values of our sample groups. However, we know that sampling pretty much never results in a perfect representation of the populations, so it is actually fairly likely that the difference in sample group means (𝑥¯𝐺1 − 𝑥¯𝐺2 ) won’t equal 0. This shouldn’t worry us too much, it is fairly likely that if the null is true then it would make sense if 𝑥¯𝐺1 − 𝑥¯𝐺2 was a value pretty close to 0 (whether positive or negative). In fact, if the null hypothesis is true, then we would assume that values of 𝑥¯𝐺1 − 𝑥¯𝐺2 further from 0 would be less likely than values closer to 0 and that negative and positive values are equally likely. Hmm…that actually sounds a lot like the normal distribution doesn’t it? 1. 0 is the most likely value 2. values closer to 0 are more likely than values further from 0 3. positive and negative values appear equally as likely to occur (i.e. symmetry) But… Introducing the Student’s t-Distribution A normal distribution is defined by a mean value 𝜇 and a standard deviation 𝜎. Unfortunately, even if we know we have measured a normally distributed variable 𝑋 , we may not know the population-level standard deviation of 𝑋. This is fairly common in epidemiologic research, because we often don’t have population-level data about the groups we are researching (such as people who inject drugs, or undergraduate students who vape, or people who access syringe exchanges). The 𝑡 -distribution is a variation of the standard normal distribution (𝑍 -distribution), to be used when the standard deviation of the population is unknown. As we discussed before, any normal distribution (𝑁(𝜇, 𝜎) ) can be transformed to the 𝑍 -distribution (𝑁(0, 1) ). Similarly, we may understand the 𝑡 -distribution as a standardized distribution. The 𝑡 -distribution is intended to be a more conservative version of the 𝑍 - distribution, where we assume a wider variability in observations. Essentially, the less information we know, the less certain we are that observations will be near the mean. We define the 𝑡 -distribution as a function of the number of degrees of freedom we have available to measure the variability in our data: Degrees of freedom refer to the number of parameters that are able to “vary freely”, given some assumed outcome. For example, let us say you have have 100 participants and you know that their mean average is 60 years old. Well, there are countless possibilities for how 100 participants age could average out to 60 (e.g., everyone could be 60 years old, half could be 59 and half could be 61, etc etc). In other words, we can see that people’s ages can “vary freely” while still maintaining an average age of 60 years old. But, let us imagine we know the exact age of 99 of the 100 individuals. The average age of the population is 60 years of age. Can the age of the final person “vary freely”? No! There is actually only one value that could take the first 99 values and get the average to 60 years of age. For example, if the average age of the first 99 people is 60 years of age, then the age of the final person must be 60 years of age. If they were older, then the average would move above 60, and vice versa if they were younger. As such to calculate a mean, we must “spend” one degree of freedom. A normal distribution is defined by a mean value 𝜇 and a standard deviation 𝜎. If we have 𝑛 observations and measure sample-mean 𝑥¯ and standard deviation 𝑠 , as discussed above, we must spend one degree of freedom to calculate 𝑥¯. This means that we have 𝑛 − 1 degrees of freedom to calculate 𝑠. The fewer observations we have (i.e., the smaller that 𝑛 is), the less information we have to estimate the variation of our observed variable 𝑋. As such, the 𝑡 -distribution is intended to capture uncertainty in the measurement of the standard deviation from a small sample. The fewer degrees of freedom (i.e., the smaller our sample), the less certain that our measured standard deviation 𝑠 represents our population-level standard deviation 𝜎. To capture this, the 𝑡 - distribution is “shorter” and “wider” than the normal distribution. Essentially, under the 𝑡 -distribution, values farther from 0 are more likely than under the 𝑍 -distribution. As we collect more data (i.e., as 𝑛 gets larger), the 𝑡 -distribution’s shapes approaches that of the 𝑍 -distribution. To picture what this means, let’s plot some distributions. First we are going to plot the 𝑍 -distribution along with 𝑡(1), the 𝑡 -distribution with one degree of freedom: ## Let's create our x-axis, ranging from -5 to 5, with increments of 0.1 x