Basic Research Statistics 2024 PDF
Document Details
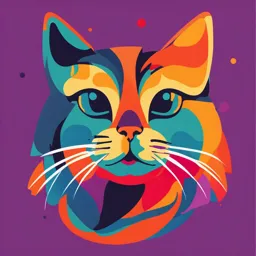
Uploaded by SpectacularHummingbird1195
2024
Ronald A. Gica, MSc
Tags
Summary
This presentation introduces basic research statistics, covering statistical methods, data description, confidence intervals, sampling techniques, comparing populations, correlation and regression, and hypothesis testing. It includes an explanation of different statistical tests, such as t-tests and ANOVA.
Full Transcript
BASIC RESEARCH STATISTICS PAKIGLAMBIGIT: Creating and Safeguarding Sustainable Investigative Project Ronald A. Gica, MSc Statistical Methods Outlin 1 Experimental e 2 Designs 3 Statisti...
BASIC RESEARCH STATISTICS PAKIGLAMBIGIT: Creating and Safeguarding Sustainable Investigative Project Ronald A. Gica, MSc Statistical Methods Outlin 1 Experimental e 2 Designs 3 Statistics Tech to Leverage 4 5 Statistical Methods Back to Basics Art of learning from the data Science that deals with the collection, organization, summarization, presentation, What is analysis, and interpretation of data Statistics? Collection of tools for converting raw data into useful information to help decision makers in their work Set or collection of quantitative data Statistical Methods 1. Methods of Data Presentation 2. Data Description 3. Confidence Intervals 4. Sampling Techniques 5. Comparing Populations 6. Correlation and Regression 7. Testing Associations Methods of Data Presentation Textual Tabular Graphical Gives brief and Large data sets Conveys data to viewers concise description of organized in rows and in pictorial form; useful the data set in columns in getting audience’s paragraph form attention Measures of Central Tendency Data Measures of Variation Descriptio Measures of Position n Boxplot Measures of Central Tendency Mean The sum of the values divided by the total number of values Applicable only to quantitative variables Easily affected by extreme values Median The midpoint of the data array Can be computed for the data in the ordinal, interval, and ratio scale Not amenable for further computations Mode Value(s) which occur most frequently on a given data set Can be determined for both quantitative and qualitative data It is not used in statistical analysis as it is not algebraically defined Measures of Variation Range The highest value minus the lowest value Easily affected by extreme values Interquartile Range of the middle 50% of the Range observation Variance Mean of the squared deviations of the observations from the mean Standard The positive square root of variance; a measure of spread about the Deviation mean Coefficient of Measures the variability of the data set relative to its mean Variation Measures of Position z- Represents the number of standard deviations that a data value falls score above or below the mean Percentile Position in hundredths that a data value holds in the distribution Decile Position in tenths that a data value holds in the distribution Quartile Position in fourths that a data value holds in the distribution Outlier An extremely high or an extremely low data value when compared with the rest of the data values. Boxplots Confidence Interval Point Estimate Interval Estimate A specific numerical value estimate of An interval or a range of values used to a parameter estimate the parameter. This estimate may or may not contain the value of the parameter being estimated Confidence Level Confidence Interval Probability that the interval estimate will Specific interval estimate of a parameter contain the parameter, assuming that a large determined by using data obtained from a number of samples are selected and that the sample and by using the specific estimation process on the same parameter is repeated confidence level of the estimate Probability Sampling 1. Simple Random Sampling 2. Systematic Random Sampling Sampling 3. Stratified Random Sampling 4. Cluster Random Sampling Technique Non-probability Sampling s 5. Convenience Sampling 6. Purposive Sampling 7. Snowball Sampling 8. Quota Sampling A sample is just a part or subset of the population. A sampling technique is a specific process by which the entities of the sample have been selected. Probability sampling is a sampling technique where a researcher sets a selection of a few criteria and chooses members of a population randomly. In non-probability sampling, the researcher chooses samples at random without a fixed or predefined selection process. Simple Random Sampling SRS is the most basic method of selecting a probability sample. Assigns all possible samples an equal chance of being selected Systematic Random Sampling Researchers use the systematic sampling method to choose the sample members of a population at regular intervals. Stratified Random Sampling The population is divided into mutually exclusive groups or strata. The strata can be organized and then draw a sample from each stratum separately. Cluster Random Sampling The population is divided into sections or clusters. Instead of sampling individuals from each subgroup, you randomly select entire subgroups. Convenience Sampling Convenience sampling involves selecting participants based on accessibility and proximity to the researcher. It's handy when time and resources are limited. Purposive Sampling Purposive sampling involves the intentional selection of participants based on the researcher's judgment and the study's objectives. Snowball Sampling Also known as referral or respondent-driven sampling. Snowball sampling is a sampling method that researchers apply when the subjects are difficult to trace. Quota Sampling Quota sampling selects participants based on predetermined quotas or characteristics to ensure representative sampling. It is a non-probabilistic version of stratified sampling. Comparing One Population Comparing Comparing Two Populations Populations Comparing Three or More Populations Type I Error Rejecting a TRUE null hypothesis. The probability of committing a Type 1 error is alpha, often called as the level of significance. Type II Error Accepting a FALSE null hypothesis. The probability of Type II error is symbolized by beta. Hypothesis Testing Procedure 1. State the null (Ho) and alternative (Ha) hypothesis Hypothesis Testing Procedure 1. State the null (Ho) and alternative (Ha) hypothesis 2. Choose a level of significance and formulate the decision rule for rejecting or not rejecting Ho. a.Critical-value approach b. p-value approach Hypothesis Testing Procedure 1. State the null (Ho) and alternative (Ha) hypothesis 2. Choose a level of significance and formulate the decision rule for rejecting or not rejecting Ho. a.Critical-value approach b. p-value approach 3. Compute the value of the test statistic. Hypothesis Testing Procedure 1. State the null (Ho) and alternative (Ha) hypothesis 2. Choose a level of significance and formulate the decision rule for rejecting or not rejecting Ho. a.Critical-value approach b. p-value approach 3. Compute the value of the test statistic. 4. Make a decision. 5. Make a conclusion Student’s One-Sample t-Test Comparing single population A one-sample test compares the mean from one sample to a hypothesized value that is pre-specified in your null hypothesis. Assumptions: 1. The variable is continuous (interval or ratio level). 2. The observations are independent from other observations. 3. There should be no significant outliers. 4. The distribution should be approximately normally distributed (Shapiro-Wilk test). Student’s One-Sample t-Test Comparing single population A one-sample test compares the mean from one sample to a hypothesized value that is pre-specified in your null hypothesis. Wilcoxon Signed-Rank Test What do we do if the normality assumption fails? The Wilcoxon Signed rank test can compare the median to a hypothesized median value. Comparing Two Populations How likely is it that our two sample means were drawn from populations with the same average? Dependent Observations Independent Observations Related: when datasets are obtained from This involve the selection of a sample from the same set of individuals but at different one population that will not affect the times selection of another sample from the second Paired: When samples are obtained population by pairing similar individuals Parametric Test: Parametric Test: Independent of Two-sample t-Test Paired t-Test Welch’s t-Test Non-parametric Test: Non-parametric Wilcoxon Signed Rank Test Test: Mann Whitney U Test Student’s Dependent-Samples t-Test Assumptions: 1. Samples are dependent. 2. The variable is continuous (interval or ratio level). 3. The pairs of observations are independent from other pairs. 4. There should be no significant outliers in the differences between the two related groups. 5. The distribution of the differences in the dependent variable between the two related groups should be approximately normally distributed. Wilcoxon Signed Rank Test Wilcoxon signed-rank test is used to compare two related samples or to conduct a paired difference test of repeated measurements on a single sample to assess whether their population mean ranks differ. Assumptions: 1. Samples are dependent. 2. Variables are measured at least ordinal scale. 3. The pairs of observations are independent from other pairs. Student’s Independent-Samples t-Test Assumptions: 1. The measurement of one sample is independent or unrelated to the other sample. 2. The variable is continuous (interval or ratio level). 3. The observations are independent from other observations within groups. 4. Both groups should have no significant outliers. 5. The population distributions are approximately normal. 6. The population variances must be approximately equal. If variances are not equal, use Welch’s t-test. Mann Whitney U Test The Mann-Whitney U Test, also known as the Wilcoxon Rank Sum Test, is a non- parametric statistical test used to compare two samples or groups. Assumptions: 1. The measurement of one sample is independent or unrelated to the other sample. 2. Variables are measured at least ordinal scale. 3. The observations are independent from other observations within groups. Comparing Three or More Populations The Analysis of Variance of ANOVA ANOVA uses the F-test to compare three of more population means. The F-test compares the ratio of the variances between multiple samples. Assumptions: 1. The variable is continuous (interval or ratio level). 2. The groups of observations should be independent of each other. 3. The population distributions are approximately normal. 4. The population variances must be approximately equal. Post-hoc Analysis If the ANOVA is significant, the Tukey test can be used to make pairwise comparisons. Ronald Fisher (1890-1962) Independent or Dependent T-test? 1) A researcher wishes to see if the average weights of newborn male infants are different from the average weights of newborn female infants. INDEPENDENT T-TEST 2) The LGU is initiating a livelihood program to help its residents raise their household income. The household income before and after the intervention of the livelihood program will be compared. DEPENDENT T-TEST Independent or Dependent T-test? 3) A medical specialist wants to see whether a new counseling program will help subjects lose their weight. Therefore, the preweights of the subjects will be compared with their postweights. DEPENDENT T-TEST 4) A tax collector wishes to see if the mean values of the tax-exempt properties are different for two cities. INDEPENDENT T-TEST Correlation and Regression Correlation Analysis Correlation analysis is used to measure strength of the relationship between two variables. It answers the following questions: 1. Are two or more variables related? 2. If so, what is the strength of the relationship? 3. What type of relationship exists? Parametric Correlation Coefficient Pearson’s r Nonparametric Correlation Coefficient Spearman’s r Regression Analysis Regression is a generic term for all methods attempting to fit a model to observed data in order to quantify the relationship between two groups of variables. The fitted model may then be used either to merely describe the relationship between the two groups of variables, or to predict new values. It answers the questions: 1. Which factors matter most? 2. Which factors can we ignore? 3. How do those factors interact with each other? 4. How certain are we about all of these factors? Regression Analysis Components of Regression Analysis Fundamental Principles in Model Building 1. Specification: the model building activity; The principle of parsimony: other things model specification the same, simple models generally are 2. Estimation: fitting the model to the data preferable to complex models, especially 3. Verification: testing the model in forecasting 4. Prediction: producing forecasts and The shrinkage principle: imposing conducting forecast evaluation restrictions either on estimated parameters or on forecasts often Data types for statistical analysis improves model performance Cross-section data The KISS principle: “Keep it Sophistically Time-series data Simple” Panel data Regression Analysis Assumptions of Linear Regression 1. Zero mean of the error term 2. Homoscedasticity 3. No serial correlation 4. Non-stochastic explanatory variable 5. Positive degrees of freedom 6. No perfect multicollinearity: no exact relationships between any regressors 7. Normality of the error term ANOVA or Regression? 1) An environmentalist wants to determine the relationship between the number of forest fires over the year and the number of acres burned. REGRESSION 2) In department of animal production is interested in discovering the effect of three enzymes, A,B, C for increasing daily milk of a specified type of cow. ANOVA ANOVA or Regression? 3) A researcher wants to see if there is a relationship between the annual energy consumption for both natural gas and coal. REGRESSION 4) In department of animal production is interested in discovering the effect of three enzymes, A,B, C for increasing daily milk of a specified type of cow. ANOVA Testing Associations Chi-Square Test of Independence The Chi-Square Test of Independence The test of independence of variables is used to determine whether two nominal (categorical) variables are independent of or related to each other when a single sample is selected. Assumptions 1. Your two variables should be measured at an ordinal or nominal level. 2. Your two variable should consist of two or more categorical, independent groups. 3. There are k mutually exclusive classes 4. Each observation will fall in one class 5. All expected frequencies for the classes must be at least one 6. Not more than 20% of all classes have expected frequencies below five (if more than 20% have expected frequencies below five, combine classes). Experiment al Designs Types of Scientific Study Experimental Study Observational Study A scientific test conducted with the The application of conditions that affect objective of studying the relationship the outcome is not directly controlled by between one or more outcome/response the scientist, however, all the key variables and one or more elements for experimental studies should condition/explanatory variables that are still be considered when you plan an intentionally manipulated to observe how observational study. changing these conditions affects the Often used in ecology studies. results. To understand cause and effect, experiments are the only source of fully convincing data. Design of experiments is a process that brings together all the key elements of experimental study to produce an experiment that efficiently answers the questions of interest and aims to obtain the maximum amount of information for the resources available, or to minimize the resources needed to obtain the information desired. Principles for Designing Experiments Replication The process of applying each treatment to more than one experimental unit, so the number of replicates of a treatment is the number of independent experimental units to which each treatment is applied Randomization Used to ensure the fair assessment of treatments without bias An insurance against potential unknown differences between units Blocking The process of identifying or constructing groups of experimental units expected to have similar responses in the absence of any treatment effects Common Experimental Designs The Completely Randomized Design (CRD) The simplest form of design and is appropriate if the experimental units are unstructured and homogeneous Each treatment is equally likely to be allocated to each unit The Randomized Complete Block Design (RCBD) The simplest design that includes blocking The number of experimental units in each block must be the same as the number of treatments The Latin Square (LS) Design Useful where patterns of heterogeneity are associated with two crossed structural factors with the same number of levels Common Experimental Designs The Split-Plot (SP) Design Used when at least two treatment factors are present Each whole plot is divided into a number of subplots, and the levels of factor B are randomized onto subplots within each whole plot The Balanced Incomplete Block Design (BIBD) Useful when there is only one blocking factor but the number of units per block is smaller than the number of treatments Each block can contain only a subset of the treatments The Factorial Design Most efficient design for two or more factors In each complete trial or replicate, all possible combinations of the levels of the factors are investigated Statistical Method for Analyzing Experiments Parametric The Analysis of Variance (ANOVA) Multivariate Analysis of Variance (MANOVA) Non-Parametric Kruskall Wallis Test Statistic al Tech to Leverage Statistical Software Python and SQL Revered as the foundation of contemporary data analysis, these programming languages are the cherished choice for data scientists and data engineers owing to their versatility, rich libraries, and superior efficiency in data extraction and transformation. Machine Learning Algorithms These algorithms, by imbibing a hint of human-like thinking into machines, enable them to learn from expansive data sets. By integrating artificial intelligence into data analytics, they bolster the accuracy of predictive analytics. Big Data Analytics Operating on a grand scale, big data analytics processes vast swathes of information, laying a solid foundation for deep insights while fostering synergies between data scientists and intelligence professionals. Open-Source Tools With the likes of Hadoop and NoSQL, data mining on a grand scale becomes a feasible endeavor. Data Visualization Tools This is where the essence of raw data is transmuted into visual artistry. Tools like Tableau and Power BI transform dense data into intuitive dashboards and engaging graphs, morphing intricate data science into visuals that enrich decision-making and spotlight trends with lucidity. Thank you!