Unit 5: Inference PDF
Document Details
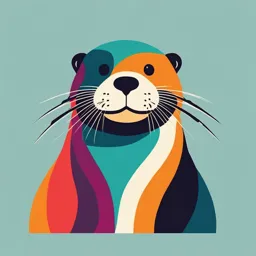
Uploaded by AwesomeCarnelian4810
Copenhagen Business School, Vienna University of Economics and Business
Martin / Zulehner
Tags
Summary
This document is about inference in econometrics. It details the classical linear model (CLM), including assumptions, and different hypothesis testing techniques, with examples and an appendix.
Full Transcript
Unit 5: Inference Martin / Zulehner: Introductory Econometrics 1 / 52 Outline 1 Motivation 2 Classical Linear Model Assumptions 3 Inference t - Test F - test 4 Exercise: Marginal eects and F-test 5 Appendix A: Restricted Model and Testing mo...
Unit 5: Inference Martin / Zulehner: Introductory Econometrics 1 / 52 Outline 1 Motivation 2 Classical Linear Model Assumptions 3 Inference t - Test F - test 4 Exercise: Marginal eects and F-test 5 Appendix A: Restricted Model and Testing more formally Restricted OLS Estimation The F-test within restricted regression Martin / Zulehner: Introductory Econometrics 2 / 52 Motivation Estimation is subject to noise In our notation: We will never know the true β; our β̂ is only a noisy estimates thereof β̂ depends on the random sample we obtained. For a dierent random sample, we would obtain a dierent estimate β̂ How precise does our estimate need to be? Martin / Zulehner: Introductory Econometrics 3 / 52 Example Suppose the true model is: wage =β educ +u and the true β = 0.1 When the sampling process is very noisy (e.g., educ ∼ N(0, 1), but u ∼ N(0, 10)), then we will also get very noisy estimates Martin / Zulehner: Introductory Econometrics 4 / 52 Example: Sample 1 n = 100: Data looks quite noisy, but seems like a negative relationship? Martin / Zulehner: Introductory Econometrics 5 / 52 Example: Sample 2 Still n = 100, same data generation process, but sample obtained on another day, and now seems like a positive relationship? Martin / Zulehner: Introductory Econometrics 6 / 52 Example: Four possible samples Martin / Zulehner: Introductory Econometrics 7 / 52 Hypothesis testing We need a way to quantify the uncertainty in our estimates... and a threshold of how much uncertainty we are willing to accept, before we accept (or reject) a certain hypothesis E.g., given what we saw, do we (still?) believe in the hypothesis that β = 0? ⇒ what can we rightfully infer from the data ⇒ inference Martin / Zulehner: Introductory Econometrics 8 / 52 1. The Classical Linear Model (CLM) To do classical hypothesis testing, we need an addition assumption (beyond the Gauss-Markov assumptions MLR.1 - MLR.5) on the distribution of the error term: Assumption MLR.6 - Normality The population error u is independent of the explanatory variables x1 , x2 ,... , xk and is normally distributed with zero mean and variance σ2 I u ∼ Normal(0, σ 2 I) (1) MLR.6 allows us to obtain the exact sampling distributions of some statistics of interest such as the t statistics and F statistics This helps us to carry out exact hypotheses tests (exact inference) We can summarize the population assumptions of CLM as follows: y |X ∼ N(β, σ 2 I) This assumption can be dropped if we have reasonably large sample sizes Martin / Zulehner: Introductory Econometrics 9 / 52 The homoskedastic normal distribution with a single explanatory variable Martin / Zulehner: Introductory Econometrics 10 / 52 Normal Sampling Distributions Under the CML assumptions MRL.1 trough MRL.6, conditional on the sample values of the independent variables : β̂ ∼ N(β, Var (β̂)) (2) where Var (β̂) is dened as σ 2 (X′ X)−1 Therefore the distribution of the normalized estimators βj is: β̂j − βj ∼ N(0, 1) sd β̂j Martin / Zulehner: Introductory Econometrics 11 / 52 Normal Sampling Distributions For the normally distributed (classical) linear model (CML), the distribution of the the variance estimator σ̂ 2 is proportional to a chi-squared distribution: ûi2 PN ′ ^ u^ u σ̂ 2 = i=1 = ∼ σ 2 χ2(N−K −1) (3) N −K −1 N −K −1 where N is the number of observations and K +1 is the number of estimated parameters. N −K −1 are the degrees of freedom In a normally distributed (classical) linear model (CLM), the variance of σ̂ 2 is given by: 2σ 4 Var (σ̂ 2 ) = N−K −1 Martin / Zulehner: Introductory Econometrics 12 / 52 2. Inference: the t - Test Under the CLM assumptions β̂j − βj tβj = ∼ tN−K −1 (4) se β̂j Note that we have to estimate σ2 by σ̂ 2 (i.e. sd(β̂) by se(β̂)) 2 Since β̂ is normally distributed and σ̂ is chi-squared distributed the t-statistic follows the t-student distribution The degrees of freedom are N −K −1 since we estimate K +1 parameters Knowing the sampling distribution for the standardized estimator allows us to carry out hypotheses tests Martin / Zulehner: Introductory Econometrics 13 / 52 Testing parameters: a typical example Consider the null hypothesis H0 : βj = 0 If we cannot reject the null, then we can state that xj has no statistically signicant eect on y , controlling for the other x 's To perform the test we rst need to dene the t statistic for β̂j : β̂j − 0 β̂j tβ̂j = = se β̂j se β̂j We will then use our t statistic together with a rejection rule to determine whether to accept the null hypothesis H0 Martin / Zulehner: Introductory Econometrics 14 / 52 One-sided alternatives Besides our null hypothesis, H0 , we need to specify an alternative hypothesis, H1 , and a signicance level α to conduct our statistical testing H1 may be one-sided, or two-sided 1 H1 : βj > 0 and H1 : βj < 0 are one-sided 2 H1 : βj ̸= 0 is a two-sided alternative If we want to have only a 5% probability of rejecting H0 in case H0 is indeed true, then we say that our signicance level is 5% Having chosen a signicance level, α (e.g. 5%), we look for the (1 − α)th percentile for a t -distribution with N −K −1 df and call this c, the critical value We can reject the null hypothesis if the t -statistic is greater than the critical value If the calculated t -statistic is smaller than the critical value c then we fail to reject the null hypothesis: If t≥c then reject the null hypothesis Martin / Zulehner: Introductory Econometrics 15 / 52 One-sided alternatives yi = β0 + β1 xi 1 +... + βk xik + ui Hence, if the value of our t -statistic is large enough, we will say that the coecient βj is signicantly greater than zero Martin / Zulehner: Introductory Econometrics 16 / 52 One-sided vs. Two-sided Since the t distribution is symmetric, testing H1 : β j < 0 is straightforward. The critical value is just the negative value of c Hence, we can reject the null if the t -statistic is < −c , and we fail to reject the null if the t -statistic is > −c For a two-sided test, we set the critical values based on α/2 and accept H1 : βj ̸= 0 if the absolute value of the t -statistic is > c, i.e. if the t-statistic is in the interval (-c,c) Generally, the alternative hypothesis is assumed to be two-sided If we reject the null hypothesis, we typically say xj is statistically signicant (dierent from zero) at the α% level If we fail to reject the null hypothesis, we typically say xj is statistically insignicant at the α% level Martin / Zulehner: Introductory Econometrics 17 / 52 Two-sided alternatives yi = β0 + β1 xi 1 +... + βk xik + ui Hence, if the value of our t -statistic is in the interval −c, c , we will say that the coecient βj is not signicantly dierent from zero Martin / Zulehner: Introductory Econometrics 18 / 52 Testing other hypotheses In more general terms, we may want to test the whether βj is dierent than other values, e.g. H0 : βj = aj In this case, the appropriate t -statistic is : β̂j − aj t= , se β̂j in our standard test, aj = 0 Martin / Zulehner: Introductory Econometrics 19 / 52 Initial example continued: large sample size Martin / Zulehner: Introductory Econometrics 20 / 52 Condence Intervals Another way to conduct classical statistical testing is to construct a condence interval using the same critical values that we used for a two-sided test A (1 − α)% condence interval is dened as: β̂j ± tα/2 · se β̂j where c is the (1 − α/2) percentile in a tN−K −1 distribution Martin / Zulehner: Introductory Econometrics 21 / 52 Computing p-values for t tests Alternatively (but equivalently) to the classical approach is to ask, what is the smallest signicance level at which the null hypothesis would be rejected? To do that, we compute the t -statistic and then look to what percentile this value corresponds in the appropriate t distribution - this is called the p -value The p -value is the probability that we would observe the realized t -statistic, if the null hypothesis was true, i.e. p -value= Pr (|T | > t) where T denotes a t -distributed random variable with N − K − 1 degrees of freedom and t denote the numerical value of the test statistic Most computer packages will compute the p -value for you, assuming a two-sided test If you want a one-sided alternative, just divide the two-sided p -value by 2 Stata and R automatically provide the t -statistic, the p -value, and the 95% condence interval for H0 : β j = 0 Martin / Zulehner: Introductory Econometrics 22 / 52 OLS estimates by Stata Martin / Zulehner: Introductory Econometrics 23 / 52 Testing Linear Combinations - I Suppose instead of testing whether β1 is equal to a constant, you want to test if it is equal to another parameter, that is H0 : β̂1 = β̂2 We use the same basic procedure for forming the t statistic: β̂ − β̂2 t= 1 (5) se β̂1 − β̂2 Since: r se β̂1 − β̂2 = Var β̂1 − β̂2 with: Var β̂1 − β̂2 = Var β̂1 + Var β̂2 − 2Cov (β̂1 , β̂2 ) Martin / Zulehner: Introductory Econometrics 24 / 52 Testing Linear Combinations - II It follows that: i2 i2 1 2 h h se β̂1 − β̂2 = se β̂1 + se β̂2 − 2s12 where S12 is an estimator of Cov (β̂1 , β̂2 ). In order to use equation (2), s12 is needed, which the standard output of Stata does not have Many packages will have an option to get it, or will just perform the test for you In Stata, after regy x1 x2... xk you would type test x1 = x2 to get a p-value for the test More generally, you can always restate the problem to get the test you want Martin / Zulehner: Introductory Econometrics 25 / 52 Example Suppose you are interested in the eect of campaign expenditures on outcomes The model is: voteA = β0 + β1 log (expendA) + β2 log (expendB) + β3 prtystrA + u (6) Suppose you want to test whether the overall campaign contributions have an impact on the voting outcome: H0 : β1 = −β2 , or H0 : θ1 = β1 + β2 = 0 Compute β1 = θ1 − β2 , substitute it into the model and rearrange to get: voteA = β0 + θ1 log (expendA) + β2 log (expendB − expendA) + β3 prtystrA + u This is the essentially the same model as (6), but now we get a standard error for β1 − β2 = θ1 directly from the basic regression Any linear combination of parameters could be tested in a similar way Other examples of hypotheses about a single linear combination of parameters: β1 = 1 + β2 ; β1 = 5β2 ; β1 = −1/2β2 ; etc. Martin / Zulehner: Introductory Econometrics 26 / 52 Multiple Linear Restrictions Everything we have done so far has involved testing a single linear restriction, (e.g. β1 = 0 or β1 = β2 ) However, we may want to jointly test multiple hypotheses about our parameters A typical example is testing exclusion restrictions - e.g. we might want to know whether a group of parameters are all equal to zero Now the null hypothesis might be something like H0 : βk−q+1 = 0,... , βk = 0 H1 : H0 is not true We can not just check each t statistic separately, because we want to know if the q parameters are jointly signicant at a given level This means that it is enough that one of them is signicant to reject the null hypothesis Martin / Zulehner: Introductory Econometrics 27 / 52 Multiple Linear Restrictions To do the test, we need to estimate the restricted model (r) without xk−q+1 ,... , xk , as well as the unrestricted model (ur) with all x 's included. Intuitively, we want to know if the change in SSR is big enough to warrant inclusion of xk−q+1 ,... , xk This is measured by the F -statistic (SSRr − SSRur ) /q F = , SSRur / (N − K − 1) where r is restricted and ur unrestricted Martin / Zulehner: Introductory Econometrics 28 / 52 The F statistic - I The F statistic is always positive, since the SSR from the restricted model can not be less than the SSR from the unrestricted Essentially the F statistic is measuring the relative increase in SSR when moving from the unrestricted to the restricted model q= number of restrictions or dfr − dfur N − K − 1 = dfur To decide if the increase in SSR when we choose the restricted model is big enough to reject the exclusions, we need to know something about the sampling distribution of our F statistic Not surprisingly, we can show that this statistic follows a F ∼ Fq,N−K −1 distribution, where q is referred to as the numerator degrees of freedom and N −K −1 as the denominator degrees of freedom Martin / Zulehner: Introductory Econometrics 29 / 52 The F statistic - II ⇒ Reject H0 at a signicance level α if F >c Martin / Zulehner: Introductory Econometrics 30 / 52 The R 2 form of the F statistic Because the SSR's may be large, an alternative form of the formula might be computationally useful We use the fact that SSR = SST (1 − R 2 ) for any regression, so we can substitute in for SSRu and SSRur and obtain: 2 − Rr2 /q Rur F = 2 ) / (N − K − 1) , (1 − Rur Martin / Zulehner: Introductory Econometrics 31 / 52 Overall signicance A special case of exclusion restrictions is to test whether all coecient of our model are jointly signicant he null hypothesis is therefore: H0 : β1 = β2 =... = βk = 0 2 Since the R from a model with only an intercept will be zero, the F statistic is simply: R 2 /K F = (1 − R 2 ) / (N − K − 1) Martin / Zulehner: Introductory Econometrics 32 / 52 General Linear Restrictions The basic form of the F statistic will work for any set of linear restrictions First estimate the unrestricted model and then estimate the restricted model In each case, make note of the SSR Imposing the restrictions can be tricky - we will likely have to redene variables again Just as with t statistics, the p-values can be calculated by looking up the percentile in the appropriate F distribution If only one exclusion is being tested, then F = t2, and the p-values will be the same we can summarize t-test and F-tests within the single, more general, framework of restricted regressions (see Appendix A) Martin / Zulehner: Introductory Econometrics 33 / 52 Example Use same voting model as before voteA = β0 + β1 log (expendA) + β2 log (expendB) + β3 prtystrA + u Now the null hypothesis is H 0 : β 1 = 1, β 3 = 0 Substituting in the restrictions: voteA = β0 + log (expendA) + β2 log (expendB) + u , so: The restricted model is hence: voteA − log (expendA) = β0 + β2 log (expendB) + u Martin / Zulehner: Introductory Econometrics 34 / 52 Interpretation of t-test and F-test. reg birthweight educ age smoker alcohol unmarried, robust Linear regression Number of obs = 3,000 F(5, 2994) = 33.34 Prob > F = 0.0000 R-squared = 0.0575 Root MSE = 575.37 Robust birthweight Coef. Std. Err. t P>|t| [95% Conf. Interval] educ 7.308416 5.587443 1.31 0.191 -3.647199 18.26403 age -2.292542 2.487134 -0.92 0.357 -7.169207 2.584122 smoker -185.2025 28.17357 -6.57 0.000 -240.444 -129.961 alcohol -39.49944 76.91142 -0.51 0.608 -190.304 111.3051 unmarried -244.3864 31.79623 -7.69 0.000 -306.731 -182.0417 _cons 3442.335 85.86685 40.09 0.000 3273.971 3610.699. test smoker alcohol ( 1) smoker = 0 ( 2) alcohol = 0 F( 2, 2994) = 22.28 Prob > F = 0.0000 Exercise: write code in R Martin / Zulehner: Introductory Econometrics 35 / 52 Chow test for structural breaks A Chow test is a F-test and tests for structural breaks in the coecients. For example, in a macro model we can test for the inuence of the oil price shock at the beginning of the 1970ies. Or, with cross section data we can test whether the coecient for the return to education in a wage regression is equal across two groups of individuals. The restricted model: yi = β0 + β1 x1i + β2 x2i + ui , i = 1,... , n The unrestricted model: yi = β10 + β11 x1i + β12 x2i + u1i , i = 1,... , N1 yi = β20 + β21 x1i + β22 x2i + u2i , i = 1,... , N2 Hypotheses: H0 : β11 = β21 ∧ β12 = β22 HA : β11 = β21 ∨ β12 = β22 Martin / Zulehner: Introductory Econometrics 36 / 52 Chow test for structural breaks Step 1: Regression with structural break ▶ Regression with sample 1, N1 number of observations and K +1=3 parameters, gives residual sum of squared SSR(1) ▶ Regression with sample 2, N2 number of observations and K +1=3 parameters, gives residual sum of squared SSR(2) ▶ USSR = SSR(1) + SSR(2) Step 2: Regression without structural break ▶ Regression with whole sample i = 1,... , (N1 + N2 ) and K +1 = 3 parameters, gives residual sum of squared RSSR Step 3: Construction of a F-test (RSSR−USSR)/K Chow-test: FK ,N1 +N2 −2(K +1) = USSR/(N1 +N2 −2(K +1)) with RSSR = SSR(1) + SSR(2) and USSR equal to the sum of squared residuals of the restricted and the unrestricted model, K the number of restrictions, N1 + N 2 the number of observations and 2(K + 1) the number of regressors. Martin / Zulehner: Introductory Econometrics 37 / 52 Exercise: Marginal eects and F-test A researcher studies the determinant of wage levels of male and female employees. To this aim, they consider observations on 526 workers, 252 of whom are females and 274 of whom are males. The data set consists of the following variables: ▶ wage : hourly wage rate, measured in euro per hour; ▶ years : number of years of education; ▶ age : age, in years; ▶ tenure : rm tenure of the worker, in years; ▶ female : equal to 1 if worker is female and equal to 0 if employee is male The following model, which satises the classic assumptions, is proposed 2 ln wagei =β0 + β1 yearsi + β2 agei + β3 agei + β4 tenurei + β5 (yearsi · tenurei ) 2 + β6 femalei + β7 (femalei · yearsi ) + β8 (agei · femalei ) + β9 (agei · femalei ) (7) + β10 (tenurei · femalei ) + β11 (yearsi · tenurei · femalei ) + ui. Martin / Zulehner: Introductory Econometrics 38 / 52 Exercise: Marginal eects and F-test continued The estimates of the above model read as follows (standard errors in parentheses) 2 wage i = − 0.5667 + 0.05937yearsi + ln\ 0.0798 agei − 0.00093agei − 0.01057tenurei (0.2385) (0.0114) (0.01216) (0.00015) (0.01128) + 0.00227(yearsi · tenurei ) + 0.03593femalei + 0.01684(femalei · yearsi ) (0.2385) (0.3373) (0.01684) 2 (8) − 0.03847(agei · femalei ) + 0.000422(agei · femalei ) + 0.01850(tenurei · femalei ) (0.01715) (0.000219) (0.02652) − 0.002107(yearsi · tenurei · femalei ). (0.002187) ▶ In addition, SSR = 80.57 and SST = 148.33. Questions: a Given the results in (8), what are the estimates of the coecients of the regressors years, age and tenure when only the 274 observations corresponding to male workers are used? What happens when only the 252 observations corresponding to female employees are used? b Based on equation (7), what is the (theoretical) marginal eect of age over ln(wage) for male workers in terms of the unknown parameters? What about female workers? c Is the marginal eect of age on ln(wage) for male employees equal to the marginal eect of age on ln(wage) for female workers? Test this hypothesis knowing that SSRR = 81.7242 and with a signicance level of 5%. d Assume that the regression equations are identical for male and female employees. Write explicitly the related null hypothesis. The restricted OLS estimation provides SSR = 93.1805. Test this hypothesis using a signicance level of 5%. Martin / Zulehner: Introductory Econometrics 39 / 52 Solution to exercise: Marginal eects and F-test a Given the results in (8), what are the estimates of the coecients of the regressors years, age and tenure when only the 274 observations corresponding to male workers are used? What happens when only the 252 observations corresponding to female employees are used? For males (femalei = 0) we have 2 wage i = − 0.5667 + 0.05937yearsi + ln\ 0.0798 agei − 0.00093agei − 0.01057tenurei (0.2385) (0.0114) (0.01216) (0.00015) (0.01128) + 0.00227(yearsi · tenurei ) (0.2385) so the coecients are β̂1 = 0.05937, β̂2 = 0.0798 and β̂4 = −0.01057. For females, (femalei = 1) we have ln\ wage i = − 0.5667 + 0.03593 + 0.05937 + 0.01684 yearsi + 0.0798 − 0.03847 agei (0.2385) (0.3373) (0.0114) (0.01684) (0.01216) (0.01715) 2 + −0.00093 + 0.000422 agei + −0.01057 + 0.01850 tenurei (0.00015) (0.000219) (0.01128) (0.02652) + 0.00227 − 0.002107 (yearsi · tenurei ). (0.2385) (0.002187) so the coecients are, respectively, β̂1 + β̂7 = 0.05937 + 0.01684 = 0.07621, β̂2 + β̂8 = 0.0798 − 0.03847 = 0.04133, β̂4 + β̂10 = −0.0157 + 0.01850 = 0.00793. Martin / Zulehner: Introductory Econometrics 40 / 52 Solution to exercise: Marginal eects and F-test b Based on equation (7), what is the (theoretical) marginal eect of age over ln(wage) for male workers in terms of the unknown parameters? What about female workers? Solution: ∂E[ln wage|years, ˆ age, tenure, male] = β2 + 2β3 age, ∂age ∂E[ln wage|years, ˆ age, tenure, female] = β2 + β8 + 2(β3 + β9 )age. ∂age For example, for male workers the eect has to be interpreted as 100(β1 + 2β3 age)% being the expected percentage change in wage when age changes in one unit, everything else xed. Martin / Zulehner: Introductory Econometrics 41 / 52 Solution to exercise: Marginal eects and F-test c Is the marginal eect of age on ln(wage) for male employees equal to the marginal eect of age on ln(wage) for female workers? Test this hypothesis knowing that SSRR = 81.7242 and with a signicance level of 5%. Solution: The null hypothesis is H0 : β8 = β9 = 0 and the restricted regression reads as follows 2 ln wagei =β0 + β1 yearsi + β2 agei + β3 agei + β4 tenurei + β5 (yearsi · tenurei ) + β6 femalei + β7 (femalei · yearsi ) + β10 (tenurei · femalei ) + β11 (yearsi · tenurei · femalei ) + εi. Then, in order to test this restriction, we use an F-test with m = 2 restrictions (SSRR − SSRU )/m (81.7242 − 80.57)/2 F = = = 3.6816. SSRU /(n − k − 1) 80.57/(526 − 11 − 1) The critical value under the null is F2,514 = 3.01326, so we reject the null hypothesis in favor of the conclusion that the marginal eect is dierent for males and females. Martin / Zulehner: Introductory Econometrics 42 / 52 Solution to exercise: Marginal eects and F-test d Assume that the regression equations are identical for male and female employees. Write explicitly the related null hypothesis. The restricted OLS estimation provides SSR = 93.1805. Test this hypothesis using a signicance level of 5%. Solution: The null hypothesis is H0 : β6 = β7 = β8 = β9 = β10 = β11 = 0 and the restricted regression reads as follows 2 ln wagei =β0 + β1 yearsi + β2 agei + β3 agei + β4 tenurei + β5 (yearsi · tenurei ) + εi. Then, in order to test this restriction, we use an F-test with m = 6 restrictions (SSRR − SSRU )/m (93.1805 − 80.57)/6 F = = = 13.408. SSRU /(n − k − 1) 80.57/(526 − 11 − 1) The critical value under the null is F6,514 = 2.116206, so we reject the null hypothesis that the regression functions are equal for males and females, so we conclude that they dier in at least one coecient. Martin / Zulehner: Introductory Econometrics 43 / 52 Appendix A: Restricted OLS Estimation We can summarize t-test and F-tests within the single, more general, framework of restricted regressions Let β̃ be an arbitrary estimator of β satisfying: Rβ̃ =r where R is a known (q × K + 1) and r a known (q × 1) vector of coecients dening the linear restrictions The restricted OLS estimator is obtained by ˆ′ ũ β̂r = arg minβ̃ ũ ˆ, s.t.Rβ̃ = r The restricted OLS estimator is then: β̂ = β̂ur + (X′ X)−1 R′ [R(X′ X)−1 R′ ]−1 (r − Rβ̂ur ) Martin / Zulehner: Introductory Econometrics 44 / 52 Moments of the OLS estimator The mean of the restricted OLS estimator is E [β̂r ] = β + (X′ X)−1 R′ [R(X′ X)−1 R′ ]−1 (r − Rβ̂) = β, if Rβ̂ =r The variance of the restricted OLS estimator is: Var [β̂r ] = Var [β̂ur + (X′ X)−1 R′ [R(X′ X)−1 R′ ]−1 (r − Rβ̂)] = Var [(I − B)β̂ur + C] where B = (X′ X)−1 R′ [R(X′ X)−1 R′ ]−1 R C = (X′ X)−1 R′ [R(X′ X)−1 R′ ]−1 r Martin / Zulehner: Introductory Econometrics 45 / 52 Moreover: Var [β̂ur ] − Var [β̂r ] = σ 2 (X′ X)−1 R′ [R(X′ X)−1 R′ ]−1 R(X′ X)−1 which is a positive semi-denite matrix ⇒ β̂r is more ecient than β̂ur ! Dene ûr = y − Xβ̂r For SSRr = û′r ûr and SSRru = û′ur ûur the following properties hold: ′ ′ i SSRr = SSRur + (β̂r − β̂ur ) X X(β̂r − β̂ur ) where β̂ur = β̂ ii SSRr ≥ SSRur iii SSRr − SSRur = (Rβ̂ − r)′ [R(X′ X)−1 R′ ]−1 (Rβ̂ − r) Martin / Zulehner: Introductory Econometrics 46 / 52 The F-test Testing hypotheses within the framework of restricted regressions H0 : Rβ̃ = r H1 : Rβ̃ ̸= r where R is a known (q × K) and r a known (q × 1) vector of coecients dening the q linear restrictions Martin / Zulehner: Introductory Econometrics 47 / 52 Examples of Restrictions Some examples of hypotheses i) H0 = βj = 0 ii) H0 = βj = aj iii) H0 = β0 = β1 iv) H0 = β2 + β3 = 1 v) Joint hypothesis β1 0 β2 0 . = . .. . . βK 0 Martin / Zulehner: Introductory Econometrics 48 / 52 Remember that R is a q×K +1 matrix of known constants and r is a q -vector of known constants. The relative restrictions are then i) R = [0,... , 1,... , 0], r = 0, and q = 1 with 1 in the i − th position ii) R = [0,... , 1,... , 0], r = aj , and q=1 with 1 in the i − th position iii) R = [1, −1, 0,... , 0], r = 0, and q=1 iv) R = [0, 0, 1, 1,... , 0], r = 1, and q=1 v) R = [0, IK ], r = 0, and q=K with 0 a vector of K zeros 0 1... 1 0 1... 1 R = ........ .... 0 1... 1 Martin / Zulehner: Introductory Econometrics 49 / 52 The test statistic is then: z = Rβ̂ − r where β̂ is the OLS estimator Exploiting the normality of β̂ z ∼ N(E (z), Var (z)) = N(Rβ − r, σ 2 R(X′ X)−1 R′ ) An under the null hypothesis: z|H0 ∼ N(0, σ 2 R(X′ X)−1 R′ ) Martin / Zulehner: Introductory Econometrics 50 / 52 The F-test The univariate test statistic 1 n = z′ Var (z)−1 z = (Rβ̂ − r)′ [R(X′ X)−1 R′ ]−1 (Rβ̂ − r) σ2 = SSRr − SSRur Under the null hypothesis H0 : n|H0 ∼ χ2q Problem: this test is infeasible, so we dene a modied statistic n/q (SSRr − SSRur )/q (SSRr − SSRur )/q = 1 ′ = v/(N − K − 1) σ2 û û/(N − K − 1 ) (SSR ur )/(N − K − 1) If the statistics n and v are independent then: (SSRr − SSRur )/q ∼ F(q,N−K −1) (SSRur )/N − K − 1 Martin / Zulehner: Introductory Econometrics 51 / 52 Special case: the t-test If we want to test only one coecient, e.g.: H0 = βj = aj vs. H1 = βj ̸= aj Then the F -test becomes (βj − aj )2 ∼ F(1,N−K ) Var d (β̂j ) And it holds that: (βj − aj ) q ∼ t(N−K ) Var d (β̂j ) Martin / Zulehner: Introductory Econometrics 52 / 52