Econometrics Lecture 8: Threats to Identification - 25117 PDF
Document Details
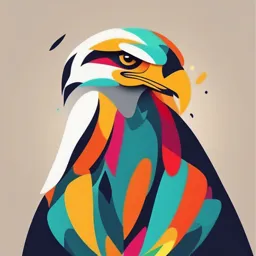
Uploaded by JollyMoldavite4497
Universitat Pompeu Fabra
2024
Tags
Summary
This document is a lecture on econometrics, specifically focusing on the threats to identification. It covers various topics like internal and external validity, with examples and meta-analyses. The lecture notes cover concepts like omitted variable bias, wrong functional form, and more.
Full Transcript
Lecture 8: Threats to Identification 25117 - Econometrics Universitat Pompeu Fabra November 13th, 2024 What we learned in the last lesson - What if the marginal effect of X on Y is not constant? A linear regression is misspecified: the functional form is wrong....
Lecture 8: Threats to Identification 25117 - Econometrics Universitat Pompeu Fabra November 13th, 2024 What we learned in the last lesson - What if the marginal effect of X on Y is not constant? A linear regression is misspecified: the functional form is wrong. - We can extend the multiple OLS framework to introduce non-linearities. The effect on Y of a change in the independent variable(s) can be computed by evaluating the regression function at two values of the independent variable(s). - A polynomial regression includes powers of X as regressors. A quadratic regression includes X and X 2 , and a cubic regression includes X , X 2 , and X 3. - Small changes in logarithms can be interpreted as proportional or percentage changes in a variable. Regressions involving logarithms are used to estimate proportional changes and elasticities. - The product of two variables is called an interaction term. When interaction terms are included as regressors, they allow the regression slope and/or intercept of one variable to depend on the value of another variable. References 2 / 21 Classic Pitfalls to Regression Analysis - Let’s step back and take a broader look at regression. Is there a systematic way to assess (critique) regression studies? We know the strengths of multiple regression – but what are the pitfalls? - We will list the most common reasons that multiple regression estimates, based on observational data, can result in biased estimates of the causal effect of interest. Internal validity The statistical inferences about causal effects are valid for the population being studied. External validity The statistical inferences can be generalized from the population and setting studied to other populations and settings, where the “setting” refers to the legal, policy, and physical environment and related salient features. References 3 / 21 External Validity Assessing threats to external validity requires detailed substantive knowledge and judgment on a case-by-case basis... How far can we generalize class size results from California? −→ Differences in time (California in 1999 vs California in 2023? ) −→ Differences in space (California in 1999 vs Catalonia in 1999?) −→ Differences in settings (Robust across different legal and institutional requirements/frameworks?) Would you expect the magnitude and direction of the effects in the 1999 California schools sample to be the same for Catalonia in 2023? A comparison of many related studies on the same topic is called a meta-analysis. References 4 / 21 A Meta-Analysis – Lane (2016) References 5 / 21 A Meta-Analysis – Hahn-Holbrook et al. (2018) References 6 / 21 Internal Validity Are the statistical inferences about causal effects valid for the population being studied? Five threats to the internal validity of regression studies: - Omitted variable bias - Wrong functional form - Errors-in-variables bias - Sample selection bias - Simultaneous causality bias All of these imply that E(ui | X1 , X2 ,... , Xk ) ̸= 0 (or that the conditional mean independence fails) – in which case O L S is biased and inconsistent! References 7 / 21 Omitted Variable Bias (revision) The bias in the OLS estimator that occurs as a result of an omitted factor, or variable, is called omitted variable bias. For omitted variable bias to occur, the omitted variable Z must satisfy two conditions: 1 Z is a determinant of Y (i.e. Z is part of u); and 2 Z is correlated with the regressor X (i.e., corr (Z , X ) ̸= 0) Omitting Z results in OVB Z X Y If the multiple regression includes control variables, then we need to ask whether there are omitted factors that are not adequately controlled for, that is, whether the error term is correlated with the variable of interest even after we have included the control variables. References 8 / 21 Solutions to OVB - If the omitted causal variable can be measured, include it as an additional regressor in multiple regression; - If you have data on one or more controls and they are adequate (that is, if conditional mean independence plausibly holds), then include the control variables; - If the omitted variable(s) cannot be measured or adequately controlled for, use instrumental variables regression (Lecture 10); - Possibly, use panel data in which each entity (individual) is observed more than once (Lecture 11); - Run a randomized controlled experiment if you can (again, randomization of treatment X ensures that X necessarily will be distributed independently of u.) References 9 / 21 Wrong Functional Form (revision) Arises if the functional form is incorrect – e.g., an interaction term is incorrectly omitted; then inferences on causal effects will be biased. Solutions to functional form mispecification: - Continuous dependent variable: use the “appropriate” nonlinear specifications in X (logarithms, interactions, etc.) (last lecture) - Discrete (example: binary) dependent variable: need an extension of multiple regression methods (“probit” or “logit” analysis for binary dependent variables). (Lecture 12) References 10 / 21 Errors-in-variables So far we have assumed that X is measured without error. In reality, economic data often have measurement error - Data entry errors in administrative data - Recollection errors in surveys (when did you start your current job?) - Ambiguous questions (what was your income last year?) - Intentionally false response problems with surveys (What is the current value of your financial assets? How often do you drink and drive?) Now consider X̃ , the mis-measured version of X (which is the actually observed data) vs X , the true, unobserved value of your treatment... References 11 / 21 Errors-in-variables You think you are running Yi = β0 + β1 Xi + ui But because X is mis-measured, you are actually running Yi = β0 + β1 X̃i + [β1 (X − X̃ ) + ui ] i.e., since X − X̃ is unobserved, Yi = β0 + β1 X̃i + ũi where ũi = β1 (X − X̃ ) + ui.. Therefore, cov (X̃i , ũi ) = β1 cov (X̃i , Xi − X̃i ) + cov (X̃i , ui ) It is often plausible that cov (X̃i , ui ) = 0, but typically cov (X̃i , X − X̃ ) ̸= 0, and therefore, cov (X̃i , ũi ) ̸= 0... yielding a biased βˆ1. References 12 / 21 Classical Measurement Error The classical measurement error model assumes that X̃i = Xi + vi where vi is is mean-zero random noise (e.g., white noise) with ρXi ,vi = 0 and ρui ,vi = 0. Under the classic measurement error model, β̂1 will be biased towards zero... Intuitively: Take the true variable and add a very large amount of random noise. In the limit, X̃i will be completely unrelated to Yi so E(β̂1 ) is zero... Before reaching this limit, the relationship between X̃i and Yi will be toned down, biasing β̂1 towards zero. References 13 / 21 Classical Measurement Error Starting from, cov (X̃i , ũi ) = β1 cov (X̃i , Xi − X̃i ) + cov (X̃i , ui ) Then, for X̃i = Xi + vi , with ρXi ,vi = 0 and ρui ,vi = 0, cov (X̃i , ũi ) = β1 cov (Xi + vi , −vi ) = −β1 σv2 var (X̃i ) = σX2 + σv2 And back to basics: p cov (X̃i , ũi ) β̂1 → β1 + var (X̃i ) σv2 σ2 = β1 − β 1 = β1 2 X 2 σX2 + σv2 σX + σv so β̂1 will be biased towards 0, even in large samples, and even approximate zero if the noise’s variance is large enough. References 14 / 21 Missing Data Data are often missing. Sometimes missing data introduces bias, sometimes it doesn’t. It is useful to consider three cases: 1 Data are missing at random. 2 Data are missing based on the value of one or more X ’s 3 Data are missing based in part on the value of Y or u Cases 1 and 2 don’t introduce bias: the standard errors are larger than they would be if the data weren’t missing but β̂k is unbiased. - Case 1: Suppose you took a random sample of 100 workers and recorded their answers – but your dog randomly ate 20 of the response sheets. This is equivalent to your having taken a simple random sample of 80 workers, so your dog didn’t introduce any bias. - Case 2: In the test scores exercise, suppose you do not observe schools with a share of subsidzied meals < 40%. You cannot draw conclusions for these schools with a share of subsidzied meals < 40%, but that does not prevent you from drawing conclusions about the subset ≥ 40% Case 3, however, introduces “sample selection” bias. References 15 / 21 Sample Selection Bias Sample selection bias Bias that arises when a selection process a) influences the availability of data, and b) is related to the dependent variable beyond depending on the regressors. Fictuous Simple Example: you want to estimate the average males’ height in Barcelona. To collect your data, you go to the Palau Blaugrana and record the heights of the basketball team. Formally, you have sampled individuals in a way that is related to the outcome Y (height), which results in bias. Real Example: In 1936, the Literary Gazette published a poll predicting that presidential candidate Landon would win by a landslide against Roosevelt. The opposite happened: Roosevelt won with 60.8% of the votes. It turned out that the Literary Gazette had sampled phone records from automobile registration files. In 1936, people owning a car and a telephone were richer, and more likely Republicans... supporting Landon. Solution: Avoid it in the first place. If you cannot, some methods for estimating models with sample selection exist (not now, a bit discussed in Lecture 12). References 16 / 21 Simultaneity Bias Simultaneity Bias Also called simultaneous equations bias. It arises in a regression of Y on X when, in addition to the causal link of interest from X to Y , there is a causal link from Y to X. This reverse causality makes X correlated with the error term in the population regression of interest. California Example: The share of subsidised meals affects schools test scores. But schools with higher test scores might be allocated more ressources, allowing them to subsidised more meals. Consider the following system of simultaneous equations: Yi = β0 + β1 Xi + ui Xi = γ0 + γ1 Yi + vi Xi is a function of ui , the unexplained variation of Yi. Solution: Mostly RCT or IV methods (Lecture 10). References 17 / 21 Inconsistent Standard Errors - Inconsistent standard errors can impact the internal validity of a multiple regression study. - Even with consistent OLS estimators and large samples, inconsistent standard errors lead to hypothesis tests with incorrect significance levels and unreliable 95% confidence intervals. - Main reasons for inconsistent standard errors: - Heteroskedasticity: For historical reasons, most regression software report by defaulkt homoskedastic-only standard errors. Use heteroskedasticity-robust standard errors and F-statistics with a heteroskedasticity-robust variance estimator. - Correlation of the Error Term Across Observations: E.g., if the omitted variables that constitute the regression error are persistent (like district demographics or geographies), these omitted variables could result in serial and/or spatial correlation of the regression errors for adjacent observations. Common in panel data or time series data, requires alternative formulas for standard errors. (more in Lecture 11) - Addressing these issues is crucial for maintaining internal validity. References 18 / 21 Internal Validity Checklist for Multiple Variables Regressions Applying this list of threats to a multiple regression study provides a systematic way to assess the internal validity of that study. E(u | X ) ̸= 0 : Inconsistent SE : 1 Omitted variables 1 Heteroskedasticity 2 Functional form misspecification 2 Serial and Spatial Correlation 3 Errors in variables (measurement error in the regressors) 4 Sample selection 5 Simultaneous causality References 19 / 21 What about prediction? Prediction and estimation of causal effects are quite different objectives. For prediction: 1 The data used to estimate the prediction model must be from the same distribution as the out-of-sample observation for which the prediction is made. This is an external validity requirement for a prediction model. 2 The predictors should be ones that substantially contribute to explaining the variation in Y. They do not need to have direct causal interpretations, and the regression coefficients in general need not estimate causal effects. 3 The estimator must be one that produces reliable out-of-sample predictions. When the number of regressors (predictors) is small relative to the number of observations, OLS can be used. But when the number of predictors is large, there are better estimators than OLS – ones developed specially for the prediction problem (Big Data techniques, beyond the scope of this course). References 20 / 21 Material I – Textbooks: - Introduction to Econometrics, 4th Edition, Global Edition, by Stock and Watson – Chapter 9 - Causal Inference, The Mixtape, by Scott Cunningham – Chapters 3 – Papers: - Lane, T. (2016). Discrimination in the laboratory: A meta-analysis of economics experiments. European Economic Review, 90, 375-402. References 21 / 21