Unit 6: Asymptotic Theory & Properties PDF
Document Details
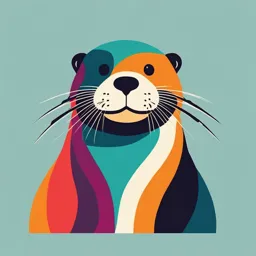
Uploaded by AwesomeCarnelian4810
Martin / Zulehner
Tags
Summary
This document is a set of notes on asymptotic theory and properties in econometrics. It includes topics like large sample properties, consistency, and asymptotic inference. It covers introductory econometrics topics and presents the material in an outline-based format, suitable for student notes.
Full Transcript
Unit 6: Asymptotic theory and properties Martin / Zulehner: Introductory Econometrics 1 / 19 Outline 1 Large sample properties Consistency Asymptotic Inference Martin / Zulehner: Introductory Econometrics 2 / 19 Large sample properties Consistency Under th...
Unit 6: Asymptotic theory and properties Martin / Zulehner: Introductory Econometrics 1 / 19 Outline 1 Large sample properties Consistency Asymptotic Inference Martin / Zulehner: Introductory Econometrics 2 / 19 Large sample properties Consistency Under the Gauss-Markov assumptions OLS is BLUE, but in other cases it won’t always be possible to find unbiased estimators In those cases, we may settle for estimators that are consistent, meaning as N → ∞, the distribution of the estimator collapses to the parameter value this means that the mean of the estimator E [β̂] = β and that the variance of the estimator tends to zero when N tends to infinity Martin / Zulehner: Introductory Econometrics 3 / 19 Sampling distribution as N ↑ Martin / Zulehner: Introductory Econometrics 4 / 19 Consistency of the OLS Estimator Consistency Under the Gauss-Markov assumptions MLR.1-MLR.5, the OLS estimator β̂j is consistent for βj for all j = 1,... , K Conveniently, the same assumptions which imply unbiasedness of the OLS estimators also implies consistency We will need to take the probability limit (plim) to establish consistency We will also assume that the second moments for the X are finite, i.e. plim N1 E (X0 X) = Q a positive definite matrix (or alternatively that Var (xj ) < ∞ for all j = 1,... , K ) Martin / Zulehner: Introductory Econometrics 5 / 19 Proving Consistency - The SLR model The OLS estimator can be written as: PN (xi1 − x̄1 )yi β̂1 = Pi=1 N 2 i=1 (xi1 − x̄1 ) 1 PN (xi1 − x̄1 )ui = β1 + N1 Pi=1N 2 N i=1 (xi1 − x̄1 ) Therefore, by applying the law of large numbers (i.e. the sample moments converge in probability to the population counterparts when N gets larger) plim N1 N P i=1 (xi1 − x̄1 )ui plimβ̂1 = plimβ1 + 1 PN plim N i=1 (xi1 − x̄1 )2 Cov (x1 , u) = β1 + Var (x1 ) = β1 since Cov (x1 , u) = 0 Martin / Zulehner: Introductory Econometrics 6 / 19 Proving Consistency - The MLR model More generally: β̂ = (X0 X)−1 X0 y = (X0 X)−1 X0 (Xβ + u) = β + (X0 X)−1 X0 u 1 1 = β + ( X0 X)−1 X0 u N N Therefore 1 0 1 0 plimβ̂ = plimβ + plim( XX )−1 plim( Xu )=β N | {z } N | {z } → − p E [xi 0 xi ]=Q−1 → − p E [xi ui ]=0 Martin / Zulehner: Introductory Econometrics 7 / 19 Convergence - The full proof The OLS estimator is N 1 0 −1 1 0 1 0 −1 1 X β̂ = β + ( X X) X u = β + ( X X) x i ui N N N N i=1 N 1 0 −1 1 X 1 0 −1 1 =β+( X X) wi = β + ( X X) w N N i=1 N N Now consider the variance Var (w) = E (Var (w|X)) + Var [E (w|X)] | {z } =0 since E (ui |xi )=0 0 1 0 0 1 = E E (ww |X) = E X E (uu )X N N σ 2 X0 X σ2 ! 0 XX =E = E N N N N Hence σ2 X0 X lim Var (w) = lim E =0·Q=0 N→∞ N→∞ N N Hence w converges in mean square to zero, so plimw = 0 and hence plimβ̂ = β Martin / Zulehner: Introductory Econometrics 8 / 19 A Weaker Assumption For unbiasedness, we assumed a zero conditional mean E (u|X) = E (u|x1 , x2 ,... , xk ) = 0 For consistency, we just need the the weaker assumptions of zero mean, E (u) = 0, and zero correlation Cov (xj , u) = E (xj , u) = 0, for j = 1, 2,... , K Without this assumption, OLS will be inconsistent! And: “If you cannot get it right as N goes to infinity, you shouldn’t be in this busi- ness"(Clive W.J. Granger) Notice that we need to assume that Var (u) < ∞ and Var (x1 ) < ∞ but we do not worry about the failing of this assumption Remember, inconsistency is a large sample problem - it does not go away by adding data Martin / Zulehner: Introductory Econometrics 9 / 19 Asymptotic Inference under the CLM assumptions, the sampling distributions are normal, so we could derive t and F distributions for testing I This exact normality was due to assuming the population error distribution was normal I This assumption of normal errors implied that the distribution of y , given the x’s, was normal as well It is easy to come up with examples for which this exact normality assumption will fail I Any clearly skewed variable, like wages, arrests, savings, etc. can not be normal, since a normal distribution is symmetric The normality assumption is not needed to conclude that OLS is BLUE, but only for doing inference (e.g. talking about “statistical significance”) using the Central Limit Theorem, we can show that the OLS estimator is asymptotically normally distributed and derive asymptotic standard errors Martin / Zulehner: Introductory Econometrics 10 / 19 Asymptotic Inference Based on the central limit theorem, we can show that OLS estimators are asymptotically normal Asymptotic normality implies that: P(Z < z) → Φ(z) as N → ∞, or P(Z < z) ≈ Φ(z) More formally: Central Limit Theorem The standardized sample mean of any population with mean µ and variance σ 2 is asymptotically ∼ N(0, 1): Y −µ a Z = √ ∼ N(0, 1) σ/ n Martin / Zulehner: Introductory Econometrics 11 / 19 Asymptotic Normality - I Under the Gauss-Markov assumptions MLR.1-MLR.5, it holds that:1 Asymptotic Normality √ a n β̂j − βj ∼ N(0, σ 2 /aj2 ), where aj2 = plim( N1 N 2 P i=1 rˆij ) 1 2 σ̂ 2 is a consistent estimator of σ 2 3 For each j = 1,... , K β̂j − βj a ∼ N(0, 1) se(β̂j ) 1 Where rˆij are the residuals from regressing xj on all the other independent variables. Martin / Zulehner: Introductory Econometrics 12 / 19 Asymptotic Normality - More generally Asymptotic Normality If ui are i.i.d. with mean zero and finite variance σ 2 and some other (Grenander) conditions on the X variables hold then σ 2 −1 a β̂ ∼ N β, Q N where Q−1 = plim((X 0 X /N)−1 ) Behind these results are various theorems (laws of large numbers for plims, Central Limit Theorems for asymptotic normality) For this course you are not required to be able to prove them nor to be able to derive the asymptotic properties But it is very useful to read the two books and get a clue of what is behind the results (Wooldridge provides only a sketch of the proof in Appendix C, while Greene proves the results in the more general case in Chapter 4.4, pg. 103-108) Martin / Zulehner: Introductory Econometrics 13 / 19 Asymptotic Normality - II Because the t distribution approaches the of normal distribution for large df , we can also say that β̂j − βj a ∼ tN−K −1 se(β̂j ) Hence we can still use our t-test “asymptotically” Note that while we no longer need to assume normality with a large sample, we do still need homoskedasticity. Martin / Zulehner: Introductory Econometrics 14 / 19 Asymptotic Standard Errors If u is not normally distributed, we sometimes will refer to the standard error as an asymptotic standard error, since s σ̂ 2 se β̂j = , SSTj 1 − Rj2 cj se β̂j ≈ √ N where cj is a constant that does not depend on the sample size So, √ we can expect standard errors to shrink at a rate proportional to the inverse of N Martin / Zulehner: Introductory Econometrics 15 / 19 Lagrange Multiplier statistic - I Once we are using large samples and relying on asymptotic normality for inference, we can use more than t and F statistics The Lagrange multiplier or LM statistic is an alternative for testing multiple exclusion restrictions Because the LM statistic uses a so-called “auxiliary regression” it is sometimes called an NR 2 statistic Martin / Zulehner: Introductory Econometrics 16 / 19 LM Statistic - II Suppose we have a model, y = β0 + β1 x1 + β2 x2 +... βK xk + u and our null hypothesis is: H0 : βK −q+1=0 ,... , βK = 0 First, we just run the restricted model: y = β̃0 + β̃1 x1 +... + β̃K −q xK −q + ũ Now take the residuals ũ and regress them on x1 , x2 ,... , xK (i.e. all the variables) Then calculate the statistics LM = NRu2 , where Ru2 comes from that “auxiliary” regression Martin / Zulehner: Introductory Econometrics 17 / 19 LM Statistic - III The distribution of the LM statistic: a LM ∼ /χ2q , thus we can choose a critical value, c from a χ2q distribution, or just calculate a p-value for χ2q With a large sample, the result from an F test and from a LM test should be similar Unlike the F test and t test for one exclusion, the LM test and F test will not be identical Martin / Zulehner: Introductory Econometrics 18 / 19 Asymptotic Efficiency Other estimators besides the OLS are consistent However, under the Gauss-Markov assumptions, the OLS estimators will have the smallest asymptotic variances We say that OLS is asymptotically efficient It is important to remember our assumptions though: if the error term is not homoskedastic, for instance, this conclusion is not true anymore Martin / Zulehner: Introductory Econometrics 19 / 19