Regression Discontinuity - Lecture Notes PDF
Document Details
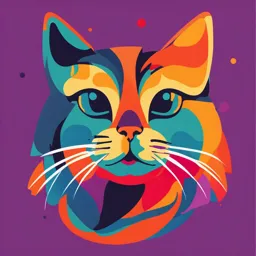
Uploaded by LongLastingGalaxy
Magnus Carlsson
Tags
Summary
These lecture notes, authored by Magnus Carlsson, delve into the concept of regression discontinuity (RD) as a causal inference method. It covers different types of RD models, including sharp and non-parametric variations, and explores its applications utilizing examples like college financial aid and union elections..
Full Transcript
Regression discontinuity 1 Magnus Carlsson Regression discontinuity (RD): A summary A. Sharp RD (this lecture) (i) Non-parametric RD (ii) Parametric RD B. Fuzzy RD (next lecture) (i) Non-parametric RD (ii) Parametric RD Regression discontinuity (RD) Some argue t...
Regression discontinuity 1 Magnus Carlsson Regression discontinuity (RD): A summary A. Sharp RD (this lecture) (i) Non-parametric RD (ii) Parametric RD B. Fuzzy RD (next lecture) (i) Non-parametric RD (ii) Parametric RD Regression discontinuity (RD) Some argue that the RD design may be as close to a randomized experiment as you get in the social sciences The RD design has become very popular during the last decades in areas such as labor economics, crime, education, environment, and health economics The RD design exploits precise knowledge about the rules determining treatment. RD is based on the idea that in a rule-based world, some rules are arbitrary and therefore provides good experiments. The RD design comes in two styles; sharp and fuzzy. RD intro The idea behind RD is very simple The basic idea is to consider some threshold, where very similar people may get very different outcomes depending on the threshold Run a regression based on a situation where you’ve may got a discontinuity in the outcomes at the threshold. Treat above-the-threshold and below-the-threshold like the treatment and control groups in an experiment! RD roadmap Some examples of RD “Sharp” regression discontinuity Parametric or non-parametric RD Threats to the RD design “Fuzzy” RD Using graphs in RD RD literature Chapter 6 in Angrist and Pischke Articles on reading list RD intro: examples Society is full of discontinuity examples: College financial aid in the US: PSAT/NMSQT Basically the top 16,000 test-takers get a scholarship. A small difference in test score can mean a discontinuous jump in scholarship amount. What is the causal effect of scholarship on college enrollment? (van der Klaauw, lER, 2002) RD intro: examples School Class Size: Maimonides’ Rule in Israel — no more than 40 kids in a class. 40 kids in school means 40 kids per class. 41 kids means two classes with 20 and 21. 81 means three classes with....etc etc. What is the causal effect of class size on study results? (Angrist & Lavy, QJE 1999) RD intro: examples Union Elections: If workers want to unionize, NLRB holds election (NLRB: US National Labor Relations Board, federal agency governing relations between unions and employers) 50% means the employer doesn’t have to recognize the union, and 51% means the employer is required to “bargain in good faith” with the union. What is the causal effect of unionization on business survival, employment, output, productivity, and wages? (DiNardo & Lee, QJE 2004) RD intro: example Air Pollution and Home Values: The US Clean Air Act’s National Ambient Air Quality Standards say if the geometric mean concentration of 5 pollutant particulates is 75 micrograms per cubic meter or greater, county is classified as “non-attainment” and are subject to much more stringent regulation. What is the causal effect of pollution on house prices? (Ken Chay, Michael Greenstone, JPE 2005) RD intro: examples What is the problem of investigating the above issues with standard regression analysis? The general problem is obviously that test scores aren’t random, and neither is class size, nor air pollution, etc. But is a kid in the 94.9th percentile of test scores really that different from the 95th percentile kid? Is a school with 40 kids that different from a school with 41? Right around the threshold, there’s a good chance things are random. Sharp RD formally Assume that treatment 𝐷𝑖 is discontinuous function of an underlying continuous variable 𝑥𝑖 Because of an exogenous or institutional rule, a discrete “treatment” 𝐷𝑖 starts prevailing whenever 𝑥𝑖 crosses a particular threshold 𝑥0. The treatment rule is thus: 𝐷𝑖 = 𝐷𝑖 𝑥𝑖 = 1[𝑥𝑖 > 𝑥0 ], where 1[𝑥𝑖 > 𝑥0 ] is an indicator function, assigning the value of one if the condition within the brackets is fulfilled Sharp RD The rules are thus: 𝐷𝑖 = 1 if 𝑥𝑖 > 𝑥0 𝐷𝑖 = 0 if 𝑥𝑖 < 𝑥0 𝐷𝑖 is a treatment indicator 𝑥𝑖 is the “forcing” variable (sometimes also called “assignment” or “running” variable) 𝑥0 is a known threshold Regression discontinuity example (Lee 2008) Incumbency advantage. Lee (2008) studies whether a Democratic candidate for a seat in the US House of Representatives has an advantage if his party won the seat last time. Incumbents may simply be better at satisfying voters or getting the vote out, thereby explaining their higher chances of being re-elected But the success of house incumbents also raises the question whether representatives use the privileges and resources of their office to gain advantage for themselves or their party What is the causal effect of incumbency on the probability of keeping one’s seat in congress? Regression discontinuity example Lee (2008) examine the probability of getting elected as a function of relative vote shares at previous election He exploits the fact that an election winner is determined by: 𝐷𝑖 = 1 if 𝑥𝑖 > 0, 𝐷 = 0 otherwise, (1) where 𝑥𝑖 is the vote share margin of victory (the difference between the vote share between Democrats and Republicans) In this example, 𝑥𝑖 is the forcing variable and 𝐷𝑖 the treatment variable. Regression discontinuity example Graphing: Data 1946-1998 ~10,000 data points Merge points at.005, that is, 10 dots per.05 interval Regression discontinuity example In the example, Figure a plots the probability the a Democrat wins against the difference between Democrats and Republicans in the vote share in previous election The probability is increasing in the difference in vote share but there is also a sharp jump around 0 At 0, there was a win in the previous election and this shifts up the probability of re-election, even if the victory last election was only by one vote Figure b does a “placebo”-type test and shows if reaching the cutoff at time t predicts wins in previous elections RD formalized We can now formalize the sharp RD idea. Start with the regression model: 𝑦𝑖 = 𝛼 + 𝛽𝑥𝑖 + 𝜌𝐷𝑖 + 𝜂𝑖 (2) The key difference between this regression and earlier models is that our treatment indicator 𝐷𝑖 is now a deterministic function of 𝑥𝑖 , i.e another exogenous covariate (the forcing variable). RD captures the causal effect by distinguishing the nonlinear and discontinuous function, 1[𝑥𝑖 > 𝑥0 ], from the smooth and (in this case) linear function, 𝑥𝑖. But why assume a linear function of 𝑥𝑖 ? Consider some hypothetical cases: Sharp RD Instead, allow for flexible, but reasonably smooth, function f (𝑥𝑖 ). We can now re-write our RD model as: 𝑦𝑖 = 𝛼 + f(𝑥𝑖 ) + 𝜌𝐷𝑖 + 𝜂𝑖 (3) If f(𝑥𝑖 ) is continuous in the neighborhood of 𝑥0 , it is possible to estimate this model, even with a flexible functional form for f(𝑥𝑖 ). We can for instance model f(𝑥𝑖 ) with a 𝜌𝑡ℎ -order polynomial, such that: 𝑦𝑖 = 𝛼 + 𝛽1 𝑥𝑖 + 𝛽2 𝑥 2𝑖 + ⋯ + 𝛽𝑝 𝑥 𝑝𝑖 + 𝜌𝐷𝑖 + 𝜂𝑖 (4) Example: Return to Lee (2008): we could for instance write his model as: 𝑦𝑖 = 𝛼 + 𝛽1 𝑥𝑖 + 𝛽2 𝑥 2𝑖 + 𝛽3 𝑥 3𝑖 + 𝜌𝐷𝑖 + 𝜂𝑖 (5) with 𝑥𝑖 denoting the difference in vote share (the forcing variable), and 𝐷𝑖 denoting the treatment (getting elected) Here, the probability of winning is a smooth and flexible function of the difference in vote share We accomplish this by including the square and cube of the difference in vote share Sharp RD ln practice, it is common to allow the forcing variable functions, 𝑓0 (𝑥𝑖 ) and 𝑓1 (𝑥𝑖 ) to differ on each side of the cutoff point: 𝐸 𝑦0𝑖 𝑥𝑖 = 𝛼0 + 𝛽01 𝑥𝑖 + 𝛽02 𝑥 2𝑖 + ⋯ + 𝛽0𝑝 𝑥 𝑝𝑖 (6) 𝐸 𝑦1𝑖 𝑥𝑖 = 𝛼1 + 𝜌 + 𝛽11 𝑥𝑖 + 𝛽12 𝑥 2𝑖 + ⋯ + 𝛽1𝑝 𝑥 𝑝𝑖 (7) where 𝑥𝑖 = 𝑥𝑖 − 𝑥0. Sharp RD A more direct way of estimating the treatment effect is to run a pooled regression on both sides of the cutoff, including interaction terms: 𝑦𝑖 = 𝛼 + 𝛽01 𝑥𝑖 + 𝛽02 𝑥 2𝑖 + ⋯ + 𝛽0𝑝 𝑥 𝑝𝑖 + 𝜌𝐷𝑖 + 𝛽 1∗ 𝐷𝑖 𝑥𝑖 + 𝛽 2∗ 𝐷𝑖 𝑥 2𝑖 + 𝛽 𝑝∗ 𝐷𝑖 𝑥 𝑝𝑖 (8) ln practice, however, it often does not seem to matter much if we constrain the forcing variable functions to be the same or not at each side of the cutoff Sharp RD. A concern for the (near) future Problem if too flexible on both sides, since then the functional form has a potential to ”create” a discontinuity Gelman & Imbens (NBER, 2015) Why High-order Polynomials Should not be Used in Regression Discontinuity Designs Solution – always do linear, square and higher, local fits……. Alternative sharp RD estimator As shown above, the validity of the RD estimates depends on the correct specification of the forcing variable This is thus an example of parametric RD where the choice of functional form for the forcing variable function is crucial What looks like a jump due to treatment may simply be some unaccounted-for nonlinearity in the forcing variable function To deal with such problems, we can instead look at data only very close to the discontinuity! Non-parametric RD Using data only very close to the discontinuity also nicely captures the intuition of the RD-design. The idea is that people who are just above or below the threshold will be similar in both observed and unobserved ways, with the exception that some are “treated, i.e. exposed to the threshold We can thus look at data in a neighborhood around the discontinuity, say the interval 𝑥0 − 𝛿, 𝑥0 + 𝛿 for some small number 𝛿: 𝐸 𝑦𝑖 𝑥0 − 𝛿 < 𝑥𝑖 < 𝑥0 ~𝐸 𝑦0𝑖 𝑥𝑖 = 𝑥0 (9) 𝐸 𝑦𝑖 𝑥0 < 𝑥𝑖 < 𝑥0 + 𝛿 ~𝐸 𝑦1𝑖 𝑥𝑖 = 𝑥0 (10) Non-parametric RD Our non-parametric RD-estimate can then be written as: 𝑙𝑖𝑚 𝛿→0 𝐸 𝑦𝑖 𝑥0 < 𝑥𝑖 < 𝑥0 + 𝛿 − 𝐸 𝑦𝑖 𝑥0 − 𝛿 < 𝑥𝑖 < 𝑥0 = 𝐸 𝑦1𝑖 − 𝑦0𝑖 𝑥𝑖 = 𝑥0 (11) Here, we compare average outcomes in a small enough neighborhood to the left and right of 𝑥0. This should provide an estimate of the treatment effect that does not depend on the correct specification of a model 𝑓(𝑥𝑖 ). ln principle, this boils down to comparing the average outcome of people at each side of the threshold Example of non-parametric RD Consider Lee (2008) again... Here, 𝐸 𝑦1𝑖 − 𝑦0𝑖 𝑥𝑖 = 𝑥0 would mean comparing the probability of re-election for candidates whose party lost or won the last election by a very small margin For instance, we could in principle compare the probability of winning for candidates who won or lost by just 1 vote in the last election With such small differences, it seems highly likely that candidates on either side of the threshold would be very similar Non-parametric RD One problem with the non-parametric RD is the data requirements We only want observations very close to the threshold, but these may be few We can include more observations at each side of the threshold by stretching the bandwidth, i.e. increasing 𝛿 This means however that we start comparing observations that lie further and further from the threshold, but such observations may then become less and less comparable Parametric or non-parametric RD? Due to data limitations, parametric RD may thus be the only option The idea of focusing on observations near the cutoff value could still be a valuable robustness check however While RD estimates get less precise as the window used to select a discontinuity sample gets smaller, the number of polynomial terms needed to model 𝑓(𝑥𝑖 ) should go down as well. As one zeros in on 𝑥0 , the estimated effect of 𝐷𝑖 should remain stable Threats to the RD design, “Manipulation” Example: ln the Netherlands employers can claim an additional tax deduction if a worker above age 40 follows on-the-job training or schooling. A researcher is interested if these type of financial incentives increase the incidence of training workers. Entitlement to financial incentives depends only on age, yielding a sharp regression-discontinuity design such that: 1 𝑖𝑓 𝑆𝑖 > 𝑆 𝐷𝑖 = ቐ 0 𝑖𝑓 𝑆𝑖 < 𝑆 In the example, 𝑆𝑖 is the age of individual 𝑖 and 𝑆 is 40 years old. How would you expect employers to behave? Threats to the RD design Training incidence by age in the Dutch population: Why a drop at just 39? A decrease before treatment rather than an increase after treatment… Threats to the RD design Regression: 𝑦𝑖 = 𝛽0 + 𝛽1 𝐷𝑖 40 + + 𝛽2 𝐴𝑔𝑒𝑖 + 𝛽3 𝐴𝑔𝑒 2𝑖 + 𝑈𝑖 Coeff. St.err 𝛽0 1.02 (0.35) 𝛽1 0.08 (0.05) 𝛽2 -0.02 (0.02) 𝛽3 0.0002 (0.0002) Use the difference-in-means estimator for the interval 39 − ε, 39 and 40,40 + ε. 𝜀 MTE St.err Sample 0 0.17 (0.07) 204 1 0.13 (0.05) 385 2 0.07 (0.04) 538 3 0.05 (0.04) 685 4 0.05 (0.04) 840 Threats of the RD design As the example shows, the RD design can be invalid if individuals can precisely manipulate or act on the “assignment variable”. ln general, if there is a payoff or benefit to receiving a treatment, it is natural for an economist to consider how an individual may behave to obtain such benefits. For example, if students could effectively “choose” their test score 𝑋 through effort, those who chose a score 𝑥0 (and hence receive a merit award) could be somewhat different from those who chose scores just below 𝑥0 Notes about the sharp RD design ln the RD approach, there is in principle no need for control variables other 2 than the “forcing” variable 𝑥𝑖 (and any transformations of it, such as 𝑥 𝑖 ) The reason is that there should not be important differences in other covariates right around the cutoff point 𝑥0. If the values of the covariates on the two sides of the cutoff were very different, this would suggest that the RD design is invalid. A useful test of the internal validity of the RD design is therefore to replace the outcome variable 𝑦𝑖 with some covariate If there is an effect on the covariate at the threshold, the RD design may be invalid