Introduction au Machine Learning (Support) - VISIPLUS Academy
Document Details
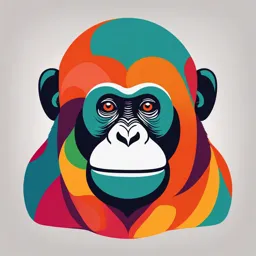
Uploaded by ReasonedFlute
VISIPLUS Academy
Tags
Related
- Grundlagen maschinelles Lernens PDF
- Priručnik za pripremu prijemnog ispita iz veštačke inteligencije za master studije Softversko inženjerstvo i veštačka inteligencija PDF
- Python Unit 1 Notes - Introduction to Python & Clean Coding PDF
- Introduction to Network and Web Application PDF
- Chapter 1 - Introduction to Machine Learning PDF
- ISTQB CT-AI Syllabus v1.0 PDF
Summary
This document provides an introduction to machine learning, covering topics such as its concepts and trends. It also includes information about various machine learning jobs and their specific tasks.
Full Transcript
SI Ac P Page 1 LU ad VI S e au...
SI Ac P Page 1 LU ad VI S e au Learning SI Ac P au Machine Introduction LU ad S em Learning Ac y a Machine Introduction U de VI S m Ac y ad VI em SI y PL U de VI S m SI y PL U VI S SI Ac P LU ad IS S em IP Ac L U ad S em A y SI Ac P ad Page 2 LU VI S e Learning SI Ac P au Machine Introduction LU ad S em Ac y a U de VI S m Ac y ad VI em SI y PL U de VI S Chapitre 1 : m SI y PL U VI S en pleine expansion Le Machine Learning SI Ac P LU ad IS S em IP Ac L U ad S em A y SI Ac P ad Page 3 LU VI S e Learning SI Ac P au Machine Introduction LU ad S em Ac y a U de VI S m Ac y ad VI em SI y PL U de VI S m SI y PL U VI S Tendances SI Ac P LU ad IS S em IP Ac L U ad S em A y e em VI S ad U ad Cas d’usage Ac PL y Ac em SI y em VI S ad Banque et U E-commerce Plateformes de ad secteur Ac PL et marketing contenu financier y Ac m S SI de LU Introduction VI S a LU au Machine P Ac SI Learning y P em S SI A Bâtiment Véhicule Génération de LU intelligent autonome contenu VI S Page 4 ad U P Ac L SI y IP m VI S IS de U SI Ac P ad Page 5 LU VI S e Learning SI Ac P au Machine Introduction LU ad S em Ac y a U de VI S m Ac y ad VI em SI y PL U de VI S m SI y PL U VI S SI Ac P « The sexiest job » LU ad IS S em IP Ac L U ad S em A y e em VI S ad U Les métiers ad Ac PL y Ac em SI y em VI S ad U Machine Learning Deep Learning ad Ac Data Scientist PL Engineer Engineer y Ac m S SI de LU Introduction VI S a LU au Machine P Ac SI Learning y P em S SI A LU VI S Page 6 ad Data Engineer Data Analyst U P Ac L SI y IP m VI S IS de U SI Ac P ad Page 7 LU VI S e Learning SI Ac P au Machine Introduction LU ad S em Ac y a U de VI S m Ac y ad VI em SI y PL U de VI S m SI y PL U VI S Les métiers SI Ac P LU ad IS S em IP Ac L U ad S em A y SI Ac P ad Page 8 LU VI S e Learning SI Ac P au Machine Introduction LU ad S em Ac y a U de VI S m Ac y ad VI em SI y PL U de VI S m SI y PL U VI S Les métiers SI Ac P LU ad IS S em IP Ac L U ad S em A y e em VI S ad U ad Ac Ce qu’il faut retenir PL y Ac em SI y em VI S ad Le Machine Learning est à la mode U ad Ac PL y Beaucoup de demandes sur le marché Ac m S SI de l’emploi de LU Introduction VI S Une grande diversité de métiers a LU au Machine P Ac SI Learning y P em S SI A LU VI S Page 9 ad U P Ac L SI y IP m VI S IS de U SI Ac P LU ad Page 10 VI S e Learning SI Ac P au Machine Introduction LU ad S em Ac y a U de VI S m Ac y ad VI em SI y PL U de VI S m Chapitre 2 : SI y PL U machine learning VI S SI Ac P Découvrir les concepts du LU ad IS S em IP Ac L U ad S em A y e em VI S ad U ad Ac PL y Intelligence artificielle Ac em SI y em VI S ad L'intelligence artificielle (IA) est U un « ensemble de théories et de techniques ad Ac mises en œuvre en vue PL y de réaliser des machines capables de simuler Ac m l'intelligence humaine » S SI de LU Introduction VI S a Wikipédia LU au Machine P Ac SI Learning y P em S SI A LU VI S Page 11 ad U P Ac L SI y IP m VI S IS de U e em VI S ad U ad Ac PL y Machine Learning Ac em SI y em VI S ad « L'apprentissage automatique est un champ U d'étude de l'intelligence artificielle qui se fonde sur ad Ac PL des approches mathématiques et statistiques pour y donner aux ordinateurs la capacité d'apprendre à Ac m S SI partir de données [...] résoudre des tâches sans être de explicitement programmés pour chacune. » LU Introduction VI S Wikipédia a LU au Machine P Ac SI Learning y P em S SI A LU VI S Page 12 ad U P Ac L SI y IP m VI S IS de U SI Ac P ad Page 13 LU VI S e Learning SI Ac P au Machine Introduction LU ad S em Ac y a U de VI S m Ac y ad VI em SI y PL U de VI S m SI y PL U VI S IA > ML > DL SI Ac P LU ad IS S em IP Ac L U ad S em A y SI Ac P ad Page 14 LU VI S e Learning SI Ac P au Machine Introduction LU ad S em Ac y a U de VI S m Ac y ad VI em SI y PL U de VI S m SI y PL U VI S SI Ac P LU ad S em Machine Learning : utilisation IS Ac IP L U ad S em A y e em VI S ad U ad Ac Les familles du Machine Learning PL y Ac em SI y em VI S ad U ad Ac Supervisé Non supervisé PL y Ac Valeur cible connue Pas de valeur cible m S SI de LU Introduction VI S a LU au Machine P Ac Prix d’une maison Clustering de base SI Learning clients y P em Détection de SPAM Réduction de S SI A … dimensions LU VI S Page 15 ad U P Ac L SI y IP m VI S IS de U e em VI S ad U ad Ac PL y Les autres types de Machine Learning Ac em SI y em VI S ad U ad Ac PL y Ac m S SI de LU Introduction VI S a RL : LU au Machine Reinforcement Semi-Supervised Self-Supervised P Ac Learning Learning Learning SI Learning y P em S SI A LU VI S Page 16 ad U P Ac L SI y IP m VI S IS de U e em VI S ad U ad Ac Ce qu’il faut retenir PL y Ac em SI y em VI S ad Le Machine Learning est un type U ad Ac d’intelligence artificielle PL y Ac m S SI Le ML diffère de la programmation de LU traditionnelle Introduction VI S Le programme est généré a LU au Machine P Ac SI Learning y On distingue deux grandes familles : P em S SI A apprentissage supervisé LU apprentissage non supervisé VI S Page 17 ad U P Ac L SI y IP m VI S IS de U SI Ac P ad Page 18 LU VI S e Learning SI Ac P au Machine Introduction LU ad S em Ac y a U de VI S m Ac y ad VI em SI y PL U de VI S m Chapitre 3 : SI Appréhender y PL U VI S SI Ac P l’apprentissage supervisé LU ad IS S em IP Ac L U ad S em A y e em VI S ad U ad Ac PL y Les données Ac em SI y em VI S ad Longueur Largeur Type Pointure du Code postal Prix U propriétaire ad Ac PL y 10 10 Maison 38 31 000 200 000 Ac m S SI 20 Maison 44 31 200 000 de LU Introduction VI S a LU 5 10 Appartement H 31 400 150 000 au Machine P Ac SI Learning y P 8 7 000 T5 31 400 140 k em S SI A LU VI S Page 19 ad U P Ac L SI y IP m VI S IS de U e em VI S ad U ad Ac PL y Les données Ac em SI y em VI S ad Longueur Largeur Type Pointure du Code postal Prix U propriétaire ad Ac PL y 10 10 Maison 38 31 000 200 000 Ac m S SI 20 Maison 44 31 200 000 de LU Introduction VI S a LU 5 10 Appartement H 31 400 150 000 au Machine P Ac SI Learning y P 8 7 000 T5 31 400 140 k em S SI A LU VI S Page 20 ad U Échantillon / Ligne P Ac Variable / Colonne L / Observation Cible / Target SI y / Feature IP / Individu m VI S IS de U e em VI S ad U ad Ac PL y Les étapes Ac em SI y em VI S ad U Training Training ad Ac New Features PL Features Target y Ac m S SI de LU Introduction VI S Model a LU au Machine Model + P Ac Rules SI Learning y P em S SI A LU VI S Page 21 ad Rules Predicted output U P Ac L SI y IP Entrainement m Inférence VI S IS de U SI Ac P LU ad Page 22 VI S e Learning SI Ac P au Machine Introduction LU ad S em Ac y a U de VI S m Ac y ad VI em SI y PL U de VI S m SI y PL U VI S SI Corrélation ≠ causalité Ac P LU ad Prédire n’est pas expliquer IS S em IP Ac L U ad S em A y SI Ac P LU ad Page 23 VI S e Learning SI Ac P au Machine Introduction LU ad S em Ac y a U de VI S m Ac y ad VI em SI y PL U de VI S m SI y PL U VI S SI Ac P LU ad Prédire n’est pas expliquer IS S em IP Ac L U ad S em A y e em VI S ad U ad Ac Apprentissage supervisé PL y Ac em SI y em VI S ad U ad Ac PL y Ac m S SI Classification Régression de LU Introduction VI S a LU au Machine P Une classe parmi un Ac Un nombre continu SI Learning nombre fini de classes y P em S SI A LU VI S Page 24 ad U P Ac L SI y IP m VI S IS de U e em VI S ad U ad Ac Ce qu’il faut retenir PL y Ac em SI y em VI S ad Les données U Échantillons, colonnes et cible ad Ac PL y Ac Une première phase d’entraînement m S SI Suivie d’une phase d’exploitation de LU Introduction VI S Faire des prédictions n’est pas expliquer a LU au Machine P Ac SI Learning Il y a deux catégories de problèmes y P supervises : em S SI A Classification LU Régression VI S Page 25 ad U P Ac L SI y IP m VI S IS de U e em VI S ad U ad Ac PL y Ac em SI y em VI S ad Chapitre 4 : U ad Ac PL y Visualiser le cycle de vie Ac m S SI de LU Introduction d’un projet VI S a LU au Machine P Ac de Machine Learning SI Learning y P em S SI A LU VI S Page 26 ad U P Ac L SI y IP m VI S IS de U SI Ac P LU ad Page 27 VI S e Learning SI Ac P au Machine Introduction LU ad S em Ac y a U de VI S m Ac y ad VI em SI y PL U de VI S m SI y PL U VI S SI Ac P LU ad Le cycle de vie d’un projet IS S em IP Ac L U ad S em A y e em VI S ad U ad Ac Ce qu’il faut retenir PL y Ac em SI y em VI S ad Un projet de Machine Learning est un U ad Ac processus itératif PL y Ac m S SI Comprendre les enjeux « business » est de LU primordial Introduction VI S a LU au Machine P Ac Analyser pour décider la prochaine SI Learning y étape à traiter est crucial P em S SI A LU VI S Page 28 ad U P Ac L SI y IP m VI S IS de U SI Ac P LU ad Page 29 VI S e Learning SI Ac P au Machine Introduction LU ad S em Ac y a U de VI S m Ac y ad VI em SI y PL U de VI S m Chapitre 5 : SI y PL U S Mettre en place son VI SI Ac P environnement de travail LU ad IS S em IP Ac L U ad S em A y SI Ac P LU ad Page 30 VI S e Learning SI Ac P au Machine Introduction LU ad S em Ac y a U de VI S m Ac y ad VI em SI y PL U de VI S m SI y PL U VI S Démonstration n°01 SI Ac P LU ad IS S em IP Ac L U ad S em A y e em VI S ad U ad Ac Ce qu’il faut retenir PL y Ac em SI y em VI S ad Jupyter est un environnement U ad Ac permettant de coder à travers des PL y notebooks Ac m S SI de LU Un notebook est constitué de cellules Introduction VI S de code et de texte a LU au Machine P Ac SI Learning y P em S SI A LU VI S Page 31 ad U P Ac L SI y IP m VI S IS de U SI Ac P LU ad Page 32 VI S e Learning SI Ac P au Machine Introduction LU ad S em Ac y a U de VI S m Ac y ad VI em SI y PL U de VI S m Chapitre 6 : SI y PL U Prendre en main Python et Numpy VI S SI Ac P LU ad IS S em IP Ac L U ad S em A y SI Ac P LU ad Page 33 VI S e Learning SI Ac P au Machine Introduction LU ad S em Ac y a U de VI S m Ac y ad VI em SI y PL U de VI S m SI y PL U VI S Démonstration n°02 SI Ac P LU ad IS S em IP Ac L U ad S em A y e em VI S ad U ad Ac Ce qu’il faut retenir PL y Ac em SI y em VI S ad Numpy est une bibliothèque de calculs U ad Ac matriciels extrêmement puissante PL y Ac m S SI Privilégier Numpy et délaisser un de LU maximum les boucles « for » Introduction VI S traditionnelles a LU au Machine P Ac SI Learning y L’élément de base est le ndarray P em S SI A LU VI S Page 34 ad U P Ac L SI y IP m VI S IS de U SI Ac P LU ad Page 35 VI S e Learning SI Ac P au Machine Introduction LU ad S em Ac y a U de VI S m Ac y ad VI em SI y PL U S Pandas de VI m Chapitre 7 : SI y PL U VI S SI Ac P LU ad IS S em IP Ac L U ad S em A y SI Ac P LU ad Page 36 VI S e Learning SI Ac P au Machine Introduction LU ad S em Ac y a U de VI S m Ac y ad VI em SI y PL U de VI S m SI y PL U VI S Démonstration n°03 SI Ac P LU ad IS S em IP Ac L U ad S em A y e em VI S ad U ad Ac Ce qu’il faut retenir PL y Ac em SI y em VI S ad Pandas est une bibliothèque U ad Ac s’appuyant sur Numpy PL y Ac m S SI Elle permet de traiter des données de LU tabulaires de manière pratique et Introduction VI S efficace a LU au Machine P Ac SI Learning y L’élément de base est le DataFrame P em S SI A LU VI S Page 37 ad U P Ac L SI y IP m VI S IS de U SI Ac P LU ad Page 38 VI S e Learning SI Ac P au Machine Introduction LU ad S em Ac y a U de VI S m Ac y ad VI em SI y PL U de VI S m Scikit-learn Chapitre 8 : SI y PL U VI S SI Ac P LU ad IS S em IP Ac L U ad S em A y SI Ac P LU ad Page 39 VI S e Learning SI Ac P au Machine Introduction LU ad S em Ac y a U de VI S m Ac y ad VI em SI y PL U de VI S m SI y PL U VI S Scikit-learn SI Ac P LU ad IS S em IP Ac L U ad S em A y SI Ac P LU ad Page 40 VI S e Learning SI Ac P au Machine Introduction LU ad S em Ac y a U de VI S m Ac y ad VI em SI y PL U de VI S m SI y PL U VI S SI Ac P Scikit-learn – v1.2 LU ad IS S em IP Ac L U ad S em A y e em VI S ad U Les autres bibliothèques ad Ac PL y Ac em SI y Deep Learning em Préparation VI S ad U ad Ac PL y Ac m S SI de LU Introduction VI S a LU au Machine P Ac SI Learning y P Typologie em S SI A Modèles LU VI S Page 41 ad U P Ac L SI y IP m VI S IS de U e em VI S ad U ad Ac PL y Ac em SI y em VI S ad U ad Ac Démonstration n°04 : PL y Ac m S SI de https://scikit- LU Introduction VI S a LU au Machine learn.org/stable/index.html P Ac SI Learning y P em S SI A LU VI S Page 42 ad U P Ac L SI y IP m VI S IS de U e em VI S ad U ad Ac Ce qu’il faut retenir PL y Ac em SI y em VI S ad Scikit-Learn est la bibliothèque U ad Ac incontournable de Machine Learning PL y Ac m S SI Pour des tâches plus spécialisées, de LU l’écosystème Python dispose Introduction VI S (forcément) d’une bibliothèque dédiée a LU au Machine P Ac SI Learning y P em S SI A LU VI S Page 43 ad U P Ac L SI y IP m VI S IS de U SI Ac P LU